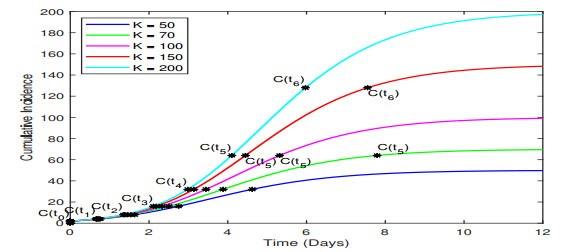
In the absence of reliable information about transmission mechanisms for emerging infectious diseases, simple phenomenological models could provide a starting point to assess the potential outcomes of unfolding public health emergencies, particularly when the epidemiological characteristics of the disease are poorly understood or subject to substantial uncertainty. In this study, we employ the modified Richards model to analyze the growth of an epidemic in terms of 1) the number of times cumulative cases double until the epidemic peaks and 2) the rate at which the intervals between consecutive doubling times increase during the early ascending stage of the outbreak. Our theoretical analysis of doubling times is combined with rigorous numerical simulations and uncertainty quantification using synthetic and real data for COVID-19 pandemic. The doubling-time approach allows to employ early epidemic data to differentiate between the most dangerous threats, which double in size many times over the intervals that are nearly invariant, and the least transmissible diseases, which double in size only a few times with doubling periods rapidly growing.
Citation: Alexandra Smirnova, Brian Pidgeon, Gerardo Chowell, Yichuan Zhao. The doubling time analysis for modified infectious disease Richards model with applications to COVID-19 pandemic[J]. Mathematical Biosciences and Engineering, 2022, 19(3): 3242-3268. doi: 10.3934/mbe.2022150
[1] | Ayako Suzuki, Hiroshi Nishiura . Transmission dynamics of varicella before, during and after the COVID-19 pandemic in Japan: a modelling study. Mathematical Biosciences and Engineering, 2022, 19(6): 5998-6012. doi: 10.3934/mbe.2022280 |
[2] | Elodie Yedomonhan, Chénangnon Frédéric Tovissodé, Romain Glèlè Kakaï . Modeling the effects of Prophylactic behaviors on the spread of SARS-CoV-2 in West Africa. Mathematical Biosciences and Engineering, 2023, 20(7): 12955-12989. doi: 10.3934/mbe.2023578 |
[3] | Tetsuro Kobayashi, Hiroshi Nishiura . Prioritizing COVID-19 vaccination. Part 2: Real-time comparison between single-dose and double-dose in Japan. Mathematical Biosciences and Engineering, 2022, 19(7): 7410-7424. doi: 10.3934/mbe.2022350 |
[4] | Lin Feng, Ziren Chen, Harold A. Lay Jr., Khaled Furati, Abdul Khaliq . Data driven time-varying SEIR-LSTM/GRU algorithms to track the spread of COVID-19. Mathematical Biosciences and Engineering, 2022, 19(9): 8935-8962. doi: 10.3934/mbe.2022415 |
[5] | Glenn Ledder . Incorporating mass vaccination into compartment models for infectious diseases. Mathematical Biosciences and Engineering, 2022, 19(9): 9457-9480. doi: 10.3934/mbe.2022440 |
[6] | Jie Bai, Xiunan Wang, Jin Wang . An epidemic-economic model for COVID-19. Mathematical Biosciences and Engineering, 2022, 19(9): 9658-9696. doi: 10.3934/mbe.2022449 |
[7] | Yukun Tan, Durward Cator III, Martial Ndeffo-Mbah, Ulisses Braga-Neto . A stochastic metapopulation state-space approach to modeling and estimating COVID-19 spread. Mathematical Biosciences and Engineering, 2021, 18(6): 7685-7710. doi: 10.3934/mbe.2021381 |
[8] | Masoud Saade, Samiran Ghosh, Malay Banerjee, Vitaly Volpert . An epidemic model with time delays determined by the infectivity and disease durations. Mathematical Biosciences and Engineering, 2023, 20(7): 12864-12888. doi: 10.3934/mbe.2023574 |
[9] | Ahmed Alshehri, Saif Ullah . A numerical study of COVID-19 epidemic model with vaccination and diffusion. Mathematical Biosciences and Engineering, 2023, 20(3): 4643-4672. doi: 10.3934/mbe.2023215 |
[10] | Sarah Treibert, Helmut Brunner, Matthias Ehrhardt . A nonstandard finite difference scheme for the SVICDR model to predict COVID-19 dynamics. Mathematical Biosciences and Engineering, 2022, 19(2): 1213-1238. doi: 10.3934/mbe.2022056 |
In the absence of reliable information about transmission mechanisms for emerging infectious diseases, simple phenomenological models could provide a starting point to assess the potential outcomes of unfolding public health emergencies, particularly when the epidemiological characteristics of the disease are poorly understood or subject to substantial uncertainty. In this study, we employ the modified Richards model to analyze the growth of an epidemic in terms of 1) the number of times cumulative cases double until the epidemic peaks and 2) the rate at which the intervals between consecutive doubling times increase during the early ascending stage of the outbreak. Our theoretical analysis of doubling times is combined with rigorous numerical simulations and uncertainty quantification using synthetic and real data for COVID-19 pandemic. The doubling-time approach allows to employ early epidemic data to differentiate between the most dangerous threats, which double in size many times over the intervals that are nearly invariant, and the least transmissible diseases, which double in size only a few times with doubling periods rapidly growing.
The modified Richards model describes epidemic dynamics in two phases of fast and slow infection spread with a transition point (also referred to as turning or inflection point), at which the maximum incidence occurs. In the slow phase of infection spread (after the turning point), the epidemic peaks and subsequently declines, while the cumulative number of cases eventually saturates. At this point, little can be done in terms of control and prevention. On the other hand, efficient mitigation measures implemented at the early ascending stage of the outbreak have the potential of significantly reducing humanitarian cost and economic impacts of the emerging disease.
In this study, we employ the modified Richards model to analyze the growth of epidemic in terms of 1) the number of times cumulative cases double until the epidemic peaks and 2) the rate at which the intervals between consecutive doubling times increase during the emerging stage of the disease. It is our expectation that the doubling-time research will become a major tool in the study of infectious disease epidemics, and potentially in understanding the underlying processes by which emerging outbreaks quantify their spread in distinct ways. Furthermore, we believe that the doubling-time metrics for the modified Richards model could be used to systematically characterize recurrent epidemics (e.g., multiple influenza seasons) or spatial heterogeneity for epidemics that can be disaggregated at finer spatial resolutions (e.g., regions, districts).
In what follows, we combine theoretical evaluation of doubling times with rigorous numerical experiments using synthetic and real epidemiological observations stemming from the well-documented COVID-19 pandemic. These observations are crucial to test, guide and improve epidemic modeling. This data driven enhancement of the modified Richards model leads to substantial improvements in our ability to capture epidemic trajectories and to a better characterization of the transmission potential of infectious diseases. Importantly, our results underscore how various underlying assumptions in epidemic models influence the doubling-time mathematical analysis and its numerical estimation. More generally, our study highlights the importance of confronting crucial modeling assumptions with real epidemic data. These assumptions undoubtedly play a key role in projecting possible outcomes of an outbreak, and estimating the intensity of intervention steps required for minimizing its impact on the population. Understanding the complete effect of parameters quantifying these assumptions and their variations on epidemic doubling times will require further methodological advancements.
