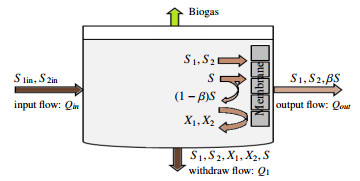
As of April 2021, the coronavirus disease (COVID-19) continues to spread in Japan. To overcome COVID-19, the Ministry of Health, Labor, and Welfare of the Japanese government developed and released the COVID-19 Contact-Confirming Application (COCOA) on June 19, 2020. COCOA users can know whether they have come into contact with infectors. If persons who receive a contact notification through COCOA undertake self-quarantine, the number of infectors in Japan will decrease. However, the effectiveness of COCOA in reducing the number of infectors depends on the usage rate of COCOA, the rate of fulfillment of contact condition, the rate of undergoing the reverse transcription polymerase chain reaction (RT-PCR) test, the false negative rate of the RT-PCR test, the rate of infection registration, and the self-quarantine rate. Therefore, we developed a Susceptible-Infected-Removed (SIR) model to estimate the effectiveness of COCOA. In this paper, we introduce the SIR model and report the simulation results for different scenarios that were assumed for Japan.
Citation: Yuto Omae, Yohei Kakimoto, Jun Toyotani, Kazuyuki Hara, Yasuhiro Gon, Hirotaka Takahashi. SIR model-based verification of effect of COVID-19 Contact-Confirming Application (COCOA) on reducing infectors in Japan[J]. Mathematical Biosciences and Engineering, 2021, 18(5): 6506-6526. doi: 10.3934/mbe.2021323
[1] | Marion Weedermann . Analysis of a model for the effects of an external toxin on anaerobic digestion. Mathematical Biosciences and Engineering, 2012, 9(2): 445-459. doi: 10.3934/mbe.2012.9.445 |
[2] | Andrés Donoso-Bravo, Pedro Gajardo, Matthieu Sebbah, Diego Vicencio . Comparison of performance in an anaerobic digestion process: one-reactor vs two-reactor configurations. Mathematical Biosciences and Engineering, 2019, 16(4): 2447-2465. doi: 10.3934/mbe.2019122 |
[3] | Tewfik Sari, Miled El Hajji, Jérôme Harmand . The mathematical analysis of a syntrophic relationship between two microbial species in a chemostat. Mathematical Biosciences and Engineering, 2012, 9(3): 627-645. doi: 10.3934/mbe.2012.9.627 |
[4] | Amer Hassan Albargi, Miled El Hajji . Mathematical analysis of a two-tiered microbial food-web model for the anaerobic digestion process. Mathematical Biosciences and Engineering, 2023, 20(4): 6591-6611. doi: 10.3934/mbe.2023283 |
[5] | Wanxiao Xu, Ping Jiang, Hongying Shu, Shanshan Tong . Modeling the fear effect in the predator-prey dynamics with an age structure in the predators. Mathematical Biosciences and Engineering, 2023, 20(7): 12625-12648. doi: 10.3934/mbe.2023562 |
[6] | Juan Wang, Chunyang Qin, Yuming Chen, Xia Wang . Hopf bifurcation in a CTL-inclusive HIV-1 infection model with two time delays. Mathematical Biosciences and Engineering, 2019, 16(4): 2587-2612. doi: 10.3934/mbe.2019130 |
[7] | Szymon Sobieszek, Matthew J. Wade, Gail S. K. Wolkowicz . Rich dynamics of a three-tiered anaerobic food-web in a chemostat with multiple substrate inflow. Mathematical Biosciences and Engineering, 2020, 17(6): 7045-7073. doi: 10.3934/mbe.2020363 |
[8] | Dongfu Tong, Yongli Cai, Bingxian Wang, Weiming Wang . Bifurcation structure of nonconstant positive steady states for a diffusive predator-prey model. Mathematical Biosciences and Engineering, 2019, 16(5): 3988-4006. doi: 10.3934/mbe.2019197 |
[9] | Andrea Franceschetti, Andrea Pugliese, Dimitri Breda . Multiple endemic states in age-structured SIR epidemic models. Mathematical Biosciences and Engineering, 2012, 9(3): 577-599. doi: 10.3934/mbe.2012.9.577 |
[10] | G.A.K. van Voorn, D. Stiefs, T. Gross, B. W. Kooi, Ulrike Feudel, S.A.L.M. Kooijman . Stabilization due to predator interference: comparison of different analysis approaches. Mathematical Biosciences and Engineering, 2008, 5(3): 567-583. doi: 10.3934/mbe.2008.5.567 |
As of April 2021, the coronavirus disease (COVID-19) continues to spread in Japan. To overcome COVID-19, the Ministry of Health, Labor, and Welfare of the Japanese government developed and released the COVID-19 Contact-Confirming Application (COCOA) on June 19, 2020. COCOA users can know whether they have come into contact with infectors. If persons who receive a contact notification through COCOA undertake self-quarantine, the number of infectors in Japan will decrease. However, the effectiveness of COCOA in reducing the number of infectors depends on the usage rate of COCOA, the rate of fulfillment of contact condition, the rate of undergoing the reverse transcription polymerase chain reaction (RT-PCR) test, the false negative rate of the RT-PCR test, the rate of infection registration, and the self-quarantine rate. Therefore, we developed a Susceptible-Infected-Removed (SIR) model to estimate the effectiveness of COCOA. In this paper, we introduce the SIR model and report the simulation results for different scenarios that were assumed for Japan.
Mathematical modeling of bioprocesses is a powerful tool to (ⅰ) explain observed phenomena, (ⅱ) understand some mechanisms of the system and predict its evolution, (ⅲ) better control the process operations and (ⅳ) build the "roots" for dialogue and discussion with biologists. In recent years, many studies were carried out on the mathematical models analysis of biological ecosystems using chemostat. A number of mathematical modeling methods that are relevant to the field of microbial ecology and bioprocesses was presented in [1]. Di and Yang [2], evaluated how structures and parametrization of synthetic microbial communities with two or three species could affect their productivity and stability. Qualitative analysis of local and global stability of steady states of a syntrophic relationship between two consortium of bacteria in a chemostat is detailed in [3,4].
The technology of Anaerobic Digestion is highly promising with the potential to substantially improve efficiency in wastewater treatment, digestate handling and bioenergy production. Anaerobic digestion is a complex process, which is widely described by the most complete model ADM1 (Anaerobic Digestion Model n.1) [5]. Because of its high complexity and strong non-linearity, ADM1 cannot be used for analytical analysis of the steady states of the system. In the literature, a number of studies have been made on equilibria and the nature of their stability of reduced and simplified models of anaerobic digestion processes using operating diagram analysis, which allows to describe the behavior of the system with respect to the control parameters. Khedim et al. [6], investigated how operating parameters (dilution rate and substrate inflow concentration) could ensure an optimal production of biogas in a Microalgae Anaerobic Digestion process. As regards [7], authors showed that the stability of the positive equilibrium of a two-tiered microbial food-chain is not affected when maintenance is included in the model and for a large class of kinetics. A generalised form of a three-tiered microbial food-web was proposed in [8]; when maintenance is not considered in the model, it was shown that one can explicitly determine the stability of the system and, boundaries between the different stability regions are characterized by analytical expressions.
A review of mathematical modeling of anaerobic digestion with respect to the theory, applications and technologies is given in [9], where it is argued that mathematical analysis tools can be appropriately applied to reduced-order models of anaerobic digestion to investigate the qualitative behavior of the system. Even if modeling of anaerobic digestion is increasing in complexity and new challenges should be addressed [10], for a simplified modeling, the biological process may be described mainly by two-steps reactional framework as given in [11]: in the first step (acidogenesis), the acidogenic bacteria consume the organic substrate and produce Volatile Fatty Acids (VFA) and CO2, while in the second step (methanogenesis), the methanogenic population consumes VFA and produces methane and CO2. A well known model for such process is the AM2 model [11] which has four main variables (two substrates and two microbial populations). In [12], it is shown that this model of two reactions represents 97.8% of biological variability, which justifies its choice to describe the main mass transfer within the bioreactor. An extended version of the AM2 model was used in [13] to predict biogas and methane production rates. Also, AM2 was compared to the ADM1 and it was shown that a tradeoff has to be made between model complexity and tractability. The AM2 model can successfully support on-line control and supervision strategies, based on state observers and feedback control [14,15]. These literature examples of some applications of the AM2 model, show that this simple model is able to predict the main dynamical behavior of ADM1, which would be considered as a virtual anaerobic bioreactor for simulation.
