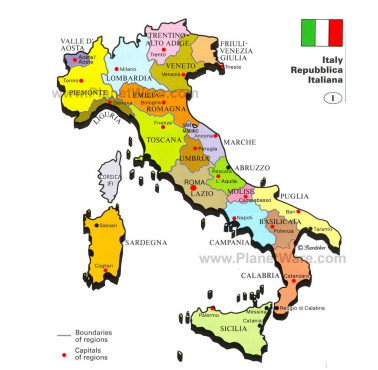
The current statistical modeling of coronavirus (COVID-19) spread has mainly focused on spreading patterns and forecasting of COVID-19 development; these patterns have been found to vary among locations. As the survival time of coronaviruses on surfaces depends on temperature, some researchers have explored the association of daily confirmed cases with environmental factors. Furthermore, some researchers have studied the link between daily fatality rates with regional factors such as health resources, but found no significant factors. As the spreading patterns of COVID-19 development vary a lot among locations, fitting regression models of daily confirmed cases or fatality rates directly with regional factors might not reveal important relationships. In this study, we investigate the link between regional spreading patterns of COVID-19 development in Italy and regional factors in two steps. First, we characterize regional spreading patterns of COVID-19 daily confirmed cases by a special patterned Poisson regression model for longitudinal count; the varying growth and declining patterns as well as turning points among regions in Italy have been well captured by regional regression parameters. We then associate these regional regression parameters with regional factors. The effects of regional factors on spreading patterns of COVID-19 daily confirmed cases have been effectively evaluated.
Citation: Youtian Hao, Guohua Yan, Renjun Ma, M. Tariqul Hasan. Linking dynamic patterns of COVID-19 spreads in Italy with regional characteristics: a two level longitudinal modelling approach[J]. Mathematical Biosciences and Engineering, 2021, 18(3): 2579-2598. doi: 10.3934/mbe.2021131
[1] | Kai Wang, Zhenzhen Lu, Xiaomeng Wang, Hui Li, Huling Li, Dandan Lin, Yongli Cai, Xing Feng, Yateng Song, Zhiwei Feng, Weidong Ji, Xiaoyan Wang, Yi Yin, Lei Wang, Zhihang Peng . Current trends and future prediction of novel coronavirus disease (COVID-19) epidemic in China: a dynamical modeling analysis. Mathematical Biosciences and Engineering, 2020, 17(4): 3052-3061. doi: 10.3934/mbe.2020173 |
[2] | Weike Zhou, Aili Wang, Fan Xia, Yanni Xiao, Sanyi Tang . Effects of media reporting on mitigating spread of COVID-19 in the early phase of the outbreak. Mathematical Biosciences and Engineering, 2020, 17(3): 2693-2707. doi: 10.3934/mbe.2020147 |
[3] | Sarah R. Al-Dawsari, Khalaf S. Sultan . Modeling of daily confirmed Saudi COVID-19 cases using inverted exponential regression. Mathematical Biosciences and Engineering, 2021, 18(3): 2303-2330. doi: 10.3934/mbe.2021117 |
[4] | Lei Nie, Xin Guo, Chengqi Yi, Ruojia Wang . Analyzing the effects of public interventions on reducing public gatherings in China during the COVID-19 epidemic via mobile terminals positioning data. Mathematical Biosciences and Engineering, 2020, 17(5): 4875-4890. doi: 10.3934/mbe.2020265 |
[5] | Vitaliy Yakovyna, Natalya Shakhovska . Modelling and predicting the spread of COVID-19 cases depending on restriction policy based on mined recommendation rules. Mathematical Biosciences and Engineering, 2021, 18(3): 2789-2812. doi: 10.3934/mbe.2021142 |
[6] | Omar El Deeb, Maya Jalloul . The dynamics of COVID-19 spread: evidence from Lebanon. Mathematical Biosciences and Engineering, 2020, 17(5): 5618-5632. doi: 10.3934/mbe.2020302 |
[7] | Sha He, Sanyi Tang, Libin Rong . A discrete stochastic model of the COVID-19 outbreak: Forecast and control. Mathematical Biosciences and Engineering, 2020, 17(4): 2792-2804. doi: 10.3934/mbe.2020153 |
[8] | Luyu Zhang, Zhaohua Zhang, Sen Pei, Qing Gao, Wei Chen . Quantifying the presymptomatic transmission of COVID-19 in the USA. Mathematical Biosciences and Engineering, 2024, 21(1): 861-883. doi: 10.3934/mbe.2024036 |
[9] | Sarita Bugalia, Vijay Pal Bajiya, Jai Prakash Tripathi, Ming-Tao Li, Gui-Quan Sun . Mathematical modeling of COVID-19 transmission: the roles of intervention strategies and lockdown. Mathematical Biosciences and Engineering, 2020, 17(5): 5961-5986. doi: 10.3934/mbe.2020318 |
[10] | Tianfang Hou, Guijie Lan, Sanling Yuan, Tonghua Zhang . Threshold dynamics of a stochastic SIHR epidemic model of COVID-19 with general population-size dependent contact rate. Mathematical Biosciences and Engineering, 2022, 19(4): 4217-4236. doi: 10.3934/mbe.2022195 |
The current statistical modeling of coronavirus (COVID-19) spread has mainly focused on spreading patterns and forecasting of COVID-19 development; these patterns have been found to vary among locations. As the survival time of coronaviruses on surfaces depends on temperature, some researchers have explored the association of daily confirmed cases with environmental factors. Furthermore, some researchers have studied the link between daily fatality rates with regional factors such as health resources, but found no significant factors. As the spreading patterns of COVID-19 development vary a lot among locations, fitting regression models of daily confirmed cases or fatality rates directly with regional factors might not reveal important relationships. In this study, we investigate the link between regional spreading patterns of COVID-19 development in Italy and regional factors in two steps. First, we characterize regional spreading patterns of COVID-19 daily confirmed cases by a special patterned Poisson regression model for longitudinal count; the varying growth and declining patterns as well as turning points among regions in Italy have been well captured by regional regression parameters. We then associate these regional regression parameters with regional factors. The effects of regional factors on spreading patterns of COVID-19 daily confirmed cases have been effectively evaluated.
