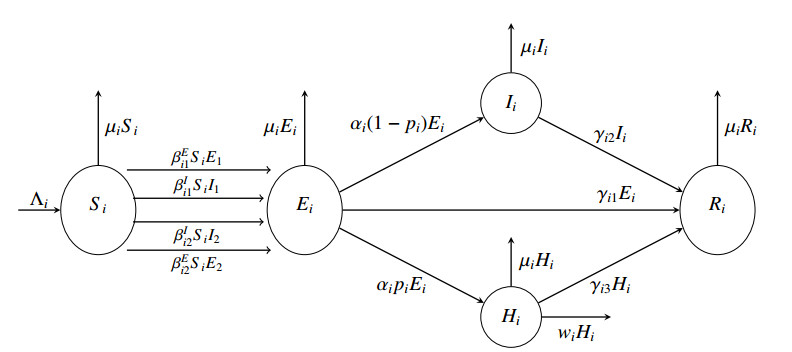
We propose a mathematical model based on a system of differential equations, which incorporates the impact of the chronic health conditions of the host population, to investigate the transmission dynamics of COVID-19. The model divides the total population into two groups, depending on whether they have underlying conditions, and describes the disease transmission both within and between the groups. As an application of this model, we perform a case study for Hamilton County, the fourth-most populous county in the US state of Tennessee and a region with high prevalence of chronic conditions. Our data fitting and simulation results quantify the high risk of COVID-19 for the population group with underlying health conditions. The findings suggest that weakening the disease transmission route between the exposed and susceptible individuals, including the reduction of the between-group contact, would be an effective approach to protect the most vulnerable people in this population group.
Citation: Chayu Yang, Jin Wang. COVID-19 and underlying health conditions: A modeling investigation[J]. Mathematical Biosciences and Engineering, 2021, 18(4): 3790-3812. doi: 10.3934/mbe.2021191
[1] | Haonan Zhong, Wendi Wang . Mathematical analysis for COVID-19 resurgence in the contaminated environment. Mathematical Biosciences and Engineering, 2020, 17(6): 6909-6927. doi: 10.3934/mbe.2020357 |
[2] | Pannathon Kreabkhontho, Watchara Teparos, Thitiya Theparod . Potential for eliminating COVID-19 in Thailand through third-dose vaccination: A modeling approach. Mathematical Biosciences and Engineering, 2024, 21(8): 6807-6828. doi: 10.3934/mbe.2024298 |
[3] | Beatriz Machado, Liliana Antunes, Constantino Caetano, João F. Pereira, Baltazar Nunes, Paula Patrício, M. Luísa Morgado . The impact of vaccination on the evolution of COVID-19 in Portugal. Mathematical Biosciences and Engineering, 2022, 19(1): 936-952. doi: 10.3934/mbe.2022043 |
[4] | Fang Wang, Lianying Cao, Xiaoji Song . Mathematical modeling of mutated COVID-19 transmission with quarantine, isolation and vaccination. Mathematical Biosciences and Engineering, 2022, 19(8): 8035-8056. doi: 10.3934/mbe.2022376 |
[5] | Sarafa A. Iyaniwura, Musa Rabiu, Jummy F. David, Jude D. Kong . Assessing the impact of adherence to Non-pharmaceutical interventions and indirect transmission on the dynamics of COVID-19: a mathematical modelling study. Mathematical Biosciences and Engineering, 2021, 18(6): 8905-8932. doi: 10.3934/mbe.2021439 |
[6] | Xiaojing Wang, Yu Liang, Jiahui Li, Maoxing Liu . Modeling COVID-19 transmission dynamics incorporating media coverage and vaccination. Mathematical Biosciences and Engineering, 2023, 20(6): 10392-10403. doi: 10.3934/mbe.2023456 |
[7] | Haiyan Wang, Nao Yamamoto . Using a partial differential equation with Google Mobility data to predict COVID-19 in Arizona. Mathematical Biosciences and Engineering, 2020, 17(5): 4891-4904. doi: 10.3934/mbe.2020266 |
[8] | Shi Zhao . Estimating the time interval between transmission generations when negative values occur in the serial interval data: using COVID-19 as an example. Mathematical Biosciences and Engineering, 2020, 17(4): 3512-3519. doi: 10.3934/mbe.2020198 |
[9] | Matthew Hayden, Bryce Morrow, Wesley Yang, Jin Wang . Quantifying the role of airborne transmission in the spread of COVID-19. Mathematical Biosciences and Engineering, 2023, 20(1): 587-612. doi: 10.3934/mbe.2023027 |
[10] | Youtian Hao, Guohua Yan, Renjun Ma, M. Tariqul Hasan . Linking dynamic patterns of COVID-19 spreads in Italy with regional characteristics: a two level longitudinal modelling approach. Mathematical Biosciences and Engineering, 2021, 18(3): 2579-2598. doi: 10.3934/mbe.2021131 |
We propose a mathematical model based on a system of differential equations, which incorporates the impact of the chronic health conditions of the host population, to investigate the transmission dynamics of COVID-19. The model divides the total population into two groups, depending on whether they have underlying conditions, and describes the disease transmission both within and between the groups. As an application of this model, we perform a case study for Hamilton County, the fourth-most populous county in the US state of Tennessee and a region with high prevalence of chronic conditions. Our data fitting and simulation results quantify the high risk of COVID-19 for the population group with underlying health conditions. The findings suggest that weakening the disease transmission route between the exposed and susceptible individuals, including the reduction of the between-group contact, would be an effective approach to protect the most vulnerable people in this population group.
COVID-19 has been a global pandemic for more than one year, with over 100 million cases reported throughout the world. In the United States (US) alone, COVID-19 already led to nearly 30 million cases and over half million deaths, as of early March, 2021. The elderly and those with chronic conditions have been among the most vulnerable groups for the COVID-19 infection [1,2].
It is estimated that 22% of the global population, or 1.7 billion people, have at least one underlying health conditions that put them at higher risk for severe COVID-19 associated illness, and that 4% of the global population, or 349 million people, would require hospital admission if infected with COVID-19 [3]. A recent study conducted by CDC reports that among COVID-19 cases, the most common underlying health conditions are cardiovascular disease (32%), diabetes (30%), and chronic lung disease (18%). It is also found that among those with reported underlying conditions, hospitalizations were 6 times higher and deaths were 12 times higher compared to those without an underlying condition [4]. In another study, it is found that among 3,142 US counties, the median estimate of the prevalence of any of five underlying medical conditions (chronic obstructive pulmonary disease, heart disease, diabetes, chronic kidney disease, and obesity) associated with increased risk of severe COVID-19 infection among adults is 47.2%. Counties with the highest prevalences of these health conditions are concentrated in Alabama, Mississippi, Tennessee, and several other southeastern states [5].
The widespread presence of underlying health conditions plays a significant role in raising the numbers of severe COVID-19 infections and subsequent hospitalizations, in contributing to the disease-induced mortality rates, and in shaping the overall pattern of the COVID-19 epidemics. On the other hand, the quantitative relationship between the transmission and spread of COVID-19 and the underlying health conditions of the population remains unclear at present, which hinders our further understanding of COVID-19 dynamics and the design of effective control strategies to protect the most vulnerable [6,7]. In this work, we propose to use mathematical modeling to study this relationship and to quantify the impact of chronic conditions on the COVID-19 transmission dynamics. Thus far, there have been a large number of mathematical, statistical and computational models developed to study the transmission and spread of COVID-19 and to forecast its epidemic development (see, e.g., [8,9,10,11,12,13,14,15,16,17] and references therein). However, to our knowledge, none of these models have been designed to investigate the effects of the chronic medical conditions on the incidence, prevalence and transmission of COVID-19 and associated severe illness.
