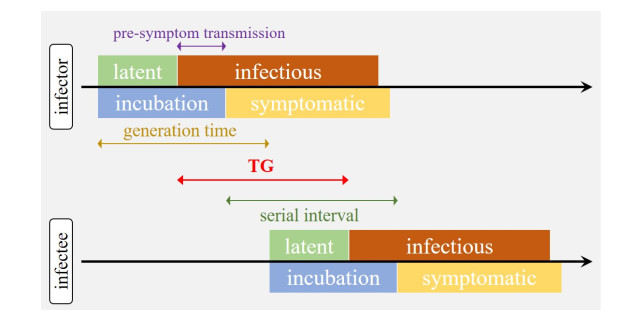
The coronavirus disease 2019 (COVID-19) emerged in Wuhan, China in the end of 2019, and soon became a serious public health threat globally. Due to the unobservability, the time interval between transmission generations (TG), though important for understanding the disease transmission patterns, of COVID-19 cannot be directly summarized from surveillance data. In this study, we develop a likelihood framework to estimate the TG and the pre-symptomatic transmission period from the serial interval observations from the individual transmission events. As the results, we estimate the mean of TG at 4.0 days (95%CI: 3.3-4.6), and the mean of pre-symptomatic transmission period at 2.2 days (95%CI: 1.3-4.7). We approximate the mean latent period of 3.3 days, and 32.2% (95%CI: 10.3-73.7) of the secondary infections may be due to pre-symptomatic transmission. The timely and effectively isolation of symptomatic COVID-19 cases is crucial for mitigating the epidemics.
Citation: Shi Zhao. Estimating the time interval between transmission generations when negative values occur in the serial interval data: using COVID-19 as an example[J]. Mathematical Biosciences and Engineering, 2020, 17(4): 3512-3519. doi: 10.3934/mbe.2020198
[1] | Juan Li, Wendi Bao, Xianghong Zhang, Yongzhong Song, Zhigui Lin, Huaiping Zhu . Modelling the transmission and control of COVID-19 in Yangzhou city with the implementation of Zero-COVID policy. Mathematical Biosciences and Engineering, 2023, 20(9): 15781-15808. doi: 10.3934/mbe.2023703 |
[2] | Alexandra Smirnova, Brian Pidgeon, Gerardo Chowell, Yichuan Zhao . The doubling time analysis for modified infectious disease Richards model with applications to COVID-19 pandemic. Mathematical Biosciences and Engineering, 2022, 19(3): 3242-3268. doi: 10.3934/mbe.2022150 |
[3] | Damilola Olabode, Jordan Culp, Allison Fisher, Angela Tower, Dylan Hull-Nye, Xueying Wang . Deterministic and stochastic models for the epidemic dynamics of COVID-19 in Wuhan, China. Mathematical Biosciences and Engineering, 2021, 18(1): 950-967. doi: 10.3934/mbe.2021050 |
[4] | Ryo Kinoshita, Sung-mok Jung, Tetsuro Kobayashi, Andrei R. Akhmetzhanov, Hiroshi Nishiura . Epidemiology of coronavirus disease 2019 (COVID-19) in Japan during the first and second waves. Mathematical Biosciences and Engineering, 2022, 19(6): 6088-6101. doi: 10.3934/mbe.2022284 |
[5] | Sha He, Sanyi Tang, Libin Rong . A discrete stochastic model of the COVID-19 outbreak: Forecast and control. Mathematical Biosciences and Engineering, 2020, 17(4): 2792-2804. doi: 10.3934/mbe.2020153 |
[6] | Dennis L. Chao, Dobromir T. Dimitrov . Seasonality and the effectiveness of mass vaccination. Mathematical Biosciences and Engineering, 2016, 13(2): 249-259. doi: 10.3934/mbe.2015001 |
[7] | Liping Wang, Jing Wang, Hongyong Zhao, Yangyang Shi, Kai Wang, Peng Wu, Lei Shi . Modelling and assessing the effects of medical resources on transmission of novel coronavirus (COVID-19) in Wuhan, China. Mathematical Biosciences and Engineering, 2020, 17(4): 2936-2949. doi: 10.3934/mbe.2020165 |
[8] | Tetsuro Kobayashi, Hiroshi Nishiura . Prioritizing COVID-19 vaccination. Part 2: Real-time comparison between single-dose and double-dose in Japan. Mathematical Biosciences and Engineering, 2022, 19(7): 7410-7424. doi: 10.3934/mbe.2022350 |
[9] | Weike Zhou, Aili Wang, Fan Xia, Yanni Xiao, Sanyi Tang . Effects of media reporting on mitigating spread of COVID-19 in the early phase of the outbreak. Mathematical Biosciences and Engineering, 2020, 17(3): 2693-2707. doi: 10.3934/mbe.2020147 |
[10] | Cong Yang, Yali Yang, Zhiwei Li, Lisheng Zhang . Modeling and analysis of COVID-19 based on a time delay dynamic model. Mathematical Biosciences and Engineering, 2021, 18(1): 154-165. doi: 10.3934/mbe.2021008 |