Many mathematical studies were carried out on the qualitative behavior of the AM2 model in generic cases [16,17], or in particular cases [18,19,20]. It is shown in [16] that the AM2 model can have at most six equilibria and it can have a monostability or a bistability behavior, according to the functioning conditions. A comparison of performance of one-reactor vs two-reactors configurations for a two-reaction (acidogenesis and methanogenesis) anaerobic digestion model were discussed in [21]. Using the AM2 model, authors have proven that separation of the reactions in two bioreactors does not improve the stability of the process nor the soluble organic matter removal capacity. Weedermann studied the effects of an external toxin on the bahavior of a two-step model of anaerobic digestion [22]. He showed under what conditions the toxin can alter the steady states of the system (wash-out of bacteria, fluctuations (limit cycles) or bistabilities).
Even if the AM2 model has proven its usefulness for the control and supervision of anaerobic digesters, it remains a very simple model, which would not able to explain certain biological phenomena as the dynamics of lower concentrations or small bacteria populations [23] and, which has a limited applicability as typically the case for anaerobic digestion of waste-activated sludge [24]. This is why more or less extented versions of AM2 have been proposed in the literature in order to better describe anaerobic digestion processes with the integration of new main variables, while remaining simple from a mathematical modeling point of view. For instance in [24], one proposed the AM2HN model, which is a modification of AM2 by adding one additional state variable XT (total particulate substrate), i.e., one additional differential equation in order to include the disintegration/hydrolysis step and, initial differential equations of AM2 was accordingly modified. The model proposed in [22] is exactly a perturbation of the AM2 model to study the effects of an externally introduced toxin. Author added one differential equation of the dynamic of the toxin T, which inhibits the growth of bacteria X1 while it is broken by bacteria X2.
A model for anaerobic membrane digesters has been proposed in [25] for control design purposes. This model named AM2b is based on the modification of the two step model AM2 and integrates the dynamic of a new variable (SMP: Soluble Microbial Products) in the system. Recently, the AM2b model was combined with a simple fouling models to describe both biological and membrane dynamics in an Anaerobic Membrane BioReactors (AnMBR) [26,27] and to assess system performance and membrane fouling [28]. A state-of-the-art on coupling of membrane fouling models with biological dynamics is provided in [29]. Authors reviewed modeling and control aspects of AnMBR and, focused on existing challenges and future perspectives to improve them. Stochastic versions of the AM2b model was proposed in [30] and [31], to provide a deeper description of the process when modeling lower concentrations or small bacteria populations, which can be seen as uncertain and noisy dynamics.
It is shown in [25] that the AM2b model is highly sensitive to the maximum growth rate of acidogenic bacteria on SMP (which is considered as a bifurcation parameter). This paper is complementary to [25] and it proposes a detailed mathematical analysis of the qualitative behavior of the model AM2, especially with respect to the bifurcation parameter. It reports briefly some results which presented in [25] and, gives pertinently their mathematical backgrounds. The paper is structured as follows: first, we recall the AM2b model and we prove positivity and boundedness of its variables. Then, we characterize equilibria in some generic cases and we explain the background of their graphical determination. Finally, we investigate through numerical simulation equilibria and their stability of the system, before conclusions and perspectives are formulated.
In Figure 1, we give a schematic representation of the anaerobic membrane bioreactor for which the model (2.5–2.9) is proposed below and, where the membrane retention of soluble and particulate components is illustrated.
We consider the anaerobic mathematical model AM2b presented in [25], where we have four reaction networks:
k1S1μ1(.)X1⟶X1+k2S2+b3S+k4CO2 | (2.1) |
k3S2μ2(.)X2⟶X2+b4S+k5CO2+k6CH4 | (2.2) |
b1Sμ(.)X1⟶X1+b2S2+k7CO2 | (2.3) |
D0X1⟶D0S,D0X2⟶D0S | (2.4) |
In the first reaction, the substrate S1 (organic matter) is degraded into substrates S2 (Volatile Fatty Acids) and S (SMP) by acidogenic bacteria X1 and then in the second reaction, S2 is converted into S by methanogenic bacteria X2. The third reaction network consist in degrading S into S2 by the consortium X1. A part of S is produced from biomasses decay. During reactions (2.1), (2.2) and (2.3), there is a production of biogas.
Mass balance equations are given by:
˙S1=D(S1in−S1)−k1μ1(S1)X1, | (2.5) |
˙X1=(μ1(S1)+μ(S)−D0−D1)X1, | (2.6) |
˙S2=D(S2in−S2)−k3μ2(S2)X2+(k2μ1(S1)+b2μ(S))X1, | (2.7) |
˙X2=(μ2(S2)−D0−D1)X2, | (2.8) |
˙S=(b3μ1(S1)+D0−b1μ(S))X1+(b4μ2(S2)+D0)X2−MS, | (2.9) |
where S1in and S2in are input substrate concentrations, D, D0 and D1 are the dilution rate, the decay rate of biomass and the withdraw rate respectively. M=[βD+(1−β)D1], where β∈[0,1] represents the fraction of S leaving the bioreactor (see [25] for more detail on the model development). Parameters ki and bi are pseudo-stoichiometric coefficients associated to the bioreactions, which represent degradation and production rates of different substrates. The identifiability and the estimation of such parameters are discussed in [11] and [12].
We make the following matter conservation principles:
● over a given period of time, the quantity of biomass (or products) produced is always smaller than the quantity of substrate degraded. Thus, from (2.1–2.3) one has:
k1≥1+b3+k2, | (2.10) |
k3≥1+b4, | (2.11) |
b1≥1+b2. | (2.12) |
● the quantity S2 produced from S1 is higher than the quantity produced from the SMP (see (2.1) and (2.3)):
k2>b2. | (2.13) |
The kinetics μ1, μ2 and μ are assumed to be dependent on S1, S2 and S respectively, satisfying the following hypotheses:
Hypothesis 2.1. μ1(S1) and μ(S) are of class C1 and satisfy the following properties:
● μ1(0)=μ(0)=0,
● μ′1(S1)>0 and μ′(S)>0 for S1>0 and S>0 respectively,
● μ1(∞)=m1 and μ(∞)=m.
Hypothesis 2.2. μ2(S2) is of class C1 and satisfies the following properties:
● μ2(0)=μ2(∞)=0,
● μ2(S2) has a maximum μ2(SM2)>0 for S2=SM2,
● μ′2(S2)>0 for 0<S2<SM2,
● μ′2(S2)<0 for S2>SM2.
The model analysis given in this paper, is valid for all functions verifying the hypothesises (2.1) and (2.2). Examples of functions satisfying these assumptions are (see appendix 1 of [32]):
● The Monod kinetics μ(ξ)=mξξ+K, the Tessier kinetics μ(ξ)=m(1−e−ξK), the Moser or the Ming et al. kinetics μ(ξ)=mξ2K+ξ2 (with m and K are constants), which all satisfy hypothesis 2.1.
● The Haldane kinetics μ(ξ)=mξξ2Ki+ξ+K, or the function μ(ξ)=K(e−α1ξ−e−α2ξ) (with m, K, Ki and α2>α1 are constants), which satisfy hypothesis 2.2.
Positivity and boundedness are very important properties for biological systems. We have to check that for zero or positive initial conditions, all variables of system (2.5–2.9) are non-negative and bounded for all time.
Proposition 2.3. The variables (S1,X1,S2,X2,S) of system (2.5–2.9) are positive and bounded.
Proof. The proof is given in Appendix A.1.
The equilibria of system are solutions of the following nonlinear algebraic system:
0=D(S1in−S1)−k1μ1(S1)X1 | (3.1) |
0=[μ1(S1)+μ(S)−D0−D1]X1 | (3.2) |
0=D(S2in−S2)−k3μ2(S2)X2+[k2μ1(S1)+b2μ(S)]X1 | (3.3) |
0=[μ2(S2)−D0−D1]X2 | (3.4) |
0=[b3μ1(S1)+D0−b1μ(S)]X1+[b4μ2(S2)+D0]X2−MS | (3.5) |
We use the following notations:
A=b4(D0+D1)+D0k3(D0+D1),B=MD=[β+(1−β)D1D]. | (3.6) |
If D0+D1<μ2(SM2) then S1∗2<SM2<S2∗2 are the roots of equation μ2(S2)=D0+D1 and, we note:
αi:=AB(S2in−Si∗2),βi=Dk3(D0+D1)(S2in−Si∗2),i=1,2 | (3.7) |
From Eq (3.2) one deduce that X1=0 or μ1(S1)+μ(S)=D0+D1. The following lemma describes the equilibria points for which X1=0, that is to say, there is a washout of X1.
Lemma 3.1. The equilibria (S∗1,0,S∗2,X∗2,S∗) of the system (2.5–2.9) are given by:
● the washout equilibrium of X1 and X2, E00=(S1in,0,S2in,0,0), which always exists,
● the washout equilibrium of X1 but not of X2,
Ei1=(S1in,0,Si∗2,Xi∗2,Si∗),i=1,2 |
where Si∗2 are the roots of equation μ2(S2)=D0+D1, Xi∗2 and Si∗ are given by the formulas:
Xi∗2=βi,Si∗=αi,i=1,2. |
The equilibrium Ei1 exists if and only if:
S2in>Si∗2. | (3.8) |
Proof. The proof is given in Appendix A.2.1.