Our aim is to develop a general modeling framework that can quantify the correlation between COVID-19 transmission and underlying medical conditions, and predict the specific numbers of the infected individuals with underlying conditions and those without such health conditions. To that end, we divide the host population into two groups, depending on whether or not they have underlying health conditions. Individuals within each group are classified into the susceptible, exposed, infected, hospitalized, and recovered compartments, where both the exposed and infected individuals are capable of transmitting the disease, and where the hospitalized compartment contains individuals with severe COVID-19 infection. Our model then describes the disease progression within each group as well as the cross-transmission of the disease between the two groups.
As a demonstration of our modeling work, we apply it to study the transmission of COVID-19 in Hamilton County, the fourth-most populous county in the US state of Tennessee. The total population of Hamilton County is 367,804 [18] and Chattanooga, the fourth-largest city in Tennessee, is its county seat. With several cities, towns, census-designated places and unincorporated communities, Hamilton County forms a region that combines both urban and rural areas. Its racial makeup is about 74.75% White and 25.25% other races. With age-adjusted death rates in the region 13.7% higher than national averages, and with the prevalence of chronic heart disease, chronic obstructive pulmonary disease, diabetes, and obesity exceeding national rates, a significant portion of the population in Hamilton County is considered highly vulnerable to COVID-19 [19]. Through a collaboration with the Chattanooga COVID-19 Data and Analytics Working Group [20], the authors of this work have been provided and continuously updated with detailed epidemic, demographic and health data for Hamilton County.
We implement our mode for Hamilton County in the time period from December 1st, 2020 to February 28th, 2021. After almost a year since COVID-19 was first reported, the general public already have a good understanding of the disease risk and get used to the social distancing normal. We thus assume that there was no significant change of human behavior during this three-month period, which allows the parameters, particularly the transmission rates, in our model to be reasonably approximated as constants [21]. On the other hand, December 2020 marked a time when the second wave of COVID-19 was spreading throughout the US. Our modeling study allows us to investigate the development and progression of this epidemic wave, taking into account the underlying health conditions of the host population, and make near-term predictions of the future evolution of COVID-19.
The remainder of this paper is organized as follows. Section 2 presents the mathematical formulation of our two-group model. Section 3 discusses parameter values, with a focus on the estimation of the transmission rates through data fitting. Section 4 conducts a sensitivity analysis to the model parameters in terms of the state variables and the basic reproduction number. Section 5 presents simulation results and near-term forecasts for the epidemic progression of COVID-19. Section 6 concludes the paper with some discussion.
We propose a mathematical model based on differential equations to investigate the transmission dynamics of COVID-19, with an emphasis on the relationship between the disease transmission and the chronic health conditions of the hosts. We divide the total human population into two groups: Group Ⅰ consists of individuals without underlying health conditions, and Group Ⅱ consists of individuals with at least one underlying health conditions. We partition each group i (i=1,2) into five compartments, including the susceptible individuals (denoted by Si), the exposed individuals (denoted by Ei), the infected but non-hospitalized individuals (denoted by Ii), the hospitalized individuals (denoted by Hi), and the recovered individuals (denoted by Ri).
Both the exposed and infected individuals are assumed to be infectious and are capable of transmitting the disease to susceptible individuals [22,23,24]. The exposed compartment in our model is treated the same as a pre-symptomatic or asymptomatic compartment in other studies [11,25]. Individuals in the exposed compartment typically do not show symptoms and have not been tested/confirmed; they may either recover directly from the exposed state, or transfer into the infected and hospitalized states after an incubation period. Individuals in the infected compartment have tested positive but only show minor or moderate symptoms. They are typically advised, though not in a mandatory manner, to self-quarantine at home until full recovery. Individuals in the hospitalized compartment have tested positive and are at high risk that necessitates hospital admission. We assume that disease-induced deaths only occur in hospitalized individuals. We also assume that hospitalized individuals do not have contact with the public due to their strict isolation, and they will not transmit the disease to others.
Our two-group COVID-19 model is described by the following system. A flow diagram for this model is given in Figure 1.
Group Ⅰ: Individuals without underlying health conditions.
dS1dt=Λ1−βE11S1E1−βI11S1I1−βE12S1E2−βI12S1I2−μ1S1,dE1dt=βE11S1E1+βI11S1I1+βE12S1E2+βI12S1I2−(γ11+α1+μ1)E1,dI1dt=α1(1−p1)E1−(γ12+μ1)I1,dH1dt=α1p1E1−(w1+γ13+μ1)H1,dR1dt=γ11E1+γ12I1+γ13H1−μ1R1. | (2.1) |
Group Ⅱ: Individuals with underlying health conditions.
dS2dt=Λ2−βE21S2E1−βI21S2I1−βE22S2E2−βI22S2I2−μ2S2,dE2dt=βE21S2E1+βI21S2I1+βE22S2E2+βI22S2I2−(γ21+α2+μ2)E2,dI2dt=α2(1−p2)E2−(γ22+μ2)I2,dH2dt=α2p2E2−(w2+γ23+μ2)H2,dR2dt=γ21E2+γ22I2+γ23H2−μ2R2. | (2.2) |
For each group i (i=1,2), Λi is the population influx rate, μi is the natural death rate, αi is the incubation rate, pi is the portion of exposed individuals who become severely ill and hospitalized after the incubation period, γi1, γi2 and γi3 are the rates of recovery from the exposed, infected (non-hospitalized), and hospitalized individuals, respectively, and wi is the disease-induced death rate. The parameters βEij and βIij (i,j=1,2) are the transmission rates between compartments Si and Ej, and between compartments Si and Ij, respectively. We assume that all these parameters are constants, and their values are discussed in the next section.
We list the model parameters and their base values in Table 1. For those parameters whose base values are not available, we will use data fitting to estimate their values. The total population of the region in this study (Hamilton County) is N=367,804 [18]. According to an estimate from CDC [5], about 40% of the population have at least one underlying health conditions. We thus set the population sizes of the two groups as N1=0.6N and N2=0.4N. We calculate the influx rate of susceptible individuals in each group (i=1,2) by Λi=μiNi, where we take μ1=μ2=μ as the natural birth and death rate in the region. The incubation period of the infection ranges between 2 and 14 days, with a mean of 5–7 days [26]. We choose the base value of α−11=α−12=7 days in our model. Among individuals who have tested positive, the portion of severe infections, which would lead to hospitalization, ranges from 5% to 20% [27]. A recent study conducted by CDC found that hospitalizations were 6 times higher and deaths were 12 times higher among those with reported underlying conditions, compared to those with none reported [4]. We thus take the values p1=0.03, p2=0.18, and w1=1.2×10−3, w2=1.44×10−2. The recovery period from COVID-19 has a wide variation (1.5–30 days) among different patients [27], depending on their severities, ages, and overall health conditions. In our model, disease recovery occurs in the exposed, infected, and hospitalized compartments. Those who recover directly from the exposed state typically exhibit no symptoms and have a fast recovery; we set their average recovery period as 5 days in the model, which gives γ11=γ21=0.2 per day. Most of the infected individuals, with minor or moderate symptoms, may recover without going to a hospital; we set their average recovery rates as γ12=0.12 per day and γ22=0.08 per day. The hospitalized individuals, typically with more severe symptoms, may need a longer recovery period; on the other hand, they receive intensive medical treatment which may accelerate their recovery process. Moreover, it is observed that the length of the average hospital stay for COVID-19 patients with chronic health conditions is 1.5 times that for those without underlying conditions [28]. Hence, we take their average recovery rates γ13=0.12 per day and γ23=0.08 per day as well.