The coronavirus disease 2019 (COVID-19) emerged in Wuhan, China in the end of 2019, and soon became a serious public health threat globally. Due to the unobservability, the time interval between transmission generations (TG), though important for understanding the disease transmission patterns, of COVID-19 cannot be directly summarized from surveillance data. In this study, we develop a likelihood framework to estimate the TG and the pre-symptomatic transmission period from the serial interval observations from the individual transmission events. As the results, we estimate the mean of TG at 4.0 days (95%CI: 3.3-4.6), and the mean of pre-symptomatic transmission period at 2.2 days (95%CI: 1.3-4.7). We approximate the mean latent period of 3.3 days, and 32.2% (95%CI: 10.3-73.7) of the secondary infections may be due to pre-symptomatic transmission. The timely and effectively isolation of symptomatic COVID-19 cases is crucial for mitigating the epidemics.
The transmission of infectious disease is commonly parameterized as a dynamical process that is governed by the reproduction number and the time interval between the transmission generations [1,2,3]. The serial interval (SI) is the period of time between the onset of symptoms in an infector to that in an associated infectee [4,5,6,7], which is observable and determined with the onsets of symptoms. The time interval between transmission generations (TG) is defined as the period of time between the onset of the infectiousness in a primary case (i.e., infector) to the onset of the infectiousness in an associated secondary case (i.e., infectee) infected by the primary case. Given that an infected individual may commonly become infectious prior to the onset of symptoms, the TG is not observable based on symptoms [6,8], which indicates the pre-symptomatic transmission may occur. Figure 1 illustrates the difference between TG and SI in a transmission chain.
When the infectious period starts with the onset of symptoms, i.e., the latent and incubation periods perfectly match, the TG coincides with the SI, and as such, the pre-symptomatic transmission will not occur [6]. In most of the situations, the latent period may be shorter than the incubation period, and thus, the pre-symptomatic transmission may occur. In minor occasions, when the onset of symptoms in the infectee is earlier than that in its infector, the observed SI will be negative. By contrast, the TG is always non-negative. In the real-world situation, due to the TG data are unobservable, the data of SI are commonly used as a proxy to approximate the true patterns of TG. This approach may become biased and inefficient when there are negative SI observations in the SI data.
Recently, the coronavirus disease 2019 (COVID-19), caused by the severe acute respiratory syndrome coronavirus 2 (SARS-CoV-2), emerged in Wuhan, China in the end of 2019 [9,10,11]. The COVID-19 spread to over 100 foreign countries in a short period of time [12,13]. The World Health Organization (WHO) declared the outbreak to be a public health emergency of international concern on January 30, 2020 [14]. By the end of March 2020, there are over 500000 COVID-19 confirmed cases globally [15]. In previous studies [16,17,18], negative SIs are observed in the transmission events of COVID-19, and considered as a support for the potential risk of pre-symptomatic transmission. Estimating the TG as well as the pre-symptomatic transmission period is essential for understanding the transmission patterns of the COVID-19 and future infectious disease modelling studies.
In this study, we developed a novel likelihood-based framework to estimate the generation time and the risk of pre-symptomatic transmission of COVID-19 by using the observed serial interval data.
The TG is defined on the basis of the infectiousness onset of the infector. Since the infectiousness onset represents the readiness (i.e., start time) of a case to generate other consecutive cases, the TG is the time interval between the start of having transmissibility of two consecutive cases in a transmission chain. Note that the definition of TG is different from that of the generation time (GT), and the latter is defined on a per the exposure of infector basis. All of GT, TG and SI measure the period of time required for an infector to generate an infectee, i.e., the 'infector' in the subsequent transmission generation. Although both GT and TG are non-negative (whereas the SI could be negative), the TG is focusing on the transmissibility, and thus more relevant to the spread of infectious diseases.