Remark 1. In this paper, we present a detailed mathematical analysis of the model equilibria. From biological realism point of view, equilibria Ei1 would not occur except in certain cases. Indeed, S2 (VFA) available for the reaction (2.2) is produced in reactions (2.1) and (2.3) when bacteria X1 degrade S1 (organic substrate) and S (SMP). Also, it can come from outside the bioreactor in S2in (see Figure 1). Often, this is not possible in the biological realism, unless we consider a third acetogenic microorganisms which produce S2in from external organic matter or, if we carry out a bench-scale study, by introducing S2in into the bioreactor.
Now, we consider equilibria for which there is no washout of X1 but washout of X2. We introduce the following notations:
F(S):=μ−11(D0+D1−μ(S)), | (3.9) |
G(S1):=(S1in−S1)(B1−B2μ1(S1)), | (3.10) |
where:
B1=b1+b3k1β,B2=b1(D0+D1)−D0k1β, | (3.11) |
Lemma 3.2. Let E02=(S∗1,X∗1,S∗2,0,S∗) an equilibrium point of the system (2.5–2.9), such that X∗1>0. Then S∗1 and S∗ are solutions of the system of equations:
{S1=F(S)S=G(S1) | (3.12) |
and X∗1 and S∗2 are given by the formulas:
X∗1=Dk1μ1(S∗1)(S1in−S∗1),S∗2=S2in+k2μ1(S∗1)+b2μ(S∗)k1μ1(S∗1)(S1in−S∗1). |
The equilibrium E∗ exists if and only if:
S1in>S∗1. | (3.13) |
Proof. The proof is given in Appendix A.2.2.
Now, we consider equilibria for which there is no washout of X1 nor X2. We introduce the following notations:
H(S1):=(S1in−S1)(C1−C2μ1(S1)), | (3.14) |
Hi(S1):=αi+H(S1),i=1,2. | (3.15) |
where:
C1=B1+A(k2−b2)k1β,C2=B2−Ab2k1β, | (3.16) |
Lemma 3.3. Let Ei2=(S∗1,X∗1,Si∗2,Xi∗2,S∗), i=1,2 an equilibrium point of the system (2.5–2.9) such that X∗1>0 and X∗2>0. Then one has Si∗2, i=1,2 are the roots of equation μ2(S2)=D0+D1, and S∗1 and S∗ are solutions of the system of equations:
{S1=F(S),S=Hi(S1),i=1,2. | (3.17) |
and X∗1 and Xi∗2 are given by the formulas:
X∗1=Dk1μ1(S∗1)(S1in−S∗1),Xi∗2=βi+Dk3(D0+D1)k2μ1(S∗1)+b2μ(S∗)k1μ1(S∗1)(S1in−S∗1) |
The equilibrium E∗ exists if and only if the following conditions hold:
S1in>S∗1andHi(S∗1)>G(S∗1),i=1,2. | (3.18) |
Proof. The proof is given in Appendix A.2.3.
Remark 2. When the system (3.12) or the system (3.17) has several solutions (S∗1j,S∗j), one notes E02j (respectively E12j and E22j), j=1,2 the corresponding equilibria (see section 6.3).
Equilibria of system (2.5–2.9) are determined, by finding graphically solutions of system (3.12) and (3.17). Values of S∗1 and S∗ should be positive and satisfy conditions (3.13) and (3.18). Thus, we should study sign of functions G(S1) and Hi(S1) and, specify the domain where they are positive.
First, let us give the following lemma:
Lemma 4.1. We have λH<λG<λ1 where λH, λG and λ1 are defined by:
λ1=μ−11(D0+D1),λG=μ−11(DG),λH=μ−11(DH), |
with DG=B2/B1 and DH=C2/C1.
Proof. The proof is given in Appendix A.3.
In Figure 2 on the left, we illustrate positions of λH, λG and λ1. On the right, we show solutions Si∗2, i=1,2 of equation μ2(S2)=D0+D1.
The function G(S1) defined by (3.10) is positive for S1 between S1in and λG, the root of:
g(S1)=B1−B2μ1(S1) |
We have two cases (see Figure 3):
● DG>m1, where g(S1) is always negative for S1<S1in (Figure 3, left) and values of S1>S1in do not satisfy the condition (3.13), or
● DG<m1, where g(S1)>0 if and only if S1>λG (Figure 3, right).
The case corresponding to Figure 3, center, is not considered since it does not satisfy the condition (3.13).
Proposition 4.2. A necessary condition for (3.12) to have positive solutions is λG<S1in that is to say μ1(S1in)>DG.
Proof. The proof is given in Appendix A.4.
The function H(S1) defined by (3.14) is positive for S1 between S1in and λH, the root of:
H(S1)=C1−C2μ1(S1) |
Two cases can be distinguished:
● DH>m1, where H(S1) is always negative for S1<S1in, or
● DH<m1, where H(S1)>0 if and only if S1in>S1>λH (see Figure 4).
In the following, we assume that μ1(S1in)>DG. Let us notice that:
● H(S1) is positive if and only if λH<S1<S1in,
● G(S1) is positive if and only if λG<S1<S1in,
● H(S1)>G(S1) for all λH<S1<S1in.
Proposition 4.3. The equilibrium Ei2, i=1,2 exists if and only if the graph of Hi(S1) intersects the axis of S1 on the right of S1in.
Proof. The proof is given in Appendix A.5.
The existence of equilibria depend on the relative positions of the value of S1in and the values of λH, λG and λ1 (see Figure 2). We have four cases:
● S1in<λH<λG<λ1
● λH<S1in<λG<λ1
● λH<λG<S1in<λ1
● λH<λG<λ1<S1in
Recall that an equilibrium exists if and only if the conditions (3.18) are satisfied. We list in the Table 1 the possible existence of equilibria in the four above cases. In all the following figures, the doted vertical line represents λ1=F(0), the blue graph represents G(S1), the green one represents H(S1) and those in red represent H1(S1) (top red graph) and H2(S1) (bottom red graph).
Case | Figure | F∩G | F∩H1 | F∩H2 | Corresponding cases in [16] and/or in [25] |
S1in<λH<λG<λ1 | Figure 5, left | 1.1 of [16] | |||
Figure 5, center | X | 1.2 of [16] | |||
Figure 5, right | X | X | 1.3 of [16] | ||
λH<S1in<λG<λ1 | Figure 6, left | X | X | 1.1 of [16] | |
Figure 6, center | X | X | 2.1 of [16] | ||
Figure 6, right | X | X | 1.3 of [16] | ||
λH<λG<S1in<λ1 | Figure 7, left | X | X | X | 1.1 of [16] |
Figure 7, center | X | X | X | 1.2 of [16] | |
C of [25] | |||||
Figure 7, right | X | X | X | 1.3 of [16] | |
B of [25] | |||||
λH<λG<λ1<S1in | Figure 8, top left | X | X | X | 2.1 of [16] |
Figure 8, top center | X | X | X | 2.2 of [16] | |
Figure 8, top right | X | X | X | 2.3 of [16] | |
Figure 8, bottom left | X | X | X | 2.4 of [16] | |
Figure 8, bottom center | X | X | X | 2.5 of [16] | |
Figure 8, bottom right | X | X | X | 2.6 of [16] | |
A of [25] |
Remark 3. The function F(S) depends on μ(S), but functions G(S1) and Hi(S1), i=1,2 do not depend on it. For μ(0)=0, intersections of F(0)=λ1 with G(S) and Hi(S) correspond to cases of [16] and [25] as mentioned in the last column of Table 1 and seen on Figure 8.
Case: S1in<λH<λG<λ1
In the case 1, illustrated by Figure 5 on the left, we have H>H1>H2 and, intersections F∩Hi and F∩G do not give any positive equilibria, because it does not satisfy the condition (3.13). In the case 2, illustrated by Figure 5 on the center, we have H1>H>H2. The equilibrium of F∩H1 can exist, but there are no equilibria of F∩H2 and F∩G. The last case 3, represented by Figure 5 on the right, we have H1>H2>H. There is a possibility of existence of equilibria F∩H1 and F∩H2 for values of m enough high, but equilibria of F∩G do not exist.
Remark 4. When we have intersection of the function F(S) with functions H1(S1) and H2(S1) at S1=S1in, then we obtain equilibria Ei1=(S1in,0,Si∗2,Xi∗2,Si∗) where Si∗=αi=Hi(S1in), i=1,2 as it can be seen on Figure 5, center, for E11 and on Figure 5, right, for Ei1, i=1,2.
Case : λH<S1in<λG<λ1
This case is illustrated by Figure 6, left, center and right for H>H1>H2, H1>H>H2 and H1>H2>H respectively. Equilibria of F∩Hi, i=1,2 can exist for higher values of m, but not those of F∩G for all values of m.