Parameter | Description | Value | Source |
N | Population size in Hamilton County | 367804 p | [18] |
μ | Natural birth and death rate | 2.74×10−5/d | [19] |
α1 | Incubation rate in group Ⅰ | 1/7/d | [26] |
α2 | Incubation rate in group Ⅱ | 1/7/d | [26] |
p1 | Ratio of hospitalization in group Ⅰ | 0.03 | [4,27] |
p2 | Ratio of hospitalization in group Ⅱ | 0.18 | [4,27] |
w1 | Disease-induced death rate in group Ⅰ | 0.0012/d | [2,4] |
w2 | Disease-induced death rate in group Ⅱ | 0.0144/d | [2,4] |
γ11 | Recovery rate of exposed individuals in group Ⅰ | 0.2/d | [27] |
γ21 | Recovery rate of exposed individuals in group Ⅱ | 0.2/d | [27] |
γ12 | Recovery rate of infected individuals in group Ⅰ | 0.12/d | [27,28] |
γ22 | Recovery rate of infected individuals in group Ⅱ | 0.08/d | [27,28] |
γ13 | Recovery rate of hospitalized individuals in group Ⅰ | 0.12/d | [27,28] |
γ23 | Recovery rate of hospitalized individuals in group Ⅱ | 0.08/d | [27,28] |
βE11 | Transmission rate between S1 and E1 | fitting by data | – |
βE12 | Transmission rate between S1 and E2 | fitting by data | – |
βE21 | Transmission rate between S2 and E1 | fitting by data | – |
βE22 | Transmission rate between S2 and E2 | fitting by data | – |
βI11 | Transmission rate between S1 and I1 | fitting by data | – |
βI12 | Transmission rate between S1 and I2 | fitting by data | – |
βI21 | Transmission rate between S2 and I1 | fitting by data | – |
βI22 | Transmission rate between S2 and I2 | fitting by data | – |
Other parameter include the 8 transmission rates βEij and βIij (i,j=1,2), which typically vary from place to place and from time to time. Prior studies [11,15,16] have shown that the transmission rates are especially sensitive for COVID-19 modeling and have significant impact on the model output. In this study, we estimate all these transmission rates through data fitting, based on the regional COVID-19 data for Hamilton County [20].
We start our numerical study on December 1, 2020, when the second wave of COVID-19 was spreading throughout the US. We run the simulation and data fitting for a three-month period (until February 28, 2021). Using the demographic and epidemic data reported in Hamilton County [19,20], we set the initial conditions as: S1(0)=203164,E1(0)=2000,I1(0)=3000,H1(0)=18,R1(0)=13000;S2(0)=144315,E2(0)=300,I2(0)=500,H2(0)=107,R2(0)=2000. Figure 2 shows the reported number of cumulative confirmed cases in Hamilton County versus our fitting curve in this three-month period. We observe a high degree of match between our simulation result and the reported data. The parameter values found through the data fitting and their 95% confidence intervals (CI) are presented in Table 2.
Parameter | Fitted value | 95% CI | Parameter | Fitted value | 95% CI |
βE11 | 1.77×10−6 | (0,3.25×10−5) | βI11 | 2.10×10−9 | (0,5.04×10−5) |
βE12 | 2.50×10−7 | (0,3.13×10−4) | βI12 | 2.63×10−10 | (0,1.71×10−5) |
βE21 | 2.76×10−7 | (0,5.67×10−4) | βI21 | 3.91×10−10 | (0,3.44×10−5) |
βE22 | 9.36×10−7 | (0,1.35×10−4) | βI22 | 8.55×10−11 | (0,1.04×10−6) |
In order to quantify the goodness-of-fit, we calculate the normalized mean square error (NMSE), which is defined by
NMSE=n∑ni=1(yi−^yi)2(∑ni=1yi)(∑ni=1^yi), |
where yi (1≤i≤n) are the reported data, ^yi (1≤i≤n) are the simulated data, and n is the number of data points used. In general, a lower value of NMSE indicates a better quality of fitting. We find that the NMSE for our data fitting is 0.00023.
In Eq (A3) of the Appendix, we have derived the basic reproduction number R0 for our model. In Theorem A1.1, we have shown that when R0<1, the disease would be eradicated. Based on the parameter values in Tables 1 and 2, we are able to evaluate the basic reproduction number in this region, and we find that R0≈1.16, which is consistent with the persistence of the disease during these three months.
In addition, we observe in Table 2 that βE11,βE12,βE21 and βE22 are much higher in values than βI11,βI12,βI21 and βI22, indicating that the exposed individuals play a significantly larger role than that of the infected individuals in the disease transmission and spread. This can be clearly expected since infected individuals who have tested positive are generally recommended or required to quarantine at home, while those severely infected ones are treated and isolated in hospitals, and so they possess a lower risk in transmitting the disease compared to the exposed individuals who are asymptomatic but infectious. Meanwhile, among the four parameters associated with the exposed-to-susceptible transmission route, we see that βE11 is the largest and βE22 is the second largest, and even the second largest one is about four times of the values of βE12 and βE22, showing that the contact and transmission within each group may play a more important role than the cross-transmission between the two groups (Ⅰ and Ⅱ).
Our model involves a relatively large number of parameters. To investigate changes of which parameters have higher impact on model output, we conduct a sensitivity analysis of the parameters with respect to the state variables and the basic reproduction number. We consider the following 20 parameters, βE11,βE12,βE21,βE22, βI11,βI12,βI21,βI22, γ11,γ12,γ13, γ21,γ22,γ23, α1,α2, p1,p2,w1, and w2, in our model. The sensitivity of the state variables measures the influence of parameters on model prediction, whereas the sensitivity of the basic reproduction number quantifies the influence of parameters in shaping the disease risk.
We first employ the basic differential equation analysis approach [29] to derive the sensitivity equations for our system (2.1) and (2.2). Denote the sets of state variables by
X1={S1,E1,I1,H1,R1},X2={S2,E2,I2,H2,R2}, |
and the set of parameters by
P={βE11,βE12,βE21,βE22,βI11,βI12,βI21,βI22,γ11,γ12,γ13,γ21,γ22,γ23,α1,α2,p1,p2,w1,w2}. |
For Xi∈Xi and y∈P, we define the relative sensitivity s(Xi,y) of the state Xi to the parameter y, non-dimensionalized by the state Xi and the parameter value y, as
s(Xi,y)=∂Xi∂y⋅yXi,Xi≠0,i=1,2. | (4.1) |
To compute the partial derivative ∂Xi∂y, which is also referred to as a quasi-state variable, we differentiate it with respect to t to obtain
∂∂t(∂Xi∂y)=∂∂y(∂Xi∂t),Xi∈Xi,y∈P,i=1,2. | (4.2) |
We then numerically solve for the quasi-state solutions {∂Xi∂y:Xi∈Xi,y∈P,i=1,2} by associating systems (2.1) and (2.2) with system (4.2).