Since the incubation period is not shorter than the latent period for an individual patient [6], we denote the difference of the incubation period minus the latent period as d, and thus d ≥ 0, see Fig 1. We name this period (d) as pre-symptomatic transmission period. Let d1 and d2 denotes the pre-symptomatic transmission periods for the infector and infectee respectively. The d1 and d2 are considered as independent and identically distributed (IID) random variables determined by a probability density function (PDF) h(∙) with mean μd and standard deviation (SD) σd. The TG, denoted by g, is determined by a PDF δ(∙) with mean μg and SD σg. Hence, the SI, denoted by s, is defined by s = g + d2 − d1, which is determined by a PDF denoted by f(∙). By convolution, the f(∙) can be formulated in Eq (1).
f(x)=∫δ(x−y)⋅[∫h(z)⋅h(y+z)dz]dy. | (1) |
Straightforwardly, the expectations of g and s are equal, i.e., E[g] = E(s), which indicates the expectation of TG and the expectation of SI are consistent.
With the PDF formulated in Eq (1), the associated likelihood profile can be adopted to estimate the parameters in PDFs of h(∙) and δ(∙). The likelihood, l(∙), is defined as in Eq (2).
l(Θ|x1,…,xn)=∑ilogf(xi|Θ), | (2) |
where the Θ denotes the vector of the PDF parameters to be estimated, and the xis are the SI observations. Following previous studies [10,17,19,20,21], Gamma distributions are presumed for both h(∙) and δ(∙) for demonstration purpose. As such, by fitting to the SI data in Du et al. [16], the means and SDs of d and g, i.e., μd, σd, μg and σg, can be estimated by using the maximum likelihood estimation approach. The 95% confidence intervals (95%CI) are calculated by using the profile likelihood estimation framework with a cutoff threshold determined using a Chi-square quantile [22].
Furthermore, the percentage of the secondary infections, denoted by η, due to pre-symptomatic transmission can be estimated by Eq (3).
η=[∫0−∞[∫δ(z)⋅h(x+z)dz]dx]×100%. | (3) |
For COVID-19, we estimate the mean of TG (μg) at 4.0 days (95%CI: 3.3−4.6) and SD (σg) at 3.6 days (95%CI: 2.6−4.5), see Figure 2. The μg estimate is consistent with mean SI at 4.0 days (95%CI: 3.5−4.4) estimated in Du et al. [16], which is also largely consistent with the mean SI estimated in [18,20,21]. The σg estimate is smaller than the SD of SI estimated at 4.8 days (95%CI: 4.5−5.1) in Du et al. [16]. The smaller SD indicates that TG may be considered as a more efficient estimator of GT than SI. From a Gamma distribution with mean 4.0 days and SD 3.6 days, we report that the TG has median at 3.0 days, interquartile range (IQR) from 1.4 to 5.5 days, 95% centile from 0.2 to 13.5 days, and 95% percentile at 11.1 days. Fixing an intrinsic growth rate of COVID-19 at 0.15 per day [23], the basic reproduction number is estimated at 1.6 (95%CI: 1.4−1.9) by using the formula in [8].
The pre-symptomatic transmission period (d) is estimated with mean (μd) 2.2 days (95%CI: 1.3−4.7) and SD (σd) 2.3 days (95%CI: 1.9−3.0), see Figure 2. From a Gamma distribution with mean 2.2 days and SD 2.3 days, we report that the d has median at 1.5 days, IQR from 0.6 to 3.0 days, 95% centile from 0.1 to 8.4 days, and 95% percentile at 6.8 days. Since the d is defined as the difference of the incubation period minus the latent period, by using the mean incubation period at 5.5 days and SD at 2.4 days estimated from previous studies on average [10,24,25,26], we approximate the mean latent period at 3.3 days.
By using Eq (3), we estimate the percentage of the secondary infections due to pre-symptomatic transmission (η) at 32.2% (95%CI: 10.3−73.7). This estimate appears largely in line with the range from 4% to 44% found in [17]. We also compare with 37% (95%CI: 28−45) in [27] and 44% (95%CI: 25−69) in [28], respectively. Although our estimate appears the lowest among the three existing estimates, we note the 95%CIs are largely aligned. On the one hand, this indicates that there will still be 32.2% of the secondary COVID-19 cases if immediate isolation is only implemented on the symptomatic cases (i.e., no quarantine for the asymptomatic close contacts). This figure increases to 72.1% if the isolation of the symptomatic cases delayed for 1 day after the symptom onset. On the other hand, if the isolation is implemented on all cases immediately after the onset of symptoms, the basic reproduction number larger than (1/32.2% = ) 3.1 is required for sustaining the COVID-19 outbreak. Given the basic reproduction number of COVID-19 is less likely to reach 3.1 [3,10,11,12,16,29,30,31], timely and effectively isolation of symptomatic cases is crucial for mitigating the outbreaks.
