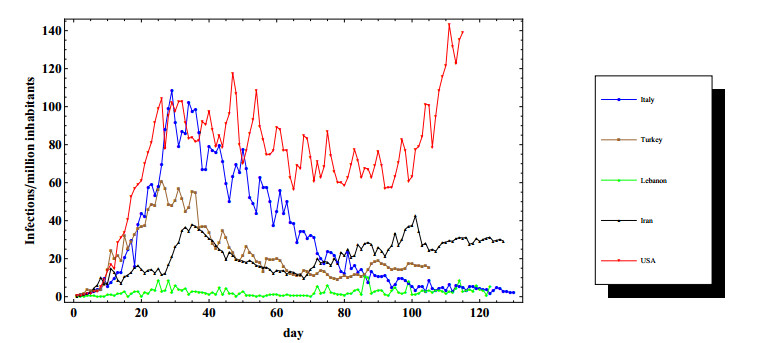
Citation: Omar El Deeb, Maya Jalloul. The dynamics of COVID-19 spread: evidence from Lebanon[J]. Mathematical Biosciences and Engineering, 2020, 17(5): 5618-5632. doi: 10.3934/mbe.2020302
[1] | Hamdy M. Youssef, Najat A. Alghamdi, Magdy A. Ezzat, Alaa A. El-Bary, Ahmed M. Shawky . A new dynamical modeling SEIR with global analysis applied to the real data of spreading COVID-19 in Saudi Arabia. Mathematical Biosciences and Engineering, 2020, 17(6): 7018-7044. doi: 10.3934/mbe.2020362 |
[2] | Sarafa A. Iyaniwura, Musa Rabiu, Jummy F. David, Jude D. Kong . Assessing the impact of adherence to Non-pharmaceutical interventions and indirect transmission on the dynamics of COVID-19: a mathematical modelling study. Mathematical Biosciences and Engineering, 2021, 18(6): 8905-8932. doi: 10.3934/mbe.2021439 |
[3] | Tao Chen, Zhiming Li, Ge Zhang . Analysis of a COVID-19 model with media coverage and limited resources. Mathematical Biosciences and Engineering, 2024, 21(4): 5283-5307. doi: 10.3934/mbe.2024233 |
[4] | Lin Feng, Ziren Chen, Harold A. Lay Jr., Khaled Furati, Abdul Khaliq . Data driven time-varying SEIR-LSTM/GRU algorithms to track the spread of COVID-19. Mathematical Biosciences and Engineering, 2022, 19(9): 8935-8962. doi: 10.3934/mbe.2022415 |
[5] | Ahmed Alshehri, Saif Ullah . A numerical study of COVID-19 epidemic model with vaccination and diffusion. Mathematical Biosciences and Engineering, 2023, 20(3): 4643-4672. doi: 10.3934/mbe.2023215 |
[6] | Ziqiang Cheng, Jin Wang . Modeling epidemic flow with fluid dynamics. Mathematical Biosciences and Engineering, 2022, 19(8): 8334-8360. doi: 10.3934/mbe.2022388 |
[7] | Fen-fen Zhang, Zhen Jin . Effect of travel restrictions, contact tracing and vaccination on control of emerging infectious diseases: transmission of COVID-19 as a case study. Mathematical Biosciences and Engineering, 2022, 19(3): 3177-3201. doi: 10.3934/mbe.2022147 |
[8] | Elodie Yedomonhan, Chénangnon Frédéric Tovissodé, Romain Glèlè Kakaï . Modeling the effects of Prophylactic behaviors on the spread of SARS-CoV-2 in West Africa. Mathematical Biosciences and Engineering, 2023, 20(7): 12955-12989. doi: 10.3934/mbe.2023578 |
[9] | Haonan Zhong, Wendi Wang . Mathematical analysis for COVID-19 resurgence in the contaminated environment. Mathematical Biosciences and Engineering, 2020, 17(6): 6909-6927. doi: 10.3934/mbe.2020357 |
[10] | Sarafa A. Iyaniwura, Rabiu Musa, Jude D. Kong . A generalized distributed delay model of COVID-19: An endemic model with immunity waning. Mathematical Biosciences and Engineering, 2023, 20(3): 5379-5412. doi: 10.3934/mbe.2023249 |
The Coronavirus disease 2019 (COVID-19) has been widely spreading worldwide since it appeared in the city of Wuhan, China towards the end of December, 2019. The World Health Organization classified the spread as a pandemic in March 2020 [1]. Europe and the United States of America have endured the severest repercussions in terms of number of infections and deaths. The US cases amounted to more than one fourth of total global infections by June 2020 [2]. Governmental and institutional reactions and measures varied across countries with respect to the time of introduction of social distancing measures (SDM, henceforth) and with respect to their degree of severity. In spite of some governments being slower in adopting mitigation measures and endorsing the epidemiological concept of herd immunity [3] to create a resistance to the contagion in the long run at the expense of short term losses while keeping the economy functional, the majority adopted SDM's that reached countrywide lockdowns.
A considerable amount of research has been carried out focusing on the dynamics and extent of the pandemic in different countries notably in the countries that witnessed the first cases [4,5,6,7,8]. In comparison with the most recent deadly mass pandemic of the "Spanish flu" that hit the world after World War I during the years of 1918–1919 and recurred in two waves, and caused the death of tens of millions of people [9,10], the extent of the spread of COVID-19 has been far less. The question of the containment of COVID-19 and preventing its spread and expansion into a similar deadly pandemic is a key motivation for the study of various models that describe, simulate and forecast epidemics and dynamics of infections under different reproductive rates and mitigation measures.