A typical set of results are presented in Figure 3, where we list the relative sensitivities of the state variables H1, H2, I1 and I2 with respect to the most sensitive parameters in the set P. Unlisted parameters have low sensitivities that are very close to 0. We clearly observe that βE11 has the highest sensitivity for H1, H2 and I1, and the second highest sensitivity for I2, which implies that the exposed-to-susceptible transmission route within Group Ⅰ has a major impact on the epidemic progression. Meanwhile, we see the other three parameters associated with the exposed-to-susceptible transmission route; i.e., βE12, βE21 and βE22, are also sensitive for all the four state variables, consistent with our observation from the data fitting result. Additionally, we find that the incubation rates (represented by αi), recovery rates (represented by γij), and hospitalization ratios (represented by pi), are also among the sensitive parameters for the four state variables. We will further explore the impact of these sensitive parameters on the simulation results in section 5.
Next, we use the expression in equation (A3) to compute the relative sensitivity of the basic reproduction number R0 with respect to each parameter y∈P; i.e., ∂R0∂y⋅yR0. The results are listed in Table 3, where the parameters are ranked in terms of their sensitivities. We observe a general pattern consistent with that in Figure 3. In particular, we observe that the four transmission rates βE11, βE22, βE12 and βE21 have the highest sensitivity for R0, indicating that the changes of their values would have most significant impact on the reduction of the basic reproduction number. According to Theorem A1.1, reducing R0 below unity would eradicate the infection. Consequently, disease control measures reducing the contact rate (such as social distancing) or the transmission probability (such as vaccination) between the exposed and susceptible individuals, may be most efficient in containing the COVID-19 epidemic.
Rank | Parameter | Sensitivity | Rank | Parameter | Sensitivity |
1 | βE11 | 0.828 | 11 | γ22 | 3.12×10−5 |
2 | βE22 | 5.26×10−2 | 12 | βI21 | 2.56×10−5 |
3 | βE12 | 1.57×10−2 | 13 | βI12 | 2.42×10−5 |
4 | βE21 | 1.56×10−2 | 14 | α2 | 1.82×10−5 |
5 | γ12 | 1.17×10−3 | 15 | βI22 | 7.04×10−6 |
6 | βI11 | 1.14×10−3 | 16 | p2 | 6.85×10−6 |
7 | α1 | 6.81×10−4 | 17 | γ13 | 0 |
8 | γ11 | 6.80×10−4 | 18 | γ23 | 0 |
9 | γ21 | 1.82×10−4 | 19 | w1 | 0 |
10 | p1 | 3.61×10−5 | 20 | w2 | 0 |
Moreover, we visualize the variations of R0 with respect to each transmission rate in Figure 4. Specifically, we change the value of each transmission rate from 50% to 200% of its base value in Table 2, and use Eq (A3) to calculate R0 correspondingly. Figures 4a and 4b again show that R0 is typically more sensitive to βEij than to βIij, i,j=1,2, which is another piece of evidence that exposed individuals play a greater role than that of infected individuals in the disease transmission and spread.
Our data fitting and numerical simulation are conducted on the three-month period from December 1, 2020 to February 28, 2021. Figure 5 displays the exposed cases, infected cases, and hospitalized cases in Group Ⅰ (without underlying health conditions) and Group Ⅱ (with underlying health conditions). We observe that the numbers of exposed and infected individuals in Group Ⅰ are much higher than those in Group Ⅱ (see Figure 5a, b). These differences can be possibly explained by: (1) the size of Group Ⅰ is larger than that of Group Ⅱ; (2) individuals in Group Ⅰ, considered as more healthy, generally have a higher level of physical activity, including mobility and personal contact, than that for individuals in Group Ⅱ; and (3) individuals in Group Ⅱ, aware of their underlying health conditions, are generally more cautious about the infection risk of COVID-19. On the other hand, Figure 5c, d shows that the numbers of hospitalizations and deaths in Group Ⅰ are significantly lower than those in Group Ⅱ, since individuals with underlying health conditions have a much higher chance to develop severe illness due to COVID-19. In particular, the number of disease-induced deaths in Group Ⅰ only increased slightly and remained at a very low level during the three-month period, whereas the number of deaths in Group Ⅱ increased by more than 100 in the same period. For the hospitalized cases, the curves for both groups reach a peak around December 30, several days behind the peaks of the exposed and infected cases, and then decline afterwards. The time interval for the occurrence of the peak values of the exposed, infected and hospitalized cases in both groups coincide with the Christmas–New Year holiday period, a reflection of the impact of the increased mobility and personal contact due to holiday travels.
Figure 5 provides base scenarios of our model simulation from December 1, 2020 to February 28, 2021, using transmission rates estimated from data fitting that are presented in Table 2. Also, from the same table, we have observed that the values of the within-group transmission rates βE11 and βE22 are much higher than those of the inter-group transmission rates βE12 and βE21. In order to quantify the role played by the cross-transmission between the two groups, we simulate a hypothetical scenario where there is no communication between the two groups; i.e., the two groups are decoupled from each other. Effectively, we set the four between-group transmission rates βE12, βE21, βI12 and βI21 to zero, and run the model simulation. Results for the numbers of exposed and infected individuals are presented in Figure 6. Compared to Figures 5a and 5b, we see that the curves for Group Ⅰ only have slight changes, whereas the curves for Group Ⅱ are dramatically different. Without the between-group transmission, the numbers of exposed and infected individuals in Group Ⅱ would both quickly approach zero. This pattern implies that the cross-transmission has a minor effect on the Group Ⅰ disease dynamics, but it is critical for the disease progression and persistence in Group Ⅱ. Neglecting such cross-transmission would severely underestimate the disease risk for Group Ⅱ.
A major concern of the health administrations is whether the hospital capacity can meet the demands of COVID-19 patients with severe illness. This underscores the importance of accurate simulation and prediction of hospitalizations that result from the COVID-19 infection. Our model is capable of computing the number of hospitalized cases from each of the two population groups. As a means to validate our model simulation, we calculate the total number of hospitalizations; i.e., H1+H2 in our model, and compare with the reported hospitalized cases from December 1, 2020 to February 28, 2021. Figure 7 depicts this comparison, and we observe a similar trend and reasonably good agreement between these two sets of (reported and simulated) data. In particular, we notice that the peak values of the hospitalized cases, for both the reported and simulated data, occur around December 30, similar to what we observed in Figure 5c. Additionally, we have also plotted in Figure 7 the simulation result for the hypothetical scenario where the inter-group transmission is removed, and we again observe a significant underestimate for the number of hospitalizations.
The results in Figures 6 and 7 imply that, from the disease control point of view, reducing the between-group contact could be a strategic approach to bring down the exposed, infected and hospitalized cases in Group Ⅱ, and to effectively protect the individuals with underlying health conditions.
In what follows, we use our model to make predictions for the near future with regard to COVID-19 epidemic development in Hamilton County. Figure 8 shows the simulation results for the numbers of exposed, infected, and hospitalized cases (two groups combined) for the one-month period from March 1, 2021 to March 31, 2021, based on the parameter values in Tables 1 and 2. We clearly see that all the three curve move downward, a continuation of the decline of the epidemic from the previous two months (see Figure 5). In particular, the decrease of the infected cases is substantial.
Figure 8 is regarded as a base scenario for our model prediction in the near term (March 1 to March 31, 2021). Since our model involves many parameters, and since some of these parameters are considerably sensitive (see section 4 for our sensitivity analysis results), we perform a detailed simulation study to quantify the changes of the model predictions when the values of these most sensible parameters vary.