We further explore the difference in the reproduction number (R) calculation based on TG or SI. By using the Lotka-Euler equation [8], we formulate R = 1/M(−γ|f). The γ is the intrinsic growth rate of the epidemic curve, which can be estimated from the disease surveillance data at the early phase of the outbreak [1,10,11,23]. The function M(∙|f) represents the Laplace transform, known to statisticians as the moment generating function, of the distribution function of SI or TG, denoted by f(∙). Here, we discuss three scenarios for comparison the R calculation. Those include considering f(∙) as
● (naive scenario:) a shifted Dirac delta function with mean at 4.0 days;
● the estimated of Gamma distribution of TG with mean at 4.0 days and SD at 3.6 days; and
● the empirical distribution of SI directly from the SI data in Du et al. [16].
In Figure 3, difference in the reproduction number calculation can be observed when using different metrics to measure the interval between transmission generations. There are considerable differences among the three calculations when the γ increases, which is also found in [8] previously. Since the R is expected to be an increasing function of γ theoretically, we remark that using TG for the reproduction number calculation may be more reasonable.
The study has limitations. First, the dataset used in this analysis might be biased toward more severe cases, as pointed out in [16], 'the rapid isolation of such case-patients might have prevented longer serial intervals', which might, together with the recalling biasness, potentially lead to an underestimation of the mean TG comparing to that in a COVID-19 outbreak without control measures. Two recent studies also used public available COVID-19 surveillance data and had the sample mean of SI at 5.0 days in [27] and 5.8 days in [28], respectively, which appear higher than the sample mean of SI at 4.0 days in [16] so as in this study. Second, as a data-driven analysis, our estimates are relying on both statistical framework and quality of the SI observations. And we remark that the differences in the datasets of [16,27,28] might lead to differences in the TG estimates, which is unsurprisingly. Third, the estimation of η is conducted under the assumption that individuals are equally infectious before and after symptoms begin. In practice, the individual infectiousness is not necessarily identical, but we presume this difference is unlikely to be large. Furthermore, if the isolation date of each individual infector were available, our likelihood function, l, in Eq (2) could be extended to a right-censoring version as below.
l(Θ|x1,…,xn;τ1,…,τn)=∑ilog[f(xi|Θ)⋅Δ(τi|Θ)], |
where the τi is the day of isolation for the i-th infector since the onset of symptoms. The Δ(∙) is the cumulative distribution function (CDF) of δ(∙), and other notations are the same as in Eq (2).
In summary, we estimate the mean of TG at 4.0 days (95%CI: 3.3−4.6), and the mean of pre-symptomatic transmission period at 2.2 days (95%CI: 1.3−4.7). We estimate the basic reproduction number of 1.6 (95%CI: 1.4−1.9), and there are 32.2% (95%CI: 10.3−73.7) of the secondary infections are due to pre-symptomatic transmission. We approximate the mean latent period of 3.3 days.
All data used in this work were publicly available via the data source in Du et al. [16].
This work is not funded. The author would like to thank Dr. Zhanwei Du for sharing the data, and Drs. Daihai He and Zuyao Yang for their insightful comments and discussion.
The author declares no conflict of interest.