We analyze the growth of epidemics as a function of the number of times the cumulative incidence doubles before the epidemic peaks, and the rate at which the doubling time increases (hence the epidemic slows down), owing to interventions, behavior changes and depletion of susceptible individuals. The most difficult to control are epidemic threats that double in size many times by the time the peak has been reached, while doubling times increase at a relatively low rate. Conversely, the least difficult to control are epidemic threats that only double in size very few times, while the sequence of doubling times rapidly increases [1]. When C′(t) represents the number of new infected cases at time t, the progression of the epidemic is often modeled by an autonomous differential equation tracking the cumulative number of infections, C(t):
dCdt=F(C). | (2.1) |
Given this equation, the doubling time, Δt, can be expressed in terms of the cumulative number of cases as follows
Δt=∫2C(t)C(t)dsF(s). | (2.2) |
Epidemics that span over a short period of time relative to the average lifetime of the host population can be routinely described by S-shaped logistic curves [2,3,4,5,6]. These differential equations rely on a small number of parameters and assume initial exponential or sub-exponential growth phase that saturates as the number of cases accumulates, a pattern that implicitly captures a gradual decrease in at-risk susceptible population. In particular, the modified Richards model with four parameters has been used to fit a range of logistic-type epidemic curves [6,7,8,9,10]. For this model,
F(s):=rsp[1−(sK)a],0<s<K, | (2.3) |
where r>0 represents the intrinsic growth rate in the absence of any limitation to disease spread, p, 0≤p≤1, is a deceleration of growth parameter, K>0 is the size of the epidemic, and a>0 is a parameter that measures the extent of deviation from the S-shaped dynamics of the classical logistic growth model. At the early stages of the epidemic, this model enables us to capture different growth profiles ranging from constant incidence (p=0), polynomial growth (0<p<1), to exponential growth (p=1). As shown in [11], the solution of the indefinite integral in (2.2) for F(s) defined in (2.3) can be expressed in terms of Gaussian Hypergeometric Function, which indicates that solution to (2.2)-(2.3) will converge under mild conditions. To derive the solution, let u:=(sK)a be a new variable. Then the doubling time, Δt, for the modified Richards model (2.1), (2.3) is given by
Δt=K1−par∫(2CK)a(CK)aduu1−1−pa(1−u)=b∫(2CK)a(CK)aduuδ(1−u), | (2.4) |
where δ:=1−1−pa and b:=K1−par. Since the cumulative number of cases cannot exceed the threshold level, K, the new variable, u, is less than 1. Thus, one can view 11−u as the sum of a geometric series and, therefore,
Δt=b∫(2CK)a(CK)a∞∑n=0un−δdu=b∞∑n=0(2CK)a(n+1−δ)−(CK)a(n+1−δ)n+1−δ. | (2.5) |
From (2.5) and the definitions of b and δ, one concludes that
Δt=K1−pr∞∑n=0(2CK)na+1−p−(CK)na+1−pna+1−p=C1−pr∞∑n=0(CK)na[2na+1−p−1]na+1−p. | (2.6) |
Consider the sequence of doubling times, {Δtj}, for the modified Richards model (2.1), (2.3) such that
Δt0:=0,2C(j∑k=0Δtk)=C(j+1∑k=0Δtk),j=0,1,2...N, | (3.1) |
where N is the finite number of times cumulative cases double until they reach half of the threshold level, K/2. Let C(0)=C0. Then clearly,
C(Δt0)=C0,C(1∑k=0Δtk)=2C0,C(2∑k=0Δtk)=22C0,...C(j∑k=0Δtk)=2jC0, | (3.2) |
and the (j+1)th element of the sequence, Δtj+1, can be evaluated as
Δtj+1=∫2j+1C02jC0dsF(s),j=0,1,2...N. | (3.3) |
For F(s) defined in (2.3), one concludes that
Δtj+1=K1−pr∞∑n=0(2j+1C0K)na+1−p−(2jC0K)na+1−pna+1−p |
=(2jC0)1−pr∞∑n=0(2jC0K)na[2na+1−p−1]na+1−p,j=0,1,2...N. | (3.4) |
Identities (3.4) yield the following breakdown for Δtj+1:
Δtj+1=(2jC0)1−p[21−p−1]r(1−p)+(2jC0)1−pr∞∑n=1(2jC0K)na[2na+1−p−1]na+1−p,j=0,1,2...N. | (3.5) |
In what follows, we consider some important special cases, for which the expression for the doubling time, Δt, admits a closed form representation. First, we explore the classical Richards model where
F(s):=rs[1−(sK)a],0<s<K, | (4.1) |
that is, a special case of (2.3) that corresponds to p=1. According to (2.4), for p=1,
Δt=1ar∫(2CK)a(CK)aduu(1−u)=ln2r+1arln{1−(CK)a1−(2CK)a}, | (4.2) |
or, alternatively, Δt=1arln[(2K)a−(2C)aKa−(2C)a]. In (4.2), the term ln2r is the reflection of the exponential growth at the early ascending stage of the outbreak. The second term, 1arln{1−(CK)a1−(2CK)a} is due to the contribution of the factor 1−(CK)a in the model, which, as opposed to a simple exponential growth, takes into account the carrying capacity of the environment. As expected, the expression for Δt implies that the doubling of cases occurs until C<K2. Taking into account (3.3) and (4.1), one arrives at the following equality for the sequence of doubling times, Δtj+1, in the case of the classical Richards model:
Δtj+1=ln2r+1arln{1−(2jC0K)a1−(2j+1C0K)a},j=0,1,2...N. | (4.3) |
Applying elementary formula ln(x)=∑∞n=1(−1)n−1(x−1)nn, 0<x≤2, with x=1−(2jC0K)a and then with x=1−(2j+1C0K)a, one gets
ln{1−(2jC0K)a1−(2j+1C0K)a}=∞∑n=1(−1)n−1(−(2jC0K)a)nn−∞∑n=1(−1)n−1(−(2j+1C0K)a)nn |
=∞∑n=1(2jC0K)na[2na−1]n,j=0,1,2...N. | (4.4) |
Thus, for the classical Richards model (2.1), (4.1), one obtains
Δtj+1=ln2r+1ar∞∑n=1(2jC0K)na[2na−1]n,j=0,1,2...N. | (4.5) |
Comparing identities (3.5) and (4.5), one can easily notice that the second term in (4.5) coincides with the second term in (3.5) when p=1. At the same time, the first term in (4.5), ln2r, is equal to limp→1(2jC0)1−p[21−p−1]r(1−p). In other words, it is the limit (as p approaches 1) of the first term in (3.5).
Another special case that also gives rise to a Bernoulli differential equation is the one where
F(s):=rsp[1−(sK)1−p],0<s<K. | (4.6) |
For F(s) defined in (4.6) and 0<p<1, one obtains a wide spectrum of early growth rates. At the same time, just like in the case of the classical Richards model, the doubling time here is a simple elementary function. From (2.4) one has
Δt=b∫(2CK)a(CK)adu1−u=K1−p(1−p)rln{1−(CK)1−p1−(2CK)1−p}, | (4.7) |
and, therefore,
Δtj+1=K1−p(1−p)rln{1−(2jC0K)1−p1−(2j+1C0K)1−p},j=0,1,2...N. | (4.8) |
We now consider the generalized-growth model, that is, a→∞ in (2.3), which results in
F(s)=rsp,s>0. | (4.9) |
Similar to (2.3) and (4.6), the generalized-growth model enables us to capture the diversity of epidemic growth profiles during the first few generations of disease transmission, including constant (p=0), polynomial (0<p<1), and exponential (p=1) growth rates. However, as opposed to (2.3), the doubling time for (4.9) admits the closed form expression for any 0≤p≤1. Indeed, according to (2.2), in the case of sub-exponential growth, i.e., when 0<p<1, for F(s) defined in (4.9) one concludes
Δt=∫2CCdsrsp=1r(1−p)[(2C(t))1−p−(C(t))1−p]=C1−p(t)[21−p−1]r(1−p). | (4.10) |
Thus, for an emerging outbreak, in the absence of reliable information about transmission mechanisms, the generalized-growth model could provide a starting point for the assessment of the potential outcomes, particularly when the epidemiological characteristics of the disease are poorly understood or subject to substantial uncertainty. This implies that the sequence of doubling times, {Δtj}, increases according to
Δtj+1=(2jC0)1−p[21−p−1]r(1−p),Δt0=0,j=0,1,2..., | (4.11) |
which is precisely the first term in (3.5). As mentioned before, limp→1(2jC0)1−p[21−p−1]r(1−p)=ln2r, the doubling time for the simple exponential growth, C′=rC.
In this section, we continue to explore the progression of doubling times for the classical Richards model
dCdt=rC[1−(CK)a]. | (5.1) |
The special S-shaped logistic curve, given by (5.1), is described by the following equation:
C(t)=K{1+[(KC0)a−1]e−art}1a. | (5.2) |
Substituting this expression for C(t) into (4.2), one arrives at
Δt=1arln{[(2KC0)a−2a]e−art+1−2a[(KC0)a−1]e−art}=ln2r−1arln{1−(2a−1)Ca0eartKa−Ca0}. | (5.3) |
This identity reinforces the fact that cumulative cases would double unless C0≥K2 (and this is unlikely for most outbreaks). The cumulative cases will continue to double until the moment
ˆt=1arlnμ,μ:=Ka−Ca0(2a−1)Ca0, | (5.4) |
that is, until the moment C(t) reaches the value K/2. At that same moment, the argument of the logarithm in (5.3) becomes negative. As t approaches ˆt=1arlnμ (and C(t) approaches K/2), the term 1arln{1−(2a−1)Ca0eartKa−Ca0} in (5.3) goes to negative infinity, which means the doubling time approaches infinity.
For comparison, the doubling time for the generalized-growth model, C′=rCp, (as a function of time passed from the start of the outbreak) takes the form
Δt=(21−p−1){t+C1−p0(1−p)r},C(0)=C0. | (5.5) |
Obviously, limp→1(21−p−1){t+C1−p0(1−p)r}=ln2r, which is the doubling time for C′=rC.
We now investigate how the doubling time depends on various values of the parameters r, K, and a for classical Richards model (4.1). In the first numerical example, we set C0=2, r=1.2, and a=0.5. Our goal is to visualize how various values of K alter the doubling times and the sequence of doubling times. From a theoretical standpoint (see (4.2)), the doubling time must be decreasing with respect to K. The numerical experiment confirms this theoretical finding as shown in Figures 1 and 4. As expected, the number of times cumulative cases double increases as the value of K goes up.
In the second numerical example, we set C0=2, r=1.2, and K=100. Here the goal is to observe how various values of a alter the doubling times and the sequence of doubling times. Again, from a theoretical standpoint (see (4.2)), the doubling time decreases with respect to a. Thus, as a increases, the doubling of cases occurs more quickly and it takes less time for the cumulative cases to approach the carrying capacity as shown in Figures 2 and 5.
In the third example, we fix C0=2, a=0.5, and K=100 and we study the dependence of doubling times on the parameter r. Numerical simulations illustrate that the dependence is very similar to the one of a, that is, the doubling time decreases as r goes up and, as r increases, the cumulative cases double more quickly and it takes less time to reach the threshold (Figures 3 and 6).
Here our goal is to compare the sequences of doubling times across various models. In the first experiment, we examine how the sequences of doubling times compare for Richards model and the modified Richards model. In the case of both models, we set a=0.5, r=1.2, C0=1, and K=100, and our goal is to see how the sequence of doubling times for the modified Richards model behaves as p approaches 1. To that end, we look at the sequence of doubling times for p=0.5,0.7,0.8,0.9, and 0.95. In line with our theoretical study, as p approaches 1, the sequence of doubling times for the modified Richards model approaches that of the Richards model as illustrated in Figure 7.