We first study the variation of the recovery rates. The parameters γi1, γi2 and γi3 in our model represent the recovery rates of the exposed, infected and hospitalized individuals, respectively, in group i (i=1,2). We consider a scenario where each recovery rate is reduced to 75% of its base value, and present the simulation result for the same period in Figure 9a. In comparison with Figure 8, we see that the decline of the exposed and infected cases slows down in Figure 9a, while there is little change to the number of hospitalizations. Meanwhile, we consider another scenario where each recovery rate is increased to 125% of its base value, and present the simulation result in Figure 9b. We see that the numbers of the exposed, infected and hospitalized individuals all decrease much faster, compared with Figure 8. The variation of recovery rates could be caused by factors such as the change of environmental conditions, the improvement of medical care standards in the region, the evolution of the immunity level in the host population, and the mutation of the viral strains. The results in Figure 9 demonstrate that higher (lower) recover rates would accelerate (slow down) the elimination of the epidemic.
We also consider the impact of the incubation periods on the epidemic progression. The parameters αi in our model represent the incubation rate (i.e., the reciprocal of the incubation length) in group i (i=1,2), and their base values are α1=α2=1/7 per day. Similar to recovery rates, the incubation rates could change due to the health conditions of the hosts and the characteristics of the coronavirus. Figure 10 shows the simulation results with decreased incubation rates α1=α2=0.1 per day, and increased rates α1=α2=0.2 per day, while other parameters are all fixed. Comparing Figures 10a and 10b, we see that there is little difference for the numbers of exposed and hospitalized individuals, while the impact is more significant on the number of infected individuals: larger values of incubation rates correspond to shorter incubation periods, resulting in a stronger influx into the infected class which leads to a higher level of infection.
Next, we consider the variation of the transmission rates. As discussed in previous sections, the transmission rates are sensitive to both the state variables and the basic reproduction number. The COVID-19 vaccination campaign is currently on-going throughout the US, with over 2 million shots administered each day. For Hamilton County, about 15.5% of the total population had been at least partially vaccinated as of March 5, 2021 [30]. As the vaccination coverage quickly increases, the probability of human hosts contracting the coronavirus will decrease, which will effectively reduce the disease transmission rates. Here we consider three possible scenarios where all the transmission rates are reduced to 90%, 75%, and 70%, respectively, and another (more hypothetical) scenario where all the transmission rates are reduced to 50%, of their respective base values given in Table 2. In other words, we assume that the disease transmission would be only 90%, 75%, 70% and 50% effective, respectively, during the month of March 2021, compared to that in previous three months. The simulation results are presented in Figure 11. As can be naturally expected, the reduction of transmission rates quickly brings down the numbers of the exposed, infected, and hospitalized individuals, and the curves all approach zero in the more hypothetical case with only 50% effective transmission.
In addition, we examine the changes of the hospitalization ratios p1 and p2 and their impact on the model prediction. The base values are p1=0.03 and p2=0.18 in our model. Figure 12a depicts the simulation result for decreased hospitalization ratios p1=0.01 and p2=0.06, and Figure 12b depicts the simulation result for increased hospitalization ratios p1=0.05 and p2=0.3. The two sets of results shows very little difference for the exposed and infected cases, while it is noticeable that the hospitalized cases decline faster with the reduced hospitalization ratios, which could be possibly achieved through the on-going vaccination campaign that places individuals with underlying health conditions into a priority group.
Finally, we discuss another modeling scenario concerned with the disease transmission by exposed individuals. In our model system (2.1) and (2.2), a person in the exposed compartment E is essentially regarded as a pre-symptomatic or asymptomatic infectious individual who can directly transmit COVID-19 to susceptible people [22,23,24,25]. For comparison, we now assume that exposed individuals in compartment E are latent and not capable of transmitting the disease [31]. To that end, we remove the exposed-to-susceptible transmission route by setting the transmission rates βEij=0 (i,j=1,2) in system (2.1) and (2.2). In this way we obtain a two-group system where each group allows only the infected-to-susceptible transmission route and is more like a traditional SEIR model. We then conduct data fitting to estimate the four transmission rates βIij (i,j=1,2) using the same reported data from December 1, 2020 to February 28, 2021. The fitting curve for the cumulative cases is shown in Figure 13. The normalized mean square error (NMSE) for this data fitting is 0.00071, in comparison to 0.00023 for our original model fitting (see Figure 2). The parameter values found through the fitting and their 95% confidence intervals are presented in Table 4. Based on these values, we find that the basic reproduction number in this case is given by R0≈1.12, which is comparable to our estimate of 1.16 for the original model (see section 3).
Parameter | Fitted value | 95% Confidence Interval |
βI11 | 1.53×10−6 | (1.38×10−8,3.05×10−6) |
βI12 | 1.36×10−7 | (0,3.67×10−6) |
βI21 | 3.30×10−8 | (0,5.40×10−7) |
βI22 | 3.23×10−10 | (0,2.57×10−8) |
Using the parameter values from this data fitting, we numerically calculate the total number of hospitalizations, and the simulation result versus the reported hospitalized cases in the three-month period (from December 1, 2020 to February 28, 2021) are presented in Figure 14. We observe that the modified model significantly underestimates the hospitalized cases, in comparison to Figure 7 where the simulation result for the original model is represented in the solid line. Since the hospitalized individuals are mainly those with underlying health conditions, this result indicates that neglecting the exposed-to-susceptible transmission route would underrate the infection risk for the more vulnerable population group (i.e., Group Ⅱ). The finding appears to be consistent with our previous observations, including the sensitivity analysis in section 4, that the transmission rates βEij (i,j=1,2) play an important role in shaping the overall transmission pattern and infection risk. Hence, our original model (2.1)(2.2) seems to be a better choice in addressing the correlation between COVID-19 transmission and underlying medical conditions, the main goal of this study.
We have presented a new mathematical model to investigate the relationship between the transmission and spread of COVID-19 and the underlying health conditions of the host population. The model divides the population into two groups based on the presence/absence of chronic conditions, and incorporates the transmission of the disease both within and between groups. As a demonstration of our model application, we have performed a case study for Hamilton County in the US state of Tennessee, a typical place with high prevalence of chronic health conditions.
With all the transmission rates estimated by parameter fitting based on the regional data, we have conducted a detailed numerical investigation on the numbers of exposed, infected and hospitalized cases that come from individuals with and without chronic conditions. Our simulation results agree well with the reported data. We have also conducted extensive simulations when a number of sensitive parameters change in values, the results of which help us to better understand the progression and evolution of COVID-19 in the near future.
Our simulation results quantify and confirm the high risk of individuals with chronic conditions. Specifically, the population group with underlying health conditions constantly produces much higher numbers of hospitalizations and deaths, compared to the group without underlying conditions. Our findings highlight the importance of weakening the disease transmission route between the exposed and susceptible individuals, for both the population groups, in fighting COVID-19. Social distancing, which reduces the personal contact, and vaccination deployment, which reduces the transmission probability, would both be critical approaches to achieve this goal. In particular, we find that reducing the between-group contact is effective in protecting the vulnerable group against the COVID-19 infection, and this control strategy seems to be productive in bringing down the numbers of infections and hospitalizations for the group with chronic conditions.
The model output predicts a general decline of the COVID-19 epidemic in the near future for the region in this study, even with the variation of several sensitive and important parameters. In particular, the on-going vaccination campaign is expected to continue improving the immunity level in the host population, particularly for those with chronic conditions, and speed up the process of containing the epidemic.
The authors would like to thank Greg Heath, Jesse Houser and Charlie Mix from the Chattanooga COVID-19 Data and Analytics Working Group for providing data resources related to COVID-19 in Hamilton County. The authors would also like to thank the two anonymous reviewers for their comments that have improved the original manuscript. This work was partially supported by the National Institutes of Health under grant number 1R15GM131315.
