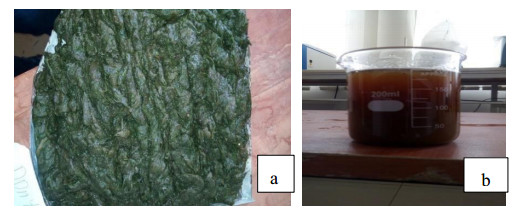
Patients with craniocerebral injury are in serious condition and inconvenient to take care of. This paper proposes a method of extracting the patient's body behavior feature based on convolution neural network, in order to reduce nursing workload and save hospital costs. The algorithm adopts double network model design, including the patient detection network model and the patient's body behavior feature extraction model. The algorithm is applied to the patient's body behavior detection system, so as to realize the recognition and monitoring of patients and improve the level of intelligent medical care for craniocerebral injury. Finally, the open source framework platform is used to test the patient behavior detection system. The experimental results show that the larger the test data set is, the higher the accuracy of patient body behavior feature extraction is. The average recognition rate of patient body behavior category is 97.8%, which verifies the effectiveness and correctness of the system. The application of convolution neural network connects image recognition with intelligent medical nursing, which provides reference and experience for intelligent medical nursing of patients with craniocerebral injury.
Citation: Limei Bai. Intelligent body behavior feature extraction based on convolution neural network in patients with craniocerebral injury[J]. Mathematical Biosciences and Engineering, 2021, 18(4): 3781-3789. doi: 10.3934/mbe.2021190
[1] | Ee Sann Tan, Kumaran Palanisamy, Teuku Meurah Indra Mahlia, Kunio Yoshikawa . Performance and emission study on waste cooking oil biodiesel and distillate blends for microturbine application. AIMS Energy, 2015, 3(4): 798-809. doi: 10.3934/energy.2015.4.798 |
[2] | Aman Santoso, Titania Nur Kusumah, Sumari Sumari, Anugrah Ricky Wijaya, Rini Retnosari, Ihsan Budi Rachman, Siti Marfuah, Muhammad Roy Asrori . Synthesis of biodiesel from waste cooking oil using heterogeneous catalyst of Na2O/γ-Al2O3 assisted by ultrasonic wave. AIMS Energy, 2022, 10(5): 1059-1073. doi: 10.3934/energy.2022049 |
[3] | Maria del Pilar Rodriguez, Ryszard Brzezinski, Nathalie Faucheux, Michèle Heitz . Enzymatic transesterification of lipids from microalgae into biodiesel: a review. AIMS Energy, 2016, 4(6): 817-855. doi: 10.3934/energy.2016.6.817 |
[4] | Shemelis Nigatu Gebremariam, Jorge Mario Marchetti . Biodiesel production technologies: review. AIMS Energy, 2017, 5(3): 425-457. doi: 10.3934/energy.2017.3.425 |
[5] | Lihao Chen, Hu Wu, Kunio Yoshikawa . Research on upgrading of pyrolysis oil from Japanese cedar by blending with biodiesel. AIMS Energy, 2015, 3(4): 869-883. doi: 10.3934/energy.2015.4.869 |
[6] | A. A. Ayoola, F. K. Hymore, C. A. Omonhinmin, O. Agboola, E. E. Alagbe, D. Oyekunle, M. O. Bello . Biodiesel production from used vegetable oil and CaO catalyst impregnated with KNO3 and NaNO3. AIMS Energy, 2020, 8(3): 527-537. doi: 10.3934/energy.2020.3.527 |
[7] | Yadessa Gonfa Keneni, Jorge Mario Marchetti . Oil extraction from plant seeds for biodiesel production. AIMS Energy, 2017, 5(2): 316-340. doi: 10.3934/energy.2017.2.316 |
[8] | Vo Thanh Phuoc, Kunio Yoshikawa . Effect of the storage condition of microalgae on hydrochar lipids and direct esterification-transesterification of hydrochar lipids for biodiesel production. AIMS Energy, 2017, 5(1): 39-53. doi: 10.3934/energy.2017.1.39 |
[9] | Vo Thanh Phuoc, Kunio Yoshikawa . Comparison between direct transesterification of microalgae and hydrochar. AIMS Energy, 2017, 5(4): 652-666. doi: 10.3934/energy.2017.4.652 |
[10] | Douglas Faria, Fernando Santos, Grazielle Machado, Rogério Lourega, Paulo Eichler, Guilherme de Souza, Jeane Lima . Extraction of radish seed oil (Raphanus sativus L.) and evaluation of its potential in biodiesel production. AIMS Energy, 2018, 6(4): 551-565. doi: 10.3934/energy.2018.4.551 |
Patients with craniocerebral injury are in serious condition and inconvenient to take care of. This paper proposes a method of extracting the patient's body behavior feature based on convolution neural network, in order to reduce nursing workload and save hospital costs. The algorithm adopts double network model design, including the patient detection network model and the patient's body behavior feature extraction model. The algorithm is applied to the patient's body behavior detection system, so as to realize the recognition and monitoring of patients and improve the level of intelligent medical care for craniocerebral injury. Finally, the open source framework platform is used to test the patient behavior detection system. The experimental results show that the larger the test data set is, the higher the accuracy of patient body behavior feature extraction is. The average recognition rate of patient body behavior category is 97.8%, which verifies the effectiveness and correctness of the system. The application of convolution neural network connects image recognition with intelligent medical nursing, which provides reference and experience for intelligent medical nursing of patients with craniocerebral injury.
Heavy dependence on fossil fuels has been highly associated with air pollution and irreversible climate changes [1]. Consumption at the current rate (4.234 billion tons per year) makes the globally available fossil fuel reserves last only for a few decades [2]. The fossil fuel-based petroleum fuel currently used in the conventional engine system has been associated with depleting reserves and disastrous environmental concerns. A sustainable alternative fuel from renewable sources such as biodiesel has been suggested to tackle these problems. Biodiesel is sustainable, non-toxic, biodegradable, carbon-neutral, economically competitive, environmentally friendly, miscible with petrodiesel [3], and a better lubricating fuel that can be used in the existing engine with little modifications [4]. Feedstock availability and costs are critical in a sustainable biodiesel production [5]. Biodiesel production from edible feedstock is associated with deforestation and biodiversity loss [6] and food vs. fuel conflict [3]. So far, several attempts have been made to produce biodiesel from single edible oil sources [6]. However, using a single edible oil as feedstock may compromise food security [7] and affect the economic viability of biodiesel production [8]. Additionally, the ever-increasing human population in low-income countries may skyrocket biodiesel production from single edible oil sources. Thus, inexpensive and non-edible mixed oils may reduce biodiesel production costs [9]. Additionally, industrial-scale biodiesel production at a reasonable price requires inexpensive and non-edible oils such as WCOs since they are much cheaper than the edible oils [10,11].
Biodiesel from mixed oil sources can satisfy the rising demands; reduce costs of feedstock; and improve fuel quality, engine performance, and pollutant emission characteristics [9]. Using blended oils may solve the insufficiency of single-oil feedstock [6]. As the cost of feedstock accounts for more than 75% of the total expenses, selecting an appropriate oil mixture is critical for sustainable biodiesel production [12,13]. Mixed-oil ratio is the most important factor influencing the conversion process and fuel quality [6]. Biodiesel yield from blended oils depends mainly on several factors including type of conversion process, fatty acid profile of the feedstock, type and amount of catalyst, and optimization of conversion process parameters. In several studies, the production of biodiesel from multi-feedstock and single feedstock revealed that the multi-feedstock produced better yield [6]. Biodiesel fuel quality from blended oils depends on the fatty acid composition of the individual oils [13]. The mixed oil properties lay mid-way between individual oil properties [5].
Repeated frying makes WCO unsuitable for human consumption due to its higher free fatty acid (FFA) contents [14]. The disposal of WCO is usually associated with the pollution of aquatic and terrestrial environments [15]. Dumping WCO into the sewer blocks the flow of wastewater to the treatment plants with the consequent flooding risk besides lowering effluent quality due to deposits and blockages. The disposal of WCO would also cause soil pollution if dumped into municipal landfills. The use of WCO as feedstock for Biodiesel production can solve the environmental pollutions associated with its disposal [16]. Thus, WCOs can serve as cheap feedstock for biodiesel production [17]. The use of WCOs for biodiesel production is a viable waste management option in alleviating its environmental impacts [3]. The wide availability of WCO makes it one of the preferred feedstocks for biodiesel production. In addition, WCO as a feedstock can reduce biodiesel production costs by 60–90% [15]. Much effort has been made to convert WCOs into biodiesels, but the FFA content and presence of water and impurities are the major obstacles [18]. The biodiesels from WCOs may fulfill the fuel quality specifications specified in the ASTM D6751 and EN 14214 standards, but low biodiesel yields, poor oxidation stability, high kinematic viscosity, and poor cold flow properties are the major issues to overcome [14]. One way to improve the physicochemical and cold flow properties of biodiesels from WCOs is blending them with other non-edible feedstocks such as microalgae oils.
Microalgae have emerged as a sustainable feedstock for biodiesel production [9]. The fast growth rate; higher biomass productivity; efficient photosynthesis; better lipid yield; and a potential to grow in fresh water, brackish water, or on marginalized lands make microalgae a better feedstock for biodiesel production [19]. Microalgae's lipid yield (30–70%) surpasses other edible and non-edible oilseed feedstocks [12]. Moreover, microalgae never compete with food production as they require neither arable land nor agricultural inputs [19]. Microalgae based biodiesel production can be coupled with mitigation of GHG emissions from power plants [20]. Moreover, microalgae have great adaptation to absorb excess nutrients from wastewater which allow them to be used in wastewater treatment plants [21,22]. An open or closed system is usually employed to cultivate microalgae [12]. The open system achieves better algal productivity at reasonable costs but it may be contaminated by undesirable species may contaminate the microalgae, controlling light and temperature is difficult, to control light and temperature and, as well cultivation depends on local conditions and limited to warmer seasons. A closed system with light and CO2 sources promotes high-quality algal biomass throughout the year, solves contamination with undesirable species, and evaporative losses. However, microalgae biomass harvesting and dewatering technologies must be well-established for cost-effective and energy-efficient operations [23]. Microalgal species with good lipid content is crucial to lower the cost of biodiesel [24]. Moreover, genetic engineering and metabolic regulations are crucial to facilitate molecular level overexpression of genes or enzymes responsible for lipid biosynthesis [24]. Such strategies help to develop microalgae strains with high lipid content and accumulation capacities for cost effective biodiesel production. Indeed, the composition and quantity of microalgal lipids can be influenced by external conditions such as light intensity, temperature, carbon dioxide, nutrient starvation, salinity, and metal stresses [25].
The direct use of crude oil in engines creates many problems, including injector coking and carbon deposits on the piston and engine head, poor ignition, and excessive engine wear [26]. The transesterification of triglyceride into biodiesel can be influenced by factors such as alcohol-to-oil molar ratio, catalyst type and concentration, reaction time and temperature, agitation speed, and FFA and water contents of the feedstock [26]. Cost reduction and yield maximization require optimization of transesterification process parameters. This study was aimed at optimizing the transesterification parameters and biodiesel yield from blended MO-WCO.
The chronicles of synthesizing biodiesel from blended feedstocks have been studied under different experimental settings and oil ratios. However, studies pertinent to biodiesel derived from blends of MO and WCO are highly limited or nonexistent. Hence, the current study unfurls to unveil the optimum oil ratio that ignites the transesterification parameters optimization and fuel qualities in order to cast light upon the prospects of large-scale biodiesel production. Utilization of WCO and oil from microalgae grown in domestic wastewater treatment plant for biodiesel production is a reliable strategy in maintaining public health and pollution prevention. Additionally, WCO and MO may serve as cost-effective and sustainable feedstock in industrial scale biodiesel production. The scope of the current study comprises of blending various MO-WCO and selecting a blend with suitable physicochemical properties and optimizing transesterification process parameters.
Analytical grade reagents and chemicals such as n-hexane (99.9% purity), anhydrous ethanol (99.9%), anhydrous methanol (99.5%), KOH (95%), anhydrous Na2SO4 (99.9%), diethyl ether (99.9%), anhydrous NaOH (98%), HCl (98%), H2SO4 (98%), anhydrous KI (98%), phenolphthalein (lab grade), starch indicator, chloroform (99%), Hanus iodine solution (analytical grade), FeCl3 (analytical grade), sodium thiosulfate (analytical grade), and distilled water were employed to perform experiments. All reagents and chemicals were from Sigma-Aldrick, UK purchased from a local vendor. Equipment used during the study includes filter screens, centrifuges, plastic bottles, moisture pans, micro-pipettes, density bottles, freeze drier, electric grinder, digital balance, digital thermometer, desiccator, rotary evaporator (RV 10, IKA, Germany), Bunsen burner, bomb calorimeter (Model 6772, Parr, Moline, IL, USA), Soxhlet apparatus, Whatman filter papers (Filtres Fioroni, France), gas chromatograph-mass spectrometer (GC–MS, Shimadzu QP-2010 Plus, Japan), watch, Erlenmeyer flasks, water bath (Wise Circu, Model WCR-P8), viscometer (Sine-Wave, Model SV-10, Australia), oven, hot plate magnetic stirrer (Model: IKA CMAG HS7), hydrometer and Pensky-Martens closed cup tester (Model SYD-261, China).