In the second experiment, we compare the generalized growth model to the modified Richards model. Clearly, the modified Richards model becomes the generalized growth model as a→∞. In order to simulate this theoretical result, for both models we set K=100, r=1.2, p=0.5, and C0=1, and the modified Richards model is considered for a=0.5,0.9,1,1.5, and 2.5. From Figure 8, one can see that as a increases, the sequences of doubling times for the modified Richards model approaches that of the generalized growth model.
In the third numerical example, we compare the sequence of doubling times for the generalized growth model and the simple exponential model. For this numerical example, we set r=1.2 and C0=1, and then the generalized growth model is studied for p=0.5,0.7,0.8,0.9, and 0.95. Figure 9 confirms that as p approaches 1, the sequence of doubling times of the generalized growth model approaches that of the exponential model.
Our goal here is to estimate unknown parameters r, a, p, and K for the modified Richards model using least squares minimization, specifically through the lsqcurvefit algorithm in MATLAB. The parameters are extracted from early wave of the outbreak. Then the doubling times are calculated from these extracted parameters.
We start by using synthetic data in order to assess if three methods for uncertainty quantification, Normal Approximation, Empirical Likelihood, and Jackknife Empirical Likelihood (see Appendix for details), employed to calculate 95% confidence intervals (CIs) for the mean doubling time, will give rise to the CIs that contain true doubling times' values. Note that the Normal Approximation (NA) method provides a formulaic representation for calculating confidence intervals, and the calculation of confidence intervals is fast. On the flip side, NA method relies on the use of the normal family of distributions which can be applied when the sample size is large (typically when n>30). In the case of limited emerging data, the method likely won't be applicable for accurate uncertainty quantification since the normal distribution may not be appropriate especially if the emerging incidence data is heavily skewed. Empirical Likelihood (EL) method doesn't require the use of this assumption and is more robust against non-normality and skewed data sets. Inferences are done using only the empirical data. The EL method can be easily implemented in most statistical software. However, if the sample size is small, the EL algorithm may diverge or fail to produce accurate confidence intervals depending on the variability of the data. And as the sample size increases, the computational burden increases over the NA method.
The Jackknife Empirical Likelihood (JEL) method makes no use of the underlying distribution of data, and inferences are done using only the empirical data. It applies to both normal and skewed distributions. But, unlike the EL method, the JEL performs well with both small and large samples. Unfortunately, the computational cost of JEL is greater than the cost of EL and NA methods. The results take longer to obtain. So, having consistent results with all three algorithms, allows to confirm that the CIs are reliable regardless of whether the incidence data is normal or heavily skewed and whether the sample size is large or limited.
To quantify uncertainty in the reconstructed doubling times' values, we conduct two numerical experiments. In the first experiment, we set
r=1.2,K=600,p=1,a=0.15. |
These parameters are used to solve the forward ODE problem and to generate cumulative data on a given time interval [t1,tn]. Afterwards, random Gaussian noise (with 0 mean and standard deviation equal to 1) is added to the generated cumulative curve to get "real" epidemic data. Then MATLAB lsqcurvefit is employed to fit the model and to extract estimated parameters a, p, K, and r. Since incidence data is known to be positive in a real-life setting, we use uniform noise in the case where the incidence becomes negative.
r | K | p | a |
1.1 (0.81, 1.4) | 620 (560,650) | 0.96 (0.77, 1.3) | 0.3 (0.13, 0.96) |
We perform uncertainty quantification for the extracted parameters by assuming Poisson error structure. A total of 400 bootstrap iterations are carried out and the extracted parameters are used to calculate the sequence of doubling times for all 400 bootstrap iterations. Displayed in Figure 10 are the graphs containing the reconstructed cumulative incidence curves with noisy data and the extracted parameters a, p, K, and r (see also Table 1). Table 4 (see Appendix) shows the true moments when the doubling of time occurs given the original values of a, p, K, and r, and the 95% confidence intervals obtained by Normal Approximation, Empirical Likelihood, and Jackknife Empirical Likelihood methods (see Appendix for details). In Table 5 (see Appendix), the true intervals of time between consecutive doublings of cases and the corresponding CIs (estimated by using the Normal Approximation, Empirical Likelihood, and Jackknife Empirical Likelihood methods) are presented. Figure 11 shows the comparison of true time when the doubling occurs with reconstructed time (left) and the comparison of true intervals of time between doubling of cases with reconstructed intervals of time (right).
One can clearly see that in the first numerical simulation, the 95% confidence intervals, obtained from the parameter estimation inverse problem, correctly capture the true parameters of the modified Richards model (see Table 1) as well as the true doubling times (Tables 4 and 5). Looking at the constructed intervals, the Normal Approximation method tends to produce the widest intervals.
r | K | p | a |
2.9 (1.9, 4.2) | 1000 (9500, 11000) | 0.47 (0.31, 0.55) | 0.25 (0.15, 0.70) |
The Empirical Likelihood and Jackknife Empirical Likelihood methods produce narrower intervals. Among the last two, the Jackknife Empirical likelihood produces narrower intervals which is to be expected. In the second experiment, we set the following original values
r=2.2,K=1000,p=0.5,a=0.25. |
The simulations are repeated using the three uncertainty quantification methods described above, and the results are displayed in Figures 12 and 13 and in Tables 2, 6, and 7 (see Appendix for table results).
In the second numerical simulation, the 95% confidence intervals accurately capture the true parameters and the true doubling times for the modified Richards model. The Empirical Likelihood and Jackknife Empirical Likelihood methods both produced narrower intervals over the NA method but from a practical standpoint, they're both roughly the same up to a certain decimal point as was seen in our simulation studies. Since narrower intervals tend to be more precise, the JEL method overall outperformed the NA and EL methods.
In the following examples, we look to estimate the doubling times using real data for the states of New Hampshire and Hawaii.
Assume that a wave of an outbreak of COVID-19 originates on day t1 and ends on day b (which is unknown at the early stage of disease transmission). Let cumulative data, dδ, be reported at t=t1<t2<...<tn with tn being smaller than b. In that case, given limited data, dδ, at the start of a new wave one has to solve the following constrained minimization problem
minr,p,a,K12‖(C−dδ)‖22=12n∑i=1(C(ti)−dδ(ti)) | (9.1) |
To solve this minimization problem in a stable manner, we use the MATLAB built-in function lsqcurvefit. This function solves the nonlinear curve fitting problem using Levenberg - Marquardt optimization algorithm, which allows to extract the system parameters of r, a, K, and p that best model the early wave of the outbreak as illustrated in Table 7. After p, K, a, and r have been recovered from the early epidemic data, 400 additional cumulative bootstrap curves are generated by adding Poisson error structure to the series of reported cases in order to quantify uncertainty in the reconstructed parameters.
In Figures 15 and 18, we show the reconstructed cumulative incidence for the two states with real cumulative incidence data for comparison (along with the histograms of the obtained values of those parameters along with their 95% confidence intervals). We also calculate the doubling times for the extracted values of the parameters r, a, K, and p. Figures 16 and 19 include the moments of time the doubling occurs and the intervals of time between consecutive doubling of cases. Expression (3.5) and the extracted parameter values are used to calculate the sequence of doubling times until cumulative cases stop doubling.
In the first example, we show the results obtained for the state of New Hampshire between March 2 to August 25, 2020 (See Figures 14–16), and in the second example, we present the results obtained for Hawaii from March 5 to May 28, 2020 (Figures 17–19) [12].
To quantify uncertainty in the extracted doubling times, we refit the model to M=400 additional data sets for cumulative cases (assuming Poisson error structure). This yields 400 sequences of doubling times for each state. Tables 8–11 display the Normal Approximation, Empirical Likelihood, and Jackknife Empirical Likelihood methods used to construct the 95% confidence intervals for the estimated doubling times (see Appendix).
State | r | K | p | a |
New Hampshire | 0.57 (0.52, 0.63) | 7200 (7000, 7300) | 0.86 (0.84, 0.88) | 0.17 (0.13, 0.2) |
Hawaii | 0.71 (0.54, 0.91) | 640 (590,690) | 1.1 (1, 1.1) | 0.094 (0.069, 0.13) |
The constructed 95% confidence intervals for the sequences of doubling times for the states of New Hampshire and Hawaii are displayed in Tables 8–11 (see Appendix). These tables show the resulting confidence intervals for the doubling times of cumulative cases during the ascending phase of the first wave of the outbreak as well as the times between consecutive doubling of cases during the same phase. By looking at the CIs, we can see that the intervals for doubling times are very narrow which is not surprising because the intervals constructed for our recovered parameters are also quite narrow. Thus, the recovered doubling times will be less variable as the iterative process is carried out. In addition, the NA confidence intervals are generally wider than the non-parametric EL and JEL methods which is consistent with the simulation studies conducted above for synthetic data. The non-parametric tests make no assumptions about the distribution of the doubling times at each time point so they are constructed from the data only which is important when we cannot make any claims about the distribution. Therefore they are considered more robust due to their reliance on fewer assumptions. As such, the JEL confidence intervals may be the most applicable to our purposes since they do not violate any major assumptions about the population distribution and they are generally the narrowest among all the intervals constructed.
In this paper, we derived theoretical formulas for calculating the doubling times for the modified Richards model and important special cases. Using these theoretical calculations, we analyzed the growth of epidemics in terms of the number of times cumulative cases double until the epidemic peaks and the rate at which intervals between consecutive doubling times change. We explored the relationships between the doubling times of the modified Richards model, the exponential growth model, the generalized growth model, and the classical Richards model.