Case: λH<λG<S1in<λ1
We represents this case by Figure 7, where all equilibria of F∩Hi, i=1,2 and F∩G can exist since condition (3.13) is satisfied. Also, some equilibria bifurcations can occur for higher values of m.
Case: λH<λG<λ1<S1in
Here we have rich situations, equilibria for F∩G exist always, while F∩H1 and F∩H2 may give both equilibria for all m (see Figure 8, top-right, bottom-center and bottom-right), only F∩H1 gives always equilibria (see Figure 8, top-center and bottom-left) or there is equilibria bifurcations for large values of m for F∩H1 and/or F∩H2 (see Figure 8, top-left for F∩H1, top-center and bottom-left for F∩H2).
For trivial equilibria given by lemma 3.1, the results on their stability are summarized in Theorem 6.1.
Theorem 6.1. Existence and stability of washout equilibria of X1 are as follows:
1. The equilirium E00 exists always and it is stable if and only if:
μ1(S1in)<D0+D1and,μ2(S2in)<D0+D1 | (6.1) |
2. The equilibrium E21 exist if and only if S2in>S2∗2 and it is always unstable.
3. The equilibrium E11 exist if and only if S2in>S1∗2 and it is stable if and only if:
μ1(S1in)+μ(S1∗)<D0+D1 | (6.2) |
Proof. The proof is given in Appendix A.6.
The condition (6.2) my be graphically explained on the Figure 9. The graph f(S1,S)=μ1(S1)+μ(S)−D0−D1=0 separates the plane (S1,S) into two zones:
● Zone Z0: where f(S1,S)>0,
● Zone Z1: where f(S1,S)<0.
According to the condition (6.2), the equilibrium E11 is stable if and only if: E11∈Z1 (the case represented on left in Figure 9).
Here, we improve numerical simulations to check the system stability. Values of model parameters are chosen as in Tables 3 and 4 except the parameter m. According to the considered generic case 1, 2 or 3 represented by Figure 10, 11 and 12 respectively, the value of the parameter m is varying in specific intervals for which we could have all possible equilibria bifurcations. Stability nature does not depend on values of m in those intervals (see the column Condition in Table 5 for values of m). Then, we proceed as follows:
λ5 | 1 | a2 | a4 | 0 |
λ4 | a1 | a3 | a5 | 0 |
λ3 | n1 | n2 | 0 | 0 |
λ2 | l1 | a5 | 0 | 0 |
λ1 | r1 | 0 | 0 | 0 |
λ0 | a5 | 0 | 0 | 0 |
0 | 0 | 0 | 0 |
Parameter | Value | Parameter | Value | Parameter | Value | Parameter | Value |
m1 | 1.2 | β | 0.6 | b1 | 5 | m | varying |
m2 | 1.5 | k1 | 25 | b2 | 0.6 | K | 3 |
K2 | 0.3 | k2 | 15 | b3 | 7 | D | 1 |
Ki | 0.9 | k3 | 16.08 | b4 | 5 | D0 | 0.25 |
Parameter | Generic case 1 (Figure 10) | Generic case 2 (Figure 11) | Generic case 3 (Figure 12) |
K1 | 10 | 16 | 18 |
S1in | 15 | 15 | 10 |
S2in | 1 | 1 | 0.6 |
D1 | 0.4 | 0.4 | 0.25 |
Cases | Condition | Equilibria and nature | ||||||||
F∩T | F∩G | F∩H1 | F∩H2 | |||||||
E00 | E11 | E21 | E021 | E022 | E121 | E122 | E221 | E222 | ||
Case 1 (Figure 10) | m≥0 | U | U | U | S | U | S | |||
Case 2 (Figure 11) | ||||||||||
2.1 | 0≤m<c1 | S | S | U | ||||||
2.2 | c1<m<c2 | S | S | U | S | U | ||||
2.3 | c2<m<c3 | S | S | U | S | U | U | U | ||
2.4 | c3<m<c4 | S | S | U | S | U | S | U | U | U |
2.5 | c4<m<c5 | S | U | U | S | U | S | U | U | |
2.6 | c5<m | S | U | U | S | U | S | U | ||
Case 3 (Figure 12) | ||||||||||
3.1 | 0≤m<c1 | U | S | |||||||
3.2 | c1<m<c2 | U | S | S | U | |||||
3.3 | c2<m<c3 | U | S | S | U | U | U | |||
3.4 | c3<m<c4 | U | S | S | U | S | U | U | U | |
3.5 | c4<m<c5 | U | S | S | U | S | U | U | ||
3.6 | c5<m | U | U | S | U | S | U |
● Develop the Jacobian matrix J of system (2.5–2.9) as given by (6.3),
● Evaluate this matrix for each equilibrium characterized by lemma 3.2 or 3.3,
● Develop the characteristic equation of the evaluated matrix,
● Use Routh-Herwitz criterion to analyze the system stability by numerical simulations (plot the coefficients of the first column of the Routh Table 2 according to m).
The jacobian matrix of system (2.5–2.9) evaluated at equilibria is given by (6.3).
J=[−D−k1μ′1(S∗1)X∗1−k1μ1(S∗1)000μ′1(S∗1)X∗1μ1(S∗1)+μ(S∗)−D0−D100μ′(S∗)X∗1k2μ′1(S∗1)X∗1k2μ1(S∗1)+b2μ(S∗)−D−k3μ′2(S∗2)X∗2−k3μ2(S∗2)b2μ′(S∗)X∗100μ′2(S∗2)X∗2μ2(S∗2)−D0−D10b3μ′1(S∗1)X∗1b3μ1(S∗1)+D0−b1μ(S∗)b4μ′2(S∗2)X∗2b4μ2(S∗2)+D0−M−b1μ′(S∗)X∗1] | (6.3) |
\normalsize Which can be symbolized as follows:
J=[j11j12000j21j2200j25j31j32j33j34j3500j43j440j51j52j53j54j55] | (6.4) |
We can distinguish two cases according to lemma 3.2 where X1>0 and X2=0 or, lemma 3.3 where X1>0 and X2>0.
● In the case X2=0, one has: j33=−D and j43=j53=0.
● In the case X1>0 and X2>0, one has: j22=j44=0 (from (3.2) and (3.4)).
The characteristic equation of the linearized system of (2.5–2.9) is:
|λ.I−J|=0⇔λ5+a1λ4+a2λ3+a3λ2+a4λ+a5=0. | (6.5) |
where ai are coefficients depending on jik, (i,k=1..5) given by (6.4). Now, one establishes the following Routh table:
with:
n1=a1a2−a3a1,n2=a1a4−a5a1,l1=n1a3−n2a1n1,r1=l1n2−a5n1l1 |
The Routh-Herwitz criterion imposes that all coefficients of the first column of the Table 2 must have the same sign, i.e., they must be positive (because the first element of the column is positive).
a1>0,n1>0,l1>0,r1>0,a5>0 | (6.6) |
To illustrate our approach, we improve numerical simulations. We present three generic cases illustrated by Figures 10, 11 and 12, which are obtained for the biological parameters values given in Tables 3 and 4 and, kinetics functions (6.7), satisfying hypotheses 2.1 and 2.2.
μ1(S1)=m1S1S1+K1,μ(S)=mSS+K,μ2(S2)=m2S2S22Ki+S2+K2. | (6.7) |
If they exist, equilibria are noted on figures by:
● E12j: equilibria given by the intersection of F(S) with H1(S1), j=1,2,
● E22j: equilibria given by the intersection of F(S) with H2(S1), j=1,2,
● E02j: equilibria given by the intersection of F(S) with G(S1), j=1,2.
The form of F(S) changes according to the value of the parameter m, the maximum growth rate of μ(S) (see (3.9)). Consequently, F(S) can have one or two intersections with each one of functions H1(S1), H2(S1) or G(S1) as illustrated in Figures 10, 11 and 12.
In Figure 10 corresponding to the generic case 1, we have only one equilibrium noted E11, E21 and E01 for each intersection of F with H1, H2 and G respectively.
In generic cases 2 and 3, we have equilibria bifurcation when m varies. For some values ci, i=1,..,5 of m, (of course, they are different between cases 2 and 3), the graph of F(S) intersects graphs of Hi(S1) and G(S1) (see Figures 13 and 14), leading to the apparition of new equilibria. The reader can refer to [25,33] for more details.
Equilibria of system and their nature according to m in the three generic cases are summarized in Table 5, where T stands for Trivial Equilibria E00, E11 and E21, F∩H1, F∩H2 and F∩G stand for Equilibria obtained by the intersections of the graph F with graphs H1, H2 and G, respectively, S and U stand for Stable and Unstable Equilibrium, respectively. If there is no symbol, then it means that the equilibrium does not exist.