A 60 µm filter screen was employed to collect wet microalgae biomass from a wastewater stabilization pond operated by the Jimma Institute of Technology, Ethiopia. A single microalgae sp. namely Chlorella sp. which is dominated by Chlorella vulgaris was used as source of MO. C. vulgaris is unicellular microalgae commonly found in municipal wastewaters [27]. The microfilter screen whose pore size can allow the passage of the microalgae (diameter 2–10 µm) through it but retained the filamentous algae (diameters approximately 100 µm) has been used to harvest the microalgae feedstock. The microalgae which have successfully passed through the microfilter screen have been flocculated using FeCl3 to concentrate the biomass. The concentrated microalgal biomass has further been filtered, dewatered, dried, and milled to powder with an electric grinder before used to extract MO. The WCO collected from selected hotels and restaurants in Jimma town, southwest Ethiopia, was filtered through Whatman filter paper to remove larger particles and impurities (Figure. 1b). The oil was centrifugated to remove insoluble solids [17]. Anhydrous Na2SO4 was added to the WCO to lower its moisture content. Decantation was employed to separate solids settled out over 24 h.
Maximizing oil extraction from feedstock requires a combination of solvents. Nevertheless, n-hexane is the most widely used solvent due to its non-toxicity, low viscosity, low affinity for non-lipids, and better selectivity by neutral lipids [28]. The Soxhlet method was employed to extract MO from microalgal biomass. Three hundred milliliters n-hexane was added per 100 g of dry microalgae powder, and the mixture was stirred at 300 rpm for 8 h while being heated at 60 ℃. MO extraction occurred when hot n-hexane and microalgae powder in the thimble interact with each other. The n-hexane-microalgae powder mixture was siphoned back to the flask and these steps repeated until the MO was completely extracted. The extracted MO accumulated at the bottom of the flask owing to its higher boiling point than n-hexane. Upon completing the extraction, the extract was subjected centrifugation and filtration to separate solids. The MO was placed in the oven at 120 ℃ to remove water vapor and residual solvent (n-hexane). The oil was washed with distilled water. Microalgae biomass oil content was estimated using Eq 1:
Oilcontent(%)=Extractedoilweight(g)Microalgaebiomassweight(g)x100 | (1) |
The oil extracted by the Soxhlet technique can be used for direct conversion [29].
Factor optimization through conventional experimentation requires intensive labor, materials, time, and financial resources. RSM is a mathematical model used to study the effects of variables on biodiesel yield [30]. Transesterification experiments were designed using the central composite design (CCD) [31]. The CCD fits the current study in obtaining reliable results since five different levels were entertained per factor [32]. Sixteen factorial (2n) and eight axial (2n) experiments were conducted, while six experiments were conducted at the center points [33]. The distance from the axial point-to-center point (±Ɵ) was calculated using Eq 2 [34]:
±Ɵ=(2n)14 | (2) |
where Ɵ is the distance from the axial-to-center point, and n is the number of factors studied. Thus, the lowest and highest coded factors were, respectively, –2 (–Ɵ) and +2 (+Ɵ). Transesterification was carried out at catalyst loading: 0.50 ≤ A ≤ 2.5 wt/v%, methanol to oil ratio: 3:1 ≤ B ≤ 15:1 v/v, reaction temperature: 48 ≤ C ≤ 64 ℃, and reaction time: 25 ≤ D ≤ 125 min (Table 1).
Factors | Symbols | Units | Coded levels | ||||
–Ɵ | –1 | 0 | +1 | +Ɵ | |||
Uncoded levels | |||||||
Catalyst loading | A | (w/v%) | 0.50 | 1.0 | 1.50 | 2.0 | 2.5 |
Methanol to oil ratio | B | (v/v) | 3:1 | 6:1 | 9:1 | 12:1 | 15:1 |
Reaction temperature | C | (℃) | 48 | 52 | 56 | 60 | 64 |
Reaction time | D | (min) | 25 | 50 | 75 | 100 | 125 |
Transesterification reaction operating limits and the literature data were used to select the actual levels of factors [35]. The lowest reaction temperature (48 ℃) was picked based on the literature data [36], whereas the temperature near the boiling point of methanol (64 ℃) was selected as the highest reaction temperature. The lowest catalyst loading (0.50 w/v%) and the highest loading (2.5 w/v%) were picked based on previous studies [5]. The methanol to oil ratio lowest level (3:1 v/v) was selected based on the minimum transesterification reaction stoichiometry. In contrast, the highest methanol to oil ratio (15:1 v/v) was selected based on the literature data [37]. The lowest reaction time (25 min) and the highest reaction time (125 min) were picked based on data available in the literature [38]. A catalyst loading of 1.50 w/v%, methanol to oil ratio of 9:1 v/v, reaction temperature of 56 ℃, and reaction time of 75 min were used as the center points [36].
Biodiesel fuel quality depends on conversion, purification, and feedstock's FFA content [1]. Before transesterification, the oil requires characterization to know if a pre-treatment step is needed [39]. Alkaline transesterification feasibility needs measuring mixed oil's acid value and FFA content. The moisture content, saponification value, acid value, FFA content, kinematic viscosity, density, iodine value, cetane number, pour point, flash point, higher heating value, and ash content of mixed oil and biodiesel were measured and compared with EN14214 and ASTM D6751 standards. Mixed oil fatty acid composition and biodiesel methyl esters content were measured using gas chromatography-spectrometry (GC-S).
Mixed oil and biodiesel moisture contents were measured by the oven dry method. An empty moisture pan was weighed, and its mass was designated M0. A 50.0 g sample was placed in the moisture pan and weighed, and its mass was designated M1. The moisture pan with the sample was put inside the oven at 105 ℃ for 3 h. Every 30 min, the sample was taken out, cooled, and reweighed until it showed a constant weight. The moisture content was estimated using Eq 3:
Moisturecontent(%)=M1−M0M2−M0x100 | (3) |
where M0 is the empty moisture pan weight (g), M1 is the moisture pan and sample weight (g) before drying, and M2 is the sample and moisture pan weight after drying (g). The sample was poured into a conical flask to measure acid value (AV). A solvent mixture (ethanol and diethyl ether 1:1) was added to the flask containing the sample, and the mixture was stirred while being boiled in a water bath. The flask containing sample-solvent mixture and a few drops of phenolphthalein was titrated with 0.10 M KOH until a pink solution appeared. The trace KOH and by-product soap were washed off with distilled water. The sample's AV was estimated using Eq 4:
Acidvalue(%)=AxVx56.10W(g)x100 | (4) |
where A is KOH normality (mol/L), W is the sample weight (g), V is the KOH volume (mL) used for the titration, and 56.10 is the KOH molecular weight (g/mol). The sample FFA percentage is one-half of the corresponding AV. Thus, AV was halved to obtain FFA content of the sample.
MO, WCO, MO-WCO, and biodiesel specific density (Sg) were measured by taking a ratio of 50 mL sample at 20 ℃ to 50.0 mL distilled water at the same temperature. First, a clean and dry empty-density bottle was weighed, and its mass was designated M0. Then, the density bottle was filled with distilled water, and its mass was designated M1. Finally, the mass of the density bottle filled with the sample was designated M2. The sample-specific density was determined using Eq 5:
Sg=M2−M0M1−M0 | (5) |
where M0 is the empty-density bottle mass (g), M1 is the density bottle plus 50.0 mL distilled water mass (g) at 20 ℃, and M2 is the density bottle plus 50 mL sample mass at 20 ℃. On the other hand, biodiesel density at 40 ℃ was measured using a hydrometer [40].
ASTM D445-09 test method was employed to determine MO-WCO and biodiesel kinematic viscosity using the digital vibro viscometer. The sample was dropped into the viscometer cup, and the viscometer tip was inserted into the cup and kept in a water bath at 40 ℃. The average flow time the sample takes to pass between two marked points on the viscometer held vertically was used to determine kinematic viscosity Eq 6 [41]:
Kinematicviscosity(mm2s)=Kt | (6) |
where K is the calibration constant from the manufacturer (0.03772 mm2/s2 at 40 ℃) and t is the average flow time (s).
The iodine value (IV) was determined by adding 10 mL anhydrous chloroform to 0.10 g sample in an Erlenmeyer flask, then by adding 30.0 mL Hanus iodine solution. The mixture was titrated with 0.142 M Na2S2O3·5H2O solution until the solution turned light yellow. A mixture containing 2.0 mL starch solution was titrated with Na2S2O3·5H2O until the blue color disappeared. A blank titration without sample was carried out similarly. The sample IV was determined using Eq 7:
Iodinevalue(gI2100gsample)=(V0−V1)Nx12.69w(g) | (7) |
where w is the sample weight (g), N is the Na2S2O3·5H2O normality, V0 is the Na2S2O3·5H2O volume (mL) for blank titration, and V1 is the Na2S2O3·5H2O volume (mL) for sample titration.
The pour point (PP) was determined according to the ASTM D90 standard test method. A water bath at 50 ℃ was used to heat the sample in a test tube. The sample was placed in a metal cylinder with a methanol bath until its temperature reached 10 ℃. The methanol bath temperature was reduced to –17 ℃ until the sample attained –7 ℃. The sample was removed from the bath at every 3 ℃ reduction to check its ability to flow [42].
To measure saponification value (SV), sample was added to solution of KOH and ethanol and then heated to 40 ℃. After cooling, a few drops of phenolphthalein were added to the mixture. The excess KOH was titrated to the endpoint with HCl. A blank without sample was titrated similarly to determine the SV using Eq 8:
Saponificationvalue(mgKOHg)=(S0−S1)xCx56.10m(g) | (8) |
where S0 is the HCl volume (mL) to titrate the blank, S1 is the HCl volume (mL) to titrate sample, C is the HCl normality (mol/L), m is the sample weight (g), and 56.10 is the KOH molecular weight (g/mol).
Pensky-Martens closed-cup flash point tester was employed to measure MO-WCO mixture and biodiesel flash point (FP). The sample was poured into the cup until it touched a mark on its interior. After fitting the cover back, the cup was heated at 5 ℃ while being stirred. As the sample FP approached, an injector was injected into the container and lit until a flash was observed. The temperature at which a flash observed was corrected to air pressure using the correction factor to measure FP using Eq 9:
Flashpoint(corrected)=T+0.033℃/mmHgx(760mmHg−P) | (9) |
where P is the air pressure (mmHg) and T is temperature reading (℃) at the time of the experiment. The average value of triplicate observations was used in the final report.
The cetane number (CN) of mixed oil and biodiesel was determined using an empirical formula suggested by [33]. The SV and IV values were used to calculate MO-WCO and biodiesel CN values Eq 10:
Cetanenumber=46.30+(5458SV)−0.225xIV | (10) |
where IV is the iodine value and SV is the saponification value.
Oxygen bomb calorimeter was employed to measure mixed oil and biodiesel's HHV, whereas the furnace method was used to determine the ash content (AC). A cup containing sample was placed in the furnace at 500 ℃. After ignition, the residue was reweighted and AC was determined as in Eq 11:
Ashcontent(%)=W2W1x100 | (11) |
where W1 is the sample mass before ignition (g) and W2 is the sample mass after ignition (g).
GC-MS interfaced with flame ionized detector (FID) with a capillary column dimension of length x diameter x thickness (30 m, 0.25 mm, and 0.25 µm) was employed to measure the FFA and methyl ester contents of mixed oil and biodiesel respectively using helium as a carrier gas. The flask containing biodiesel and n-hexane was put on a shaker and an anhydrous Na2SO4 was added, and stood idle until settle out. The column temperature was programmed to increase from 50 to 250 ℃ at 4 ℃/min, whereas detector and injector temperatures were fixed at 230 ℃ and 250 ℃, respectively [43]. One microliter sample at a 1:100 split ratio and standard fatty acids were injected into GC-S. The fatty acid and methyl ester contents were obtained from the peak areas [44].
Five different MO-WCO blend ratios were prepared. The selection was based on physicochemical properties like kinematic viscosity, density, HHV, and AV. To reduce the MO-WCO mixture AV, acid-catalyzed esterification was conducted in a three-necked round bottom flask. The esterified oil was transferred to a separating funnel and subjected to six hours long gravitational sedimentation. The rotary evaporator removed unreacted methanol [45]. The transesterification reaction was conducted in a 0.50 L three-necked flask fitted with a reflux condenser, hot magnetic stirrer, sample outlet, and thermostat. Freshly prepared KOH-methanol solution was added to the esterified oil in the pre-heated flask submerged in a water bath. Transesterification experiments were conducted at CCD-designed actual levels for the four factors at constant mixing speed (600 rpm). At the end of the transesterification experiments, the mixture was subjected to 12 h settling to separate it into glycerol and biodiesel layers.