The derived formulas have been investigated numerically using, first, synthetic data sets with predetermined values of the parameters governing the modified Richards model to assess model accuracy and validate our use of various uncertainty quantification methods. We employed three methods to quantify uncertainty: Normal Approximation, Empirical Likelihood, and Jackknife Empirical Likelihood. Normal Approximation tended to always produce the widest intervals due to the assumed structure of the probability distribution underlying the doubling times at each moment cumulative cases double. Empirical Likelihood and Jackknife Empirical Likelihood produced narrower intervals and are generally considered more precise due to this property. The Normal approximation method can be calculated quickly using common statistical functions built into R. Empirical Likelihood and Jackknife Empirical Likelihood computationally take a bit longer to construct as there are no built in functions to calculate them quickly. Nevertheless, due to their non-parametric nature, whenever the calculations are entirely based on the data, we recommend using Jackknife Empirical Likelihood since the constructed intervals are the most precise, and in practice have better small sample performance.
In the future, we would like to try other forms of Empirical Likelihood methods to assess their accuracy and to see how they perform in determining the true doubling time for each time point. Another important topic that needs to be studied next is the extension of the modified Richards model to the case of elaborate epidemic trajectories aggregating multiple asynchronous sub-epidemics, since a large number of COVID-19 incidence data curves do not have a simple bell-shape behavior for each phase of the outbreak [13,14].
This study is supported by NSF awards 1818886, 2011622, 1610429, and 1633381, by NIH grant R01 GM 130900, and by Simons Foundation grant 638679.
The authors declare that there is no conflict of interest.
All epidemic models considered above are nested in the generalized logistic model [5] defined as
dCdt=F(C),F(s):=rsp[1−(sK)a]γ,0<s<K, | (A.1) |
where r>0 represents the intrinsic growth rate in the absence of any limitation to disease spread, 0≤p≤1, is a "deceleration of growth" parameter, K>0 is the size of the epidemic, a>0 is a parameter that measures the extent of deviation from the S-shaped dynamics of the classical logistic growth model, and γ>0 controls the flexibility of the inflection point. Just like the modified Richards model, the generalized logistic model (A.1) enables us to capture different growth profiles ranging from constant incidence (p=0), polynomial growth (0<p<1), to exponential growth (p=1) at the early accenting stage of the outbreak. While it is unclear if the addition of extra parameter, γ, gives any considerable advantage from biological standpoint, mathematically the study of doubling times can easily be extended to the generalized logistic model (A.1). Indeed, using the same substitute, u:=(sK)a, for the doubling time, Δt, one obtains
Δt=K1−par∫(2CK)a(CK)aduu1−1−pa(1−u)γ=b∫(2CK)a(CK)aduuδ(1−u)γ, | (A.2) |
where δ:=1−1−pa and b:=K1−par. Given that
1(1−u)γ=∞∑n=0γ(γ+1)(γ+2)...(γ+n−1)n!un=∞∑n=0Γ(n+γ)Γ(γ)n!un, |
one arrives at the following expression for the doubling times
Δt=b∫(2CK)a(CK)a∞∑n=0Γ(n+γ)Γ(γ)n!un−δdu=bΓ(γ)∞∑n=0Γ(n+γ)[(2CK)a(n+1−δ)−(CK)a(n+1−δ)]n!(n+1−δ). | (A.3) |
In the above, Γ(γ+1):=∫∞0e−ttγdt is the gamma function. From (A.3) and the definitions of b and δ, one concludes that
Δt=K1−prΓ(γ)∞∑n=0Γ(n+γ)[(2CK)na+1−p−(CK)na+1−p]n!(na+1−p)=C1−prΓ(γ)∞∑n=0Γ(n+γ)(CK)na[2na+1−p−1]n!(na+1−p). |
This brings about the doubling times sequence in the form:
Δtj+1=(2jC0)1−prΓ(γ)∞∑n=0Γ(n+γ)(2jC0K)na[2na+1−p−1]n!(na+1−p),j=0,1,2...N. | (A.4) |
In practice, in order to estimate the doubling times of an outbreak, one has to extract the unknown parameters by fitting model predictions to the epidemic data. Using these extracted parameters, one employs (2.6) to calculate the sequence of doubling times until cumulative cases cannot double anymore.
True Moment of time cumulative cases double | n | NA | EL | JEL |
0.969 | 1 | (0.957748, 0.977638) | (0.958551, 0.976556) | (0.958579, 0.976554) |
2.016 | 2 | (1.992155, 2.028633) | (1.993715, 2.026684) | (1.993718, 2.026680) |
3.165 | 3 | (3.129453, 3.179136) | (3.131634, 3.176540) | (3.131644, 3.176530) |
4.455 | 4 | (4.414135, 4.473760) | (4.416851, 4.470673) | (4.416836, 4.470694) |
5.946 | 5 | (5.905395, 5.972138) | (5.908445, 5.968766) | (5.908472, 5.968749) |
7.752 | 6 | (7.714451, 7.786448) | (7.717759, 7.782801) | (7.717767, 7.782793) |
10.113 | 7 | (10.074112, 10.151846) | (10.077642, 10.147870) | (10.077629, 10.147860) |
13.719 | 8 | (13.673559, 13.768391) | (13.677840, 13.763624) | (13.677850, 13.763615) |
23.350 | 9 | (23.162364, 23.534539) | (23.184927, 23.522837) | (23.184957, 23.522801) |
In order to quantify uncertainty in the extracted doubling times, we refit the model to M=400 additional data sets for cumulative cases (assuming Poisson error structure), which results in M approximate sequences of doubling times. Our goal is to construct 95% confidence intervals for the mean doubling time at every moment where cases double, i.e., such confidence intervals where the true doubling time falls within the interval 95% of the time. Define the true doubling time at each point where cases double as μdouble. We calculate and compare three different confidence intervals for estimating μdouble: Normal Approximation, Empirical Likelihood, and Jackknife Empirical Likelihood.
True Interval of time Between Consecutive Doubling | n | NA | EL | JEL |
0.969 | 1 | (0.957748, 0.977638) | (0.958551, 0.976556) | (0.958579, 0.976554) |
1.047 | 2 | (1.034359, 1.051043) | (1.035081, 1.050180) | (1.035091, 1.050165) |
1.149 | 3 | (1.137063, 1.150738) | (1.137697, 1.150032) | (1.137689, 1.150040) |
1.290 | 4 | (1.283906, 1.295400) | (1.284455, 1.294837) | (1.284426, 1.294810) |
1.491 | 5 | (1.489251, 1.500387) | (1.489739, 1.499857) | (1.489765, 1.499835) |
1.806 | 6 | (1.805016, 1.818351) | (1.805718, 1.817770) | (1.805707, 1.817778) |
2.361 | 7 | (2.352960, 2.372099) | (2.353951, 2.371263) | (2.353953, 2.371261) |
3.606 | 8 | (3.589092, 3.626900) | (3.590984, 3.625175) | (3.590991, 3.625160) |
9.631 | 9 | (9.474994, 9.779960) | (9.494552, 9.771645) | (9.494577, 9.771616) |
True Moment of time cumulative cases doubles | n | NA | EL | JEL |
0.468 | 1 | (0.462279, 0.476864) | (0.463066, 0.476257) | (0.463062, 0.476260) |
1.162 | 2 | (1.138896, 1.172211) | (1.140686, 1.170805) | (1.140680, 1.170821) |
2.202 | 3 | (2.177769, 2.234873) | (2.180796, 2.232448) | (2.180820, 2.232471) |
3.788 | 4 | (3.746427, 3.833379) | (3.751046, 3.829665) | (3.751051, 3.829685) |
6.261 | 5 | (6.189960, 6.313948) | (6.196485, 6.308599) | (6.196486, 6.308594) |
10.244 | 6 | (10.139300, 10.309129) | (10.148049, 10.301598) | (10.148059, 10.301585) |
16.992 | 7 | (16.853192, 17.082037) | (16.864593, 17.071438) | (16.864613, 17.071418) |
29.502 | 8 | (29.323407, 29.646882) | (29.339147, 29.631349) | (29.339174, 29.631322) |
57.856 | 9 | (57.507775, 58.133745) | (57.540453, 58.106540) | (57.540501, 58.106465) |
For the Normal Approximation method [15,16], which is based on the Central Limit Theorem, with M=400, we have that the distribution of the mean doubling time at each point is approximately normal. Let X1,...,XM be an i.i.d random sample of doubling times at each moment cumulative cases double. Let ˉxdouble be the sample mean of X1,...,XM at each moment cumulative cases double. Therefore, we calculate 95% confidence intervals for the mean doubling time, μdouble, by adding and subtracting 1.96 standard errors from the mean. In other words, our 95% confidence intervals for the mean doubling time is calculated by:
(ˉxdouble−1.96√var(ˉxdouble),ˉxdouble+1.96√var(ˉxdouble)) | (B.1) |
Empirical Likelihood is a non-parametric method for constructing confidence intervals without having to assume the form of the underlying distribution introduced by Owen [17,18]. Empirical likelihood enables us to successfully incorporate the advantages of the likelihood methods. Unnecessary assumption of a family of distributions can be avoided in empirical likelihood inference in the sense that the object is driven by data.