Much of the effort on statistical modeling of coronavirus (COVID-19) spread has contributed to spreading patterns and forecasting of COVID-19 development; these patterns have been found to vary among locations [1,2]. As the survival time of coronaviruses on surfaces has found to depend on temperature, some researchers have explored the association of daily or cumulative confirmed cases with environmental factors [3,4,5]. To forecast COVID-19 related new cases and deaths, various authors have employed deep learning, artificial intelligence (AI) and time series approaches. For example, recurrent neural network (RNN) based deep learning techniques is proposed by [6] for forecasting COVID-19 related new cases. For predicting the dynamical behavior of COVID-19 cases various artificial intelligence (AI) based modeling techniques such as Bayesian regression neural network, cubist regression, k-nearest neighbors, quantile random forest, and support vector regression were employed in [7]. Autoregressive integrated moving average (ARIMA) based time series forecasting techniques were also incorporated in the literature to envision COVID-19 related new cases as well as deaths [8,9,10,11,12]. To predict the short-term spread of COVID-19, Singhal et al. [13] have proposed Gaussian mixture model-based techniques. Batista [14] has used susceptible-infected-recovered (SIR) model for estimating the final size of the COVID-19 pandemic spread. To accommodate time varying transmission and removal rate, and temporal trend of COVID-19 disease, Hong and Li [15] proposed time-dependent Poisson model. Bertozzi et al. [16] incorporated three models such as exponential growth, self-existing branching process and SIR models to predict the behavior of COVID-19 transmission in various stages of the diseases. These models in [13,14,15,16] are suitable for analyzing time series data without accommodating any covariate. But incorporating covariate information for predicting the COVID-19 disease spread may reveal important information. Furthermore, some researchers have studied the link between daily fatality rates with regional factors such as health resources, but found no significant factors [17]. As the spreading patterns of COVID-19 development vary a lot among locations, fitting regression models of daily confirmed cases or fatality rates directly with regional factors might not always reveal important relationships. In this study, we investigate the link between regional spreading patterns of COVID-19 development in Italy and regional factors in two steps. First, we extend the method of Zhang et al.[1] to handle longitudinal count in order to characterize regional spreading patterns of COVID-19 daily confirmed cases; the varying growth and declining patterns as well as turning points among regions in Italy have been well captured by regional regression parameters of this model. We then associate these regional regression parameters with regional factors. The effects of regional factors on regional spreading patterns of COVID-19 daily confirmed cases have been effectively evaluated.
The coronavirus disease 2019 (COVID-2019) has so far spread to over 200 countries causing more than 20 million confirmed cases globally by August 15, 2020. In addition to countermeasures such as lockdowns and social distancing, it is also important to explore and reveal the relationship between virus transmission and potential environmental covariates such as temperature, to help us better understand the behavior of the virus, and further implement more efficient public interventions to contain the spread. Italy, as one of the countries first affected by the virus with a total number of more than 240,000 confirmed cases by the end of June, has experienced the transmission periods from exponential growth, turning point to flattened tail, which provides us an ideally mature data set for relevant regression analysis. Furthermore, potential covariates such as demographic and economic status vary from region to region in the country, and specially, as Italy has a geographically narrow and long shape from north to south, plus the mountainous terrain in midland, temperature conditions also vary at regional level. The variability of covariates makes regression analysis approach more feasible. In addition, the fact that Italy has carried out a unified countermeasure policy across the country helps minimize various effects brought by human intervention at regional level.
Our proposed hierarchical linear model deals with the analysis of the daily confirmed cases from February 24 to June 30, 2020 from various regions in Italy. To accommodate the heterogeneity among various regions, we consider regional level random effects. To depict infection-Time relationship, we adopt a power law with exponential cutoff (PLEC) function [1,18], which has exponential increase and decay terms. By incorporating the PLEC function into the hierarchical linear model, we can achieve the two major goals in this study: First, we construct a new hierarchical model to fit and predict the COVID-19 transmission trend in Italy, and compare it with the PLEC model [1,18]. Second, we conduct regression analysis on the relationship between COVID-19 transmission in Italy and spatially-varied covariates, including population density, GDP per capita and temperature. Our main interest in this study is the relationship between transmission and temperature.
Construction of the manuscript is as follows. After the proposed methodology in Section 2, we discuss our analysis results in Section 3. Some further discussions and conclusions of the proposed research are in Sections 4 and 5, respectively.
In this section, we briefly discuss the dataset used in the manuscript first before introducing the model. We collected daily confirmed cases data in Italy from Github [19] website from February 24, 2020 to June 30, 2020, from each of the 21 regions in Italy (including autonomous areas). There are situations when daily new cases are negative in certain regions due to data correction by the Italian authority, and we treat these observations as 0. Coordinates of each region were also gathered for further use. Italian demographic and economic data were collected from Wikipedia [20], including each region's population density and Gross Domestic Product (GDP) per capita. We also collected each region's historical daily average temperature from January 1 to June 30, 2020 on Wunderground website [21], and then calculated an average temperature in Fahrenheit during this period for each region. To better understand the geography, we present the map of Italy with various regions presented in Figure 1.
We consider regional factors such as population density, GDP per capita, and average temperature on the transmission of COVID-19 virus in Italy. To accommodate the different longitudinal patterns among the regions, we introduce three region-specific random effects, corresponding the three parameters in the PLEC function. Figure 2 demonstrates how fixed and random effects affect the transmission of the virus in different regions. Curves in different regions generally follow patterns that can be described by some PLEC curves, experiencing exponential growth and decay process, but the starting and turning point, as well as the growth rate of each curve differ across the regions in Italy, due to the influence from both fixed and random effects. By adding legends on average temperature and population density, some fixed effects from these two covariates can be visualized: Regions with higher average temperature appear to have curves at lower position, while curves of higher population density regions are at higher positions in the graph.
In this section we present the PLEC function which will be used in the level-one model. It is noteworthy to point out that the PLEC function has a satisfactory performance on depicting COVID-19 transmission curves in different countries [18,22]. Following Ma [18], Wei and Zhang [22], the PLEC function has the following form
I=cTwexp(−dT) | (2.1) |
where T is the time(in days) since the transmission begins, I is the number of daily new infections, c, w, and d are three positive parameters to be estimated from the data. Each of the parameters has its biological interpretation: cTw term is a power function with w dominating the transmission growth with a multiplier c, and the exponential term exp(−dT) characterizes the declining trend of the transmission curve. Following [1], we can get the maximum value of infection by taking the derivative of df(I)/dT and setting it to zero as,
Imax=c(wd)wexp(−w), | (2.2) |
which occurs at the turning point of
Tmax( peak)=wd. | (2.3) |
In order to incorporate the PLEC function with spatially-varied covariates in Italy, we assume that the daily confirmed case, which is a count response, follows a Poisson distribution as,
Yij∼Poisson(μij), |
where
μij=ecj−djTiTwji, | (2.4) |
is the PLEC function in an alternative form, and ecj corresponds to c in Eq (2.1). In (2.4), μij, the mean value of daily new cases, on the ith level-one unit (T), representing 128 longitudinal daily observations from February 24 to June 30, nested within jth level-two (regional level) unit, where j = 1, 2, ..., 21, representing 21 regions in Italy. The maximum value of infection and its turning point in jth region can be derived as
μmax,j=ecj−wj(wjdj)wj,Tmax,j=wjdj. | (2.5) |
If we further apply log-transformation, Eq (2.4) can be expressed as
log(μij)=cj+wjlog(Ti)+(−djTi). | (2.6) |
In (2.6), T, and its log form log(T) are level-one (day) predictors, which take identical day serial values across regions, with −dj and wj as coefficients, and cj can be treated as the intercept term.