The glycerol layer was drained off and discarded after being neutralized with an acid, whereas the biodiesel layer was distilled under a vacuum rotary evaporator to recover unreacted methanol [42]. The biodiesel was washed with distilled water at 70 ℃ until the wash water achieved a neutral pH (Figure 2). The biodiesel was dried under the rotary evaporator at 80 ℃ and passed over anhydrous Na2SO4 to remove water remained after washing. The biodiesel yield was determined using Eq 12:
Biodieselyield(%)=MbMox100 | (12) |
where Mb is the biodiesel mass obtained (g) and M0 is the oil mass used (g).
The overall experimental procedure is summarized in Figure 3.
Transesterification results were fitted to the model equation to relate biodiesel yield and the factors [5]. The contribution and significance of each factor and biodiesel yield as a function of coded factors were determined using Eq 13 [30]:
Y=β0+4∑i=1βiXi+4∑i=1βiiXi2+4∑i=14∑j=i+1βijXij | (13) |
where Y is the predicted biodiesel yield (%), βo is the intercept, βi is the first-order coefficient, βii is the quadratic coefficient of i the factor, βij is the linear interaction coefficient between the i and j factors, and Xi and Xj are the coded factors. The coefficient of determination (R2), predicted R2, adjusted R2, p-value, and lack of fit (LOF) were used to evaluate model significance and adequacy [43]. ANOVA and significance tests were used to assess the quality of model fitness. The p and F values were used to evaluate the parameters' statistical significance. Response surface curves were generated using the fitted quadratic equation to study variables interactive effects on biodiesel yield [33]. The relative influence of each parameter on biodiesel yield was investigated using sensitivity analysis. Validation experiments were conducted at the optimum conditions to confirm the predicted yield.
A 2.04 kg dry microalgae biomass was used to extract 733.72 g of MO. The oil content of microalgae biomass was found to be 35.97%. The percentage of oil content obtained agrees with the MO content (30–70%) available in the literature [9]. The optimization process was performed with the oil mixture having the most suitable physicochemical properties. In this case, MO70WCO30 was the most suitable oil blend as it gives the best trade-off in kinematic viscosity, density, HHV, and AV compared to the other blends. MO70WCO30 possessed the lowest kinematic viscosity at 40 ℃, density at 20 ℃, and AV compared to the other blends (Table 2).
Property | Individual oils | Oil mixtures | |||||
MO | WCO | MO50:WCO50 | MO60:WCO40 | MO70:WCO30 | MO80:WCO20 | MO90:WCO10 | |
Density at 20 ℃ (Kg/m3) | 902.1 | 902.51 | 903.15 | 902.50 | 901.01 | 902.21 | 902.67 |
Viscosity (mm2/s) | 42.40 | 45.10 | 50.19 | 51.02 | 41.47 | 45.91 | 44.82 |
AV (mg KOH/100g) | 16.46 | 21.21 | 18.11 | 15.01 | 13.02 | 14.52 | 14.58 |
HHV (MJ/Kg) | 40.81 | 39.63 | 38.90 | 39.95 | 40.23 | 37.99 | 38.99 |
Biodiesel properties mainly depend on the feedstock's fatty acid composition [46]. The WCO mainly consisted of oleic acid, palmitic acid, linoleic acid, palmitoleic acid, stearic acid, and small amounts of myristic acid, eicosenic acid, linolenic acid, arachidic acid, erucic acid, behenic acid, and tetracoanic acid. About 25.75% of the fatty acid profile comes from saturated fatty acids, whereas the monounsaturated fatty acids (MUSFAs) account for 57.64% while polysaturated fatty acids account for 14.28% of fatty acid profiles. About 84.39% of WCO fatty acid compositions originated from saturated and monounsaturated fatty acids (Table 3). High palmitic acid and oleic acid profile indicate a biodiesel with better quality can be synthesized from WCO. The use of microalgae oil as feedstock for biodiesel production depends on its fatty acid profiles. MO contains saturated and unsaturated fatty acids [47]. The MO from Chlorella vulgaris contains palmitic acid (22.70%), stearic acid (21.50%), oleic acid (7.81%), linoleic acid (6.90%), palmitoleic acid (11.30%), myristic acid (7.10%), eicosapentaenoic acid (6.10%), and linolenic acid (16.11%) [9]. The saturated and polyunsaturated fatty acids profiles in the lipids of microalgae are 19.11% and 29.11% respectively. The MUFAs account for 51.30% of MO lipids. Microalgae with a high content of SFAs and MUFAs in lipids are the most promising feedstock for the biodiesel production [48]. Both MO and WCO are dominated by saturated and monounsaturated fatty acids. Since fuel quality depends on the proportion of saturated and monounsaturated fatty acid profiles, the better fuel property of biodiesel synthesized from mixed MO-WCO might be attributed to this fact. Higher monounsaturated fatty acids (MUFAs) proportions in both oils give biodiesel liquidity in cold climates. The significant proportion of saturated fatty acid (SFA) in MO and WCO renders higher oxidation stability to biodiesel made from the mixed MO-WCO.
WCO | Fatty acid type and number of carbon atom in the chain | |||||||||||||||||
Palmitic (C16:0) | Stearic (C18:0) | Oleic (C18:1) | Linoleic (C18:2) | Linolenic (C18:3) | Myristic (C14:0) | Erucic (C22:1) | Palmitoleic (C16:1) | Eicosenic (C20:1) | Tetracoanic (C24:0) | Arachidic (C20:0) | Behenic (C22:0) | |||||||
(Wt%) | 20.42 | 4.18 | 52.09 | 13.45 | 0.83 | 0.95 | 0.07 | 4.61 | 0.87 | 0.03 | 0.13 | 0.04 | ||||||
Microalgae (C. vulgaris) | Fatty acid type and number of carbon atom in the chain | |||||||||||||||||
Myristic (C14:0) | Palmitic (C16:0) | Stearic (C18:0) | Palmitoleic (C16:1) | Oleic (C18:1) | Linoleic (C18:2) | Linolenic (C18:3) | Eicosapentaenoic (C20:5) | |||||||||||
(Wt%) | 7.10 | 22.70 | 21.50 | 11.30 | 7.81 | 6.90 | 16.11 | 6.10 |
In this study, biodiesel fuel properties such as kinematic viscosity at 40 ℃, density at 20 ℃, flash point (FP), HHV, IV, AV, AC, fatty acid methyl ester content, and CN were studied and compared with the ASTM D6751 and EN14214 standards. A biodiesel could be used in an existing engine if its fuel properties conform to these standards [49]. The results revealed that most of the physicochemical properties of biodiesel from MO-WCO satisfied the standards and could be a prospective candidate to the petrodiesel. The MO-WCO mixture AV was found to be 13.02 mg KOH/g, while its corresponding FFA was 6.51 mg KOH/g. This high acid value of MO-WCO might be due to the high free fatty acid content [49]. The amount of lubrication in the fuel channel can be indicated from the acid value. The AV reduction was achieved by esterifying the mixed oil with H2SO4 prior to transesterification. AV is the KOH (mg) required to neutralize 1 g of biodiesel [39]. Biodiesel has a higher AV than petrodiesel (0.017 mg KOH/g) [5]. Biodiesel AV was found to be 0.049 mg KOH/g. Biodiesel with higher AV corrodes fuel supply system of internal engine [50]. Biodiesel AV satisfies both ASTM D6751 and EN14214 standards.
Density at 20 ℃ of biodiesel synthesized from the mixed oil was found to be 890.0 kg/m3 which meets both ASTM D6751 and EN14214 standards of 860–900 kg/m3. Biodiesels have more density as compared to petrodiesel [51]. The degree of unsaturation influences biodiesel density [49]. Biodiesel density indicates the energy content, the delay time between injection and combustion, and air-fuel ratio within the combustion chamber [40]. The biodiesel density indicates the sufficient volume of fuel is delivered by injection systems for exact combustion. The energy amount and air to fuel ratio in the combustion chamber depend on the fuel density due to the fact that high density fuel contains more mass as compared to low density fuel [49]. Biodiesel density depends on several factors including the type of conversion, methyl ester profile, and feedstock type [50]. Biodiesel density plays a very crucial role in designing injector nozzle as it directly influences engine working and fuel atomization which finally influences engine thermal efficiency [1]. Biodiesel density limits its use for wide applications [1].
Cetane number (CN) measures the ignition quality of biodiesel [40]. It directly affects the fuel ignition delay (time gap between fuel discharge into combustion chamber and start of ignition) [50]. The CN of the biodiesel from MO-WCO blend showed a value of 77.76, which agrees with ASTM D6751 and EN 14214 standards of ≥47 and ≥51 respectively. The higher biodiesel CN value signifies a shorter delay time between fuel injection and initiation of ignition, combustion efficiency, and lesser pollutants emission [49]. A low CN value is associated with higher emissions from engine exhaust due to incomplete combustion and increased knocking [50]. The higher biodiesel CN value of biodiesel from MO-WCO mixture might be due to higher content of saturated fatty acids in mixed oil. The biodiesel CN increases with degree of saturation and fatty acids chain length [50]. A higher biodiesel CN value signifies better engine performance and lower pollutant emissions.
The saponification value (SV) is the base catalyst needed to saponify 1 g of oil [52]. Also, SV of biodiesel fuel directly measures its average molecular weight [53]. In this study, SV of mixed oil was found to be 180.92, which falls within the ASTM D6751 standard. Mixed oil's higher SV might be due to the higher amount of low molecular weight fatty acid chain [49]. A higher SV of raw oil shows the existence of high fatty acid proportion [49]. An alkali-catalyzed transesterification of oils with higher SV lowers biodiesel yield due to soap formation [26]. Oil with lower SV is desirable to obtain a better biodiesel yield [1]. According to ASTM D6751 standard, the saponification value varies from 0–370 mg KOH/g [50].
The iodine value (IV) is the amount of iodine (g) consumed by 100 g of fuel [49]. IV measures the degree of unsaturation of the biodiesel fuel [50]. Biodiesel IV of 12.90 g I2/100 g falls within the ASTM D6751 standard. This IV of biodiesel indicates less engine deposits formation. IV strongly influences the oxidation stability and polymerization of glycerides. It leads the formation of deposits in diesel engine injectors [53]. The iodine value (IV) is closely correlated to biodiesel viscosity, CN and cold flow characteristics [50].
Biodiesel kinematic viscosity at 40 ℃ was found to be 4.50 mm2/s, which falls within the ASTM D6751 (1.90–6.0 mm2/s) and EN 14214 (3.50–5.0 mm2/s) standards. The higher kinematic viscosity of MO-WCO mixture has been decreased significantly after the transesterification process. Since the WCO is dominated by oleic acid which results in lower viscosity, mixing of WCO with MO might have resulted in the biodiesel with lower viscosity. The lower kinematic viscosity (4.50 mm2/s) of the biodiesel might be related to its fatty acid methyl ester profile. It can be concluded that the biodiesel from MO-WCO will lead to less operational problems like engine deposits and low temperature engine start. A higher kinematic viscosity is detrimental since it reduces fuel fluidity, injection, and air-fuel ratio in the combustion chamber [52]. Moreover, a high viscosity may cause the formation of soot and engine deposits due to insufficient atomization [1]. On the other hand, lower viscosity leads to easy fuel pump and achieve final droplets to injector. Large molecular mass and chemical structure gives biodiesel a viscosity of 10–15 times higher than that of petrodiesel [1].
Flash point (FP) is the lowest temperature at which vapors of fuel catch fire when come in contact with flame [50]. The biodiesel FP in the current study falls within the EN 14214 and ASTM D6751 standards. The biodiesels have higher FP than petrodiesel fuel (≈55–65 ℃). The FP value of the biodiesel from MO-WCO blend will not induce fire risk during transport, storage, and usage. The biodiesel produced from MO-WCO mixture meets the specification given in ASTM 6751 and EN 14214 standards. The major reason why the FP specification required is to make sure that the biodiesel has been sufficiently purified by removing extremely volatile component such as residual methanol [50]. Biodiesel volatility is inversely related to FP. The biodiesel made from MO-WCO mixture has a higher FP since both MO and WCO have more heavy fatty acid profiles (C > 12).
Pour point (PP) is the lowest temperature at which a liquid fuel loses its flow properties [50]. The PP value of biodiesel fuel is determined using the ASTM D97 methodology. The PP value of biodiesel synthesized from MO-WCO mixture was found to be –2.5 ℃, indicating the biodiesel could be used in cold weather. PP is an important fuel property as it may cause fuel lines filter blockage and engine damage. Biodiesels usually have more PP than diesel fuel. The PP of biodiesel is directly proportional to unsaturation level of fatty acids [49]. A fuel flows properly just above its PP value [50].