True Interval of time Between Consecutive Doubling | n | NA | EL | JEL |
0.468 | 1 | (0.462279, 0.476864) | (0.463066, 0.476257) | (0.463062, 0.476260) |
0.694 | 2 | (0.676606, 0.695358) | (0.677604, 0.694572) | (0.677607, 0.694570) |
1.041 | 3 | (1.038819, 1.062716) | (1.040080, 1.061703) | (1.040086, 1.061696) |
1.586 | 4 | (1.568465, 1.598699) | (1.570054, 1.597358) | (1.570040, 1.597375) |
2.473 | 5 | (2.442930, 2.481171) | (2.444849, 2.479447) | (2.444861, 2.479426) |
3.982 | 6 | (3.947514, 3.997008) | (3.949911, 3.994645) | (3.949921, 3.994635) |
6.748 | 7 | (6.708214, 6.778586) | (6.711649, 6.775220) | (6.711656, 6.775212) |
12.510 | 8 | (12.452887, 12.582173) | (12.459753, 12.576669) | (12.459761, 12.576658) |
28.354 | 9 | (28.143750, 28.527481) | (28.165397, 28.513089) | (28.165422, 28.513042) |
n | NA | EL | JEL |
1 | (1.296990, 1.325518) | (1.298529, 1.324277) | (1.298505, 1.324302) |
2 | (2.900348, 2.956805) | (2.903265, 2.954363) | (2.903280, 2.954331) |
3 | (4.884038, 4.966410) | (4.888216, 4.962692) | (4.888211, 4.962693) |
4 | (7.340890, 7.445314) | (7.346032, 7.440461) | (7.346034, 7.440458) |
5 | (10.388441, 10.508826) | (10.394176, 10.503061) | (10.394188, 10.503050) |
6 | (14.177891, 14.305781) | (14.183782, 14.299462) | (14.183791, 14.299455) |
7 | (18.909441, 19.034630) | (18.915030, 19.028286) | (18.915037, 19.028282) |
8 | (24.862485, 24.975516) | (24.867473, 24.969776) | (24.867484, 24.969766) |
9 | (32.465413, 32.564050) | (32.469979, 32.559271) | (32.469989, 32.559260) |
10 | (42.492654, 42.589418) | (42.497416, 42.584906) | (42.497426, 42.584896) |
11 | (56.776063, 56.886604) | (56.781603, 56.881411) | (56.781586, 6.881409) |
12 | (82.431949, 82.618957) | (82.441320, 82.610516) | (82.441336, 82.610500) |
Empirical Likelihood has become very popular in recent years. Huang and Zhao [19] used empirical likelihood inference on a bivariate survival function under univariate censoring. Cheng et al. [20] introduced empirical likelihood inference for semi-parametric additive isotonic regression. In addition, Zhang et. al. [21] used empirical likelihood for testing the symmetry of statistical distributions. Let X1,...,XM be an i.i.d. random sample of doubling times from an unknown distribution function F(x)=P(X≤x). For notation, define F(x−)=P(X<x). Thus, P(X=x)=F(x)−F(x−). For simplicity, we denote P(X=Xi)=pi, i=1,...,M, where pi≥0 and ∑Mi=1pi=1. The empirical cumulative distribution function (ECDF) of X1,...,XM is
Fn(x)=1nM∑i=11Xi≤x. |
The notation 1Xi≤x outputs a value of 1 if Xi≤x is true, and 0 otherwise. In addition, given X1,...,XM, which are assumed independent with common CDF F0, the non-parametric likelihood of the CDF F is
L(F)=M∏i=1(F(Xi)−F(Xi−))=M∏i=1pi. | (B.2) |
The empirical maximum likelihood estimator (EMLE) based on the empirical likelihood, puts equal probability mass 1/M on the M observed values X1,X2,...,XM. This allows us to calculate the empirical likelihood ratio. Using Lagrange multipliers, we can show that the pi's which maximize L(F)=∏Mi=1pi subject to pi≥0, ∑Mi=1piXi=μdouble, and ∑Mi=1pi=1 are given by:
pi(μdouble)=M−1(1+λ(Xi−μdouble))−1,i=1,...,M. | (B.3) |
The empirical likelihood ratio is therefore:
R(F)=L(F)L(Fn)=∏Mi=1pi(1/M)M=M∏i=1Mpi=M∏i=1(1+λ(Xi−μdouble))−1, | (B.4) |
where λ is the unique solution of
M∑i=1Xi−μdouble1+λ(Xi−μdouble)=0. |
Let E(X21)<∞. Then, the empirical loglikelihood ratio for the true mean doubling time is
l(μdouble)=−2log(R(F))=−2M∑i=1log(Mpi)=2M∑i=1log(1+λ(Xi−μdouble)). | (B.5) |
Lastly, let μ0 be the true doubling time at a certain moment in time. We have as n→∞
−2log(l(μ0))D→χ21. |
According to Owen [18], the 100(1−α)% confidence interval for μdouble is given by
{μdouble:l(μdouble)≤χ21,1−α}, | (B.6) |
where χ21,1−α is the 1−α quantile of the χ21 distribution.
There are some computational challenges for the application of empirical likelihood methods in practice. To avoid these, Jing et al. [22] introduced the "jackknife", as a kind of re-sample method, and combined it with empirical likelihood and called it the Jackknife Empirical Likelihood (JEL). This method can greatly simplify the optimization procedures of traditional empirical likelihood methods. Various research articles have been published using jackknife empirical likelihood. Zhao et al. [23] used JEL inference for the mean absolute deviation. Sang et al. [24] proposed JEL method for estimating Gini correlations. Lin et al. [25] published the method for the error variance using JEL in linear regression models. In addition, the JEL method can also be applied to Bayesian inference, which was published by Cheng and Zhao [26].
n | NA | EL | JEL |
1 | (1.296990, 1.325518) | (1.298529, 1.324277) | (1.298505, 1.324302) |
2 | (1.603345, 1.631299) | (1.604792, 1.630013) | (1.604765, 1.630041) |
3 | (1.983635, 2.009660) | (1.984865, 2.008437) | (1.984885, 2.008417) |
4 | (2.456660, 2.479097) | (2.457661, .477939) | (2.457663, 2.477955) |
5 | (3.046878, 3.064184) | (3.047593, 3.063270) | (3.047599, 3.063260) |
6 | (3.786726, 3.799679) | (3.787323, 3.799023) | (3.787311, 3.799037) |
7 | (4.721794, 4.738605) | (4.722481, 4.737708) | (4.722489, 4.737693) |
8 | (5.932581, 5.961348) | (5.933821, 5.959773) | (5.933798, 5.959794) |
9 | (7.574728, 7.616734) | (7.576687, 7.614647) | (7.576684, 7.614652) |
10 | (10.001532, 10.051077) | (10.004001, 10.048830) | (10.004011, 10.048820) |
11 | (14.265515, 14.315081) | (14.267936, 14.312761) | (14.267946, 14.312752) |
12 | (25.628555, 25.759683) | (25.635179, 25.753758) | (25.635190, 25.753718) |
n | NA | EL | JEL |
1 | (2.198740, 2.226115) | (2.200083, 2.224878) | (2.200113, 2.224848) |
2 | (4.465612, 4.515581) | (4.468100, 4.513272) | (4.468110, 4.513261) |
3 | (6.841237, 6.909199) | (6.844618, 6.906041) | (6.844624, 6.906035) |
4 | (9.385318, 9.466835) | (9.389377, 9.463027) | (9.389371, 9.463032) |
5 | (12.192842, 12.283751) | (12.197337, 12.279508) | (12.197345, 12.279500) |
6 | (15.431831, 15.528894) | (15.436600, 15.524349) | (15.436610, 15.524339) |
7 | (19.450330, 19.554213) | (19.455392, 19.549313) | (19.455394, 19.549311) |
8 | (25.200992, 25.331885) | (25.207450, 25.325901) | (25.207461, 25.325874) |
9 | (38.314156, 38.720210) | (38.338153, 38.706650) | (38.338161, 38.706624) |
Let ˆxi,double denote the mean doubling time calculated with the ith observation deleted where i=1,..,M. We define ˉxdouble as the mean doubling time of the entire i.i.d random sample X1,...,XM. Suppose that ˆVi denotes the jackknife pseudo-value, which is obtained by
ˆVi=Mˉxdouble−(M−1)ˆxi,double,i=1,...,M. | (B.7) |
Thus, the jackknife empirical likelihood evaluated at μdouble can be defined as
J(μdouble)=M∏i=1(1+λ(ˆVi−μdouble))−1, | (B.8) |
where λ is the solution of the following equation
M∑i=1ˆVi−μdouble1+λ(ˆVi−μdouble)=0. | (B.9) |
With λ, we can now calculate the −2log of the empirical likelihood ratio as
−2log(J(μdouble))=2M∑i−1log(1+λ(ˆVi−μdouble)). |
Let E(X21)<∞. Then, by Jing et al. by [22] the empirical loglikelihood ratio for the true mean doubling time is
−2log(J(μdouble))=2M∑i=1log(1+λ(ˆVi−μdouble)). | (B.10) |
Lastly, let μ0 be the true doubling time at a certain moment in time. We have as n→∞
−2logJ(μ0)D→χ21. |
Thus, the Jackknife Empirical Likelihood confidence interval for μdouble is obtained by
{μdouble:−2log(J(μdouble))≤χ21,1−α}, | (B.11) |
where χ21,1−α is the 1−α quantile of χ21.