In level-two model, we consider the regression coefficients cj, wj, and −dj from level-one models as dependent variables incorporated with corresponding level-two covariates.
{cj=α0+x⊺jβ0+e0jwj=α1+x⊺jβ1+e1j−dj=α2+x⊺jβ2+e2j | (2.7) |
In our case, xj is the vector of spatially-varied covariates at regional level, incorporated with level-one regression coefficients, and it may contain multiple covariates of interest. In addition, the xj expression can be different for the three equations in (2.7). β0, β1 and β2 are coefficients of level-two covariates and α0, α1, α2 are corresponding intercepts. The residual terms e0j, e1j, and e2j are the random effects of the jth level-two unit (region) on the level-one parameters cj, wj, and −dj respectively. Following the derivation of Sullivan et al.[23], We assume that the error terms ej=(e0j,e1j,e2j)⊺ follow a tri-variate normal with mean (0, 0, 0) and an unknown covariance matrix G.
We can derive our combined model by substituting (2.7) into (2.6):
Yij∼Poisson(μij)log(μij)=(α0+e0j)+(α1+e1j)log(Ti)+(α2+e2j)Ti+x⊺jβ0+x⊺jβ1log(Ti)+x⊺jβ2Ti | (2.8) |
Now both level-one and level-two covariates (time and spatially-varied covariates), interaction terms, and error terms are included in one model. It is then clear that the hierarchical formulation is equivalent to the random-intercept-and-random-slope model.
We derive our computational method in matrix form. First, we rewrite Eq (2.6) into the following expression,
log(μj)=yj=Djγj, | (2.9) |
where log(μj)=(log(μ1j),log(μ2j),…,log(μ128,j))⊺, is the log form of mean response in 128 days in jth region, and γj=(cj,wj,−dj)⊺, is the parameter vector at level-one. Dj is a known design matrix with following form identical across regions,
Dj=[1log(1)11log(2)2......1log(128)128]. | (2.10) |
The first column in Dj is a designed column for intercept term cj, and third and second columns take day serial values and their log forms. We further rewrite level-two equation in the following matrix form
γj=Wjβ+ej, | (2.11) |
where β = (α0,β⊺0,α1,β⊺1,α2,β⊺2)⊺ is a vector of level-two coefficients, including both intercept and slope terms, which need to be estimated in the regression, and ej=(e1j,e2j,e3j)⊺ is a residual vector for random effects associated with three covariates from level-one model. As the known designed matrix of β, Wj takes the following form as
Wj=[1x⊺j00⊺00⊺00⊺1x⊺j00⊺00⊺00⊺1x⊺j], | (2.12) |
where β, xj and 0 have the same length, depending on the number of level-two covariates we select in xj. For a combined model, it has the following matrix form,
log(μj)=Djγj=Dj(Wjβ+ej)=DjWjβ+Djej, | (2.13) |
where
ej∼N(0,G),G=[τ00τ01τ02τ01τ11τ12τ02τ12τ22]. | (2.14) |
We define Aj=DjWj, and it has a matrix expression as following:
Aj=[1x⊺jlog(1)log(1)x⊺j11x⊺j1x⊺jlog(2)log(2)x⊺j22x⊺j............1x⊺jlog(128)log(128)x⊺j128128x⊺j] | (2.15) |
Then we can use least squares method to estimate β by applying following formula:
ˆβ=(A⊺ˆV−1A)−1A⊺ˆV−1YV=var(Y)=DGD⊺ | (2.16) |
A, D are design matrices across regions, and ˆV can be achieved with G's maximum likelihood estimates. Variances of ˆβ can be estimated by:
^var(β)=(A⊺ˆV−1A)−1 | (2.17) |
As our data is balanced with identical day counts in each region, G can be estimated by using closed-form maximum likelihood formulae. Random effects can be predicted by using best linear unbiased prediction (BLUP) method.
In this section we present our analysis results. We first include all potential spatially-varied covariates of interest into the level-two model, including regional average temperature, GDP per capita and population density. By inserting these level-two covariates into (2.7) and (2.8), we have the expressions of the first model as follows:
{cj=α0+β00AverageTemperaturej+β01PopulationDensityj+β02GDPPerCapitaj+e0jwj=α1+β10AverageTemperaturej+β11PopulationDensityj+β12GDPPerCapitaj+e1j−dj=α2+β20AverageTemperaturej+β21PopulationDensityj+β22GDPPerCapitaj+e2j | (3.1) |
Yij∼Poisson(μij)log(μij)=(α0+e0j)+(α1+e1j)log(Ti)+(α2+e2j)Ti+β00AverageTemperature+β01PopulationDensity+β02GDPPerCapita+β10AverageTemperature∗log(Ti)+β11PopulationDensity∗log(Ti)+β12GDPPerCap∗log(Ti)+β20AverageTemperature∗Ti+β21PopulationDensity∗Ti+β22GDPPerCapita∗Ti | (3.2) |
We use R function "glmer" in "lme4" package [24] to estimate the coefficients and predict random effects by specifying a nested structure at regional level on all three level-one covariates, which are the intercept term, day and log(day). Before applying "glmer" function, all level-two covariates were scaled so that the algorithm can converge more easily. We present the results of Model 1 in Table 1.
Coefficients | Fixed effects | Estimate | Std.Error | z value | P-value |
α0 | Intercept | -7.3347 | 0.5248 | -13.977 | <2e−16 |
α1 | log(day) | 4.6780 | 0.1918 | 24.395 | <2e−16 |
α2 | day | -0.1330 | 0.0062 | -21.486 | <2e−16 |
β00 | AverageTemperature | 0.0885 | 0.7353 | 0.120 | 0.9042 |
β01 | PopulationDensity | 2.3096 | 0.5264 | 4.388 | 1.15e-05 |
β02 | GDP per capita | 1.8953 | 0.7234 | 2.620 | 0.0088 |
β10 | log(day)*AverageTemperature | -0.3293 | 0.2689 | -1.225 | 0.2206 |
β11 | log(day)*PopulationDensity | -0.6027 | 0.1923 | -3.134 | 0.0017 |
β12 | log(day)*GDPPerCapita | -0.6909 | 0.2644 | -2.613 | 0.0090 |
β20 | day*AverageTemperature | 0.0160 | 0.0086 | 1.857 | 0.0633 |
β21 | day*PopulationDensity | 0.0185 | 0.0062 | 2.980 | 0.0029 |
β22 | day*GDPPerCapita | 0.0225 | 0.0086 | 2.628 | 0.0086 |
Based on the p-values, the three spatially-varied covariates or their interaction terms have significant effects at 0.1 significance level. Our results indicate that population density and GDP Per capita related terms have similar fixed effects on estimated mean value of daily cases, which is a sign that these two regional covariates could be correlated, thus we might need to drop one of them in further analysis. Moreover, we need to take the geographic factor into consideration when studying virus transmission, and certain geographic transmission trend could produce confounding variables. When COVID-19 broke out in Italy, it was believed that the virus hit the north first, and then it started to spread to the south. Therefore, if level-two covariates have a corresponding geographic trend, they could be confounded.