The calorific value or heating value of a fuel denotes the amount of heat energy released by the combustion of a unit mass of a fuel [54]. The heating or caloric value of biodiesel from the current study was found to be 43.25 MJ/kg, which satisfies both ASTM D6751 and EN14214 standards. A larger HV indicates a better energy content of the biodiesel. The larger heating value (HV) and lowekinematic viscosity at 40 ℃ of the biodiesel might be due to its methyl ester profile. Also, increasing fatty acid carbon chain length without changing saturation level might have contributed to higher heating value of biodiesel. The heating value of biodiesel is 10–11% lower than petrodiesel due to its higher oxygen content [54].The methyl esters make up 99.44% of the biodiesel which meets both EN 14214 and ASTM D6751 standards. The mixed oil properties and biodiesel fuel quality were compared to ASTM D6751 and EN 14214 standards (Table 4). Generally, it can be concluded that all tested biodiesel properties conform to and fulfill the ASTM D6751and EN 14214 standards. Table 5 shows comparison of properties of biodiesel produced from various mixed oils with the current study. Most of the properties of biodiesels from various mixed oils full fill conditions set in EN 14214 and ASTM D6751 the standards.
Physicochemical properties | Unit | Determined value | Standards [9] | ||
MO70WCO30 | Biodiesel | ASTM D6751 | EN 14214 | ||
Relative density at 20 ℃ | - | 0.901 | 0.890 | 0.86–0.90 | 0.86–0.90 |
Density at 20 ℃ | [kg/m3] | 901.0 | 890.0 | 860–900 | 860–900 |
Kinematic viscosity at 40 ℃ | [mm2/s] | 41.47 | 4.50 | 1.9–6.0 | 3.5–5.0 |
Flash point | [℃] | 168.33 | 180.0 | ≥130 | ≥120 |
Cetane number | - | 46.83 | 77.76 | ≥47 | ≥51 |
Acid value | [mg KOH/g] | 13.02 | 0.049 | ≤0.05 | ≤0.05 |
Free fatty acid content | [%] | 6.51 | 0.445 | ≤0.05 | ≤0.05 |
Saponification value | [mg KOH/g] | 180.92 | 146.1 | 0–370 | - |
Iodine value | [g I2/100 g] | 118.40 | 12.90 | ≤120 | ≤140 |
Water content | [%] | 0.033 | 0.020 | ≤0.03 | ≤0.05 |
Ash content | [%] | 0.061 | 0.003 | ≤0.03 | ≤0.02 |
Pour point | [℃] | –5.0 | –2.50 | –5 to 10 | –5 to 10 |
Heating value | [MJ/kg] | 40.23 | 43.25 | ≥42.0 | ≥35.0 |
Methyl ester content | [%] | - | 99.44 | ≥96.50 | ≥96.50 |
Biodiesel feedstock | Properties | Ref. | ||||||||
Density (kg/m3) | Kinematic viscosity at 40 ℃ (mm2/s) | CN (-) | PP (℃) | FP (℃) | AV (mg KOH/g) | HV (MJ/kg) | SV (mg KOH/g of oil) | IV (gI2/100 g) | ||
Jatropha curcas and Ceiba pentandra oils | 831.2 | 3.95 | 58.0 | 0.50 | 196 | 0.025 | 40.929 | - | - | [5] |
Castor seed and waste fish oils | 898.9 | 3.61 | 57.0 | –2.0 | 84.0 | 0.06 | - | 175.24 | 91.0 | [55] |
Neem and pig fat oils | 825.0 | 2.20 | 64.6 | 3.0 | 120 | 0.42 | - | 188.60 | 47.2 | [56] |
Jatropha and algae oils | - | 4.1 | - | - | 115 | - | - | - | 83.2 | [57] |
WCO and Calophyllum inophyllum oil | 861.6 | 4.72 | - | 2.0 | 160.5 | 0.46 | 41.35 | - | - | [18] |
Castor and karanja oils | 944.7 | 7.83 | - | 12 | 160 | 0.48 | 38.63 | - | - | [58] |
Castor seed and microalgae oils | 890.0 | 5.80 | 58.1 | - | 133.3 | 0.10 | 48.12 | 1.80 | 82.22 | [9] |
Palm and sesame oils | 880.0 | 4.42 | 53.4 | 3.80 | > 150 | 0.37 | 41.24 | - | - | [59] |
Carica papaya, Citrus sinensis, and Hibiscus sabdariffa seed oils | 872.0 | 1.82 | 64.4 | –12 | 130 | 0.36 | - | 178.24 | 52.62 | [60] |
Mahua and simarouba oils | 856.0 | 4.77 | - | 3.60 | 134 | 0.445 | 37.02 | - | - | [61] |
Waste cooking oil and Schleichera oleosa oil | 857.0 | 4.459 | - | 9.0 | 172 | 0.28 | 39.95 | - | - | [54] |
Microalgae oil and WCO | 890.0 | 4.50 | 77.8 | –2.5 | 180.0 | 0.049 | 43.25 | 146.1 | 12.90 | This study |
The RSM model was used to optimize MO-WCO transesterification process. The four independent variables were varied over five levels to optimize the biodiesel yield. Thirty experimental runs designed with CCD were performed to investigate variables effects on biodiesel yield (Table 5). A regression analysis was performed to generate a quadratic model equation in order to calculate biodiesel yield (Y) as a function of the coded factors. The equation consists of a center point (β0), four linear terms (A, B, C, and D), six interacting terms (AB, AC, AD, BC, BD, and CD), and four quadratic terms (A2, B2, C2, and D2). The final equation containing only significant parameters was used to estimate biodiesel yield (Eq 14):
Y=71.93+5.06A+5.49B+12.56C+12.61D+3.92AC+1.56BC+5.24CD−6.64A2−7.15B2−2.70C2−3.42D2 | (14) |
Factors with positive coefficients (A, B, C, D, AC, BC, and CD) had positive effects on the biodiesel yield, whereas those with negative coefficients (the four quadratic terms) showed antagonistic effects. The magnitudes of regression coefficients were used to infer variables' degree of significance. In this case, reaction time and temperature were found to be the most significant parameters. Thus, the RSM model has been successfully employed to establish the relationship between biodiesel yield and the dependent factors and their degree of significance [62]. The point of prediction methodology was used to predict the optimum level of variables (i.e., catalyst loading of 2.0 w/v%, molar ratio of 12:1, temperature of 60 ℃, and reaction time of 100 min) at which maximum biodiesel yield of 98.8% has been achieved (Table 6).
S.No. | Factors actual levels | Factors coded levels | Biodiesel yield (%) | ||||||||
Catalyst loading (w/v%) | Molar ratio (v/v) | Temperature (℃) | Time (min) | ||||||||
A | B | C | D | Actual | Predicted | Residual | |||||
1 | 2.0 | 6:1 | 52.0 | 50.0 | 1 | –1 | –1 | –1 | 29.05 | 29.60 | –0.55 |
2 | 2.0 | 12:1 | 60.0 | 50.0 | 1 | 1 | 1 | –1 | 64.44 | 62.94 | 1.50 |
3 | 1.0 | 12:1 | 52.0 | 100.0 | –1 | 1 | –1 | 1 | 51.02 | 49.93 | 1.09 |
4 | 2.0 | 12:1 | 52.0 | 50.0 | 1 | 1 | –1 | –1 | 37.62 | 37.34 | 0.28 |
5 | 1.0 | 6:1 | 60.0 | 100.0 | –1 | –1 | 1 | 1 | 67.68 | 66.59 | 1.09 |
6 | 2.0 | 12:1 | 60.0 | 100.0 | 1 | 1 | 1 | 1 | 98.80 | 99.10 | –0.30 |
7 | 2.0 | 6:1 | 52.0 | 100.0 | 1 | –1 | –1 | 1 | 42.88 | 42.93 | –0.52 |
8 | 2.0 | 12:1 | 52.0 | 100.0 | 1 | 1 | –1 | 1 | 53.24 | 52.53 | 0.71 |
9 | 1.0 | 12:1 | 60.0 | 100.0 | –1 | 1 | 1 | 1 | 81.24 | 80.82 | 0.42 |
10 | 1.0 | 6:1 | 52.0 | 50.0 | –1 | –1 | –1 | –1 | 29.32 | 27.66 | 1.66 |
11 | 1.0 | 6:1 | 52.0 | 100.0 | –1 | –1 | –1 | 1 | 40.32 | 41.95 | –1.63 |
12 | 1.0 | 12:1 | 52.0 | 50.0 | –1 | 1 | –1 | –1 | 33.68 | 33.78 | –0.10 |
13 | 1.0 | 6:1 | 60.0 | 50.0 | –1 | –1 | 1 | –1 | 30.50 | 31.34 | –0.84 |
14 | 2.0 | 6:1 | 60.0 | 50.0 | 1 | –1 | 1 | –1 | 49.24 | 48.96 | 0.28 |
15 | 2.0 | 6:1 | 60.0 | 100.0 | 1 | –1 | 1 | 1 | 83.22 | 83.25 | –0.03 |
16 | 1.0 | 12:1 | 60.0 | 50.0 | –1 | 1 | 1 | –1 | 45.12 | 43.70 | 1.42 |
17 | 1.50 | 15:1 | 56.0 | 75.0 | 0 | 2 | 0 | 0 | 52.42 | 54.31 | –1.89 |
18 | 2.50 | 9:1 | 56.0 | 75.0 | 2 | 0 | 0 | 0 | 55.20 | 55.49 | –0.29 |
19 | 1.50 | 9:1 | 56.0 | 125.0 | 0 | 0 | 0 | 2 | 83.44 | 83.47 | –0.03 |
20 | 1.50 | 9:1 | 48.0 | 75.0 | 0 | 0 | –2 | 0 | 35.94 | 36.03 | –0.09 |
21 | 0.50 | 9:1 | 56.0 | 75.0 | –2 | 0 | 0 | 0 | 34.32 | 35.26 | –0.94 |
22 | 1.50 | 9:1 | 64.0 | 75.0 | 0 | 0 | 2 | 0 | 85.12 | 86.27 | –1.15 |
23 | 1.50 | 9:1 | 56.0 | 25.0 | 0 | 0 | 0 | –2 | 31.83 | 33.03 | –1.20 |
24 | 1.50 | 3:1 | 56.0 | 75.0 | 0 | –2 | 0 | 0 | 33.00 | 32.35 | 0.65 |
25 | 1.50 | 9:1 | 56.0 | 75.0 | 0 | 0 | 0 | 0 | 71.30 | 71.93 | –0.63 |
26 | 1.50 | 9:1 | 56.0 | 75.0 | 0 | 0 | 0 | 0 | 70.50 | 71.93 | –1.43 |
27 | 1.50 | 9:1 | 56.0 | 75.0 | 0 | 0 | 0 | 0 | 70.50 | 71.93 | –1.43 |
28 | 1.50 | 9:1 | 56.0 | 75.0 | 0 | 0 | 0 | 0 | 70.50 | 71.93 | –1.43 |
29 | 1.50 | 9:1 | 56.0 | 75.0 | 0 | 0 | 0 | 0 | 74.40 | 71.93 | 2.47 |
30 | 1.50 | 9:1 | 56.0 | 75.0 | 0 | 0 | 0 | 0 | 74.40 | 71.93 | 2.47 |
The optimization results of MO-WCO transesterification results have been compared with the previously published studies. The present study shows that the maximum biodiesel yield from MO70WCO30 is 97.72% which is better than most of the transesterification results of mixed oils (Table 7). The result of current study showed that the highest biodiesel yield from was achieved in 100 min reaction time which is much lower than those reported for Jatropha curcas-Ceiba pentandra (180 min with 93.33% yield), castor & karanja oils (1440 min, 78% yield), and C. inophyllum & WCO oils (129 min, 96.5% yield). Nevertheless, the optimal yield of biodiesel among various mixed oils could be attributed to many factors such as the acid values of mixed oils, the reactivity of catalyst used, and mode of heating used during the transesterification process [63]. The optimization result of MO-WCO indicates that this feedstock can be used as cost-effective, sustainable, and environmentally friendly fuel source.