We derive the basic reproduction number for the proposed two-group COVID-19 model. Note that in the original system (2.1) and (2.2), the compartments Si,Ei, and Ii do not depend on the compartments Hi and Ri (i=1,2). We can thus combine the two subsystems (2.1) and (2.2) and study the following reduced system instead.
dS1dt=Λ1−βE11S1E1−βI11S1I1−βE12S1E2−βI12S1I2−μ1S1,dE1dt=βE11S1E1+βI11S1I1+βE12S1E2+βI12S1I2−(γ11+α1+μ1)E1,dI1dt=α1(1−p1)E1−(γ12+μ1)I1,dS2dt=Λ2−βE21S2E1−βI21S2I1−βE22S2E2−βI22S2I2−μ2S2,dE2dt=βE21S2E1+βI21S2I1+βE22S2E2+βI22S2I2−(γ21+α2+μ2)E2,dI2dt=α2(1−p2)E2−(γ22+μ2)I2. | (A1) |
It is easy to verify that the system (A1) has a unique disease-free equilibrium (DFE) at
x0=(S01,E01,I01,S02,E02,I02)=(Λ1μ1,0,0,Λ2μ2,0,0). | (A2) |
Based on the next-generation matrix technique [32], the new infection matrix F and the transition matrix V are given by
F=[βE11S01βI11S01βE12S01βI12S010000βE21S02βI21S02βE22S02βI22S020000] |
and
V=[u11000−α1(1−p1)u120000u21000−α2(1−p2)u22], |
where ui1=γi1+αi+μi and ui2=γi2+μi for i=1,2. The basic reproduction number R0 is defined as the spectral radius of the next-generation matrix
FV−1=[a11βI11S01u12a12βI12S01u220000a21βI21S01u12a22βI22S02u220000], |
where
a11=βE11S01u11+α1(1−p1)βI11S01u11u12,a12=βE12S01u21+α2(1−p2)βI12S01u21u22,a21=βE21S02u11+α1(1−p1)βI21S02u11u12,a22=βE22S02u21+α2(1−p2)βI22S02u21u22. |
Hence we have
R0=ρ(FV−1)=a11+a22+√(a11−a22)2+4a12a212, | (A3) |
which provides a measurement for the disease risk.
The disease-free equilibrium has a special importance in an epidemic model. Mathematically, it represents a stationary state where there is no infection; practically, it represents the eventual goal of disease control measures: to eliminate the infection. We establish the following result for our two-group COVID-19 model, which indicates that if the basic reproduction number is reduced below unity, then the DFE is globally attractive; i.e., the disease would be eradicated.
Theorem A1.1. If R0<1, the DFE of system (A1) is globally asymptotically stable in
Ω={(S1,E1,I1,S2,E2,I2)∈R6+:S1+E1+I1≤S01,S2+E2+I2≤S02}. |
Proof. Apparently, Ω is a positively invariant set for system (A1). Let X=(E1,I1,E2,I2)T. It is easy to observe that
dXdt≤(F−V)X. |
Since R0=ρ(FV−1)=ρ(V−1F) and V−1F is a positive matrix, then by Perron Theorem, V−1F has a positive left eigenvector u corresponding to the eigenvalue R0; i.e., uV−1F=R0u. Consider the following Lyapunov function
L=uV−1X. |
Differentiating L along the solutions of (A1), we have
dLdt=uV−1dXdt≤uV−1(F−V)X=(R0−1)uX. | (A4) |
Clearly, if R0<1, the equality dLdt=0 implies that uX=0 by Eq (A4). Hence X=0 and thereby S1=S01,E1=0,I1=0,S2=S02,E2=0, and I2=0. Thus, the largest invariant set on which dLdt=0 consists of only the singleton x0=(S01,0,0,S02,0,0). By LaSalle's Invariance Principle [33], the DFE x0 is globally asymptotically stable in Ω if R0<1.
[1] | Centers for Disease Control and Prevention, Coronavirus (COVID-19). Available from: https://www.cdc.gov/coronavirus/2019-ncov. |
[2] | World Health Organization, Coronavirus disease (COVID-19) pandemic. Available from: https://www.who.int/emergencies/diseases/novel-coronavirus-2019. |
[3] |
A. Clark, M. Jit, C. Warren-Gash, B. Guthrie, H. H. X. Wang, S. W. Mercer, et al., Global, regional, and national estimates of the population at increased risk of severe COVID-19 due to underlying health conditions in 2020: A modelling study, Lancet Glob. Health, 8 (2020), e1003–e1017. doi: 10.1016/S2214-109X(20)30264-3
![]() |
[4] |
E. K. Stokes, L. D. Zambrano, K. N. Anderson, E. P. Marder, K. M. Raz, S. E. B. Felix, et al., Coronavirus disease 2019 case surveillance – United States, January 22–May 30, 2020, Morb. Mortal. Wkly. Rep., 69 (2020), 759-765. doi: 10.15585/mmwr.mm6924e2
![]() |
[5] |
H. Razzaghi, Y. Wang, H. Lu, K. E. Marshall, N. F. Dowling, G. Paz-Bailey, et al., Estimated county-level prevalence of selected underlying medical conditions associated with increased risk for severe COVID-19 illness – United States, 2018, Morb. Mortal. Wkly. Rep., 69 (2020), 945–950. doi: 10.15585/mmwr.mm6929a1
![]() |
[6] |
Z. J. Cheng, J. Shan, 2019 Novel coronavirus: where we are and what we know, Infection, 48 (2020), 155–163. doi: 10.1007/s15010-020-01401-y
![]() |
[7] | A. Sahin, A. Erdogan, P. M. Agaoglu, Y. Dineri, A. Cakirci, M. Senel, et al., 2019 novel coronavirus (COVID-19) outbreak: A review of the current literature, Eurasian J. Med. Oncol., 4 (2020), 1–7. |
[8] |
K. Leung, J. T. Wu, D. Liu, G. M. Leung, First-wave COVID-19 transmissibility and severity in China outside Hubei after control measures, and second-wave scenario planning: a modelling impact assessment, Lancet, 395 (2020), 1382–1393. doi: 10.1016/S0140-6736(20)30746-7
![]() |
[9] |
R. Li, S. Pei, B. Chen, Y. Song, T. Zhang, W. Yang, et al., Substantial undocumented infection facilitates the rapid dissemination of novel coronavirus (SARS-CoV2), Science, 368 (2020), 489–493. doi: 10.1126/science.abb3221
![]() |
[10] | J. M. Read, J. R. E. Bridgen, D. A. T. Cummings, A. Ho, C. P. Jewell, Novel coronavirus 2019-nCoV: early estimation of epidemiological parameters and epidemic predictions, MedRxiv, 2020. Available from: https://doi.org/10.1101/2020.01.23.20018549. |
[11] |
B. Tang, X. Wang, Q. Li, N. L. Bragazzi, S. Tang, Y. Xiao, et al., Estimation of the Transmission Risk of 2019-nCoV and Its Implication for Public Health Interventions, J. Clin. Med., 9 (2020), 462. doi: 10.3390/jcm9020462
![]() |
[12] |
J. Wang, Mathematical models for COVID-19: applications, limitations, and potentials, J. Public Health Emerg., 4 (2020), 9. doi: 10.21037/jphe-2020-05