Figure 3 helps visualize the confounding effects caused by the geographic pattern. The size of the circles, with gradient color, represent number of cumulative cases, and the circles' growth trend can be observed by adding animation effects. Figure 3 implies that transmission associated with both average temperature and GDP per capita also follows a certain geographic pattern from north to south. To incorporate this potential confounding geographic effect, we introduce a new covariate "Distance" into the level-two model. The coordinates are used to calculate the geographic distance between each region and region "Lombardia", where people believe the virus first started to spread. The level-two covariate matrix in this "full model" becomes:
β0=(β00,β01,β02,β03)⊺β1=(β10,β11,β12,β13)⊺β2=(β20,β21,β22,β23)⊺xj=(AverageTemperaturej,PopulationDensityj,GDPPerCapitaj,Distancej)⊺ | (3.3) |
Results from the full model are displayed in Table 2. After bringing "Distance" covariate into the model, all GDP per capita related terms become insignificant at any conventional level of significance, so the GDP per capita covariate could be confounded with distance, and thus should be dropped.
Coefficients | Fixed effects | Estimate | Std.Error | z value | P-value |
α0 | Intercept | -7.3336 | 0.5166 | -14.197 | <2e−16 |
α1 | log(day) | 4.6775 | 0.1897 | 24.659 | <2e−16 |
α2 | day | -0.1330 | 0.0061 | -21.862 | <2e−16 |
β00 | AverageTemperature | 0.2644 | 0.7534 | 0.351 | 0.7256 |
β01 | PopulationDensity | 2.0910 | 0.5314 | 4.157 | 3.22e-05 |
β02 | GDP per capita | 1.1906 | 1.1067 | 1.0760 | 0.2820 |
β03 | Distance | -0.9478 | 1.1468 | -0.827 | 0.4085 |
β10 | log(day)*AverageTemperature | -0.3821 | 0.2767 | -1.381 | 0.1673 |
β11 | log(day)*PopulationDensity | -0.5721 | 0.1951 | -2.932 | 0.0034 |
β12 | log(day)*GDPPerCapita | -0.4770 | 0.4071 | -1.172 | 0.2413 |
β13 | log(day)*Distance | 0.2873 | 0.4213 | 0.682 | 0.4953 |
β20 | day*AverageTemperature | 0.0181 | 0.0088 | 2.055 | 0.0399 |
β21 | day*PopulationDensity | 0.0173 | 0.0063 | 2.761 | 0.0058 |
β22 | day*GDPPerCapita | 0.0134 | 0.0131 | 1.062 | 0.2881 |
β23 | day*Distance | -0.0115 | 0.0136 | -0.848 | 0.3966 |
After dropping the level-two covariate of GDP per capita, and using backward stepwise method to drop AverageTemp*log(T) term that is not statistically significant (P-value =0.257), we have our final level-two model:
{cj=α0+β00AverageTemperaturej+β01PopulationDensityj+β02Distancej+e0jwj=α1+β11PopulationDensityj+β12Distancej+e1j−dj=α2+β20AverageTemperaturej+β21PopulationDensityj+β22Distancej+e2j | (3.4) |
By substituting (3.4) into (2.8), we have the combined final model:
Yij∼Poisson(μij)log(μij)=(α0+e0j)+(α1+e1j)log(Ti)+(α2+e2j)Ti+β00AverageTemperature+β01PopulationDensity+β02Distance+β11PopulationDensity∗log(Ti)+β12Distance∗log(Ti)+β20AverageTemperature∗Ti+β21PopulationDensity∗Ti+β22Distance∗Ti | (3.5) |
The final model is also equivalent to a random-intercept-and-random-slope model.
The values of estimated fixed effects using the above-mentioned final model are given in Table 3. It turns out that all remaining terms are significantly associated with COVID-19 transmission at 0.1 significance level, including the one of our main interests, temperature. Comparing the full model, final model and results in Table 1 from Model 1, we find that bringing the geographic covariate "Distance" makes all GDP per capita related terms insignificant, and dropping GDP per capita related terms does not change much on coefficients of population density related terms. As of our main interest, the sign and magnitude of coefficients related to average temperature effect have changed, compared with results in Table 1.
L1 | L2 Coefficients | Fixed effects | Estimate | Std.Error | z value | P-value |
c | α0 | (Intercept) | -7.3458 | 0.5431 | -13.526 | <2e−16 |
c | β00 | AverageTemperature | -0.7244 | 0.2132 | -3.398 | 0.0007 |
c | β01 | PopulationDensity | 2.2358 | 0.5509 | 4.0580 | 4.94e-05 |
c | β02 | Distance | -1.3180 | 0.5741 | -2.2960 | 0.0217 |
w | α1 | log(day) | 4.6820 | 0.2010 | 23.3060 | <2e−16 |
w | β11 | log(day)*PopulationDensity | -0.5811 | 0.2036 | -2.855 | 0.0043 |
w | β12 | log(day)*Distance | 0.4453 | 0.2047 | 2.175 | 0.0296 |
-d | α2 | day | -0.1331 | 0.0064 | -20.862 | <2e−16 |
-d | β20 | day*AverageTemperature | 0.0070 | 0.0036 | 1.9310 | 0.0534 |
-d | β21 | day*PopulationDensity | 0.0175 | 0.0065 | 2.7040 | 0.0069 |
-d | β22 | day*Distance | -0.0161 | 0.0070 | -2.2960 | 0.0217 |
We also present predicted random effects results from "lme4" package [24] using BLUP method in Table 4. Random effects have a range of -3.98 to 4.22 on intercept, a range from -0.075 to 0.063 on "day" covariate, and a range from -2 to 1.63 on "logday" covariate. We can visualize the ranges of random effects on each of the level-one covariates in Figure 4, and further detect possible outliers of Region Molise, P.A. Trento and Umbria, as indicated in the figure. To check whether our predicted random effects satisfy the assumptions of the multivariate normal distribution, we draw normal probability plots and present results in Figure 5. Our graphs in Figure 5 show that the predicted random effects satisfy the multivariate normality assumption, and possible outliers from normality plots are consistent with the results in box plots.