Mixed feedstock | Optimum parameters | Biodiesel yield (%) | References | |||
Catalyst loading (Wt%) | Alcohol to oil ratio | Time (min) | Temperature (℃) | |||
Castor & karanja oils | 10.0 | 6:1 | 1440 | 50 | 78.0 | [58] |
Castor seed oil & microalgae oil | 1.23 | 6:1 | - | 51 | 92.36 | [9] |
C. inophyllum & WCO oils | 0.50 | 60% | 90 | 55 | 96.84 | [64] |
J. curcas & C. pentandra oils | 0.50 | - | 180 | 60 | 93.33 | [6] |
Mahua & simarouba oils | 3.50 | 5:1 | 30 | 60 | 98.0 | [65] |
Castor bean & WCO oils | 0.75 | 8:1 | 30 | 60 | 97.20 | [53] |
Sterculia foetida & rice bran oils | 0.70 | 42% | 60 | 51 | 98.93 | [45] |
J. curcas, castor seed & WCO oils | 7.0 | 12:1 | 59 | 59 | 92.35 | [62] |
MO & WCO oils | 2.0 | 12:1 | 100 | 60 | 97.72 | This study |
The ANOVA was employed to verify significance of individual and interacting variables on biodiesel yield and model fitness. Model significance in predicting biodiesel yield was evaluated with the help of a p-value (p < 0.05 indicates significance) [66]. The F value of 319.25, p-value < 0.0001, and high value of the sum of squares (12057.84) of the model reveal that the factors studied were statistically significant at 95% confidence limit. Statistical analysis of variables shows that A (catalyst loading linear term, p < 0.0001), B (molar ratio linear term, p < 0.0001), C (reaction temperature linear term, p < 0.0001), D (reaction time linear term, p < 0.0001), AC (catalyst loading and reaction temperature interaction term, p < 0.0001), BC (molar ratio and reaction temperature interaction term, p = 0.0017), CD (reaction temperature and time interaction term, p < 0.0001), A2 (catalyst loading quadratic term, p < 0.0001), B2 (molar ratio quadratic term, p < 0.0001), C2 (reaction temperature quadratic term, P < 0.0001), and D2 (reaction time quadratic term, p < 0.0001) were found to be the significant model parameters. Nevertheless, AB (catalyst loading and molar ratio interaction term, p = 0.3404), AD (catalyst loading and reaction time interaction term, p = 0.5666), and BD (molar ratio and reaction time interaction term, p = 0.2746) were not the significant model terms. It can be concluded that the linear model parameters are more significant than the quadratic and interactives model terms (Table 8). Model p-value of < 0.0001 further reveals that the probability of obtaining a large F value due to noise is < 0.01%. The LoF's F value of 0.5801 indicates the model adequately describes the variations in response variable. The lack of fit insignificance (p = 0.7833) reveals that the model fits the experimental data. Additionally, it indicates a sufficient relationship exists between independent variables and the dependent variables (biodiesel yield).
Source | The sum of squares (SSs) | DF | Mean Square | F-value | P-value | Status |
Model | 12057.84 | 14 | 861.27 | 319.25 | < 0.0001 | Significant |
A | 613.78 | 1 | 613.78 | 227.51 | < 0.0001 | |
B | 723.69 | 1 | 723.69 | 268.25 | < 0.0001 | |
C | 3786.84 | 1 | 3786.84 | 1403.66 | < 0.0001 | |
D | 3816.54 | 1 | 3816.54 | 1414.67 | < 0.0001 | |
AB | 2.62 | 1 | 2.62 | 0.9698 | 0.3404 | |
AC | 245.78 | 1 | 245.78 | 91.10 | < 0.0001 | |
AD | 0.9264 | 1 | 0.9264 | 0.3434 | 0.5666 | |
BC | 38.97 | 1 | 38.97 | 14.44 | 0.0017 | |
BD | 3.47 | 1 | 3.47 | 1.29 | 0.2746 | |
CD | 439.43 | 1 | 439.43 | 162.88 | < 0.0001 | |
A² | 1208.90 | 1 | 1208.90 | 448.10 | < 0.0001 | |
B² | 1402.75 | 1 | 1402.75 | 519.95 | < 0.0001 | |
C² | 199.41 | 1 | 199.41 | 73.92 | < 0.0001 | |
D² | 320.84 | 1 | 320.84 | 118.92 | < 0.0001 | |
Residual | 40.47 | 15 | 2.70 | - | - | |
LoF | 21.73 | 10 | 2.17 | 0.5801 | 0.7833 | Not significant |
Pure error | 18.73 | 5 | 3.75 | - | - | |
Cor total | 12098.31 | 29 | - | - | - |
Model fitness to the experimental data was evaluated using the coefficient of determination (R2). The R2 shows variability of independent variables with dependent variable. A higher R2 value indicates a good fit between model predicted and experimental data [33]. The R2 value for this study (R2 = 0.9967) shows that 99.67% of the experimental results are compatible with model predicted data (i.e., only 0.33% of total variability cannot be explained by the model). The higher R2 adjusted value (R2 adj. = 0.9935) indicates 99.35% variability of the biodiesel yield. The adjusted coefficient of determination (R2 adj.) shows how the model fits well with the experimental data [3]. R2 adj. increases as desirable variables become part of the model but decreases upon inclusion of undesirable variables [5]. Model fitness to the experimental data was inferred from the higher R2 predicted value (R2 pred = 0.9874). The difference between R2 pred. and R2 adj. of 0.61% signifies that the insignificant model terms have been eliminated [9].
The Design Expert® software Version 13.5.0 (Stat-Ease, Inc., USA) was employed to select the model algorithm that fits best for variables optimization and the biodiesel yield maximization. The R2, R2 adj., R2 pred., SD, and PRESS were employed to select the model that fits better [62]. The model fit statistical data (Table 9) indicate that the quadratic model fits better as it shows the highest R2, R2 adj., and R2 pred. values and the smallest standard deviation (SD) and PRESS values compared to the non-aliased models (i.e., linear and 2FI).
Model | SD | R² | R² adjusted | R² predicted | PRESS | Status |
Linear | 11.24 | 0.7390 | 0.6973 | 0.6549 | 4175.10 | Not aliased |
2FI | 11.30 | 0.7995 | 0.6939 | 0.6744 | 3939.57 | Not aliased |
Quadratic | 1.64 | 0.9967 | 0.9935 | 0.9874 | 152.16 | Suggested |
Cubic | 1.91 | 0.9979 | 0.9912 | 0.9165 | 1009.68 | Aliased |
A CV value < 10% signifies the model is more accurate and reliable and the experimental results are reproducible. Thus, CV value of 2.93% for the current study shows a higher precision and good reliability of the experimental results. The R2 approaching unity and the smaller SD value of the quadratic model also indicate model suitability to predict the biodiesel yield. The difference between R2 adj. and R2 pred. < 20% indicates a good agreement between experimental and model predicted data [62]. An adequate precision was used to measure the signal-to-noise ratio. The adeq. Prec. > 4.0 is usually desirable. The adequate precision for this study was found to be 61.51, which indicates the signal is adequate and quadratic model is precise to navigate variables effect on biodiesel yield. The R2 pred. value of 0.9874 confirms that the quadratic model is adequate to predict biodiesel yield. In conclusion, all the statistical parameters reveal that the quadratic regression model equation is highly accurate and reliable for predicting biodiesel yield (Table 10).
Factor | Value |
SD | 1.64 |
Mean | 56.01 |
CV | 2.93 |
R² | 0.9967 |
R² adj. | 0.9935 |
R² pred. | 0.9874 |
Adeq. Prec. | 61.5104 |
Plotting the predicted biodiesel yield against actual yield confirmed that the predicted data closely fit the experimental data. A minimal data points divergence from the diagonal line also indicates a good fit between the predicted and experimental data [66]. The differences between the experimental and predicted values < 0.20 indicate that there is a high-quality agreement between the model predicted and experimental biodiesel yields (Figure 4). The finding agrees with the R2 and adjusted R2 values very close to 1.0 [5]. It can be concluded that the regression model gives a good estimate of the biodiesel yield with variations in the studied independent variables.
Residuals follow a normal distribution provided that the experimental errors are random. The residuals normal distribution indicates adequacy of the quadratic regression model. In order to obtain studentized residuals, the residuals were normalized and divided by their estimated standard deviations. The studentized residuals then plotted against the studentized residuals obtained from experimental data in order to predict the best-fit normal distribution. It can be observed that the studentized residuals follow a normal distribution, as can be seen from the data points close to the straight-line (Figure 5). The residuals normal distribution plot reveals that the quadratic regression model is reliable, adequate, and better estimates biodiesel yield with variations in the studied variables.
Input variables' effects on biodiesel yield were found using regression coefficients. The effect of each variable and the interaction between the variables on biodiesel yield was investigated using sensitivity analysis (Si). Equation 15 uses the sum of squares of the model and individual parameters to obtain parameters sensitivity analysis [45]:
Si(%)=(βi2∑βi2)x100 | (15) |
where βi2> is the sum of squares of each parameter and ∑βi2> is the total sum of squares of all the variables.
The sensitivity analysis revealed that the most significant model parameter was reaction time, followed by reaction temperature, methanol to oil ratio quadratic term, and catalyst loading quadratic term (Figure 6).
The interaction effects between variables on biodiesel yield were studied using three-dimensional (3D) response surface curves. The interaction effects between variable were performed by holding two of the variables at center points [17]. The positive coefficient of the interacting variables signifies that biodiesel yield increases with increase in both variables until the optimum yield reached after which further increase lowered the yield. Figure 7 shows the interaction effect between catalyst loading and reaction temperature on biodiesel yield at reaction time of 100 min and methanol: oil ratio of 9:1. At the lowest catalyst loading (0.50 w/v%) and reaction temperature of 48 ℃, a 49.1% biodiesel yield was obtained. A 63.9% biodiesel yield was achieved as catalyst loading increased from 0.50 to 2.0 w/v% while the reaction temperature was kept at 48 ℃. However, further increase in catalyst loading towards upper level reduced the yield. At the optimum catalyst loading (2.0% w/v) and reaction temperature (60 ℃), greater than 80% biodiesel yield was achieved.
Analysis of the interaction effects between methanol to oil ratio and temperature on biodiesel yield at a catalyst loading of 1.5 w/v% and reaction time of 100 min revealed that the interaction of the two variables showed a significant positive effect on the biodiesel yield (Figure 8). At a lower reaction temperature, an increase in methanol to oil ratio from 3:1 to 12:1 increased the yield from 45.88% to 57.10%. An increase in biodiesel yield was observed at all levels of methanol to oil ratio as the reaction temperature rises from 48 ℃ to 60 ℃. At the optimum methanol: oil ratio of 12:1 and reaction temperature of 60 ℃, approximately 80% biodiesel yield was achieved. This might be attributed to the shifting of reaction equilibrium towards the product side at a higher level of methanol concentration. Increase in both reaction temperature and methanol: oil ratio increased the biodiesel yield.
Figure 9 shows the response surface curve for the interaction effects between reaction temperature and reaction time on biodiesel yield at catalyst loading of 1.50 w/v% and methanol to oil ratio of 9:1. The positive interaction between the two parameters revealed that the yield increases with increase in both variables while catalyst loading and methanol with oil ratio being kept at the center points. At the temperature of 48 ℃ and time of 25 min, a nearly 46.10% yield was achieved. A greater than 95% yield was achieved with increase in reaction time further while temperature being kept at 60 ℃. An increase in biodiesel yield with temperature might be attributed to the fact that increasing reaction temperature lowers oil viscosity which promotes its mixing with alcohol-catalyst to give a better yield. Temperature and reaction time interaction showed more impact on biodiesel yield compared to other interacting variables.
The point prediction tool in Design Expert® 13.0.5.0 software was employed to solve the quadratic equation (Eq 14) to find the optimum value for the transesterification variables which give the maximum biodiesel yield. All factors were set to the goal of "in range", whereas biodiesel yield was set to the goal of "maximize". After setting the lower and upper limit, lower and upper weights, and importance, the program was run to give the top 100 solutions of the optimum values of the four variables and biodiesel yield. To validate the model predicted optimum biodiesel yield, triplicate confirmatory experiments were performed under optimum transesterification variables (catalyst loading of 2 w/v%; methanol to oil ratio of 12:1 v/v; reaction temperature of 60 ℃; and reaction time of 100 min). An actual biodiesel yield of 97.72% was obtained from the validation experiments. The result (mean ± SD; n = 3) revealed that the biodiesel yield from the validation experiments is in a good agreement with the model predicted result (99.10%). The difference between the predicted and experimental value revealed that the developed quadratic model was accurate and the conditions were optimum to produce the optimum biodiesel yield.
Cost-effective biodiesel production requires the selection of a sustainable feedstock and process optimization. In this study, maximization of biodiesel yield from MO-WCO has been studied by optimizing transestrification variables. CCD designed transestrification experimental results were subjected to regression analysis to obtain a quadratic model equation for predicting biodiesel yield. Investigation of the effect of transestrification process variables revealed that reaction time and reaction temperature were the most significant variables. Although not as strong as time and temperature, catalyst loading and methanol to oil molar ratio were also significant. Process variables optimization for the transestrification of MO-WCO mixture in producing biodiesel indicated optimum catalyst loading 2.0 of w/v%, methanol to oil molar ratio of 12:1, reaction temperature of 60 ℃, and reaction time of 100 min. Validation experiments confirmed the predicted yield is in good agreement with the experimental result. The study indicated that biodiesel from MO-WCO mixture is a feasible process and fulfills international fuel quality standards to be used as an alternative fuel to the environmentally disastrous petrodiesel fuel. It can be concluded that the use of blended microalgae and waste cooking oil in biodiesel production achieves a remarkable yield (97.72%) and better-quality biodiesel that satisfies the ASTM D6751 and EN 14214 standards as a promising future alternative fuel.