[1] | A. R. Tuite, D. N. Fisman, Reporting, Epidemic Growth, and Reproduction Numbers for the 2019 Novel Coronavirus (2019-nCoV) Epidemic, Ann. Intern. Med., (2020). |
[2] | S. Zhao, P. Cao, D. Gao, Z. Zhuang, Y. Cai, J. Ran, et al., Serial interval in determining the estimation of reproduction number of the novel coronavirus disease (COVID-19) during the early outbreak, J. Travel Med., (2020), taaa033. |
[3] | J. Riou, C. L. Althaus, Pattern of early human-to-human transmission of Wuhan 2019 novel coronavirus (2019-nCoV), December 2019 to January 2020, Euro. Surveill., 25 (2020), 2000058. |
[4] |
P. E. M. Fine, The Interval between Successive Cases of an Infectious Disease, Am. J. Epidemiol., 158 (2003), 1039-1047. doi: 10.1093/aje/kwg251
![]() |
[5] |
L. F. White, J. Wallinga, L. Finelli, C. Reed, S. Riley, M. Lipsitch, et al., Estimation of the reproductive number and the serial interval in early phase of the 2009 influenza A/H1N1 pandemic in the USA, Influenza Other Respir. Viruses, 3 (2009), 267-276. doi: 10.1111/j.1750-2659.2009.00106.x
![]() |
[6] | R. Milwid, A. Steriu, J. Arino, J. Heffernan, A. Hyder, D. Schanzer, et al., Toward Standardizing a Lexicon of Infectious Disease Modeling Terms, Front. Public Health, 4 (2016), 213. |
[7] |
M. A. Vink, M. C. J. Bootsma, J. Wallinga, Serial Intervals of Respiratory Infectious Diseases: A Systematic Review and Analysis, Am. J. Epidemiol., 180 (2014), 865-875. doi: 10.1093/aje/kwu209
![]() |
[8] | J. Wallinga, M. Lipsitch, How generation intervals shape the relationship between growth rates and reproductive numbers, P. Roy. Soc. B, 274 (2007), 599-604. |
[9] |
C. Huang, Y. Wang, X. Li, L. Ren, J. Zhao, Y. Hu, et al., Clinical features of patients infected with 2019 novel coronavirus in Wuhan, China, Lancet, 395 (2020), 497-506. doi: 10.1016/S0140-6736(20)30183-5
![]() |
[10] |
Q. Li, X. Guan, P. Wu, X. Wang, L. Zhou, Y. Tong, et al., Early Transmission Dynamics in Wuhan, China, of Novel Coronavirus-Infected Pneumonia, New Engl. J. Med., 382 (2020), 1199-1207. doi: 10.1056/NEJMoa2001316
![]() |
[11] |
S. Zhao, S. S. Musa, Q. Lin, J. Ran, G. Yang, W. Wang, et al., Estimating the Unreported Number of Novel Coronavirus (2019-nCoV) Cases in China in the First Half of January 2020: A Data-Driven Modelling Analysis of the Early Outbreak, J. Clin. Med., 9 (2020), 388. doi: 10.3390/jcm9020388
![]() |
[12] |
J. T. Wu, K. Leung, G. M. Leung, Nowcasting and forecasting the potential domestic and international spread of the 2019-nCoV outbreak originating in Wuhan, China: a modelling study, Lancet, 395 (2020), 689-697. doi: 10.1016/S0140-6736(20)30260-9
![]() |
[13] | S. Zhao, Z. Zhuang, P. Cao, J. Ran, D. Gao, Y. Lou, et al., Quantifying the association between domestic travel and the exportation of novel coronavirus (2019-nCoV) cases from Wuhan, China in 2020: a correlational analysis, J. Travel Med., 27 (2020), taaa022. |
[14] | World Health Organization, Statement on the second meeting of the International Health Regulations (2005) Emergency Committee regarding the outbreak of novel coronavirus (2019-nCoV), 2020. |
[15] | World Health Organization, Novel Coronavirus (2019-nCoV) situation reports, 3. 2020. |
[16] | Z. Du, X. Xu, Y. Wu, L. Wang, B. J. Cowling, L. A. Meryers, Serial Interval of COVID-19 among Publicly Reported Confirmed Cases, Emerg. Infecti. Dis., 26 (2020). |
[17] | S. Ma, J. Zhang, M. Zeng, Q. Yun, W. Guo, Y. Zheng, et al., Epidemiological parameters of coronavirus disease 2019: a pooled analysis of publicly reported individual data of 1155 cases from seven countries, medRxiv (2020), 2020.03.21.20040329. |