Region | Intercept (e0) | logday (e1) | day (e2) |
Abruzzo | -1.4778 | 0.3643 | 4.8068e-03 |
Basilicata | -0.1659 | 0.0777 | -5.8952e-03 |
Calabria | 1.0968 | -0.1965 | -1.8788e-05 |
Campania | -3.1115 | 0.7663 | -2.2389e-02 |
Emilia-Romagna | 3.3658 | -1.0811 | 3.0806e-02 |
Friuli Venezia Giulia | 0.6879 | -0.4002 | 1.0400e-02 |
Lazio | -0.4633 | -0.1086 | 9.4084e-03 |
Liguria | -1.1703 | 0.3127 | -5.6459e-03 |
Lombardia | 2.2558 | -0.6270 | 7.4296e-03 |
Marche | 3.7051 | -1.1192 | 1.7932e-02 |
Molise | 4.2204 | -2.0073 | 6.2852e-02 |
P.A. Bolzano | -2.1480 | 0.8520 | -2.8011e-02 |
P.A. Trento | 3.9894 | -1.7144 | 5.7475e-02 |
Piemonte | -1.9840 | 0.8564 | 6.7807e-04 |
Puglia | -1.3040 | 0.4321 | 3.9145e-03 |
Sardegna | -0.5926 | 0.4834 | -2.1216e-02 |
Sicilia | 0.5414 | 0.1143 | -4.0148e-03 |
Toscana | -0.3367 | 0.4356 | -1.3019e-02 |
Umbria | -3.9813 | 1.6267 | -7.5136e-02 |
Valle d'Aosta | -3.6609 | 0.9154 | -2.3201e-02 |
Veneto | 0.9086 | -0.1221 | -3.6045e-03 |
To check the performance of the proposed model we use first days of observations as the training data to construct the model to predict the daily new cases in the rest of the days, and then check the deviation. With the variance-covariance matrix of both fixed effects and random effects given in "lme4" [24] results of our final model, We can further calculate a prediction interval for our proposed model by using R package "merTools" [25]. Figure 6 demonstrates the results using the first 60 days of data to construct the model and then to predict the rest of the days in each region.
Once we sum the fitted and predicted values from every region, we can compare our proposed model with models only using the PLEC function at national level. Based on the result from Figure 7, the proposed approach has less deviation in expected prediction than only using the PLEC function.
Results in Table 3 show that all three estimated effects associated with population density are significant at 0.01 significance level, the effect of "AverageTemperature" is significant at 0.01 significance level, and that associated with the interaction term "day*AverageTemperature" is significant at 0.1 significance level. So we have decided to keep "day*AverageTemperature" term in the model as it is an interaction term of our main interest and its p-value is close to 0.05. In order to link results from combined model to PLEC parameters, we group coefficients in Table 3 by their corresponding level-one parameters. Thus, we can directly interpret these covariates' fixed effects on parameters c, w and −d respectively.
Our results indicates that average temperature is negatively associated with parameter c. Holding other variables constant, the increase of every one scaled unit average temperature will lead to a 0.7244 decrease on parameter c, which downsizes the scale of the gamma curve. Also, due to the fact that average temperature is positively associated with parameter −d, the same increase on average temperature will cause −d to increase 0.0069. As a result, with time parameter T as a multiplier, d∗T will counter off the shrinkage effect from parameter c. Thus, the effect from change of average temperature on mean value of daily case is also related to time predictor, and we can derive its pattern by following approach. Based on (2.4), we set μ0=ec0−d0TTw0 and μ1=ec1−d1TTw1, where μ1 is the new function by only increasing x scaled units average temperature (x>0) while other variables are all held constant, thus, c1−c0=−0.7244x, (−d1)−(−d0)=d0−d1=0.00699x, and w0=w1. Further, we take μ1/μ0=e(c1−c0)+T(d0−d1)=e−0.7244x+0.00699xT. It is clear that μ1>μ0 when −0.7244+0.00699T>0, and it is free from x, which means at day 104, the effect from parameter −d will exceed that from parameter c, and before this, it is always true that μ1<μ0. This pattern is applicable to all regions. Similarly, we can also derive the effect of temperature change on the maximum value of infection and its turning point based on the result in (2.5). We set μmax,0=ec0−w0(w0/d0)w0, Tmax,0=w0/d0, and μmax,1=ec1−w1(w1/d1)w1, Tmax,1=w1/d1. Thus, Tmax,1/Tmax,0=d0/d1=d0/(d0−0.00699x)>1, which means higher average temperature leads to a delayed turning point, and μmax,1/μmax,0=ec1−c0(Tmax,1/Tmax,0)=e−0.7244x(d0/(d0−0.00699x))w0, suggesting that whether or not the ratio is larger than 1 depends on the value of parameter −d and w in each region. However, from the previous derived property, we know that as long as Tmax,0 occurs before the 104th day, μmax,1<μmax,0, which means in every region in Italy, increase of average temperature will reduce maximum infections and postpone the turning point. Similarly, we can derive the properties of effects from population density change. From Table 3, we know that population density is positively associated with parameter c and −d, and negatively associated with parameter w. Therefore, the final effect from population density on mean response depends on a comprehensive results from all three parameters. We assume an increase of x scaled units (x>0) on population density while all other variables are held constant. Thus c1−c0=2.2358x, w1−w0=−0.5811x, and d0−d1=0.0175x. We then derive μ1/μ0=e(c1−c0)+(d0−d1)TTw1−w0=e2.2358x+0.0175xTT−0.5811x. If we set d(μ1/μ0)/dT=0 and solve for T, it can be shown that μ1/μ0 takes its minimal value when T=33.206. Thus, (μ1/μ0)min=e2.8169x33.2057−0.5811x. We can further derive (d(μ1/μ0)/dx)min=0.7815e2.8169x33.2057−0.5811x>0 (strictly increasing) at x's domain, and (μ1/μ0)min=1 when x=0, so it's proved that μ1>μ0 when x>0, which suggests the number of mean infections will always become larger when population density is increased.
Figure 8 demonstrates the pattern illustrated above in Region Lombardia. The pattern is consistent across regions in the country, as shown in Figures 9, 10 and 11.
One of the goals of Covid-19 modelling is to predict the turning point of the transmission curve before it occurs. In fact, PLEC model starts to predict reasonable turning points after detecting a "slowing down" trend due to its mathematical property, meanwhile, our proposed model may make this detection earlier in some regions by borrowing strength from other regions, which is an advantage of mixed-effects model.