The authors are very grateful to Excellence in Science and Technology (ExiST) project; Ethiopia funded by KfW, Germany, through Center of Excellence, Jimma Institute of Technology (KfW Project No. 51235) for the financial support to conduct the study.
The authors declare no competing interests.
All authors made equal and significant contributions. DB, BA and DB conducted data collection, sample preparation, experimental design, and data analysis. DB and BA prepared the manuscript. All authors approved the manuscript.
A financial support has been received from Jimma Institute of Technology, Center of Excellence to conduct the study.
Data are available in the manuscript but additional data shall be available upon request.
The authors declare that Artificial Intelligence (AI) tools have not been used in the preparation of this article.
[1] |
D. S. Burstein, Conflating medical care with patient care, J. Grad. Med. Educ., 9 (2017), 671. doi: 10.4300/JGME-D-17-00458.1
![]() |
[2] | E. Codier, D. D. Codier, Could emotional intelligence make patients safer, Am. J. Nurs., 117 (2017), 58-62. |
[3] |
R. Diver, T. Quince, S. Barclay, J. Benson, J. Brimicombe, D. Wood, et al., Palliative care in medical practice: medical students' expectations, BMJ Support Palliat Care, 8 (2018), 285-288. doi: 10.1136/bmjspcare-2017-001486
![]() |
[4] |
S. M. Anwar, M. Majid, A. Qayyum, M. Awais, M. Alnowami, M. K. Khan, Medical image analysis using convolutional neural networks: a review, J. Med. Syst., 42 (2018), 226. doi: 10.1007/s10916-018-1001-y
![]() |
[5] |
W. Luo, W. Yang, Y. Zhang, Convolutional neural network for detecting odontocete echolocation clicks, J. Acoust. Soc. Am., 145 (2019), 7. doi: 10.1121/1.5085647
![]() |
[6] |
M. Galgano, G. Toshkezi, X. Qiu, T. Russell, L. Chin, L. R. Zhao, Traumatic brain injury: current treatment strategies and future endeavors, Cell Transplant, 26 (2017), 1118-1130. doi: 10.1177/0963689717714102
![]() |
[7] |
A. Khellaf, D. Z. Khan, A. Helmy, Recent advances in traumatic brain injury, J. Neur., 266 (2019), 2878-2889. doi: 10.1007/s00415-019-09541-4
![]() |
[8] |
D. Najem, K. Rennie, M. Ribecco-Lutkiewicz, D. Ly, J. Haukenfrers, Q. Liu, et al., Traumatic brain injury: classification, models, and markers, Biochem. Cell Biol., 96 (2018), 391-406. doi: 10.1139/bcb-2016-0160
![]() |
[9] | W. Lina, J. Ding, Behavior detection method of openpose combined with Yolo network, (2020), 326-330. |
[10] | S. Sivamani, S. H. Choi, D. H. Lee, J. Park, S. Chon, Automatic posture detection of pigs on real-time using Yolo framework, Int. J. Res. Trends Innov., 5 (2020), 81-88. |
[11] |
M. Sarıgül, B. M. Ozyildirim, M. Avci, Differential convolutional neural network, Neur. Netw., 116 (2019), 279-287. doi: 10.1016/j.neunet.2019.04.025
![]() |
[12] | P. Xuan, S. Pan, T. Zhang, Y. Liu, H. Sun, Graph convolutional network and convolutional neural network based method for predicting lncRNA-disease associations, Cells, 8 (2019). |
[13] |
K. Yasaka, H. Akai, A. Kunimatsu, S. Kiryu, O. Abe, Deep learning with convolutional neural network in radiology, Jpn. J. Radiol., 36 (2018), 257-272. doi: 10.1007/s11604-018-0726-3
![]() |
[14] |
A. O. Alia, M. L. Petrunich-Rutherford, Anxiety-like behavior and whole-body cortisol responses to components of energy drinks in zebrafish (Danio rerio), Peer J., 7 (2019), e7546. doi: 10.7717/peerj.7546
![]() |
[15] | F. Barthels, J. Kisser, R. Pietrowsky, Orthorexic eating behavior and body dissatisfaction in a sample of young females, Eat. Weight Disord., 2020. |
[16] |
A. Mathis, P. Mamidanna, K. M. Cury, T. Abe, V. N. Murthy, M. W. Mathis, et al., DeepLabCut: markerless pose estimation of user-defined body parts with deep learning, Nat. Neurosci., 21 (2018), 1281-1289. doi: 10.1038/s41593-018-0209-y
![]() |
[17] | E. Štefanová, P. Bakalár, T. Baška, Eating-disordered behavior in adolescents: associations with body image, body composition and physical activity, Int. J. Environ. Res. Public Health, 17 (2020). |
[18] |
E. Yilmaz, Influence of lubricating conditions on the two-body wear behavior and hardness of titanium alloys for biomedical applications, Comput. Methods Biomech. Biomed. Engin., 23 (2020), 1377-1386. doi: 10.1080/10255842.2020.1804882
![]() |
[19] |
M. Atzori, H. Müller, PaWFE: Fast signal feature extraction using parallel time windows, Front. Neur., 13 (2019), 74. doi: 10.3389/fnins.2019.00074
![]() |
[20] |
X. Fang, N. Han, J. Wu, Y. Xu, J. Yang, W. K. Wong, et al., Approximate low-rank projection learning for feature extraction, IEEE Trans. Neur. Netw. Learn. Syst., 29 (2018), 5228-5241. doi: 10.1109/TNNLS.2018.2796133
![]() |
[21] |
Q. Shi, Y. M. Cheung, Q. Zhao, H. Lu, Feature extraction for incomplete data via low-rank tensor decomposition with feature regularization, IEEE Trans. Neur. Netw. Learn. Syst., 30 (2019), 1803-1817. doi: 10.1109/TNNLS.2018.2873655
![]() |
[22] |
Z. Y. Zhang, D. D. Gui, M. Sha, J. Liu, H. Y. Wang, Raman chemical feature extraction for quality control of dairy products, J. Dairy Sci., 102 (2019), 68-76. doi: 10.3168/jds.2018-14569
![]() |
1. | Samuel Kofi Tulashie, Enoch Mbawin Alale, Philip Quasi Agudah, Clement Akonnor Osei, Caleb Addai Munumkum, Bright Kombla Gah, Emmanuel Boafo Baidoo, A review on the production of biodiesel from waste cooking oil: a circular economy approach, 2024, 1759-7269, 1, 10.1080/17597269.2024.2384277 | |
2. | Bezu Abera Geresu, Million Ebba, Investigations on the removal of phosphate and nitrate using a mixture of cactus and Moringa seed powder via RSM techniques, 2024, 320, 19443986, 100856, 10.1016/j.dwt.2024.100856 | |
3. | Nakachew Genet Mengistu, Menelik Walle Mekonen, Yewondwosen Gzate Ayalew, Leul Fenta Demisie, Tayachew Nega, Experimental investigation on diesel engine performance and emission characteristics using waste cooking oil blended with diesel as biodiesel fuel, 2024, 4, 2730-7719, 10.1007/s43937-024-00051-7 | |
4. | Hussein A. Mahmood, Ali O. Al-Sulttani, Hayder A. Alrazen, Osam H. Attia, The impact of different compression ratios on emissions, and combustion characteristics of a biodiesel engine, 2024, 12, 2333-8334, 924, 10.3934/energy.2024043 | |
5. | M. Sabri, Edo Fransisko Ginting, Josua Jose Silalahi, Biodiesel Production of WCO-Neem Oil and Mixed Using Pilot Plant Scale with Ultrasound and Overhead Stirred and Characteristic of Emissions in Fire Tube Boiler, 2024, 26660164, 101029, 10.1016/j.cscee.2024.101029 | |
6. | E.O. Babatunde, S. Enomah, O.M. Akwenuke, T.F. Adepoju, C.O. Okwelum, M.M. Mundu, A. Aiki, O.D. Oghenejabor, Novel-Activated Carbon from Waste Green Coconut Husks for the Synthesis of Biodiesel from Pig Fat Oil Blends with Tallow Seed Oil, 2024, 26660164, 101058, 10.1016/j.cscee.2024.101058 | |
7. | Md. Amjad Patwary, M.A.K. Azad, Iftekhar Ahmad, Md. Kashem Ali, Jahid Hasan Shourove, Jenat Yeasmin, G.M. Rabiul Islam, Effectiveness, characterization and optimization of botanical extracts: An ecofriendly approach for management of thrips infestation on tea in Bangladesh, 2025, 12, 27730786, 100118, 10.1016/j.napere.2025.100118 | |
8. | Sadia Husaini, Akshara Kadire, Raj Kumar Verma, Madhuri Pydimalla, Biodiesel production from non-edible mixed oils: a sustainable approach using jatropha, karanja and waste cooking oil, 2025, 48, 0973-7669, 10.1007/s12034-025-03414-5 |
Factors | Symbols | Units | Coded levels | ||||
–Ɵ | –1 | 0 | +1 | +Ɵ | |||
Uncoded levels | |||||||
Catalyst loading | A | (w/v%) | 0.50 | 1.0 | 1.50 | 2.0 | 2.5 |
Methanol to oil ratio | B | (v/v) | 3:1 | 6:1 | 9:1 | 12:1 | 15:1 |
Reaction temperature | C | (℃) | 48 | 52 | 56 | 60 | 64 |
Reaction time | D | (min) | 25 | 50 | 75 | 100 | 125 |
Property | Individual oils | Oil mixtures | |||||
MO | WCO | MO50:WCO50 | MO60:WCO40 | MO70:WCO30 | MO80:WCO20 | MO90:WCO10 | |
Density at 20 ℃ (Kg/m3) | 902.1 | 902.51 | 903.15 | 902.50 | 901.01 | 902.21 | 902.67 |
Viscosity (mm2/s) | 42.40 | 45.10 | 50.19 | 51.02 | 41.47 | 45.91 | 44.82 |
AV (mg KOH/100g) | 16.46 | 21.21 | 18.11 | 15.01 | 13.02 | 14.52 | 14.58 |
HHV (MJ/Kg) | 40.81 | 39.63 | 38.90 | 39.95 | 40.23 | 37.99 | 38.99 |