[18] | C. You, Y. Deng, W. Hu, J. Sun, Q. Lin, F. Zhou, et al., Estimation of the Time-Varying Reproduction Number of COVID-19 Outbreak in China, medRxiv (2020), 2020.02.08.20021253. |
[19] |
B. J. Cowling, V. J. Fang, S. Riley, J. M. Peiris, G. M. Leung, Estimation of the serial interval of influenza, Epidemiol., 20 (2009), 344. doi: 10.1097/EDE.0b013e31819d1092
![]() |
[20] |
H. Nishiura, N. M. Linton, A. R. Akhmetzhanov, Serial interval of novel coronavirus (COVID-19) infections, Int. J. Infect. Dis., 93 (2020), 284-286. doi: 10.1016/j.ijid.2020.02.060
![]() |
[21] | S. Zhao, D. Gao, Z. Zhuang, M. Chong, Y. Cai, J. Ran, et al., Estimating the serial interval of the novel coronavirus disease (COVID-19): A statistical analysis using the public data in Hong Kong from January 16 to February 15, 2020, medRxiv (2020), 2002.02.21.20026559. |
[22] | J. Fan, T. Huang, Profile likelihood inferences on semiparametric varying-coefficient partially linear models, Bernoulli, 11 (2005), 1031-1057. |
[23] |
S. Zhao, Q. Lin, J. Ran, S. S. Musa, G. Yang, W. Wang, et al., Preliminary estimation of the basic reproduction number of novel coronavirus (2019-nCoV) in China, from 2019 to 2020: A data-driven analysis in the early phase of the outbreak, Int. J. Infect. Dis., 92 (2020), 214-217. doi: 10.1016/j.ijid.2020.01.050
![]() |
[24] | J. A. Backer, D. Klinkenberg, J. Wallinga, Incubation period of 2019 novel coronavirus (2019-nCoV) infections among travellers from Wuhan, China, 20-28 January 2020, Euro. Surveill., 25 (2020), 2000062. |
[25] |
W. J. Guan, Z. Y. Ni, Y. Hu, W. H. Liang, C. Q. Ou, J. X. He, et al., Clinical Characteristics of Coronavirus Disease 2019 in China, N. Engl. J. Med., 382 (2020), 1708-1720. doi: 10.1056/NEJMoa2002032
![]() |
[26] | S. A. Lauer, K. H. Grantz, Q. Bi, F. K. Jones, Q. Zheng, H. R. Meredith, et al., The incubation period of coronavirus disease 2019 (COVID-19) from publicly reported confirmed cases: estimation and application, Ann. Intern. Med., (2020). |
[27] | L. Ferretti, C. Wymant, M. Kendall, L. Zhao, A. Nurtay, L. Abeler-Dorner, et al., Quantifying SARS-CoV-2 transmission suggests epidemic control with digital contact tracing, Science, 368 (2020), eabb6936. |
[28] | X. He, E. H. Y. Lau, P. Wu, X. Deng, J. Wang, X. Hao, et al., Temporal dynamics in viral shedding and transmissibility of COVID-19, Nat. Med., (2020), 1-4. |
[29] | Y. Liu, A. A. Gayle, A. Wilder-Smith, J. Rocklov, The reproductive number of COVID-19 is higher compared to SARS coronavirus, J. Travel. Med., 27 (2020), taaa021. |
[30] |
R. Li, S. Pei, B. Chen, Y. Song, T. Zhang, W. Yang, et al., Substantial undocumented infection facilitates the rapid dissemination of novel coronavirus (SARS-CoV2), Science, 368 (2020), 489-493. doi: 10.1126/science.abb3221
![]() |
[31] |
Z. Zhuang, S. Zhao, Q. Lin, P. Cao, Y. Lou, L. Yang, et al., Preliminary estimation of the novel coronavirus disease (COVID-19) cases in Iran: A modelling analysis based on overseas cases and air travel data, Int. J. Infect. Dis., 94 (2020), 29-31. doi: 10.1016/j.ijid.2020.03.019
![]() |
1. | Shi Zhao, To avoid the noncausal association between environmental factor and COVID-19 when using aggregated data: Simulation-based counterexamples for demonstration, 2020, 748, 00489697, 141590, 10.1016/j.scitotenv.2020.141590 | |
2. | Shi Zhao, A simple approach to estimate the instantaneous case fatality ratio: Using the publicly available COVID-19 surveillance data in Canada as an example, 2020, 5, 24680427, 575, 10.1016/j.idm.2020.08.002 | |