In this study, we have examined the association between regional spreading patterns of COVID-19 development in Italy and regional factors in two steps. In the first step, we have symbolized the regional spreading patterns of the daily confirmed cases of COVID-19 in Italy by a special patterned Poisson regression model for repeated daily counts. Our proposed regression model can capture the varying growth and declining patterns as well as turning points among regions in Italy by regional regression parameters. We then incorporate these regional regression parameters with regional factors as a second step to effectively evaluate the affect of regional factors on regional spreading patterns of COVID-19 daily confirmed cases.
Our two level longitudinal model performs better on prediction than models only using the PLEC function. This is because of the fact that two level model can incorporate regional covariates into the modelling approach, increasing the goodness of fit and predictive power. It also gives some mathematical properties linking to the level-one PLEC parameters, inferring that regional covariates average temperature and population density are associated with COVID-19 infection. This methodology could be useful in future epidemiological study when transmission pattern is potentially associated with social or environmental covariates. Neither our modelling approach nor PLEC function alone works well if we use data before the turning point to construct the model, due to the fact that lockdowns policy plays a crucial role affecting the curve. To incorporate this effect, we can use segmented models suggested by Zhang et al. [1] and Wei and Zhang [22]. Our analysis results indicate that geographical patterns perform an important role as it could confound other variables in epidemiological studies. More complicated models specifying geographic correlation could be explored on similar topics. A final point of caution is that this modelling procedure is not a designed experiment, which means that it can only explore associations between potential covariates and response, but can not reveal causal relationship, and there might be more confounding or lurking variables to be found.
This research was supported in part by grants from the Natural Sciences and Engineering Research Council of Canada. The authors thank the editor and three anonymous referees for their helpful comments that greatly improved the presentation of these results.
The authors declare there is no conflicts of interest.
[1] |
X. Zhang, R. Ma, L. Wang, Predicting turning point, duration and attack rate of COVID-19 outbreaks in major Western countries, Chaos Solitons Fract., 135 (2020), 109829. doi: 10.1016/j.chaos.2020.109829
![]() |
[2] | A. Feoli, A. L. Iannella, E. Benedetto, Three pictures of COVID-19 behavior in Italy: similar growth and different degrowth, medRxiv, 2000.05.09.20096149. Doi: https://doi.org/10.1101/2020.05.09.20096149. |
[3] |
M. F. Bashir, B. Ma, Bilal, B. Komal, M. A. Bashir, D. Tan, M. Bashir, Correlation between climate indicators and COVID-19 pandemic in New York, USA, Sci. Total Environ., 728 (2020), 138835. doi: 10.1016/j.scitotenv.2020.138835
![]() |
[4] |
D. N. Prata, W. Rodrigues, P. H. Bermejo, Temperature significantly changes COVID-19 transmission in (sub) tropical cities of Brazil, Sci. Total Environ., 729 (2020), 138862. doi: 10.1016/j.scitotenv.2020.138862
![]() |
[5] |
Y. Wu, W. Jing, J. Liu, Q. Ma, J. Yuan, Y. Wang, et al., Effects of temperature and humidity on the daily new cases and new deaths of COVID-19 in 166 countries, Sci. Total Environ., 729 (2020), 139051. doi: 10.1016/j.scitotenv.2020.139051
![]() |
[6] |
S. Shastri, K. Singh, S. Kumar, P. Kour, V. Mansotra, Time series forecasting of Covid-19 using deep learning models: India-USA comparative case study, Chaos Solitons Fract., 140 (2020), 110227. doi: 10.1016/j.chaos.2020.110227
![]() |
[7] |
R. G. da Silva, M. H. D. M. Ribeiro, V. C. Mariani, L. dos Santos Coelho, Forecasting Brazilian and American COVID-19 cases based on artificial intelligence coupled with climatic exogenous variables, Chaos Solitons Fract., 139 (2020), 110027. doi: 10.1016/j.chaos.2020.110027
![]() |
[8] |
A. K. Sahai, N. Rath, V. Sood, M. P. Singh, ARIMA modelling & forecasting of COVID-19 in top five affected countries, Diabetes Metab. Syndr., 14 (2020), 1419–1427. doi: 10.1016/j.dsx.2020.07.042
![]() |
[9] |
M. H. D. M. Ribeiro, R. G. da Silva, V. C. Mariani, L. dos Santos Coelho, Short-term forecasting COVID-19 cumulative confirmed cases: Perspectives for Brazil, Chaos Solitons Fract., 135 (2020), 109853. doi: 10.1016/j.chaos.2020.109853
![]() |
[10] |
A. Vena, D. R. Giacobbe, A. Di Biagio, M. Mikulska, L. Taramasso, A. De Maria, et. al., Clinical characteristics, management and in-hospital mortality of patients with coronavirus disease 2019 in Genoa, Italy, Clin. Microbiol. Infect., 26 (2020), 1537–1544. doi: 10.1016/j.cmi.2020.07.049
![]() |
[11] |