Stability nature of equilibria corresponding to the washout of X2 (F∩G) and the existence of both X1 and X2 (F∩H1 and F∩H2) is checked by using the Routh-Herwitz criterion as detailed in the section 6.2.
On Figures 15, 16, and 17, we represent the coefficients of the first column of Table 2 with different colors: a1 in black, n1 in blue, l1 in red, r1 in magenta and a5 in green. On Figures 16, and 17, vertical lines represent bifurcation values ci, i=1..5 of the parameter m (they are different between the two figures). According to the considered case, coefficients are represented only for values of m, for which equilibria may exist. For instance, Routh coefficients for the equilibrium E122 are represented on Figure 16, bottom-left, only for c1≤m≤c4. If equilibrium is stable, then all coefficients must be positive in the corresponding interval of m.
Remark 5. Stability nature of equilibria E00,E11 and E21 of the case 1 in Table 5, can be seen on Figure 10 as follows:
● λ1<S1in⇒μ1(λ1)<μ1(S1in), that is to say D0+D1<μ1(S1in)⇒E00 is unstable according to theorem 6.1.1
● (S1in,S1∗)∈Z0⇒E11 is unstable according to condition (6.2) of theorem 6.1.3 and, Figure 9.
● E21 does exist according to proposition 4.3 and, is unstable thanks to theorem 6.1.2.
Remark 6. Stability nature of the equilibrium E00 of the cases 2 and 3 in Table 5, can be analyzed as follows:
● μ1(S1in)<D0+D1 as it is seen on Figure 11 and 12 for both cases.
● From parameters values in Tables 3 and 4 and, according to condition (6.1) of theorem 6.1 we have:
μ2(S2in)<D0+D1 for the case 2, thus the equilibrium E00 is Stable.
μ2(S2in)>D0+D1 for the case 3, thus the equilibrium E00 is Unstable.
As can be seen on figures, if they exist:
● The first equilibrium E121 of F∩H1 and the first equilibrium E021 of F∩G are always stable. Coefficients given by (6.6) of the first column of Routh table are always positive.
● The second equilibrium E122 of F∩H1 and the second equilibrium E022 of F∩G are always unstable. Some coefficients given by (6.6) are (or become) negative (for instance a5 on Figure 16, second sub-Fig from top, is always negative, or r1 in magenta on Figure 17, last sub-Fig, becomes negative).
● both equilibria E221 and E222 of F∩H2 are unstable. Some coefficients of (6.6) are always negative (for instance r1 in magenta and a5 in green on Figure 15).
At this stage of discussion about stability nature of possible equilibria, we give a conjecture on positive ones which are obtained for F∩Hi, i=1,2.
Conjecture 6.2..
● Equilibria E22j, j=1,2, resulting resulting from F∩H2 are unstable if they exist.
● The only stable equilibrium E121 resulting from F∩H1, is the one which corresponds to the smallest value of S∗1.
The simple model AM2 is widely used in the literature to describe anaerobic digestion in two-step of biological reactions. This model proved its ability to adequately predict dynamics of the main variables of the anaerobic digestion and, it was used with efficiency for control and supervision purposes. It has been shown in [16] that the AM2 model can have at most six steady states depending on its operating parameters. Nevertheless, the AM2 model was not able to simulate some phenomena in many practical biological experiments. There is why extended versions of the AM2 model were proposed in the literature, by integrating some few new variables.
In this paper we investigated the effect of a new variable S (SMP: Soluble Microbial Product) integration on steady states of a two-step anaerobic digestion model. Indeed, this model initially proposed in [25] for control purposes, is an extension of the AM2 model for anaerobic membrane bioreactors. We consider the dynamics of five variables: two bacteria populations (X1, X2) and three substrates including the new variable (S1, S2, S), where one microorganism X1 can growth on one substrate S1 to produce both the second and the third substrates S2 and S and also on S to produce S2, while the second microorganism X2 can only growth on S2 and, could be inhibited by an excess quantity of this substrate. S is produced from degradation of S1 and S2 and, death of bacteria X1 and X2. One important parameter which could considerably alter the system behavior is the maximum growth rate m of the first bacteria population X1 on the new substrate S. Indeed, this biological parameter is considered as a bifurcation parameter in addition to the conventional operating parameters which are the dilution rate D and the inlet substrate concentrations S1in and S2in.
In this paper, the model equilibria and their stability were analyzed analytically and using numerical simulations according to this bifurcation parameter m. We distinguished three generic cases accordingly to the system parameters values (Tables 3 and 4), where the system can exhibit rich qualitative behavior in terms of equilibria bifurcation and multistability. We have highlighted that in the first generic case (Figure 10), the behavior of the extended model is exactly similar to the AM2 one (i.e., six equilibria with bistability). While in the second generic case (Figure 11), for a set of parameters values, especially the maximum growth rate m of X1 on S (the new variable), the system can have until nine equilibria where four of them are stable (multistability). In the third generic case (Figure 12), we can have eight equilibria with trystability.
Our study shows how the behavior of a two-step model of anaerobic digestion can be altered by the integration of the new variable in some generic cases and, how the model equilibria and their stability would be sensible to the bifurcation parameter m. Our results would be useful for both mathematicians and biologists communities and, could build the roots for dialogue between them as noted in the introduction. If a mathematical model as the one used in this paper is fitted accurately with experimental data of microorganisms and substrates, then biologists can use trustfully this model to predict future main behaviors of their anaerobic digesters. Also, they can explain and understand some observed phenomena using results of our analysis. For instance, depending on the value of the parameter m of the growth kinetics of X1 on S, biologists can interpret why they find different concentrations for bacteria and substrates at steady state, when doing the experiment starting from different initial concentrations. This is exactly the multistability which is predicted by the model when the value of the bifurcation parameter m varies. On the other hand, experimenters can act on the operating parameters S1in and S2in and the bifurcation parameter m in order to force the behavior of the biological process towards a desired steady state.
In light of these results, our main perspective consists of establishing of a complete operating diagram of the considered model with respect of operating parameters which are D, S1in, S2in and, especially the maximum growth rate m of the first bacteria X1 on the new variable S. In other terms, we wish to explore the different asymptotic behaviors of the system in 2 dimensional planes where one of the plane coordinates is the maximum growth rate m. Such operating diagrams if well established and discussed, can be really useful to interpret experimental results and, to help biologists to best choose values of operating parameters for controlling their experiments.
The authors thank the Euro-Mediterranean research network Treasure (https://www6.inrae.fr/treasure) who partially financed this research. Part of the work was completed during the mission of the first author in Narbonne. This mission was publicly funded through ANR (the French National Research Agency) under the "Investissements d'avenir" program with the reference ANR-16-IDEX-0006. The first author would like to thank: Direction Générale de la Recherche Scientifique et du Développement Technologique (DG RSDT), Algeria, for support. We thank the reviewers for their valuable comments, which helped to improve the quality of the paper.
The authors declare that they have no competing interests.
We have the following solutions for equations (2.6) and (2.8):
X1(t)=X1(0)e∫t0[μ1(S1(τ))+μ(S(τ))−D0−D1]dτ, |
X2(t)=X2(0)e∫t0[μ2(S2(τ))−D0−D1]dτ. |
Thus, we have:
● X1(0)=0⇒X1(t)=0 and X2(0)=0⇒X2(t)=0,
● X1(0)>0⇒X1(t)>0 and X2(0)>0⇒X2(t)>0.
To prove the positivity of S1, S2 and S, we set these variables equal to zero in (2.5), (2.7) and (2.9) respectively and, we verify if their derivatives are positives:
● ˙S1=DS1in>0,
● ˙S2=DS2in>0,
● ˙S=D0X1+D0X2>0, if X1>0 and X2>0.
Notice that ˙S1, ˙S2 and ˙S are positives. All vector fields at bounds are inside directed. Consequently, the variables S1, S2 and S remain positives for positive initial conditions.
Let us define the quantity:
Σ=S1+S2+X1+X2+S. |
The dynamic of Σ is written as follows:
˙Σ=D(S1in+S2in)−D(S1+S2)−D1(X1+X2)−MS |
−(k1−1−b3−k2)μ1(S1)X1−(k3−1−b4)μ2(S2)X2−(b1−1−b2)μ(S)X1. |
We have three dilution rates: D,D1 and M which is a combination of D and D1. Let us set Dmin=min(D,D1), which allows to write:
˙Σ≤D(S1in+S2in)−DminΣ−(k1−1−b3−k2)μ1X1−(k3−1−b4)μ2X2−(b1−1−b2)μX1. | (A.1) |
By using inequalities (2.10), (2.11) and (2.12), we can write:
˙Σ≤D(S1in+S2in)−DminΣ. |
Since the solution of the equation ˙Σ0=D(S1in+S2in)−DminΣ0 is:
Σ0(t)=D(S1in+S2in)Dmin+Ce−Dmint,with C is constant, |
then, we have Σ(t)≤Σ0(t), i.e.:
Σ(t)≤D(S1in+S2in)Dmin+Ce−Dmint⟹limt→+∞Σ(t)≤D(S1in+S2in)Dmin. |
Consequently, the variables of system (2.5–2.9) remain bounded.