n | NA | EL | JEL |
1 | (2.198740, 2.226115) | (2.200083, 2.224878) | (2.200113, 2.224848) |
2 | (2.266848, 2.289491) | (2.267973, 2.288438) | (2.267975, 2.288435) |
3 | (2.375497, 2.393745) | (2.376394, 2.392893) | (2.376398, 2.392889) |
4 | (2.543588, 2.558130) | (2.544302, 2.557439) | (2.544298, 2.557443) |
5 | (2.805798, 2.818642) | (2.806437, 2.818023) | (2.806423, 2.818036) |
6 | (3.234070, 3.250063) | (3.234861, 3.249375) | (3.234882, 3.249360) |
7 | (4.008355, 4.035462) | (4.009760, 4.034378) | (4.009787, 4.034351) |
8 | (5.735666, 5.792668) | (5.738761, 5.790458) | (5.738777, 5.790478) |
9 | (13.099598, 13.401891) | (13.118536, 13.393273) | (13.118561, 13.393238) |
[1] |
A. Smirnova, L. DeCamp, G. Chowell, Mathematical and statistical analysis of doubling times to investigate the early spread of epidemics: Application to the COVID-19 pandemic, Mathematics, 9 (2021), 625. https://doi.org/10.3390/math9060625 doi: 10.3390/math9060625
![]() |
[2] | G. Chowell, L. Simonsen, C. Viboud, Y. Kuang, Is west Africa approaching a catastrophic phase or is the 2014 Ebola epidemic slowing down? Different models yield different answers for Liberia, PLoS Curr., 6 (2014). https://doi.org/10.1371/currents.outbreaks.b4690859d91684da963dc40e00f3da81 |
[3] | B. Hau, E. Kosman, Comparative analysis of flexible two-parameter models of plant disease epidemics, Phytopathology, 97(10), (2007), 1231–1244. https://doi.org/10.1094/PHYTO-97-10-1231 |
[4] |
Y. H. Hsieh, Pandemic influenza A (H1N1) during winter influenza season in the southern hemisphere, Influenza Other Respi. Viruses, 4 (2010), 187–197. https://doi.org/10.1111/j.1750-2659.2010.00147.x doi: 10.1111/j.1750-2659.2010.00147.x
![]() |
[5] |
A. N. Tsoularis, J. Wallace, Analysis of Logistic Growth Models, Math. Biosci., 179 (2002), 21–55. https://doi.org/10.1016/S0025-5564(02)00096-2 doi: 10.1016/S0025-5564(02)00096-2
![]() |
[6] | M. E. J. Turner, E. L. J. Bradley, K. Kirk, K. M. Pruitt, A theory of growth Math. Biosci., 29 (1976), 367–373. https://doi.org/10.1016/0025-5564(76)90112-7 |
[7] |
S. A. Colgate, E. A. Stanley, J. M. Hyman, S. P. Layne, C. Qualls, Risk behavior-based model of the cubic growth of acquired immunodeficiency syndrome in the United States, Proc. Natl Acad. Sci. U. S. A., 86 (1989), 4793–4797. https://doi.org/10.1073/pnas.86.12.4793 doi: 10.1073/pnas.86.12.4793
![]() |
[8] |
J. Ma, J. Dushoff, B. M. Bolker, D. J. Earn, Estimating initial epidemic growth rates, Bull. Math. Biol., 76 (2014), 245–60. https://doi.org/10.1007/s11538-013-9918-2 doi: 10.1007/s11538-013-9918-2
![]() |
[9] |
B. Szendroi, G. Csanyi, Polynomial epidemics and clustering in contact networks, Proc. Biol. Sci., 271 (2004), S364–S366. https://doi.org/10.1098/rsbl.2004.0188 doi: 10.1098/rsbl.2004.0188
![]() |
[10] |
C. Viboud, L. Simonsen, G. Chowell, A generalized-growth model to characterize the early ascending phase of infectious disease outbreaks epidemics, Epidemics, 15 (2016), 27–37. https://doi.org/10.1016/j.epidem.2016.01.002 doi: 10.1016/j.epidem.2016.01.002
![]() |
[11] | C. Jan, Gradually-varied Flow Profiles in Open Channels. Analytical Solutions by Using Gaussian Hypergeometric Function, Springer-Verlag, 2014. https://doi.org/10.1007/978-3-642-35242-3 |
[12] | Trends in Number of COVID-19 Cases and Deaths in the US Reported to CDC, by State/Territory, 2022. Available from: https://stacks.cdc.gov/view/cdc/102187. |
[13] |
G. Chowell, A. Tariq, J. M. Hyman, A novel sub-epidemic modeling framework for short-term forecasting epidemic waves, BMC Med., 17 (2019), 164. https://doi.org/10.1186/s12916-019-1406-6 doi: 10.1186/s12916-019-1406-6
![]() |
[14] |
K. Roosa, Y. Lee, R. Luo, A. Kirpich, R. Rothenberg, J. M. Hyman, et al., Short-term forecasts of the COVID-19 epidemic in Guangdong and Zhejiang, China, J. Clin. Med., 9 (2020), 596. https://doi.org/10.3390/jcm9020596 doi: 10.3390/jcm9020596
![]() |
[15] | N. Mukhopadhyay, Asymptotic normality of sequential stopping times with applications: Confidence intervals for an exponential mean, Calcutta Stat. Assoc. Bull., 72 (2020). https://doi.org/10.1177/0008068320923895 |
[16] | W. Zhu, N. Zeng, N. Wang, Sensitivity, Specificity, Accuracy, Associated Confidence Interval and ROC Analysis with Practical SAS Implementations, 2010. Available from: http://www.cpdm.ufpr.br/documentos/ROC.pdf |
[17] |
A. Owen, Empirical likelihood ratio confidence intervals for a single functional, Biometrika, 75 (1988), 237–249. https://doi.org/10.1093/biomet/75.2.237 doi: 10.1093/biomet/75.2.237
![]() |
[18] |
A. Owen, Empirical likelihood ratio confidence regions, Ann. Stat., 18 (1990), 90–120. https://doi.org/10.1214/aos/1176347494 doi: 10.1214/aos/1176347494
![]() |
[19] |
H. Huang, Y. Zhao, Empirical likelihood for the bivariate survival function under univariate censoring, J. Stat. Plann. Inference, 194 (2018), 32–46. https://doi.org/10.1016/j.jspi.2017.10.002 doi: 10.1016/j.jspi.2017.10.002
![]() |
[20] | G. Cheng, Y. Zhao, B. Li, Empirical likelihood inferences for the semiparametric additive, isotonic regression J. Multivar. Anal., 112 (2012), 172–182. https://doi.org/10.1016/j.jmva.2012.06.003 |
[21] |
J. Zhang, J. Zhang, X. Zhu, T. Lu, Testing symmetry based on empirical likelihood, J. Appl. Stat., 45 (2018), 2429–2445. https://doi.org/10.1080/02664763.2017.1421917 doi: 10.1080/02664763.2017.1421917
![]() |
[22] |
B. Y. Jing, J. Yuan, W. Zhou, Jackknife empirical likelihood, J. Am. Stat. Assoc., 104 (2009), 1224–1232. https://doi.org/10.1198/jasa.2009.tm08260 doi: 10.1198/jasa.2009.tm08260
![]() |
[23] | Y. Zhao, X. Meng, H. Yang, Jackknife empirical likelihood inference for the mean absolute deviation, Comput. Stat. Data Anal., 91, (2015), 92–101. https://doi.org/10.1016/j.csda.2015.06.001 |
[24] |
Y. Sang, X. Dang, Y. Zhao, Jackknife empirical likelihood methods for Gini correlations and their equality testing, J. Stat. Plann. Inference, 199 (2019), 45–59. https://doi.org/10.1016/j.jspi.2018.05.004 doi: 10.1016/j.jspi.2018.05.004
![]() |
[25] |
H. Lin, Z. Li, D. Wang, Y. Zhao, Jackknife empirical likelihood for the error variance in linear models, J. Nonparametr. Stat., 29 (2017), 151–166. https://doi.org/10.1080/10485252.2017.1285028 doi: 10.1080/10485252.2017.1285028
![]() |
[26] |
Y. Cheng, Y. Zhao, Bayesian jackknife empirical likelihood, Biometrika, 106 (2019), 981–988. https://doi.org/10.1093/biomet/asz031 doi: 10.1093/biomet/asz031
![]() |
1. | Jianhui Liang, Lifang Wang, Miao Ma, Miaolei Zhou, An Improved Chicken Swarm Optimization Algorithm for Solving Multimodal Optimization Problems, 2022, 2022, 1687-5273, 1, 10.1155/2022/5359732 | |
2. | Scott Greenhalgh, Anna Dumas, A generalized ODE susceptible-infectious-susceptible compartmental model with potentially periodic behavior, 2023, 8, 24680427, 1190, 10.1016/j.idm.2023.11.007 | |
3. | Antonio Di Crescenzo, Paola Paraggio, Francisco Torres-Ruiz, A Bertalanffy–Richards growth model perturbed by a time-dependent pattern, statistical analysis and applications, 2024, 139, 10075704, 108258, 10.1016/j.cnsns.2024.108258 | |