Z. Ceylan, Estimation of COVID-19 prevalence in Italy, Spain, and France, Sci. Total Environ., 729 (2020), 138817. doi: 10.1016/j.scitotenv.2020.138817
![]() |
[12] |
Q. Yang, J. Wang, H. Ma, X. Wang, Research on COVID-19 based on ARIMA modelΔ—Taking Hubei, China as an example to see the epidemic in Italy, J. Infect. Public Health, 13 (2020), 1415–1418. doi: 10.1016/j.jiph.2020.06.019
![]() |
[13] |
A. Singhal, P. Singh, B. Lall, S. D. Joshi, Modeling and prediction of COVID-19 pandemic using Gaussian mixture model, Chaos Solitons Fract., 138 (2020), 110023. doi: 10.1016/j.chaos.2020.110023
![]() |
[14] | M. Batista, Estimation of the final size of the COVID-19 epidemic, MedRxiv, 2020.02.16.20023606. Doi: https://doi.org/10.1101/2020.02.16.20023606. |
[15] |
H. G. Hong, Y. Li, Estimation of time-varying reproduction numbers underlying epidemiological processes: A new statistical tool for the COVID-19 pandemic, PLOS One, 15 (2020), e0236464. doi: 10.1371/journal.pone.0236464
![]() |
[16] |
A. Bertozzi, E. Franco, G. Mohler, M. B. Short, D. Sledge, The challenges of modeling and forecasting the spread of COVID-19, PNAS, 117 (2020), 16732–16738. doi: 10.1073/pnas.1914072117
![]() |
[17] | K. Cai, W. He, G. Y. Yi, COVID-19 Fatality: A Cross-Sectional Study using Adaptive Lasso Penalized Sliced Inverse Regression, J. Data Sci., 18 (2020), 483–494. |
[18] | Z. S. Ma, A Simple Mathematical Model for Estimating the Inflection Points of COVID-19 Outbreaks, medRxiv, 2020.03.25.20043893. DOI: 10.1101/2020.03.25.20043893. |
[19] | Github, available from: https://github.com/pcm-dpc/COVID-19/tree/master/dati-regioni. |
[20] | Wikipedia, available from: https://en.wikipedia.org/wiki/Regions_of_Italy. |
[21] | Wunderground, available from: https://www.wunderground.com/history. |
[22] |
X. Zhang, An updated analysis of turning point, duration and attack rate of COVID-19 outbreaks in major Western countries with data of daily new cases, Data Brief, 31 (2020), 105830. doi: 10.1016/j.dib.2020.105830
![]() |
[23] |
L. M. Sullivan, K. A. Dukes, E. Losina, An introduction to hierarchical linear modelling, Stat. Med., 18 (1999), 855–888. doi: 10.1002/(SICI)1097-0258(19990415)18:7<855::AID-SIM117>3.0.CO;2-7
![]() |
[24] | D. Bates, M. Mächler, B. Bolker, S. Walker, Fitting Linear Mixed-Effects Models Using lme4, J. Stat. Software, 67 (2015), 1–48. |
[25] | J. E. Knowles, C. Frederick, merTools: Tools for Analyzing Mixed Effect Regression Models, 2020. Available from: https://CRAN.R-project.org/package=merTools. |
1. | Dmytro Chumachenko, Tetiana Dudkina, Tetyana Chumachenko, Plinio Pelegrini Morita, Epidemiological Implications of War: Machine Learning Estimations of the Russian Invasion’s Effect on Italy’s COVID-19 Dynamics, 2023, 11, 2079-3197, 221, 10.3390/computation11110221 |
Coefficients | Fixed effects | Estimate | Std.Error | z value | P-value |
α0 | Intercept | -7.3347 | 0.5248 | -13.977 | <2e−16 |
α1 | log(day) | 4.6780 | 0.1918 | 24.395 | <2e−16 |
α2 | day | -0.1330 | 0.0062 | -21.486 | <2e−16 |
β00 | AverageTemperature | 0.0885 | 0.7353 | 0.120 | 0.9042 |
β01 | PopulationDensity | 2.3096 | 0.5264 | 4.388 | 1.15e-05 |
β02 | GDP per capita | 1.8953 | 0.7234 | 2.620 | 0.0088 |
β10 | log(day)*AverageTemperature | -0.3293 | 0.2689 | -1.225 | 0.2206 |
β11 | log(day)*PopulationDensity | -0.6027 | 0.1923 | -3.134 | 0.0017 |
β12 | log(day)*GDPPerCapita | -0.6909 | 0.2644 | -2.613 | 0.0090 |
β20 | day*AverageTemperature | 0.0160 | 0.0086 | 1.857 | 0.0633 |
β21 | day*PopulationDensity | 0.0185 | 0.0062 | 2.980 | 0.0029 |
β22 | day*GDPPerCapita | 0.0225 | 0.0086 | 2.628 | 0.0086 |
Coefficients | Fixed effects | Estimate | Std.Error | z value | P-value |
α0 | Intercept | -7.3336 | 0.5166 | -14.197 | <2e−16 |
α1 | log(day) | 4.6775 | 0.1897 | 24.659 | <2e−16 |
α2 | day | -0.1330 | 0.0061 | -21.862 | <2e−16 |
β00 | AverageTemperature | 0.2644 | 0.7534 | 0.351 | 0.7256 |
β01 | PopulationDensity | 2.0910 | 0.5314 | 4.157 | 3.22e-05 |
β02 | GDP per capita | 1.1906 | 1.1067 | 1.0760 | 0.2820 |
β03 | Distance | -0.9478 | 1.1468 | -0.827 | 0.4085 |
β10 | log(day)*AverageTemperature | -0.3821 | 0.2767 | -1.381 | 0.1673 |
β11 | log(day)*PopulationDensity | -0.5721 | 0.1951 | -2.932 | 0.0034 |
β12 | log(day)*GDPPerCapita | -0.4770 | 0.4071 | -1.172 | 0.2413 |
β13 | log(day)*Distance | 0.2873 | 0.4213 | 0.682 | 0.4953 |
β20 | day*AverageTemperature | 0.0181 | 0.0088 | 2.055 | 0.0399 |
β21 | day*PopulationDensity | 0.0173 | 0.0063 | 2.761 | 0.0058 |
β22 | day*GDPPerCapita | 0.0134 | 0.0131 | 1.062 | 0.2881 |
β23 | day*Distance | -0.0115 | 0.0136 | -0.848 | 0.3966 |
L1 | L2 Coefficients | Fixed effects | Estimate | Std.Error | z value | P-value |
c | α0 | (Intercept) | -7.3458 | 0.5431 | -13.526 | <2e−16 |
c | β00 | AverageTemperature | -0.7244 | 0.2132 | -3.398 | 0.0007 |
c | β01 | PopulationDensity | 2.2358 | 0.5509 | 4.0580 | 4.94e-05 |
c | β02 | Distance | -1.3180 | 0.5741 | -2.2960 | 0.0217 |
w | α1 | log(day) | 4.6820 | 0.2010 | 23.3060 | <2e−16 |