WCO | Fatty acid type and number of carbon atom in the chain | |||||||||||||||||
Palmitic (C16:0) | Stearic (C18:0) | Oleic (C18:1) | Linoleic (C18:2) | Linolenic (C18:3) | Myristic (C14:0) | Erucic (C22:1) | Palmitoleic (C16:1) | Eicosenic (C20:1) | Tetracoanic (C24:0) | Arachidic (C20:0) | Behenic (C22:0) | |||||||
(Wt%) | 20.42 | 4.18 | 52.09 | 13.45 | 0.83 | 0.95 | 0.07 | 4.61 | 0.87 | 0.03 | 0.13 | 0.04 | ||||||
Microalgae (C. vulgaris) | Fatty acid type and number of carbon atom in the chain | |||||||||||||||||
Myristic (C14:0) | Palmitic (C16:0) | Stearic (C18:0) | Palmitoleic (C16:1) | Oleic (C18:1) | Linoleic (C18:2) | Linolenic (C18:3) | Eicosapentaenoic (C20:5) | |||||||||||
(Wt%) | 7.10 | 22.70 | 21.50 | 11.30 | 7.81 | 6.90 | 16.11 | 6.10 |
Physicochemical properties | Unit | Determined value | Standards [9] | ||
MO70WCO30 | Biodiesel | ASTM D6751 | EN 14214 | ||
Relative density at 20 ℃ | - | 0.901 | 0.890 | 0.86–0.90 | 0.86–0.90 |
Density at 20 ℃ | [kg/m3] | 901.0 | 890.0 | 860–900 | 860–900 |
Kinematic viscosity at 40 ℃ | [mm2/s] | 41.47 | 4.50 | 1.9–6.0 | 3.5–5.0 |
Flash point | [℃] | 168.33 | 180.0 | ≥130 | ≥120 |
Cetane number | - | 46.83 | 77.76 | ≥47 | ≥51 |
Acid value | [mg KOH/g] | 13.02 | 0.049 | ≤0.05 | ≤0.05 |
Free fatty acid content | [%] | 6.51 | 0.445 | ≤0.05 | ≤0.05 |
Saponification value | [mg KOH/g] | 180.92 | 146.1 | 0–370 | - |
Iodine value | [g I2/100 g] | 118.40 | 12.90 | ≤120 | ≤140 |
Water content | [%] | 0.033 | 0.020 | ≤0.03 | ≤0.05 |
Ash content | [%] | 0.061 | 0.003 | ≤0.03 | ≤0.02 |
Pour point | [℃] | –5.0 | –2.50 | –5 to 10 | –5 to 10 |
Heating value | [MJ/kg] | 40.23 | 43.25 | ≥42.0 | ≥35.0 |
Methyl ester content | [%] | - | 99.44 | ≥96.50 | ≥96.50 |
Biodiesel feedstock | Properties | Ref. | ||||||||
Density (kg/m3) | Kinematic viscosity at 40 ℃ (mm2/s) | CN (-) | PP (℃) | FP (℃) | AV (mg KOH/g) | HV (MJ/kg) | SV (mg KOH/g of oil) | IV (gI2/100 g) | ||
Jatropha curcas and Ceiba pentandra oils | 831.2 | 3.95 | 58.0 | 0.50 | 196 | 0.025 | 40.929 | - | - | [5] |
Castor seed and waste fish oils | 898.9 | 3.61 | 57.0 | –2.0 | 84.0 | 0.06 | - | 175.24 | 91.0 | [55] |
Neem and pig fat oils | 825.0 | 2.20 | 64.6 | 3.0 | 120 | 0.42 | - | 188.60 | 47.2 | [56] |
Jatropha and algae oils | - | 4.1 | - | - | 115 | - | - | - | 83.2 | [57] |
WCO and Calophyllum inophyllum oil | 861.6 | 4.72 | - | 2.0 | 160.5 | 0.46 | 41.35 | - | - | [18] |
Castor and karanja oils | 944.7 | 7.83 | - | 12 | 160 | 0.48 | 38.63 | - | - | [58] |
Castor seed and microalgae oils | 890.0 | 5.80 | 58.1 | - | 133.3 | 0.10 | 48.12 | 1.80 | 82.22 | [9] |
Palm and sesame oils | 880.0 | 4.42 | 53.4 | 3.80 | > 150 | 0.37 | 41.24 | - | - | [59] |
Carica papaya, Citrus sinensis, and Hibiscus sabdariffa seed oils | 872.0 | 1.82 | 64.4 | –12 | 130 | 0.36 | - | 178.24 | 52.62 | [60] |
Mahua and simarouba oils | 856.0 | 4.77 | - | 3.60 | 134 | 0.445 | 37.02 | - | - | [61] |
Waste cooking oil and Schleichera oleosa oil | 857.0 | 4.459 | - | 9.0 | 172 | 0.28 | 39.95 | - | - | [54] |
Microalgae oil and WCO | 890.0 | 4.50 | 77.8 | –2.5 | 180.0 | 0.049 | 43.25 | 146.1 | 12.90 | This study |
S.No. | Factors actual levels | Factors coded levels | Biodiesel yield (%) | ||||||||
Catalyst loading (w/v%) | Molar ratio (v/v) | Temperature (℃) | Time (min) | ||||||||
A | B | C | D | Actual | Predicted | Residual | |||||
1 | 2.0 | 6:1 | 52.0 | 50.0 | 1 | –1 | –1 | –1 | 29.05 | 29.60 | –0.55 |
2 | 2.0 | 12:1 | 60.0 | 50.0 | 1 | 1 | 1 | –1 | 64.44 | 62.94 | 1.50 |
3 | 1.0 | 12:1 | 52.0 | 100.0 | –1 | 1 | –1 | 1 | 51.02 | 49.93 | 1.09 |
4 | 2.0 | 12:1 | 52.0 | 50.0 | 1 | 1 | –1 | –1 | 37.62 | 37.34 | 0.28 |
5 | 1.0 | 6:1 | 60.0 | 100.0 | –1 | –1 | 1 | 1 | 67.68 | 66.59 | 1.09 |
6 | 2.0 | 12:1 | 60.0 | 100.0 | 1 | 1 | 1 | 1 | 98.80 | 99.10 | –0.30 |
7 | 2.0 | 6:1 | 52.0 | 100.0 | 1 | –1 | –1 | 1 | 42.88 | 42.93 | –0.52 |
8 | 2.0 | 12:1 | 52.0 | 100.0 | 1 | 1 | –1 | 1 | 53.24 | 52.53 | 0.71 |
9 | 1.0 | 12:1 | 60.0 | 100.0 | –1 | 1 | 1 | 1 | 81.24 | 80.82 | 0.42 |
10 | 1.0 | 6:1 | 52.0 | 50.0 | –1 | –1 | –1 | –1 | 29.32 | 27.66 | 1.66 |
11 | 1.0 | 6:1 | 52.0 | 100.0 | –1 | –1 | –1 | 1 | 40.32 | 41.95 | –1.63 |
12 | 1.0 | 12:1 | 52.0 | 50.0 | –1 | 1 | –1 | –1 | 33.68 | 33.78 | –0.10 |
13 | 1.0 | 6:1 | 60.0 | 50.0 | –1 | –1 | 1 | –1 | 30.50 | 31.34 | –0.84 |
14 | 2.0 | 6:1 | 60.0 | 50.0 | 1 | –1 | 1 | –1 | 49.24 | 48.96 | 0.28 |
15 | 2.0 | 6:1 | 60.0 | 100.0 | 1 | –1 | 1 | 1 | 83.22 | 83.25 | –0.03 |
16 | 1.0 | 12:1 | 60.0 | 50.0 | –1 | 1 | 1 | –1 | 45.12 | 43.70 | 1.42 |
17 | 1.50 | 15:1 | 56.0 | 75.0 | 0 | 2 | 0 | 0 | 52.42 | 54.31 | –1.89 |
18 | 2.50 | 9:1 | 56.0 | 75.0 | 2 | 0 | 0 | 0 | 55.20 | 55.49 | –0.29 |
19 | 1.50 | 9:1 | 56.0 | 125.0 | 0 | 0 | 0 | 2 | 83.44 | 83.47 | –0.03 |
20 | 1.50 | 9:1 | 48.0 | 75.0 | 0 | 0 | –2 | 0 | 35.94 | 36.03 | –0.09 |
21 | 0.50 | 9:1 | 56.0 | 75.0 | –2 | 0 | 0 | 0 | 34.32 | 35.26 | –0.94 |
22 | 1.50 | 9:1 | 64.0 | 75.0 | 0 | 0 | 2 | 0 | 85.12 | 86.27 | –1.15 |
23 | 1.50 | 9:1 | 56.0 | 25.0 | 0 | 0 | 0 | –2 | 31.83 | 33.03 | –1.20 |
24 | 1.50 | 3:1 | 56.0 | 75.0 | 0 | –2 | 0 | 0 | 33.00 | 32.35 | 0.65 |
25 | 1.50 | 9:1 | 56.0 | 75.0 | 0 | 0 | 0 | 0 | 71.30 | 71.93 | –0.63 |
26 | 1.50 | 9:1 | 56.0 | 75.0 | 0 | 0 | 0 | 0 | 70.50 | 71.93 | –1.43 |
27 | 1.50 | 9:1 | 56.0 | 75.0 | 0 | 0 | 0 | 0 | 70.50 | 71.93 | –1.43 |
28 | 1.50 | 9:1 | 56.0 | 75.0 | 0 | 0 | 0 | 0 | 70.50 | 71.93 | –1.43 |
29 | 1.50 | 9:1 | 56.0 | 75.0 | 0 | 0 | 0 | 0 | 74.40 | 71.93 | 2.47 |
30 | 1.50 | 9:1 | 56.0 | 75.0 | 0 | 0 | 0 | 0 | 74.40 | 71.93 | 2.47 |
Mixed feedstock | Optimum parameters | Biodiesel yield (%) | References | |||
Catalyst loading (Wt%) | Alcohol to oil ratio | Time (min) | Temperature (℃) | |||
Castor & karanja oils | 10.0 | 6:1 | 1440 | 50 | 78.0 | [58] |
Castor seed oil & microalgae oil | 1.23 | 6:1 | - | 51 | 92.36 | [9] |
C. inophyllum & WCO oils | 0.50 | 60% | 90 | 55 | 96.84 | [64] |
J. curcas & C. pentandra oils | 0.50 | - | 180 | 60 | 93.33 | [6] |
Mahua & simarouba oils | 3.50 | 5:1 | 30 | 60 | 98.0 | [65] |
Castor bean & WCO oils | 0.75 | 8:1 | 30 | 60 | 97.20 | [53] |
Sterculia foetida & rice bran oils | 0.70 | 42% | 60 | 51 | 98.93 | [45] |
J. curcas, castor seed & WCO oils | 7.0 | 12:1 | 59 | 59 | 92.35 | [62] |
MO & WCO oils | 2.0 | 12:1 | 100 | 60 | 97.72 | This study |
Source | The sum of squares (SSs) | DF | Mean Square | F-value | P-value | Status |
Model | 12057.84 | 14 | 861.27 | 319.25 | < 0.0001 | Significant |
A | 613.78 | 1 | 613.78 | 227.51 | < 0.0001 | |
B | 723.69 | 1 | 723.69 | 268.25 | < 0.0001 | |
C | 3786.84 | 1 | 3786.84 | 1403.66 | < 0.0001 | |
D | 3816.54 | 1 | 3816.54 | 1414.67 | < 0.0001 | |
AB | 2.62 | 1 | 2.62 | 0.9698 | 0.3404 | |
AC | 245.78 | 1 | 245.78 | 91.10 | < 0.0001 | |
AD | 0.9264 | 1 | 0.9264 | 0.3434 | 0.5666 | |
BC | 38.97 | 1 | 38.97 | 14.44 | 0.0017 | |
BD | 3.47 | 1 | 3.47 | 1.29 | 0.2746 | |
CD | 439.43 | 1 | 439.43 | 162.88 | < 0.0001 | |
A² | 1208.90 | 1 | 1208.90 | 448.10 | < 0.0001 | |
B² | 1402.75 | 1 | 1402.75 | 519.95 | < 0.0001 | |
C² | 199.41 | 1 | 199.41 | 73.92 | < 0.0001 | |
D² | 320.84 | 1 | 320.84 | 118.92 | < 0.0001 | |
Residual | 40.47 | 15 | 2.70 | - | - | |
LoF | 21.73 | 10 | 2.17 | 0.5801 | 0.7833 | Not significant |
Pure error | 18.73 | 5 | 3.75 | - | - | |
Cor total | 12098.31 | 29 | - | - | - |
Model | SD | R² | R² adjusted | R² predicted | PRESS | Status |
Linear | 11.24 | 0.7390 | 0.6973 | 0.6549 | 4175.10 | Not aliased |
2FI | 11.30 | 0.7995 | 0.6939 | 0.6744 | 3939.57 | Not aliased |
Quadratic | 1.64 | 0.9967 | 0.9935 | 0.9874 | 152.16 | Suggested |
Cubic | 1.91 | 0.9979 | 0.9912 | 0.9165 | 1009.68 | Aliased |
Factor | Value |
SD | 1.64 |
Mean | 56.01 |
CV | 2.93 |
R² | 0.9967 |
R² adj. | 0.9935 |
R² pred. | 0.9874 |
Adeq. Prec. | 61.5104 |
Factors | Symbols | Units | Coded levels | ||||
–Ɵ | –1 | 0 | +1 | +Ɵ | |||
Uncoded levels | |||||||
Catalyst loading | A | (w/v%) | 0.50 | 1.0 | 1.50 | 2.0 | 2.5 |
Methanol to oil ratio | B | (v/v) | 3:1 | 6:1 | 9:1 | 12:1 | 15:1 |
Reaction temperature | C | (℃) | 48 | 52 | 56 | 60 | 64 |
Reaction time | D | (min) | 25 | 50 | 75 | 100 | 125 |
Property | Individual oils | Oil mixtures | |||||