3. | Shi Zhao, Jingzhi Lou, Lirong Cao, Hong Zheng, Marc K. C. Chong, Zigui Chen, Benny C. Y. Zee, Paul K. S. Chan, Maggie H. Wang, Modelling the association between COVID-19 transmissibility and D614G substitution in SARS-CoV-2 spike protein: using the surveillance data in California as an example, 2021, 18, 1742-4682, 10.1186/s12976-021-00140-3 | |
4. | Robin N. Thompson, T. Déirdre Hollingsworth, Valerie Isham, Daniel Arribas-Bel, Ben Ashby, Tom Britton, Peter Challenor, Lauren H. K. Chappell, Hannah Clapham, Nik J. Cunniffe, A. Philip Dawid, Christl A. Donnelly, Rosalind M. Eggo, Sebastian Funk, Nigel Gilbert, Paul Glendinning, Julia R. Gog, William S. Hart, Hans Heesterbeek, Thomas House, Matt Keeling, István Z. Kiss, Mirjam E. Kretzschmar, Alun L. Lloyd, Emma S. McBryde, James M. McCaw, Trevelyan J. McKinley, Joel C. Miller, Martina Morris, Philip D. O'Neill, Kris V. Parag, Carl A. B. Pearson, Lorenzo Pellis, Juliet R. C. Pulliam, Joshua V. Ross, Gianpaolo Scalia Tomba, Bernard W. Silverman, Claudio J. Struchiner, Michael J. Tildesley, Pieter Trapman, Cerian R. Webb, Denis Mollison, Olivier Restif, Key questions for modelling COVID-19 exit strategies, 2020, 287, 0962-8452, 20201405, 10.1098/rspb.2020.1405 | |
5. | Gerry F. Killeen, Patricia M. Kearney, Ivan J. Perry, Niall Conroy, Long, thin transmission chains of Severe Acute Respiratory Syndrome Coronavirus 2 may go undetected for several weeks at low to moderate reproduction numbers: Implications for containment and elimination strategy, 2021, 6, 24680427, 474, 10.1016/j.idm.2021.02.002 | |
6. | Jinjun Ran, Shi Zhao, Lefei Han, Marc K.C. Chong, Yulan Qiu, Yiwei Yang, Jiayi Wang, Yushan Wu, Mohammad Javanbakht, Maggie H. Wang, Daihai He, The changing patterns of COVID-19 transmissibility during the social unrest in the United States: A nationwide ecological study with a before-and-after comparison, 2021, 12, 23527714, 100201, 10.1016/j.onehlt.2020.100201 | |
7. | Zhongxing Ding, Kai Wang, Mingwang Shen, Kai Wang, Shi Zhao, Wenyu Song, Rui Li, Zhongjie Li, Liping Wang, Ganzhu Feng, Zhiliang Hu, Hongxia Wei, Yanni Xiao, Changjun Bao, Jianli Hu, Liguo Zhu, Yong Li, Xufeng Chen, Yi Yin, Weiming Wang, Yongli Cai, Zhihang Peng, Hongbing Shen, Estimating the time interval between transmission generations and the presymptomatic period by contact tracing surveillance data from 31 provinces in the mainland of China, 2021, 1, 26673258, 104, 10.1016/j.fmre.2021.02.002 | |
8. | Chunyu Li, Shi Zhao, Biao Tang, Yuchen Zhu, Jinjun Ran, Xiujun Li, Daihai He, Estimating the Instantaneous Asymptomatic Proportion With a Simple Approach: Exemplified With the Publicly Available COVID-19 Surveillance Data in Hong Kong, 2021, 9, 2296-2565, 10.3389/fpubh.2021.604455 | |
9. | Salihu Sabiu Musa, Amna Tariq, Liu Yuan, Wei Haozhen, Daihai He, Infection fatality rate and infection attack rate of COVID-19 in South American countries, 2022, 11, 2049-9957, 10.1186/s40249-022-00961-5 | |
10. | Shi Zhao, Yu Zhao, Biao Tang, Daozhou Gao, Zihao Guo, Marc K.C. Chong, Salihu S Musa, Yongli Cai, Weiming Wang, Daihai He, Maggie H Wang, Shrinkage in serial intervals across transmission generations of COVID-19, 2021, 529, 00225193, 110861, 10.1016/j.jtbi.2021.110861 | |
11. | Lefei Han, Shi Zhao, Peihua Cao, Marc K. C. Chong, Jingxuan Wang, Daihai He, Xiaobei Deng, Jinjun Ran, How Transportation Restriction Shapes the Relationship Between Ambient Nitrogen Dioxide and COVID-19 Transmissibility: An Exploratory Analysis, 2021, 9, 2296-2565, 10.3389/fpubh.2021.697491 | |