w | β11 | log(day)*PopulationDensity | -0.5811 | 0.2036 | -2.855 | 0.0043 |
w | β12 | log(day)*Distance | 0.4453 | 0.2047 | 2.175 | 0.0296 |
-d | α2 | day | -0.1331 | 0.0064 | -20.862 | <2e−16 |
-d | β20 | day*AverageTemperature | 0.0070 | 0.0036 | 1.9310 | 0.0534 |
-d | β21 | day*PopulationDensity | 0.0175 | 0.0065 | 2.7040 | 0.0069 |
-d | β22 | day*Distance | -0.0161 | 0.0070 | -2.2960 | 0.0217 |
Region | Intercept (e0) | logday (e1) | day (e2) |
Abruzzo | -1.4778 | 0.3643 | 4.8068e-03 |
Basilicata | -0.1659 | 0.0777 | -5.8952e-03 |
Calabria | 1.0968 | -0.1965 | -1.8788e-05 |
Campania | -3.1115 | 0.7663 | -2.2389e-02 |
Emilia-Romagna | 3.3658 | -1.0811 | 3.0806e-02 |
Friuli Venezia Giulia | 0.6879 | -0.4002 | 1.0400e-02 |
Lazio | -0.4633 | -0.1086 | 9.4084e-03 |
Liguria | -1.1703 | 0.3127 | -5.6459e-03 |
Lombardia | 2.2558 | -0.6270 | 7.4296e-03 |
Marche | 3.7051 | -1.1192 | 1.7932e-02 |
Molise | 4.2204 | -2.0073 | 6.2852e-02 |
P.A. Bolzano | -2.1480 | 0.8520 | -2.8011e-02 |
P.A. Trento | 3.9894 | -1.7144 | 5.7475e-02 |
Piemonte | -1.9840 | 0.8564 | 6.7807e-04 |
Puglia | -1.3040 | 0.4321 | 3.9145e-03 |
Sardegna | -0.5926 | 0.4834 | -2.1216e-02 |
Sicilia | 0.5414 | 0.1143 | -4.0148e-03 |
Toscana | -0.3367 | 0.4356 | -1.3019e-02 |
Umbria | -3.9813 | 1.6267 | -7.5136e-02 |
Valle d'Aosta | -3.6609 | 0.9154 | -2.3201e-02 |
Veneto | 0.9086 | -0.1221 | -3.6045e-03 |
Coefficients | Fixed effects | Estimate | Std.Error | z value | P-value |
α0 | Intercept | -7.3347 | 0.5248 | -13.977 | <2e−16 |
α1 | log(day) | 4.6780 | 0.1918 | 24.395 | <2e−16 |
α2 | day | -0.1330 | 0.0062 | -21.486 | <2e−16 |
β00 | AverageTemperature | 0.0885 | 0.7353 | 0.120 | 0.9042 |
β01 | PopulationDensity | 2.3096 | 0.5264 | 4.388 | 1.15e-05 |
β02 | GDP per capita | 1.8953 | 0.7234 | 2.620 | 0.0088 |
β10 | log(day)*AverageTemperature | -0.3293 | 0.2689 | -1.225 | 0.2206 |
β11 | log(day)*PopulationDensity | -0.6027 | 0.1923 | -3.134 | 0.0017 |
β12 | log(day)*GDPPerCapita | -0.6909 | 0.2644 | -2.613 | 0.0090 |
β20 | day*AverageTemperature | 0.0160 | 0.0086 | 1.857 | 0.0633 |
β21 | day*PopulationDensity | 0.0185 | 0.0062 | 2.980 | 0.0029 |
β22 | day*GDPPerCapita | 0.0225 | 0.0086 | 2.628 | 0.0086 |
Coefficients | Fixed effects | Estimate | Std.Error | z value | P-value |
α0 | Intercept | -7.3336 | 0.5166 | -14.197 | <2e−16 |
α1 | log(day) | 4.6775 | 0.1897 | 24.659 | <2e−16 |
α2 | day | -0.1330 | 0.0061 | -21.862 | <2e−16 |
β00 | AverageTemperature | 0.2644 | 0.7534 | 0.351 | 0.7256 |
β01 | PopulationDensity | 2.0910 | 0.5314 | 4.157 | 3.22e-05 |
β02 | GDP per capita | 1.1906 | 1.1067 | 1.0760 | 0.2820 |
β03 | Distance | -0.9478 | 1.1468 | -0.827 | 0.4085 |
β10 | log(day)*AverageTemperature | -0.3821 | 0.2767 | -1.381 | 0.1673 |
β11 | log(day)*PopulationDensity | -0.5721 | 0.1951 | -2.932 | 0.0034 |
β12 | log(day)*GDPPerCapita | -0.4770 | 0.4071 | -1.172 | 0.2413 |
β13 | log(day)*Distance | 0.2873 | 0.4213 | 0.682 | 0.4953 |
β20 | day*AverageTemperature | 0.0181 | 0.0088 | 2.055 | 0.0399 |
β21 | day*PopulationDensity | 0.0173 | 0.0063 | 2.761 | 0.0058 |
β22 | day*GDPPerCapita | 0.0134 | 0.0131 | 1.062 | 0.2881 |
β23 | day*Distance | -0.0115 | 0.0136 | -0.848 | 0.3966 |
L1 | L2 Coefficients | Fixed effects | Estimate | Std.Error | z value | P-value |
c | α0 | (Intercept) | -7.3458 | 0.5431 | -13.526 | <2e−16 |
c | β00 | AverageTemperature | -0.7244 | 0.2132 | -3.398 | 0.0007 |
c | β01 | PopulationDensity | 2.2358 | 0.5509 | 4.0580 | 4.94e-05 |
c | β02 | Distance | -1.3180 | 0.5741 | -2.2960 | 0.0217 |
w | α1 | log(day) | 4.6820 | 0.2010 | 23.3060 | <2e−16 |
w | β11 | log(day)*PopulationDensity | -0.5811 | 0.2036 | -2.855 | 0.0043 |
w | β12 | log(day)*Distance | 0.4453 | 0.2047 | 2.175 | 0.0296 |
-d | α2 | day | -0.1331 | 0.0064 | -20.862 | <2e−16 |
-d | β20 | day*AverageTemperature | 0.0070 | 0.0036 | 1.9310 | 0.0534 |
-d | β21 | day*PopulationDensity | 0.0175 | 0.0065 | 2.7040 | 0.0069 |
-d | β22 | day*Distance | -0.0161 | 0.0070 | -2.2960 | 0.0217 |
Region | Intercept (e0) | logday (e1) | day (e2) |
Abruzzo | -1.4778 | 0.3643 | 4.8068e-03 |
Basilicata | -0.1659 | 0.0777 | -5.8952e-03 |
Calabria | 1.0968 | -0.1965 | -1.8788e-05 |
Campania | -3.1115 | 0.7663 | -2.2389e-02 |
Emilia-Romagna | 3.3658 | -1.0811 | 3.0806e-02 |
Friuli Venezia Giulia | 0.6879 | -0.4002 | 1.0400e-02 |
Lazio | -0.4633 | -0.1086 | 9.4084e-03 |
Liguria | -1.1703 | 0.3127 | -5.6459e-03 |
Lombardia | 2.2558 | -0.6270 | 7.4296e-03 |
Marche | 3.7051 | -1.1192 | 1.7932e-02 |
Molise | 4.2204 | -2.0073 | 6.2852e-02 |
P.A. Bolzano | -2.1480 | 0.8520 | -2.8011e-02 |
P.A. Trento | 3.9894 | -1.7144 | 5.7475e-02 |
Piemonte | -1.9840 | 0.8564 | 6.7807e-04 |
Puglia | -1.3040 | 0.4321 | 3.9145e-03 |
Sardegna | -0.5926 | 0.4834 | -2.1216e-02 |
Sicilia | 0.5414 | 0.1143 | -4.0148e-03 |
Toscana | -0.3367 | 0.4356 | -1.3019e-02 |
Umbria | -3.9813 | 1.6267 | -7.5136e-02 |
Valle d'Aosta | -3.6609 | 0.9154 | -2.3201e-02 |
Veneto | 0.9086 | -0.1221 | -3.6045e-03 |