MO | WCO | MO50:WCO50 | MO60:WCO40 | MO70:WCO30 | MO80:WCO20 | MO90:WCO10 | |
Density at 20 ℃ (Kg/m3) | 902.1 | 902.51 | 903.15 | 902.50 | 901.01 | 902.21 | 902.67 |
Viscosity (mm2/s) | 42.40 | 45.10 | 50.19 | 51.02 | 41.47 | 45.91 | 44.82 |
AV (mg KOH/100g) | 16.46 | 21.21 | 18.11 | 15.01 | 13.02 | 14.52 | 14.58 |
HHV (MJ/Kg) | 40.81 | 39.63 | 38.90 | 39.95 | 40.23 | 37.99 | 38.99 |
WCO | Fatty acid type and number of carbon atom in the chain | |||||||||||||||||
Palmitic (C16:0) | Stearic (C18:0) | Oleic (C18:1) | Linoleic (C18:2) | Linolenic (C18:3) | Myristic (C14:0) | Erucic (C22:1) | Palmitoleic (C16:1) | Eicosenic (C20:1) | Tetracoanic (C24:0) | Arachidic (C20:0) | Behenic (C22:0) | |||||||
(Wt%) | 20.42 | 4.18 | 52.09 | 13.45 | 0.83 | 0.95 | 0.07 | 4.61 | 0.87 | 0.03 | 0.13 | 0.04 | ||||||
Microalgae (C. vulgaris) | Fatty acid type and number of carbon atom in the chain | |||||||||||||||||
Myristic (C14:0) | Palmitic (C16:0) | Stearic (C18:0) | Palmitoleic (C16:1) | Oleic (C18:1) | Linoleic (C18:2) | Linolenic (C18:3) | Eicosapentaenoic (C20:5) | |||||||||||
(Wt%) | 7.10 | 22.70 | 21.50 | 11.30 | 7.81 | 6.90 | 16.11 | 6.10 |
Physicochemical properties | Unit | Determined value | Standards [9] | ||
MO70WCO30 | Biodiesel | ASTM D6751 | EN 14214 | ||
Relative density at 20 ℃ | - | 0.901 | 0.890 | 0.86–0.90 | 0.86–0.90 |
Density at 20 ℃ | [kg/m3] | 901.0 | 890.0 | 860–900 | 860–900 |
Kinematic viscosity at 40 ℃ | [mm2/s] | 41.47 | 4.50 | 1.9–6.0 | 3.5–5.0 |
Flash point | [℃] | 168.33 | 180.0 | ≥130 | ≥120 |
Cetane number | - | 46.83 | 77.76 | ≥47 | ≥51 |
Acid value | [mg KOH/g] | 13.02 | 0.049 | ≤0.05 | ≤0.05 |
Free fatty acid content | [%] | 6.51 | 0.445 | ≤0.05 | ≤0.05 |
Saponification value | [mg KOH/g] | 180.92 | 146.1 | 0–370 | - |
Iodine value | [g I2/100 g] | 118.40 | 12.90 | ≤120 | ≤140 |
Water content | [%] | 0.033 | 0.020 | ≤0.03 | ≤0.05 |
Ash content | [%] | 0.061 | 0.003 | ≤0.03 | ≤0.02 |
Pour point | [℃] | –5.0 | –2.50 | –5 to 10 | –5 to 10 |
Heating value | [MJ/kg] | 40.23 | 43.25 | ≥42.0 | ≥35.0 |
Methyl ester content | [%] | - | 99.44 | ≥96.50 | ≥96.50 |
Biodiesel feedstock | Properties | Ref. | ||||||||
Density (kg/m3) | Kinematic viscosity at 40 ℃ (mm2/s) | CN (-) | PP (℃) | FP (℃) | AV (mg KOH/g) | HV (MJ/kg) | SV (mg KOH/g of oil) | IV (gI2/100 g) | ||
Jatropha curcas and Ceiba pentandra oils | 831.2 | 3.95 | 58.0 | 0.50 | 196 | 0.025 | 40.929 | - | - | [5] |
Castor seed and waste fish oils | 898.9 | 3.61 | 57.0 | –2.0 | 84.0 | 0.06 | - | 175.24 | 91.0 | [55] |
Neem and pig fat oils | 825.0 | 2.20 | 64.6 | 3.0 | 120 | 0.42 | - | 188.60 | 47.2 | [56] |
Jatropha and algae oils | - | 4.1 | - | - | 115 | - | - | - | 83.2 | [57] |
WCO and Calophyllum inophyllum oil | 861.6 | 4.72 | - | 2.0 | 160.5 | 0.46 | 41.35 | - | - | [18] |
Castor and karanja oils | 944.7 | 7.83 | - | 12 | 160 | 0.48 | 38.63 | - | - | [58] |
Castor seed and microalgae oils | 890.0 | 5.80 | 58.1 | - | 133.3 | 0.10 | 48.12 | 1.80 | 82.22 | [9] |
Palm and sesame oils | 880.0 | 4.42 | 53.4 | 3.80 | > 150 | 0.37 | 41.24 | - | - | [59] |
Carica papaya, Citrus sinensis, and Hibiscus sabdariffa seed oils | 872.0 | 1.82 | 64.4 | –12 | 130 | 0.36 | - | 178.24 | 52.62 | [60] |
Mahua and simarouba oils | 856.0 | 4.77 | - | 3.60 | 134 | 0.445 | 37.02 | - | - | [61] |
Waste cooking oil and Schleichera oleosa oil | 857.0 | 4.459 | - | 9.0 | 172 | 0.28 | 39.95 | - | - | [54] |
Microalgae oil and WCO | 890.0 | 4.50 | 77.8 | –2.5 | 180.0 | 0.049 | 43.25 | 146.1 | 12.90 | This study |
S.No. | Factors actual levels | Factors coded levels | Biodiesel yield (%) | ||||||||
Catalyst loading (w/v%) | Molar ratio (v/v) | Temperature (℃) | Time (min) | ||||||||
A | B | C | D | Actual | Predicted | Residual | |||||
1 | 2.0 | 6:1 | 52.0 | 50.0 | 1 | –1 | –1 | –1 | 29.05 | 29.60 | –0.55 |
2 | 2.0 | 12:1 | 60.0 | 50.0 | 1 | 1 | 1 | –1 | 64.44 | 62.94 | 1.50 |
3 | 1.0 | 12:1 | 52.0 | 100.0 | –1 | 1 | –1 | 1 | 51.02 | 49.93 | 1.09 |
4 | 2.0 | 12:1 | 52.0 | 50.0 | 1 | 1 | –1 | –1 | 37.62 | 37.34 | 0.28 |
5 | 1.0 | 6:1 | 60.0 | 100.0 | –1 | –1 | 1 | 1 | 67.68 | 66.59 | 1.09 |
6 | 2.0 | 12:1 | 60.0 | 100.0 | 1 | 1 | 1 | 1 | 98.80 | 99.10 | –0.30 |
7 | 2.0 | 6:1 | 52.0 | 100.0 | 1 | –1 | –1 | 1 | 42.88 | 42.93 | –0.52 |
8 | 2.0 | 12:1 | 52.0 | 100.0 | 1 | 1 | –1 | 1 | 53.24 | 52.53 | 0.71 |
9 | 1.0 | 12:1 | 60.0 | 100.0 | –1 | 1 | 1 | 1 | 81.24 | 80.82 | 0.42 |
10 | 1.0 | 6:1 | 52.0 | 50.0 | –1 | –1 | –1 | –1 | 29.32 | 27.66 | 1.66 |
11 | 1.0 | 6:1 | 52.0 | 100.0 | –1 | –1 | –1 | 1 | 40.32 | 41.95 | –1.63 |
12 | 1.0 | 12:1 | 52.0 | 50.0 | –1 | 1 | –1 | –1 | 33.68 | 33.78 | –0.10 |
13 | 1.0 | 6:1 | 60.0 | 50.0 | –1 | –1 | 1 | –1 | 30.50 | 31.34 | –0.84 |
14 | 2.0 | 6:1 | 60.0 | 50.0 | 1 | –1 | 1 | –1 | 49.24 | 48.96 | 0.28 |
15 | 2.0 | 6:1 | 60.0 | 100.0 | 1 | –1 | 1 | 1 | 83.22 | 83.25 | –0.03 |
16 | 1.0 | 12:1 | 60.0 | 50.0 | –1 | 1 | 1 | –1 | 45.12 | 43.70 | 1.42 |
17 | 1.50 | 15:1 | 56.0 | 75.0 | 0 | 2 | 0 | 0 | 52.42 | 54.31 | –1.89 |
18 | 2.50 | 9:1 | 56.0 | 75.0 | 2 | 0 | 0 | 0 | 55.20 | 55.49 | –0.29 |
19 | 1.50 | 9:1 | 56.0 | 125.0 | 0 | 0 | 0 | 2 | 83.44 | 83.47 | –0.03 |
20 | 1.50 | 9:1 | 48.0 | 75.0 | 0 | 0 | –2 | 0 | 35.94 | 36.03 | –0.09 |
21 | 0.50 | 9:1 | 56.0 | 75.0 | –2 | 0 | 0 | 0 | 34.32 | 35.26 | –0.94 |
22 | 1.50 | 9:1 | 64.0 | 75.0 | 0 | 0 | 2 | 0 | 85.12 | 86.27 | –1.15 |
23 | 1.50 | 9:1 | 56.0 | 25.0 | 0 | 0 | 0 | –2 | 31.83 | 33.03 | –1.20 |
24 | 1.50 | 3:1 | 56.0 | 75.0 | 0 | –2 | 0 | 0 | 33.00 | 32.35 | 0.65 |
25 | 1.50 | 9:1 | 56.0 | 75.0 | 0 | 0 | 0 | 0 | 71.30 | 71.93 | –0.63 |
26 | 1.50 | 9:1 | 56.0 | 75.0 | 0 | 0 | 0 | 0 | 70.50 | 71.93 | –1.43 |
27 | 1.50 | 9:1 | 56.0 | 75.0 | 0 | 0 | 0 | 0 | 70.50 | 71.93 | –1.43 |
28 | 1.50 | 9:1 | 56.0 | 75.0 | 0 | 0 | 0 | 0 | 70.50 | 71.93 | –1.43 |
29 | 1.50 | 9:1 | 56.0 | 75.0 | 0 | 0 | 0 | 0 | 74.40 | 71.93 | 2.47 |
30 | 1.50 | 9:1 | 56.0 | 75.0 | 0 | 0 | 0 | 0 | 74.40 | 71.93 | 2.47 |
Mixed feedstock | Optimum parameters | Biodiesel yield (%) | References | |||
Catalyst loading (Wt%) | Alcohol to oil ratio | Time (min) | Temperature (℃) | |||
Castor & karanja oils | 10.0 | 6:1 | 1440 | 50 | 78.0 | [58] |
Castor seed oil & microalgae oil | 1.23 | 6:1 | - | 51 | 92.36 | [9] |
C. inophyllum & WCO oils | 0.50 | 60% | 90 | 55 | 96.84 | [64] |
J. curcas & C. pentandra oils | 0.50 | - | 180 | 60 | 93.33 | [6] |
Mahua & simarouba oils | 3.50 | 5:1 | 30 | 60 | 98.0 | [65] |
Castor bean & WCO oils | 0.75 | 8:1 | 30 | 60 | 97.20 | [53] |
Sterculia foetida & rice bran oils | 0.70 | 42% | 60 | 51 | 98.93 | [45] |
J. curcas, castor seed & WCO oils | 7.0 | 12:1 | 59 | 59 | 92.35 | [62] |
MO & WCO oils | 2.0 | 12:1 | 100 | 60 | 97.72 | This study |
Source | The sum of squares (SSs) | DF | Mean Square | F-value | P-value | Status |
Model | 12057.84 | 14 | 861.27 | 319.25 | < 0.0001 | Significant |
A | 613.78 | 1 | 613.78 | 227.51 | < 0.0001 | |
B | 723.69 | 1 | 723.69 | 268.25 | < 0.0001 | |
C | 3786.84 | 1 | 3786.84 | 1403.66 | < 0.0001 | |
D | 3816.54 | 1 | 3816.54 | 1414.67 | < 0.0001 | |
AB | 2.62 | 1 | 2.62 | 0.9698 | 0.3404 | |
AC | 245.78 | 1 | 245.78 | 91.10 | < 0.0001 | |
AD | 0.9264 | 1 | 0.9264 | 0.3434 | 0.5666 | |
BC | 38.97 | 1 | 38.97 | 14.44 | 0.0017 | |
BD | 3.47 | 1 | 3.47 | 1.29 | 0.2746 | |
CD | 439.43 | 1 | 439.43 | 162.88 | < 0.0001 | |
A² | 1208.90 | 1 | 1208.90 | 448.10 | < 0.0001 | |
B² | 1402.75 | 1 | 1402.75 | 519.95 | < 0.0001 | |
C² | 199.41 | 1 | 199.41 | 73.92 | < 0.0001 | |
D² | 320.84 | 1 | 320.84 | 118.92 | < 0.0001 | |
Residual | 40.47 | 15 | 2.70 | - | - | |
LoF | 21.73 | 10 | 2.17 | 0.5801 | 0.7833 | Not significant |
Pure error | 18.73 | 5 | 3.75 | - | - | |
Cor total | 12098.31 | 29 | - | - | - |
Model | SD | R² | R² adjusted | R² predicted | PRESS | Status |
Linear | 11.24 | 0.7390 | 0.6973 | 0.6549 | 4175.10 | Not aliased |
2FI | 11.30 | 0.7995 | 0.6939 | 0.6744 | 3939.57 | Not aliased |
Quadratic | 1.64 | 0.9967 | 0.9935 | 0.9874 | 152.16 | Suggested |
Cubic | 1.91 | 0.9979 | 0.9912 | 0.9165 | 1009.68 | Aliased |
Factor | Value |
SD | 1.64 |
Mean | 56.01 |
CV | 2.93 |
R² | 0.9967 |
R² adj. | 0.9935 |
R² pred. | 0.9874 |
Adeq. Prec. | 61.5104 |