The equilibrium points are solutions of the nonlinear algebraic system obtained from (2.5–2.9) by setting the right-hand sides equal to zero.
From (3.2), we can have a trivial solution X∗1=0, which if replaced in (3.1), then we obtain S∗1=S1in. From Eq (3.4), we can have two cases:
● A trivial solution X∗2=0: which if replaced in (3.3) and (3.5), then we have S∗2=S2in and S∗=0 respectively. This is the equilibrium E00.
● A nontrivial solution S∗2=μ−12(D0+D1)=Si∗2, i=1,2: which if replaced in (3.3) and (3.5), then we deduce corresponding values of Xi∗2 and Si∗ respectively. These are equilibria Ei1, i=1,2.
Let (S∗1,X∗1,S∗2,X∗2,S∗) a solution of system (3.1)–(3.5).
Since X∗1>0, from (3.2) we have μ1(S∗1)+μ(S∗)=D0+D1, i.e.:
S∗1=μ−11(D0+D1−μ(S∗))=F(S∗). |
From (3.1), we deduce:
X∗1=DS1in−S∗1k1μ1(S∗1), |
which is positive and bounded if S∗1<S1in. By replacing X∗2=0 and X∗1 in (3.3) we obtain:
S∗2=S2in+[k2μ1(S∗1)+b2μ(S∗)]S1in−S∗1k1μ1(S∗1). |
Finally, if we replace X∗2, X∗1 and μ(S∗)=D0+D1−μ1(S∗1) in (3.5), then we have after simplification:
S∗=(S1in−S∗1)(B1+B2μ1(S∗1))=G(S∗1), |
with:
B1=b3+b1k1B,B2=D0−b1(D0+D1)k1B,B=β+(1−β)D1D. |
Then S∗1 and S∗ are solutions of the system of Eqs (3.12).
Let (S∗1,X∗1,S∗2,X∗2,S∗) a solution of system (3.1)–(3.5).
Since X∗2>0, from (3.4) we have the nontrivial solution:
S∗2=μ−12(D0+D1)=Si∗2,i=1,2. |
Since X∗1>0, from (3.2) we have:
S∗1=μ−11(D0+D1−μ(S∗))=F(S∗), |
and thus, if 0<S∗1<S1in then, we deduce from (3.1):
X∗1=D[S1in−S∗1]k1μ1(S∗1). |
By replacing X∗1 in (3.3), we obtain:
Xi∗2=βi+Dk3(D0+D1)k2μ1(S∗1)+b2μ(S∗)k1μ1(S∗1)(S1in−S∗1), |
with : βi=Dk3(D0+D1)(S2in−Si∗2),i=1,2
Finally, from (3.5) we have after simplification:
S∗=αi+(S1in−S∗1)(C1−C2μ1(S∗1))=Hi(S∗1),i=1,2, |
with:
αi=AB(S2in−Si∗2),C1=B1+A(k2−b2)k1β,C2=B2−Ab2k1β. |
A=b4(D0+D1)+D0k3(D0+D1),B=β+(1−β)D1D. |
Then S∗1 and S∗ are solutions of the system of Eqs (3.17).
The function Hi(S∗1) can be written as:
Hi(S1)=G(S1)+AB[S2in−Si∗2+(k2μ1(S1)+b2μ(S))S1in−S1k1μ1(S1)]. |
The condition for which Xi∗2>0 is:
S2in−Si∗2+(k2μ1(S∗1)+b2μ(S∗))S1in−S∗1k1μ1(S∗1)>0, |
which is equivalent to: Hi(S∗1)>G(S∗1),i=1,2. (condition of lemma 3.3).
Using (2.13), we have C1>B1 and C2>B2>0, consequently: DH<DG<D0+D1.
Using the fact that mu1 is increasing, we deduce that:
λH<λG<λ1 | (A.2) |
with: λ1=μ−11(D0+D1), λG=μ−11(DG) and λH=μ−11(DH), where DG=B2/B1 and DH=C2/C1.
The function G(S1) given by (3.10) is positive between S1in>S1>λG and, solutions of the system (3.12) must satisfy S1in>S∗1, then S1in>λG that is to say μ1(S1in)>DG.
Functions Hi(S1), i=1,2 given by (3.15) are translations of the function H(S1) with quantities αi given by (3.7). The sign of this later indicates if the equilibrium Ei2, i=1,2 does exist or not (see Figure 4).
The study of the local stability of trivial equilibria follows easily from the study of the Jacobian matrix of system (2.5–2.9), which has a block-diagonal structure:
J=[A00CB0M1M2−M], |
Hence, the eigenvalues of J are the eigenvalues of A, the eigenvalues of B and −M (which is always negative).
For the X1 and X2 washout equilibrium E00=[S1in,0,S2in,0,0]:
one has:
A=[−D−k1μ1(S1in)0μ1(S1in)−D0−D1], |
B=[−D−k3μ2(S2in)0μ2(S2in)−D0−D1]. |
Conditions of stability are: tr(A)<0, tr(B)<0, det(A)>0 and det(B)>0. Thus, E00 is stable if and only if:
μ1(S1in)<D0+D1 and, μ2(S2in)<D0+D1 |
For the X1 washout equilibria Ei1=[S1in,0,Si∗2,Xi∗2,Si∗], i=1,2:
one has:
A=[−D−k1μ1(S1in)0μ1(S1in)+μ(Si∗)−D0−D1], |
B=[−D−k3μ′2(Si∗2)Xi∗2−k3μ2(Si∗2)μ′2(Si∗2)Xi∗20]. |
One can easily deduce that if E21 exists, then it is unstable because det(B)<0, since μ′2(S2∗2)<0.
On the other hand, stability of E11 depends on μ(S1∗). Indeed, E11 is stable if and only if:
μ1(S1in)+μ(S1∗)<D0+D1. |
[1] | Ministry of Health, Labour, and Welfare, Request to install the COVID-19 contact-confirming application (COCOA) 2021, Available from: https://www.mhlw.go.jp/content/10900000/000773753.pdf. |
[2] | Department of Health and Social Care, NHS. NHS COVID-19 App 2020, Available from: https://www.nhsx.nhs.uk/covid-19-response/nhs-covid-19-app/. |
[3] | Anti-Covid-19 Tech Team, Trends in each country regarding the introduction of contact confirmation applications, 2020, Available from: https://cio.go.jp/sites/default/files/uploads/documents/techteam_20200508_02.pdf |
[4] |
F. Yang, Q. Yang, X. Liu, P. Wang, SIS evolutionary game model and multi-agent simulation of an infectious disease emergency, Technol. Health Care, 23 (2015), 603–613. doi: 10.3233/THC-150999
![]() |
[5] | J. B. Dunham, An agent-based spatially explicit epidemiological model in MASON, J. Artif. Soc. Social Simul., 9 (2005). |
[6] | H. Hirose, Pandemic simulations by MADE: A combination of multi-agent and differential equations, with a novel influenza a (H1N1) case, Information, 16 (2013), 5365–5390. |
[7] | D. Chumachenko, V. Dobriak, M. Mazorchuk, I. Meniailov, K. Bazilevych, On agent-based approach to influenza and acute respiratory virus infection simulation, 14th International Conference on Advanced Trends in Radioelectronics, Telecommunications, and Computer Engineering, (2018), 192–195, doi: 10.1109/TCSET.2018.8336184 |
[8] |
C. Hou, J. Chen, Y. Zhou, L. Hua, J. Yuan, S. He, et al., The effectiveness of quarantine in Wuhan city against coronavirus disease 2019 (COVID-19): a well-mixed SEIR model analysis, J. Med. Virol, 92 (2020), 841–848, doi: 10.1002/jmv.25827 doi: 10.1002/jmv.25827
![]() |
[9] |
B. Prasse, M. A. Achterberg, L. Ma, P. V. Mieghem, Network-inference-based prediction of the COVID-19 epidemic outbreak in the Chinese province Hubei, Appl. Network Sci., 5 (2020), doi: 10.1007/s41109-020-00274-2 doi: 10.1007/s41109-020-00274-2
![]() |
[10] |
Q. Yang, C. Yi, A. Vajdi, L. W. Cohnstaedt, H. Wu, X. Guo, et al., Short-term and long-term mitigation evaluations for the COVID-19 epidemic in Hubei province, China, Infect. Dis. Modell., 5 (2020), 563–574. doi: 10.1016/j.idm.2020.08.001
![]() |
[11] |
K. Chatterjee, K. Chatterjee, A. Kumar, S. Shankar, Healthcare impact of COVID-19 epidemic in India: a stochastic mathematical model, Med. J. Armed Forces India, 76 (2020), 147–155. doi: 10.1016/j.mjafi.2020.03.022
![]() |
[12] | M. A. Achterberg, B. Prasse, L. Ma, S. Trajanovski, M. Kitsak, P. V. Mieghem, Comparing the accuracy of several network-based COVID-19 prediction algorithms, Int. J. Forecast., (2020), doi: 10.1016/j.ijforecast.2020.10.001 |