![]() |
[13] |
J. T. Wu, K. Leung, G. M. Leung, Nowcasting and forecasting the potential domestic and international spread of the 2019-nCoV outbreak originating in Wuhan, China: a modelling study, Lancet, 395 (2020), 689–697. doi: 10.1016/S0140-6736(20)30260-9
![]() |
[14] |
C. Yang, J. Wang, A mathematical model for the novel coronavirus epidemic in Wuhan, China, Math. Bios. Eng., 17 (2020), 2708–2724. doi: 10.3934/mbe.2020148
![]() |
[15] |
C. Yang, J. Wang, Modeling the transmission of COVID-19 in the US – A case study, Infect. Disease Modelling, 6 (2021), 195–211. doi: 10.1016/j.idm.2020.12.006
![]() |
[16] |
C. Yang, J. Wang, Transmission rates and environmental reservoirs for COVID-19: A modeling study, J. Biol. Dyn., 15 (2021), 86–108. doi: 10.1080/17513758.2020.1869844
![]() |
[17] |
H. Zhong, W. Wang, Mathematical analysis for COVID-19 resurgence in the contaminated environment, Math. Bios. Eng., 17 (2020), 6909–6927. doi: 10.3934/mbe.2020357
![]() |
[18] | Data USA: Chattanooga, TN. Available from: https://datausa.io/profile/geo/chattanooga-tn/. |
[19] | Hamilton County Health Department. Available from: https://health.hamiltontn.org/default.aspx. |
[20] | COVID-19 in Hamilton County, TN. Available from: https://sites.google.com/view/hamiltoncounty-tn-covid19. |
[21] |
C. Yang, X. Wang, D. Gao, J. Wang, Impact of awareness programs on cholera dynamics: Two modeling approaches, Bull. Math. Biol., 79 (2017), 2109–2131. doi: 10.1007/s11538-017-0322-1
![]() |
[22] |
J. F. W. Chan, S. Yuan, K. H. Kok, K. K. W. To, H. Chu, J. Yang, et al., A familial cluster of pneumonia associated with the 2019 novel coronavirus indicating person-to-person transmission: a study of a family cluster, Lancet, 395 (2020), 514–523. doi: 10.1016/S0140-6736(20)30154-9
![]() |
[23] |
A. Kimball, K. M. Hatfield, M. Arons, A. James, J. Taylor, K. Spicer, et al., Asymptomatic and presymptomatic SARS-CoV-2 infections in residents of a long-term care skilled nursing facility – King County, Washington, March 2020, Morb. Mortal. Wkly. Rep., 69 (2020), 377–381. doi: 10.15585/mmwr.mm6913e1
![]() |
[24] |
W. E. Wei, Z. Li, C. J. Chiew, S. E. Yong, M. P. Toh, V. J. Lee, Presymptomatic transmission of SARS-CoV-2 – Singapore, January 23 - March 16, 2020, Morb. Mortal. Wkly. Rep., 69 (2020), 411–415. doi: 10.15585/mmwr.mm6914e1
![]() |
[25] |
M. A. Johansson, T. M. Quandelacy, S. Kada, P. V. Prasad, M. Steele, J. T. Brooks, et al., SARS-CoV-2 transmission from people without COVID-19 symptoms, JAMA Network Open, 4 (2021), e2035057. doi: 10.1001/jamanetworkopen.2020.35057
![]() |
[26] | J. A. Spencer, D. P. Shutt, S. K. Moser, H. Clegg, H. J. Wearing, H. Mukundan, et al., Epidemiological parameter review and comparative dynamics of influenza, respiratory syncytial virus, rhinovirus, human coronvirus, and adenovirus, MedRxiv, 2020. Available from: http://dx.doi.org/10.1101/2020.02.04.20020404. |
[27] | World Health Organization, Coronavirus disease (COVID-19) situation reports. Available from: https://www.who.int/emergencies/diseases/novel-coronavirus-2019/situation-reports. |
[28] |
Z. S. Almalki, M. F. Khan, S. Almazrou, A. S. Alanazi, M. S. Iqbal, A. Alqahtani, et al., Clinical characteristics and outcomes among COVID-19 hospitalized patients with chronic conditions: A retrospective single-center study, J. Multidiscip. Healthc., 13 (2020), 1089–1097. doi: 10.2147/JMDH.S273918
![]() |
[29] |
L. Ellwein, H. Tran, C. Zapata, V. Novak, M. Olufsen, Sensitivity analysis and model assessment: Mathematical models for arterial blood flow and blood pressure, J. Cardiovasc. Eng., 8 (2008), 94–108. doi: 10.1007/s10558-007-9047-3
![]() |
[30] | COVID-19 Vaccinations in Hamilton County, TN. Available from: https://datastudio.google.com/reporting/1d5c1095-e7a1-4a05-80db-e81e33f323fb/page/mxd0B?s=pj_BWXEzAU8. |
[31] |
H. J. Wearing, P. Rohani, M. J. Keeling, Appropriate models for the management of infectious diseases, PLoS Med., 2 (2005), e174. doi: 10.1371/journal.pmed.0020174
![]() |
[32] |
P. van den Driessche, J. Watmough, Reproduction numbers and sub-threshold endemic equilibria for compartmental models of disease transmission, Math. Bios., 180 (2002), 29–48. doi: 10.1016/S0025-5564(02)00108-6
![]() |
[33] | J. P. LaSalle, The Stability of Dynamical Systems, CBMS-NSF Regional Conference Series in Applied Mathematics, Philadelphia, 1976. |
1. | Samuel Okyere, Joseph Ackora-Prah, Shih Pin Chen, A Mathematical Model of Transmission Dynamics of SARS-CoV-2 (COVID-19) with an Underlying Condition of Diabetes, 2022, 2022, 1687-0425, 1, 10.1155/2022/7984818 | |
2. | Jie Bai, Xiunan Wang, Jin Wang, An epidemic-economic model for COVID-19, 2022, 19, 1551-0018, 9658, 10.3934/mbe.2022449 | |
3. | Matthew Hayden, Bryce Morrow, Wesley Yang, Jin Wang, Quantifying the role of airborne transmission in the spread of COVID-19, 2022, 20, 1551-0018, 587, 10.3934/mbe.2023027 | |
4. | Ziqiang Cheng, Jin Wang, Modeling epidemic flow with fluid dynamics, 2022, 19, 1551-0018, 8334, 10.3934/mbe.2022388 | |
5. | Chayu Yang, Bo Deng, Dynamics of Infectious Diseases Incorporating a Testing Compartment, 2024, 12, 2227-7390, 1797, 10.3390/math12121797 | |
6. | JAI PRAKASH TRIPATHI, NITESH KUMAWAT, KOMAL TANWAR, DHANUMJAYA PALLA, MAIA MARTCHEVA, TRANSMISSION DYNAMICS OF COVID-19 WITH DIABETES IN INDIA: A COST-EFFECTIVE AND OPTIMAL CONTROL ANALYSIS, 2024, 32, 0218-3390, 643, 10.1142/S0218339024500232 | |