4. | Iz‐iddine EL‐Fassi, Juan J. Nieto, Masakazu Onitsuka, A new representation for the solution of the Richards‐type fractional differential equation, 2024, 0170-4214, 10.1002/mma.10394 | |
5. | Iván Area, Juan J. Nieto, On a Quadratic Nonlinear Fractional Equation, 2023, 7, 2504-3110, 469, 10.3390/fractalfract7060469 | |
6. | Jianhui Liang, Lifang Wang, Miao Ma, An Adaptive Dual-Population Collaborative Chicken Swarm Optimization Algorithm for High-Dimensional Optimization, 2023, 8, 2313-7673, 210, 10.3390/biomimetics8020210 |
r | K | p | a |
1.1 (0.81, 1.4) | 620 (560,650) | 0.96 (0.77, 1.3) | 0.3 (0.13, 0.96) |
r | K | p | a |
2.9 (1.9, 4.2) | 1000 (9500, 11000) | 0.47 (0.31, 0.55) | 0.25 (0.15, 0.70) |
State | r | K | p | a |
New Hampshire | 0.57 (0.52, 0.63) | 7200 (7000, 7300) | 0.86 (0.84, 0.88) | 0.17 (0.13, 0.2) |
Hawaii | 0.71 (0.54, 0.91) | 640 (590,690) | 1.1 (1, 1.1) | 0.094 (0.069, 0.13) |
True Moment of time cumulative cases double | n | NA | EL | JEL |
0.969 | 1 | (0.957748, 0.977638) | (0.958551, 0.976556) | (0.958579, 0.976554) |
2.016 | 2 | (1.992155, 2.028633) | (1.993715, 2.026684) | (1.993718, 2.026680) |
3.165 | 3 | (3.129453, 3.179136) | (3.131634, 3.176540) | (3.131644, 3.176530) |
4.455 | 4 | (4.414135, 4.473760) | (4.416851, 4.470673) | (4.416836, 4.470694) |
5.946 | 5 | (5.905395, 5.972138) | (5.908445, 5.968766) | (5.908472, 5.968749) |
7.752 | 6 | (7.714451, 7.786448) | (7.717759, 7.782801) | (7.717767, 7.782793) |
10.113 | 7 | (10.074112, 10.151846) | (10.077642, 10.147870) | (10.077629, 10.147860) |
13.719 | 8 | (13.673559, 13.768391) | (13.677840, 13.763624) | (13.677850, 13.763615) |
23.350 | 9 | (23.162364, 23.534539) | (23.184927, 23.522837) | (23.184957, 23.522801) |
True Interval of time Between Consecutive Doubling | n | NA | EL | JEL |
0.969 | 1 | (0.957748, 0.977638) | (0.958551, 0.976556) | (0.958579, 0.976554) |
1.047 | 2 | (1.034359, 1.051043) | (1.035081, 1.050180) | (1.035091, 1.050165) |
1.149 | 3 | (1.137063, 1.150738) | (1.137697, 1.150032) | (1.137689, 1.150040) |
1.290 | 4 | (1.283906, 1.295400) | (1.284455, 1.294837) | (1.284426, 1.294810) |
1.491 | 5 | (1.489251, 1.500387) | (1.489739, 1.499857) | (1.489765, 1.499835) |
1.806 | 6 | (1.805016, 1.818351) | (1.805718, 1.817770) | (1.805707, 1.817778) |
2.361 | 7 | (2.352960, 2.372099) | (2.353951, 2.371263) | (2.353953, 2.371261) |
3.606 | 8 | (3.589092, 3.626900) | (3.590984, 3.625175) | (3.590991, 3.625160) |
9.631 | 9 | (9.474994, 9.779960) | (9.494552, 9.771645) | (9.494577, 9.771616) |
True Moment of time cumulative cases doubles | n | NA | EL | JEL |
0.468 | 1 | (0.462279, 0.476864) | (0.463066, 0.476257) | (0.463062, 0.476260) |
1.162 | 2 | (1.138896, 1.172211) | (1.140686, 1.170805) | (1.140680, 1.170821) |
2.202 | 3 | (2.177769, 2.234873) | (2.180796, 2.232448) | (2.180820, 2.232471) |
3.788 | 4 | (3.746427, 3.833379) | (3.751046, 3.829665) | (3.751051, 3.829685) |
6.261 | 5 | (6.189960, 6.313948) | (6.196485, 6.308599) | (6.196486, 6.308594) |
10.244 | 6 | (10.139300, 10.309129) | (10.148049, 10.301598) | (10.148059, 10.301585) |
16.992 | 7 | (16.853192, 17.082037) | (16.864593, 17.071438) | (16.864613, 17.071418) |
29.502 | 8 | (29.323407, 29.646882) | (29.339147, 29.631349) | (29.339174, 29.631322) |
57.856 | 9 | (57.507775, 58.133745) | (57.540453, 58.106540) | (57.540501, 58.106465) |
True Interval of time Between Consecutive Doubling | n | NA | EL | JEL |
0.468 | 1 | (0.462279, 0.476864) | (0.463066, 0.476257) | (0.463062, 0.476260) |
0.694 | 2 | (0.676606, 0.695358) | (0.677604, 0.694572) | (0.677607, 0.694570) |
1.041 | 3 | (1.038819, 1.062716) | (1.040080, 1.061703) | (1.040086, 1.061696) |
1.586 | 4 | (1.568465, 1.598699) | (1.570054, 1.597358) | (1.570040, 1.597375) |
2.473 | 5 | (2.442930, 2.481171) | (2.444849, 2.479447) | (2.444861, 2.479426) |
3.982 | 6 | (3.947514, 3.997008) | (3.949911, 3.994645) | (3.949921, 3.994635) |
6.748 | 7 | (6.708214, 6.778586) | (6.711649, 6.775220) | (6.711656, 6.775212) |
12.510 | 8 | (12.452887, 12.582173) | (12.459753, 12.576669) | (12.459761, 12.576658) |
28.354 | 9 | (28.143750, 28.527481) | (28.165397, 28.513089) | (28.165422, 28.513042) |
n | NA | EL | JEL |
1 | (1.296990, 1.325518) | (1.298529, 1.324277) | (1.298505, 1.324302) |
2 | (2.900348, 2.956805) | (2.903265, 2.954363) | (2.903280, 2.954331) |
3 | (4.884038, 4.966410) | (4.888216, 4.962692) | (4.888211, 4.962693) |
4 | (7.340890, 7.445314) | (7.346032, 7.440461) | (7.346034, 7.440458) |
5 | (10.388441, 10.508826) | (10.394176, 10.503061) | (10.394188, 10.503050) |
6 | (14.177891, 14.305781) | (14.183782, 14.299462) | (14.183791, 14.299455) |
7 | (18.909441, 19.034630) | (18.915030, 19.028286) | (18.915037, 19.028282) |
8 | (24.862485, 24.975516) | (24.867473, 24.969776) | (24.867484, 24.969766) |
9 | (32.465413, 32.564050) | (32.469979, 32.559271) | (32.469989, 32.559260) |
10 | (42.492654, 42.589418) | (42.497416, 42.584906) | (42.497426, 42.584896) |
11 | (56.776063, 56.886604) | (56.781603, 56.881411) | (56.781586, 6.881409) |
12 | (82.431949, 82.618957) | (82.441320, 82.610516) | (82.441336, 82.610500) |
n | NA | EL | JEL |
1 | (1.296990, 1.325518) | (1.298529, 1.324277) | (1.298505, 1.324302) |
2 | (1.603345, 1.631299) | (1.604792, 1.630013) | (1.604765, 1.630041) |
3 | (1.983635, 2.009660) | (1.984865, 2.008437) | (1.984885, 2.008417) |
4 | (2.456660, 2.479097) | (2.457661, .477939) | (2.457663, 2.477955) |
5 | (3.046878, 3.064184) | (3.047593, 3.063270) | (3.047599, 3.063260) |
6 | (3.786726, 3.799679) | (3.787323, 3.799023) | (3.787311, 3.799037) |
7 | (4.721794, 4.738605) | (4.722481, 4.737708) | (4.722489, 4.737693) |
8 | (5.932581, 5.961348) | (5.933821, 5.959773) | (5.933798, 5.959794) |
9 | (7.574728, 7.616734) | (7.576687, 7.614647) | (7.576684, 7.614652) |
10 | (10.001532, 10.051077) | (10.004001, 10.048830) | (10.004011, 10.048820) |
11 | (14.265515, 14.315081) | (14.267936, 14.312761) | (14.267946, 14.312752) |
12 | (25.628555, 25.759683) | (25.635179, 25.753758) | (25.635190, 25.753718) |
n | NA | EL | JEL |
1 | (2.198740, 2.226115) | (2.200083, 2.224878) | (2.200113, 2.224848) |
2 | (4.465612, 4.515581) | (4.468100, 4.513272) | (4.468110, 4.513261) |
3 | (6.841237, 6.909199) | (6.844618, 6.906041) | (6.844624, 6.906035) |
4 | (9.385318, 9.466835) | (9.389377, 9.463027) | (9.389371, 9.463032) |
5 | (12.192842, 12.283751) | (12.197337, 12.279508) | (12.197345, 12.279500) |
6 | (15.431831, 15.528894) | (15.436600, 15.524349) | (15.436610, 15.524339) |
7 | (19.450330, 19.554213) | (19.455392, 19.549313) | (19.455394, 19.549311) |
8 | (25.200992, 25.331885) | (25.207450, 25.325901) | (25.207461, 25.325874) |
9 | (38.314156, 38.720210) | (38.338153, 38.706650) | (38.338161, 38.706624) |
n | NA | EL | JEL |
1 | (2.198740, 2.226115) | (2.200083, 2.224878) | (2.200113, 2.224848) |
2 | (2.266848, 2.289491) | (2.267973, 2.288438) | (2.267975, 2.288435) |
3 | (2.375497, 2.393745) | (2.376394, 2.392893) | (2.376398, 2.392889) |
4 | (2.543588, 2.558130) | (2.544302, 2.557439) | (2.544298, 2.557443) |
5 | (2.805798, 2.818642) | (2.806437, 2.818023) | (2.806423, 2.818036) |
6 | (3.234070, 3.250063) | (3.234861, 3.249375) | (3.234882, 3.249360) |