12. | Shi Zhao, Kai Wang, Marc K.C. Chong, Salihu S. Musa, Mu He, Lefei Han, Daihai He, Maggie H. Wang, The non-pharmaceutical interventions may affect the advantage in transmission of mutated variants during epidemics: A conceptual model for COVID-19, 2022, 542, 00225193, 111105, 10.1016/j.jtbi.2022.111105 | |
13. | Hualei Xin, Jessica Y Wong, Caitriona Murphy, Amy Yeung, Sheikh Taslim Ali, Peng Wu, Benjamin J Cowling, The Incubation Period Distribution of Coronavirus Disease 2019: A Systematic Review and Meta-analysis, 2021, 73, 1058-4838, 2344, 10.1093/cid/ciab501 | |
14. | Mohammed M. Al-Shomrani, Salihu S. Musa, Abdullahi Yusuf, Unfolding the Transmission Dynamics of Monkeypox Virus: An Epidemiological Modelling Analysis, 2023, 11, 2227-7390, 1121, 10.3390/math11051121 | |
15. | Bin Deng, Weikang Liu, Zhinan Guo, Li Luo, Tianlong Yang, Jiefeng Huang, Buasiyamu Abudunaibi, Yidun Zhang, Xue Ouyang, Demeng Wang, Chenghao Su, Tianmu Chen, Natural history and cycle threshold values analysis of COVID-19 in Xiamen City, China, 2022, 7, 24680427, 486, 10.1016/j.idm.2022.07.007 | |
16. | Shi Zhao, Peihua Cao, Daozhou Gao, Zian Zhuang, Weiming Wang, Jinjun Ran, Kai Wang, Lin Yang, Mohammad R. Einollahi, Yijun Lou, Daihai He, Maggie H. Wang, Modelling COVID-19 outbreak on the Diamond Princess ship using the public surveillance data, 2022, 7, 24680427, 189, 10.1016/j.idm.2022.05.005 | |
17. | Paul Tupper, Caroline Colijn, Philip K. Maini, COVID-19 in schools: Mitigating classroom clusters in the context of variable transmission, 2021, 17, 1553-7358, e1009120, 10.1371/journal.pcbi.1009120 | |
18. | Xiujuan Tang, Salihu S. Musa, Shi Zhao, Shujiang Mei, Daihai He, Using Proper Mean Generation Intervals in Modeling of COVID-19, 2021, 9, 2296-2565, 10.3389/fpubh.2021.691262 | |
19. | Shi Zhao, Biao Tang, Salihu S Musa, Shujuan Ma, Jiayue Zhang, Minyan Zeng, Qingping Yun, Wei Guo, Yixiang Zheng, Zuyao Yang, Zhihang Peng, Marc KC Chong, Mohammad Javanbakht, Daihai He, Maggie H. Wang, Estimating the generation interval and inferring the latent period of COVID-19 from the contact tracing data, 2021, 36, 17554365, 100482, 10.1016/j.epidem.2021.100482 | |
20. | Tenglong Li, Laura F. White, Alex Perkins, Bayesian back-calculation and nowcasting for line list data during the COVID-19 pandemic, 2021, 17, 1553-7358, e1009210, 10.1371/journal.pcbi.1009210 | |
21. | Shi Zhao, Jingzhi Lou, Lirong Cao, Hong Zheng, Marc K. C. Chong, Zigui Chen, Renee W. Y. Chan, Benny C. Y. Zee, Paul K. S. Chan, Maggie H. Wang, Real-time quantification of the transmission advantage associated with a single mutation in pathogen genomes: a case study on the D614G substitution of SARS-CoV-2, 2021, 21, 1471-2334, 10.1186/s12879-021-06729-w | |
22. | Mahesh Jayaweera, Chamath Dannangoda, Dilum Dilshan, Janith Dissanayake, Hasini Perera, Jagath Manatunge, Buddhika Gunawardana, Grappling with COVID-19 by imposing and lifting non-pharmaceutical interventions in Sri Lanka: A modeling perspective, 2021, 6, 24680427, 820, 10.1016/j.idm.2021.06.004 | |
23. | Salihu Sabiu Musa, Zainab Umar Abdullahi, Shi Zhao, Umar Muhammad Bello, Nafiu Hussaini, Abdulrazaq Garba Habib, Daihai He, Transmission Dynamics of Monkeypox Virus in Nigeria during the Current COVID-19 Pandemic and Estimation of Effective Reproduction Number, 2022, 10, 2076-393X, 2153, 10.3390/vaccines10122153 | |
24. | Binod Pant, Abba B. Gumel, Mathematical assessment of the roles of age heterogeneity and vaccination on the dynamics and control of SARS-CoV-2, 2024, 9, 24680427, 828, 10.1016/j.idm.2024.04.007 | |
25. | Yeonji Seo, Okyu Kwon, Hang-Hyun Jo, Statistical properties of COVID-19 transmission intervals in Republic of Korea, 2024, 84, 0374-4884, 83, 10.1007/s40042-023-00952-1 |