7. | Samuel Okyere, Joseph Ackora-Prah, Ebenezer Bonyah, Bennedict Barnes, Maxwell Akwasi Boateng, Ishmael Takyi, Samuel Akwasi Adarkwa, Hypertension and COVID-19 fractional derivative model with double dose vaccination, 2023, 12, 2046-1402, 495, 10.12688/f1000research.133768.1 | |
8. | Jie Bai, Jin Wang, Modeling long COVID dynamics: Impact of underlying health conditions, 2024, 576, 00225193, 111669, 10.1016/j.jtbi.2023.111669 | |
9. | Ziqiang Cheng, Jin Wang, A two-phase fluid model for epidemic flow, 2023, 8, 24680427, 920, 10.1016/j.idm.2023.07.001 |
Parameter | Description | Value | Source |
N | Population size in Hamilton County | 367804 p | [18] |
μ | Natural birth and death rate | 2.74×10−5/d | [19] |
α1 | Incubation rate in group Ⅰ | 1/7/d | [26] |
α2 | Incubation rate in group Ⅱ | 1/7/d | [26] |
p1 | Ratio of hospitalization in group Ⅰ | 0.03 | [4,27] |
p2 | Ratio of hospitalization in group Ⅱ | 0.18 | [4,27] |
w1 | Disease-induced death rate in group Ⅰ | 0.0012/d | [2,4] |
w2 | Disease-induced death rate in group Ⅱ | 0.0144/d | [2,4] |
γ11 | Recovery rate of exposed individuals in group Ⅰ | 0.2/d | [27] |
γ21 | Recovery rate of exposed individuals in group Ⅱ | 0.2/d | [27] |
γ12 | Recovery rate of infected individuals in group Ⅰ | 0.12/d | [27,28] |
γ22 | Recovery rate of infected individuals in group Ⅱ | 0.08/d | [27,28] |
γ13 | Recovery rate of hospitalized individuals in group Ⅰ | 0.12/d | [27,28] |
γ23 | Recovery rate of hospitalized individuals in group Ⅱ | 0.08/d | [27,28] |
βE11 | Transmission rate between S1 and E1 | fitting by data | – |
βE12 | Transmission rate between S1 and E2 | fitting by data | – |
βE21 | Transmission rate between S2 and E1 | fitting by data | – |
βE22 | Transmission rate between S2 and E2 | fitting by data | – |
βI11 | Transmission rate between S1 and I1 | fitting by data | – |
βI12 | Transmission rate between S1 and I2 | fitting by data | – |
βI21 | Transmission rate between S2 and I1 | fitting by data | – |
βI22 | Transmission rate between S2 and I2 | fitting by data | – |
Parameter | Fitted value | 95% CI | Parameter | Fitted value | 95% CI |
βE11 | 1.77×10−6 | (0,3.25×10−5) | βI11 | 2.10×10−9 | (0,5.04×10−5) |
βE12 | 2.50×10−7 | (0,3.13×10−4) | βI12 | 2.63×10−10 | (0,1.71×10−5) |
βE21 | 2.76×10−7 | (0,5.67×10−4) | βI21 | 3.91×10−10 | (0,3.44×10−5) |
βE22 | 9.36×10−7 | (0,1.35×10−4) | βI22 | 8.55×10−11 | (0,1.04×10−6) |
Rank | Parameter | Sensitivity | Rank | Parameter | Sensitivity |
1 | βE11 | 0.828 | 11 | γ22 | 3.12×10−5 |
2 | βE22 | 5.26×10−2 | 12 | βI21 | 2.56×10−5 |
3 | βE12 | 1.57×10−2 | 13 | βI12 | 2.42×10−5 |
4 | βE21 | 1.56×10−2 | 14 | α2 | 1.82×10−5 |
5 | γ12 | 1.17×10−3 | 15 | βI22 | 7.04×10−6 |
6 | βI11 | 1.14×10−3 | 16 | p2 | 6.85×10−6 |
7 | α1 | 6.81×10−4 | 17 | γ13 | 0 |
8 | γ11 | 6.80×10−4 | 18 | γ23 | 0 |
9 | γ21 | 1.82×10−4 | 19 | w1 | 0 |
10 | p1 | 3.61×10−5 | 20 | w2 | 0 |
Parameter | Fitted value | 95% Confidence Interval |
βI11 | 1.53×10−6 | (1.38×10−8,3.05×10−6) |
βI12 | 1.36×10−7 | (0,3.67×10−6) |
βI21 | 3.30×10−8 | (0,5.40×10−7) |
βI22 | 3.23×10−10 | (0,2.57×10−8) |
Parameter | Description | Value | Source |
N | Population size in Hamilton County | 367804 p | [18] |
μ | Natural birth and death rate | 2.74×10−5/d | [19] |
α1 | Incubation rate in group Ⅰ | 1/7/d | [26] |
α2 | Incubation rate in group Ⅱ | 1/7/d | [26] |
p1 | Ratio of hospitalization in group Ⅰ | 0.03 | [4,27] |
p2 | Ratio of hospitalization in group Ⅱ | 0.18 | [4,27] |
w1 | Disease-induced death rate in group Ⅰ | 0.0012/d | [2,4] |
w2 | Disease-induced death rate in group Ⅱ | 0.0144/d | [2,4] |
γ11 | Recovery rate of exposed individuals in group Ⅰ | 0.2/d | [27] |
γ21 | Recovery rate of exposed individuals in group Ⅱ | 0.2/d | [27] |
γ12 | Recovery rate of infected individuals in group Ⅰ | 0.12/d | [27,28] |
γ22 | Recovery rate of infected individuals in group Ⅱ | 0.08/d | [27,28] |
γ13 | Recovery rate of hospitalized individuals in group Ⅰ | 0.12/d | [27,28] |
γ23 | Recovery rate of hospitalized individuals in group Ⅱ | 0.08/d | [27,28] |
βE11 | Transmission rate between S1 and E1 | fitting by data | – |
βE12 | Transmission rate between S1 and E2 | fitting by data | – |
βE21 | Transmission rate between S2 and E1 | fitting by data | – |
βE22 | Transmission rate between S2 and E2 | fitting by data | – |
βI11 | Transmission rate between S1 and I1 | fitting by data | – |
βI12 | Transmission rate between S1 and I2 | fitting by data | – |
βI21 | Transmission rate between S2 and I1 | fitting by data | – |
βI22 | Transmission rate between S2 and I2 | fitting by data | – |
Parameter | Fitted value | 95% CI | Parameter | Fitted value | 95% CI |
βE11 | 1.77×10−6 | (0,3.25×10−5) | βI11 | 2.10×10−9 | (0,5.04×10−5) |
βE12 | 2.50×10−7 | (0,3.13×10−4) | βI12 | 2.63×10−10 | (0,1.71×10−5) |
βE21 | 2.76×10−7 | (0,5.67×10−4) | βI21 | 3.91×10−10 | (0,3.44×10−5) |
βE22 | 9.36×10−7 | (0,1.35×10−4) | βI22 | 8.55×10−11 | (0,1.04×10−6) |
Rank | Parameter | Sensitivity | Rank | Parameter | Sensitivity |
1 | βE11 | 0.828 | 11 | γ22 | 3.12×10−5 |
2 | βE22 | 5.26×10−2 | 12 | βI21 | 2.56×10−5 |
3 | βE12 | 1.57×10−2 | 13 | βI12 | 2.42×10−5 |
4 | βE21 | 1.56×10−2 | 14 | α2 | 1.82×10−5 |
5 | γ12 | 1.17×10−3 | 15 | βI22 | 7.04×10−6 |
6 | βI11 | 1.14×10−3 | 16 | p2 | 6.85×10−6 |
7 | α1 | 6.81×10−4 | 17 | γ13 | 0 |
8 | γ11 | 6.80×10−4 | 18 | γ23 | 0 |
9 | γ21 | 1.82×10−4 | 19 | w1 | 0 |
10 | p1 | 3.61×10−5 | 20 | w2 | 0 |
Parameter | Fitted value | 95% Confidence Interval |
βI11 | 1.53×10−6 | (1.38×10−8,3.05×10−6) |
βI12 | 1.36×10−7 | (0,3.67×10−6) |
βI21 | 3.30×10−8 | (0,5.40×10−7) |
βI22 | 3.23×10−10 | (0,2.57×10−8) |