7 | (4.008355, 4.035462) | (4.009760, 4.034378) | (4.009787, 4.034351) |
8 | (5.735666, 5.792668) | (5.738761, 5.790458) | (5.738777, 5.790478) |
9 | (13.099598, 13.401891) | (13.118536, 13.393273) | (13.118561, 13.393238) |
r | K | p | a |
1.1 (0.81, 1.4) | 620 (560,650) | 0.96 (0.77, 1.3) | 0.3 (0.13, 0.96) |
r | K | p | a |
2.9 (1.9, 4.2) | 1000 (9500, 11000) | 0.47 (0.31, 0.55) | 0.25 (0.15, 0.70) |
State | r | K | p | a |
New Hampshire | 0.57 (0.52, 0.63) | 7200 (7000, 7300) | 0.86 (0.84, 0.88) | 0.17 (0.13, 0.2) |
Hawaii | 0.71 (0.54, 0.91) | 640 (590,690) | 1.1 (1, 1.1) | 0.094 (0.069, 0.13) |
True Moment of time cumulative cases double | n | NA | EL | JEL |
0.969 | 1 | (0.957748, 0.977638) | (0.958551, 0.976556) | (0.958579, 0.976554) |
2.016 | 2 | (1.992155, 2.028633) | (1.993715, 2.026684) | (1.993718, 2.026680) |
3.165 | 3 | (3.129453, 3.179136) | (3.131634, 3.176540) | (3.131644, 3.176530) |
4.455 | 4 | (4.414135, 4.473760) | (4.416851, 4.470673) | (4.416836, 4.470694) |
5.946 | 5 | (5.905395, 5.972138) | (5.908445, 5.968766) | (5.908472, 5.968749) |
7.752 | 6 | (7.714451, 7.786448) | (7.717759, 7.782801) | (7.717767, 7.782793) |
10.113 | 7 | (10.074112, 10.151846) | (10.077642, 10.147870) | (10.077629, 10.147860) |
13.719 | 8 | (13.673559, 13.768391) | (13.677840, 13.763624) | (13.677850, 13.763615) |
23.350 | 9 | (23.162364, 23.534539) | (23.184927, 23.522837) | (23.184957, 23.522801) |
True Interval of time Between Consecutive Doubling | n | NA | EL | JEL |
0.969 | 1 | (0.957748, 0.977638) | (0.958551, 0.976556) | (0.958579, 0.976554) |
1.047 | 2 | (1.034359, 1.051043) | (1.035081, 1.050180) | (1.035091, 1.050165) |
1.149 | 3 | (1.137063, 1.150738) | (1.137697, 1.150032) | (1.137689, 1.150040) |
1.290 | 4 | (1.283906, 1.295400) | (1.284455, 1.294837) | (1.284426, 1.294810) |
1.491 | 5 | (1.489251, 1.500387) | (1.489739, 1.499857) | (1.489765, 1.499835) |
1.806 | 6 | (1.805016, 1.818351) | (1.805718, 1.817770) | (1.805707, 1.817778) |
2.361 | 7 | (2.352960, 2.372099) | (2.353951, 2.371263) | (2.353953, 2.371261) |
3.606 | 8 | (3.589092, 3.626900) | (3.590984, 3.625175) | (3.590991, 3.625160) |
9.631 | 9 | (9.474994, 9.779960) | (9.494552, 9.771645) | (9.494577, 9.771616) |
True Moment of time cumulative cases doubles | n | NA | EL | JEL |
0.468 | 1 | (0.462279, 0.476864) | (0.463066, 0.476257) | (0.463062, 0.476260) |
1.162 | 2 | (1.138896, 1.172211) | (1.140686, 1.170805) | (1.140680, 1.170821) |
2.202 | 3 | (2.177769, 2.234873) | (2.180796, 2.232448) | (2.180820, 2.232471) |
3.788 | 4 | (3.746427, 3.833379) | (3.751046, 3.829665) | (3.751051, 3.829685) |
6.261 | 5 | (6.189960, 6.313948) | (6.196485, 6.308599) | (6.196486, 6.308594) |
10.244 | 6 | (10.139300, 10.309129) | (10.148049, 10.301598) | (10.148059, 10.301585) |
16.992 | 7 | (16.853192, 17.082037) | (16.864593, 17.071438) | (16.864613, 17.071418) |
29.502 | 8 | (29.323407, 29.646882) | (29.339147, 29.631349) | (29.339174, 29.631322) |
57.856 | 9 | (57.507775, 58.133745) | (57.540453, 58.106540) | (57.540501, 58.106465) |
True Interval of time Between Consecutive Doubling | n | NA | EL | JEL |
0.468 | 1 | (0.462279, 0.476864) | (0.463066, 0.476257) | (0.463062, 0.476260) |
0.694 | 2 | (0.676606, 0.695358) | (0.677604, 0.694572) | (0.677607, 0.694570) |
1.041 | 3 | (1.038819, 1.062716) | (1.040080, 1.061703) | (1.040086, 1.061696) |
1.586 | 4 | (1.568465, 1.598699) | (1.570054, 1.597358) | (1.570040, 1.597375) |
2.473 | 5 | (2.442930, 2.481171) | (2.444849, 2.479447) | (2.444861, 2.479426) |
3.982 | 6 | (3.947514, 3.997008) | (3.949911, 3.994645) | (3.949921, 3.994635) |
6.748 | 7 | (6.708214, 6.778586) | (6.711649, 6.775220) | (6.711656, 6.775212) |
12.510 | 8 | (12.452887, 12.582173) | (12.459753, 12.576669) | (12.459761, 12.576658) |
28.354 | 9 | (28.143750, 28.527481) | (28.165397, 28.513089) | (28.165422, 28.513042) |
n | NA | EL | JEL |
1 | (1.296990, 1.325518) | (1.298529, 1.324277) | (1.298505, 1.324302) |
2 | (2.900348, 2.956805) | (2.903265, 2.954363) | (2.903280, 2.954331) |
3 | (4.884038, 4.966410) | (4.888216, 4.962692) | (4.888211, 4.962693) |
4 | (7.340890, 7.445314) | (7.346032, 7.440461) | (7.346034, 7.440458) |
5 | (10.388441, 10.508826) | (10.394176, 10.503061) | (10.394188, 10.503050) |
6 | (14.177891, 14.305781) | (14.183782, 14.299462) | (14.183791, 14.299455) |
7 | (18.909441, 19.034630) | (18.915030, 19.028286) | (18.915037, 19.028282) |
8 | (24.862485, 24.975516) | (24.867473, 24.969776) | (24.867484, 24.969766) |
9 | (32.465413, 32.564050) | (32.469979, 32.559271) | (32.469989, 32.559260) |
10 | (42.492654, 42.589418) | (42.497416, 42.584906) | (42.497426, 42.584896) |
11 | (56.776063, 56.886604) | (56.781603, 56.881411) | (56.781586, 6.881409) |
12 | (82.431949, 82.618957) | (82.441320, 82.610516) | (82.441336, 82.610500) |
n | NA | EL | JEL |
1 | (1.296990, 1.325518) | (1.298529, 1.324277) | (1.298505, 1.324302) |
2 | (1.603345, 1.631299) | (1.604792, 1.630013) | (1.604765, 1.630041) |
3 | (1.983635, 2.009660) | (1.984865, 2.008437) | (1.984885, 2.008417) |
4 | (2.456660, 2.479097) | (2.457661, .477939) | (2.457663, 2.477955) |
5 | (3.046878, 3.064184) | (3.047593, 3.063270) | (3.047599, 3.063260) |
6 | (3.786726, 3.799679) | (3.787323, 3.799023) | (3.787311, 3.799037) |
7 | (4.721794, 4.738605) | (4.722481, 4.737708) | (4.722489, 4.737693) |
8 | (5.932581, 5.961348) | (5.933821, 5.959773) | (5.933798, 5.959794) |
9 | (7.574728, 7.616734) | (7.576687, 7.614647) | (7.576684, 7.614652) |
10 | (10.001532, 10.051077) | (10.004001, 10.048830) | (10.004011, 10.048820) |
11 | (14.265515, 14.315081) | (14.267936, 14.312761) | (14.267946, 14.312752) |
12 | (25.628555, 25.759683) | (25.635179, 25.753758) | (25.635190, 25.753718) |
n | NA | EL | JEL |
1 | (2.198740, 2.226115) | (2.200083, 2.224878) | (2.200113, 2.224848) |
2 | (4.465612, 4.515581) | (4.468100, 4.513272) | (4.468110, 4.513261) |
3 | (6.841237, 6.909199) | (6.844618, 6.906041) | (6.844624, 6.906035) |
4 | (9.385318, 9.466835) | (9.389377, 9.463027) | (9.389371, 9.463032) |
5 | (12.192842, 12.283751) | (12.197337, 12.279508) | (12.197345, 12.279500) |
6 | (15.431831, 15.528894) | (15.436600, 15.524349) | (15.436610, 15.524339) |
7 | (19.450330, 19.554213) | (19.455392, 19.549313) | (19.455394, 19.549311) |
8 | (25.200992, 25.331885) | (25.207450, 25.325901) | (25.207461, 25.325874) |
9 | (38.314156, 38.720210) | (38.338153, 38.706650) | (38.338161, 38.706624) |
n | NA | EL | JEL |
1 | (2.198740, 2.226115) | (2.200083, 2.224878) | (2.200113, 2.224848) |
2 | (2.266848, 2.289491) | (2.267973, 2.288438) | (2.267975, 2.288435) |
3 | (2.375497, 2.393745) | (2.376394, 2.392893) | (2.376398, 2.392889) |
4 | (2.543588, 2.558130) | (2.544302, 2.557439) | (2.544298, 2.557443) |
5 | (2.805798, 2.818642) | (2.806437, 2.818023) | (2.806423, 2.818036) |
6 | (3.234070, 3.250063) | (3.234861, 3.249375) | (3.234882, 3.249360) |
7 | (4.008355, 4.035462) | (4.009760, 4.034378) | (4.009787, 4.034351) |
8 | (5.735666, 5.792668) | (5.738761, 5.790458) | (5.738777, 5.790478) |
9 | (13.099598, 13.401891) | (13.118536, 13.393273) | (13.118561, 13.393238) |