[13] |
Z. Liu, P. Magal, G. Webb, Predicting the number of reported and unreported cases of COVID-19 epidemics in China, South Korea, Italy, France, Germany, and the United Kingdom, J. Theor. Biol, 509 (2020), doi: 10.1016/j.jtbi.2020.110501 doi: 10.1016/j.jtbi.2020.110501
![]() |
[14] |
E. A. Iboi, O. Sharomi, C. N. Ngonghala, A. B. Gumel, Mathematical modeling and analysis of COVID-19 pandemic in Nigeria, Math. Biosci. Eng., 17 (2020), 7192–7220, doi: 10.3934/mbe.2020369 doi: 10.3934/mbe.2020369
![]() |
[15] |
H. Wang, N. Yamamoto, Using a partial differential equation with Google Mobility data to predict COVID-19 in Arizona, Math. Biosci. Eng., 17 (2020), 4891–4904, doi: 10.3934/mbe.2020266 doi: 10.3934/mbe.2020266
![]() |
[16] |
S. Wang, Y. Liu, T. Hu, Examining the change of human mobility adherent to social restriction policies and its effect on COVID-19 cases in Australia, Int. J. Environ. Res. Public Health, 17 (2020), doi: 10.3390/ijerph17217930 doi: 10.3390/ijerph17217930
![]() |
[17] |
C. Zeng, J. Zhang, Z. Li, X. Sun, B. Olatosi, S. Weissman, X. Li, Spatial-temporal relationship between population mobility and COVID-19 outbreaks in South Carolina: time series forecasting analysis, J. Med. Internet Res., 23 (2021), doi:10.2196/27045 doi: 10.2196/27045
![]() |
[18] |
M. Sulyokab, M. D. Walker, Mobility and COVID-19 mortality across Scandinavia: A modeling study, Travel Med. Infect. Dis., 41 (2021), doi: 10.1016/j.tmaid.2021.102039 doi: 10.1016/j.tmaid.2021.102039
![]() |
[19] |
I. F. F. dos Santos, G. M. A. Almeida, F. A. B. F. de Moura, Adaptive SIR model for propagation of SARS-CoV-2 in Brazil, Phys. A, 569 (2021), doi: 10.1016/j.physa.2021.125773 doi: 10.1016/j.physa.2021.125773
![]() |
[20] |
I. F. F. dos Santos, G. M. A. Almeida, F. A. B. F. de Moura, Evolution of SARS-CoV-2 in the state of Alagoas-Brazil via an adaptive SIR model, Int. J. Mod. Phys. C, 32 (2021), doi: 10.1142/S0129183121500406 doi: 10.1142/S0129183121500406
![]() |
[21] | M. Niwa, Y. Hara, S. Sengoku, K. Kodama, Effectiveness of social measures against COVID-19 outbreaks in several Japanese regions analyzed by system dynamic modeling, SSRN 3653579 (2020), doi: 10.2139/ssrn.3653579 |
[22] |
S. Kurahashi, Estimating effectiveness of preventing measures for 2019 novel coronavirus diseases (COVID-19), Trans. Jpn. Soc. Artif. Intell., 35 (2020), D-K28-1, doi: 10.1527/tjsai.D-K28 doi: 10.1527/tjsai.D-K28
![]() |
[23] |
G. L. Vasconcelos, A. M. S. Macêdo, G. C. Duarte-Filho, A. A. Brum, R. Ospina, F. A. G. Almeida, Power law behaviour in the saturation regime of fatality curves of the COVID-19 pandemic, Sci. Rep., 11 (2021), article number: 4619, doi: 10.1038/s41598-021-84165-1 doi: 10.1038/s41598-021-84165-1
![]() |
[24] | A. M. S. Macêdo, A. A. Brum, G. C. Duarte-Filho, F. A. G. Almeida, R. Ospina, G. L. Vasconcelos, A comparative analysis between a SIRD compartmental model and the Richards growth model, medRxiv, doi: 10.1101/2020.08.04.20168120 |
[25] | R. Hinch, W. Probert, A. Nurtay, M. Kendall, C. Wymant, M. Hall, et al., Effective configurations of a digital contact tracing app: a report to NHSX, 2020. Available from: https://045.medsci.ox.ac.uk/files/files/report-effective-app-configurations.pdf. |
[26] |
E. Hernandez-Orallo, P. Manzoni, C. T. Calafate, J. C. Cano, Evaluating how smartphone contact tracing technology can reduce the spread of infectious diseases: The case of COVID-19, IEEE Access, 8 (2020), 99083–99097. doi: 10.1109/ACCESS.2020.2998432
![]() |
[27] |
M. E. Kretzschmar, G. Rozhnova, M. C. J. Bootsma, M. Boven, J. H. H. M. Wijgert, M. J. M. Bonten, Impact of delays on effectiveness of contact tracing strategies for COVID-19: A modeling study, Lancet Public Health, 5 (2020), e452–e459. doi: 10.1016/S2468-2667(20)30157-2
![]() |
[28] |
J. A. Kucharski, P. Klepac, A. J. K. Conlan, S. M. Kissler, M. L. Tang, H. Fry, et al., Effectiveness of isolation, testing, contact tracing, and physical distancing on reducing transmission of SARS-CoV-2 in different settings: A mathematical modeling study, Lancet Infect. Dis., 20 (2020), 1151–1160. doi: 10.1016/S1473-3099(20)30457-6
![]() |
[29] |
L. Ferretti, C. Wymant, M. Kendall, L. Zhao, A. Nurtay, L. Abeler-Dorner, et al., Quantifying SARS-CoV-2 transmission suggests epidemic control with digital contact tracing, Science, 368 (2020), doi: 10.1126/science.abb6936 doi: 10.1126/science.abb6936
![]() |
[30] | Y. Omae, J. Toyotani, K. Hara, H. Takahashi, Effectiveness of COVID-19 contact-confirming application (COCOA) based on a multi-agent simulation, preprint, arXiv: 2008.13166. |
[31] | J. Kurita, T. Sugawara, Y. Ohkusa, Effectiveness of COCOA, a COVID-19 contact notification application, in Japan, preprint, medRxiv (2020) doi: 10.1101/2020.07.11.20151597 |
[32] |
G. Kobayashi, S. Sugasawa, H. Tamae, T. Ozu, Predicting intervention effect for COVID-19 in Japan: State space modeling approach, Biosci. Trends, 14 (2020), 174–181, doi: 10.5582/bst.2020.03133 doi: 10.5582/bst.2020.03133
![]() |
[33] | M. S. Boudrioua, A. Boudrioua, Predicting the COVID-19 epidemic in algeria using the SIR model, preprint, medrxiv (2020) doi: 10.1101/2020.04.25.20079467 |
[34] | B. Malavika, S. Marimuthu, M. Joy, A. Nadaraj, E. S. Asirvatham, L. Jeyaseelan, Forecasting COVID-19 epidemic in India and high incidence states using SIR and logistic growth models, Clin. Epidemiol. Glob. Health, 9 (2020), 26–33. |
[35] |
I. Cooper, A. Mondal, C. G. Antonopoulos, A SIR Model Assumption for the spread of COVID-19 in different communities, Chaos Solitons Fractals, 139 (2020), doi: 10.1016/j.chaos.2020.110057 doi: 10.1016/j.chaos.2020.110057
![]() |
[36] |
G. Nakamura, B. Grammaticos, M. Badoual, Confinement strategies in a simple SIR Model, Regul. Chaot. Dyn., 25 (2020), 509–521. doi: 10.1134/S1560354720060015
![]() |
[37] | M. Itakura, M. Asahi, T. Yamaguchi, Proposal of an infection model for foot-and-mouth disease epidemic, Oper. Res. Manag. Sci. Res., 56 (2011), 728–734, Available from: http://www.orsj.or.jp/archive2/or56-12/or56-12_728.pdf |
[38] | H. Nishiura, H. Inaba, Prediction of infectious disease outbreak with particular emphasis on statistical issues using the transmission model, Proc. Inst. Stat. Math., 54 (2006), 461–480, Available from: https://www.ism.ac.jp/editsec/toukei/pdf/54-2-461.pdf |
[39] |
T. Tsuchiya, A study on the spread of new coronavirus infections, Commun. Oper. Res. Soc. Jpn., 66 (2021), 90–103, doi: 10.24545/00001758 doi: 10.24545/00001758
![]() |
[40] | Ministry of Health, Labour, and Welfare, Open data of positive result of COVID-19, 2021, Available from: https://www.mhlw.go.jp/content/pcr_positive_daily.csv. |
[41] | Ministry of Health, Labour, and Welfare, COVID-19 Contact-Confirming Application (COCOA), 2020, Available from: https://www.mhlw.go.jp/stf/seisakunitsuite/bunya/cocoa_00138.html. |
[42] |
L. M. Kucirka, S. A. Lauer, O. Laeyendecker, D. Boon, J. Lessler, Variation in false-negative rate of reverse transcriptase polymerase Chain reaction-based SARS-CoV-2 tests by time since exposure, Ann. Intern. Med., 173 (2020), 262–267. doi: 10.7326/M20-1495
![]() |
[43] | Python 3.7.3, 2019, Available from: https://www.python.org/downloads/release/python-373/ |
[44] | Numpy, 2021, Available from: https://numpy.org |
[45] | E. B. Postnikov, Estimation of COVID-19 dynamics "on a back-of-envelope": Does the simplest SIR model provide quantitative parameters and predictions?, Chaos Solitons Fractals, 135 (2020), doi: 10.1016/j.chaos.2020.109841 |
1. | Tewfik Sari, Best Operating Conditions for Biogas Production in Some Simple Anaerobic Digestion Models, 2022, 10, 2227-9717, 258, 10.3390/pr10020258 | |
2. | Radhouane Fekih-Salem, Yessmine Daoud, Nahla Abdellatif, Tewfik Sari, A Mathematical Model of Anaerobic Digestion with Syntrophic Relationship, Substrate Inhibition, and Distinct Removal Rates, 2021, 20, 1536-0040, 1621, 10.1137/20M1376480 |
Case | Figure | F∩G | F∩H1 | F∩H2 | Corresponding cases in [16] and/or in [25] |
S1in<λH<λG<λ1 | Figure 5, left | 1.1 of [16] | |||
Figure 5, center | X | 1.2 of [16] | |||
Figure 5, right | X | X | 1.3 of [16] | ||
λH<S1in<λG<λ1 | Figure 6, left | X | X | 1.1 of [16] | |
Figure 6, center | X | X | 2.1 of [16] | ||
Figure 6, right | X | X | 1.3 of [16] | ||
λH<λG<S1in<λ1 | Figure 7, left | X | X | X | 1.1 of [16] |
Figure 7, center | X | X | X | 1.2 of [16] | |
C of [25] | |||||
Figure 7, right | X | X | X | 1.3 of [16] | |
B of [25] | |||||
λH<λG<λ1<S1in | Figure 8, top left | X | X | X | 2.1 of [16] |
Figure 8, top center | X | X | X | 2.2 of [16] | |
Figure 8, top right | X | X | X | 2.3 of [16] | |
Figure 8, bottom left | X | X | X | 2.4 of [16] | |
Figure 8, bottom center | X | X | X | 2.5 of [16] | |
Figure 8, bottom right | X | X | X | 2.6 of [16] | |
A of [25] |
λ5 | 1 | a2 | a4 | 0 |
λ4 | a1 | a3 | a5 | 0 |
λ3 | n1 | n2 | 0 | 0 |
λ2 | l1 | a5 | 0 | 0 |
λ1 | r1 | 0 | 0 | 0 |
λ0 | a5 | 0 | 0 | 0 |
0 | 0 | 0 | 0 |
Parameter | Value | Parameter | Value | Parameter | Value | Parameter | Value |
m1 | 1.2 | β | 0.6 | b1 | 5 | m | varying |
m2 | 1.5 | k1 | 25 | b2 | 0.6 | K | 3 |
K2 | 0.3 | k2 | 15 | b3 | 7 | D | 1 |
Ki | 0.9 | k3 | 16.08 | b4 | 5 | D0 | 0.25 |
Parameter | Generic case 1 (Figure 10) | Generic case 2 (Figure 11) | Generic case 3 (Figure 12) |
K1 | 10 | 16 | 18 |
S1in | 15 | 15 | 10 |
S2in | 1 | 1 | 0.6 |
D1 | 0.4 | 0.4 | 0.25 |
Cases | Condition | Equilibria and nature | ||||||||
F∩T | F∩G | F∩H1 | F∩H2 | |||||||
E00 | E11 | E21 | E021 | E022 | E121 | E122 | E221 | E222 | ||
Case 1 (Figure 10) | m≥0 | U | U | U | S | U | S | |||
Case 2 (Figure 11) | ||||||||||
2.1 | 0≤m<c1 | S | S | U | ||||||
2.2 | c1<m<c2 | S | S | U | S | U | ||||
2.3 | c2<m<c3 | S | S | U | S | U | U | U | ||
2.4 | c3<m<c4 | S | S | U | S | U | S | U | U | U |
2.5 | c4<m<c5 | S | U | U | S | U | S | U | U | |
2.6 | c5<m | S | U | U | S | U | S | U | ||
Case 3 (Figure 12) | ||||||||||
3.1 | 0≤m<c1 | U | S | |||||||
3.2 | c1<m<c2 | U | S | S | U | |||||
3.3 | c2<m<c3 | U | S | S | U | U | U | |||
3.4 | c3<m<c4 | U | S | S | U | S | U | U | U | |
3.5 | c4<m<c5 | U | S | S | U | S | U | U | ||
3.6 | c5<m | U | U | S | U | S | U |
Case | Figure | F∩G | F∩H1 | F∩H2 | Corresponding cases in [16] and/or in [25] |
S1in<λH<λG<λ1 | Figure 5, left | 1.1 of [16] | |||
Figure 5, center | X | 1.2 of [16] | |||
Figure 5, right | X | X | 1.3 of [16] | ||
λH<S1in<λG<λ1 | Figure 6, left | X | X | 1.1 of [16] | |
Figure 6, center | X | X | 2.1 of [16] | ||
Figure 6, right | X | X | 1.3 of [16] | ||
λH<λG<S1in<λ1 | Figure 7, left | X | X | X | 1.1 of [16] |
Figure 7, center | X | X | X | 1.2 of [16] | |
C of [25] | |||||
Figure 7, right | X | X | X | 1.3 of [16] | |
B of [25] | |||||
λH<λG<λ1<S1in | Figure 8, top left | X | X | X | 2.1 of [16] |
Figure 8, top center | X | X | X | 2.2 of [16] | |
Figure 8, top right | X | X | X | 2.3 of [16] | |
Figure 8, bottom left | X | X | X | 2.4 of [16] | |
Figure 8, bottom center | X | X | X | 2.5 of [16] | |
Figure 8, bottom right | X | X | X | 2.6 of [16] | |
A of [25] |
λ5 | 1 | a2 | a4 | 0 |
λ4 | a1 | a3 | a5 | 0 |
λ3 | n1 | n2 | 0 | 0 |
λ2 | l1 | a5 | 0 | 0 |
λ1 | r1 | 0 | 0 | 0 |
λ0 | a5 | 0 | 0 | 0 |
0 | 0 | 0 | 0 |
Parameter | Value | Parameter | Value | Parameter | Value | Parameter | Value |
m1 | 1.2 | β | 0.6 | b1 | 5 | m | varying |
m2 | 1.5 | k1 | 25 | b2 | 0.6 | K | 3 |
K2 | 0.3 | k2 | 15 | b3 | 7 | D | 1 |
Ki | 0.9 | k3 | 16.08 | b4 | 5 | D0 | 0.25 |
Parameter | Generic case 1 (Figure 10) | Generic case 2 (Figure 11) | Generic case 3 (Figure 12) |
K1 | 10 | 16 | 18 |
S1in | 15 | 15 | 10 |
S2in | 1 | 1 | 0.6 |
D1 | 0.4 | 0.4 | 0.25 |
Cases | Condition | Equilibria and nature | ||||||||
F∩T | F∩G | F∩H1 | F∩H2 | |||||||
E00 | E11 | E21 | E021 | E022 | E121 | E122 | E221 | E222 | ||
Case 1 (Figure 10) | m≥0 | U | U | U | S | U | S | |||
Case 2 (Figure 11) | ||||||||||
2.1 | 0≤m<c1 | S | S | U | ||||||
2.2 | c1<m<c2 | S | S | U | S | U | ||||
2.3 | c2<m<c3 | S | S | U | S | U | U | U | ||
2.4 | c3<m<c4 | S | S | U | S | U | S | U | U | U |
2.5 | c4<m<c5 | S | U | U | S | U | S | U | U | |
2.6 | c5<m | S | U | U | S | U | S | U | ||
Case 3 (Figure 12) | ||||||||||
3.1 | 0≤m<c1 | U | S | |||||||
3.2 | c1<m<c2 | U | S | S | U | |||||
3.3 | c2<m<c3 | U | S | S | U | U | U | |||
3.4 | c3<m<c4 | U | S | S | U | S | U | U | U | |
3.5 | c4<m<c5 | U | S | S | U | S | U | U | ||
3.6 | c5<m | U | U | S | U | S | U |