In Lebanon, the spread of COVID-19 coincided with a period of political turmoil, few months of popular uprising and economic collapse finally depicted by the default on debts in early March 2020 [11].
Lebanon SDM SDM were adopted at a relatively early stage in Lebanon. The first confirmed case was recorded on February 21, 2020, and consequently all educational institutions were closed starting the first of March. This was followed by a resolution of "Public Mobilization" and ban of public gatherings that imposed closure of all places of worship, shops, restaurants, etc. except for grocery stores and drugstores on March 22 [12]. Another subsequent measure consisted in constraining vehicles' mobility to alternating between odd- and even-ending plate numbers, while fully prohibiting mobility on Sundays. Gradual and partial relaxation started on May 10, but the deep economic crisis helped in slowing down social activity despite the easing of measures. The government decided to open the airport with one-fifth of its normal capacity starting July 1st, after a period of limited returns for Lebanese people living abroad between April 8 and June 30.
The source of the first infection was documented before the international travel ban to be from a traveler coming from Iran [13] where the spread of the virus had started early on [14]. However, it has been discussed that many of the following first cases were transmitted by travelers coming from the Vatican city during the early stages of the pandemic spread there [15]. The efficacy of SDM's can be examined by discerning the daily rate of infection as shown in the daily data of the first 130 days [16]. Our results were obtained in relation to data available until June 30, 2020.
Literature and methodology Models exploring contagion and particularly spread of infections were developed and extensively studied in various fields of mathematics, physics, economics and epidemiology [17,18,19,20,21,22]. Mitigation measures have been shown to be effective in reducing and slowing down the spread of infection by [23,24,25].
The SEIR model [26,27,28] has been widely employed to investigate contagion of diseases including that of COVID-19 recently. In this paper, we establish a novel STEIR model, which consists of modifying the SEIR model to take into account the effect of travel. We use the STEIR and a repeated iterations model presented in [29] (henceforth the RI model) and implement it on the daily data of the Coronavirus in Lebanon provided mainly by the Ministry of Public Health [16] over a duration of 130 days.
The structure of the paper is as follows. In section 2, we present the two models. Results and forecasts are discussed in section 3 and section 4 concludes the paper.
We describe here two models that we use to forecast the path of daily cases and to measure the extent of the epidemic in Lebanon. Following an abrupt rise in infected cases at the start, the rate has fallen to a significantly low level after implementation of severe SDM, in comparison with other regional and country-level data [30] before slowly rising again after partial relaxation. Figure 1 illustrates the progression of the number of daily infections over time in different countries: Lebanon, Turkey, Iran, Italy and the USA. Day 0 represents the date of the first reported infection. Lebanon has a relatively low number of infections per capita, despite the fact that the first case was recorded relatively early. The proposed models accommodate for the actual data and allow for future predictions.
The spread of epidemics can be studied by the prominent SIR model first introduced by Kermack and McKendrick [31], that consists of dividing the population into different compartments and investigating the contagion of the disease by examining the rate of change of the sizes of these groups. Later versions were developed to accommodate for different settings and assumptions [32,33,34]. The STEIR model that we use is described as follows. Consider a population N initially normalized to size 1 and divided into five categories of individuals: susceptible S, incoming travellers T, exposed E, infectious I and removed (through recovery or death) R *. The cumulative number of cases is provided by C=I+R, since each recorded case would at a given time be either infectious or recovered or dead. The rates of change of these categories are given by dSdt,dTdt,dEdt,dIdt and dRdt respectively. The model is formally characterized using the following differential equations:
dSdt=−βtSNI+(1−θ)τ | (2.1) |
dEdt=βtSNI−σE | (2.2) |
dIdt=σE−γI+θτ | (2.3) |
dRdt=γI | (2.4) |
dNdt=τN | (2.5) |
*The exposed individuals represented by E are those who are infected but not yet infectious, as defined in [35]. Recent studies reveal that during incubation period, individuals are not infectious, and infectivity starts on an average of only 1-2 days before symptoms [23].
with βt=Rtγ where βt is the rate at which infected individuals come across others (the SN susceptibles), σ is the rate at which exposed individuals become infected and it is associated to the mean incubation period; γ is the rate of exit by recovery or death per-day and it is associated to the average illness period. In this sense, Rt=βtγ is the ratio of meeting rate to exit rate; it determines the transmission from susceptible to infected and is a proxy for social distancing measures. τ is the daily rate of incoming travellers relative to the total population N and it contributes to both, the infected I and the susceptible S. θ is the average infection rate among the travellers. The population size N slightly increases due to the influx of travellers whose relative contribution is more apparent in the increase in the number of infections I. We neglect the natural changes in the population as the time scale of the epidemic is much faster than that of demographic changes [35].
There are several ways to model Rt. It can be taken as a constant parameter in some contexts, or as a time dependent function as in [26]. Here, we introduce a novel parameterization for Rt. Using data available from the first 130 days in Lebanon, we parameterize Rt by the step function
Rt={R00<t<t1R1t1<t<t2R2t2<t<t3 | (2.6) |
after the application of SDM, before any relaxation, then after partial relaxation at time t2 respectively. t3=130 days, corresponding to the last day of used data. When the measures are changed after this period of SDM, Rt is parameterized as follows:
Rt={R00<t<t1R1t1<t<t2R2t2<t<t3R3t>t3 | (2.7) |
where R0, R1, R2 and R3 are constant parameters that depend on the severity of measures and commitment to those measures. R0 is the reproductive transmission rate of the disease in the initial phase, while R1 and R2 are the reproductive transmission factors under strict and relaxed SDM respectively. R3 represents the potential future rate under different possible relaxation schemes. Using the estimates in [35], we take σ=15.2 in relation to an average period of incubation of 5.2 days. We use γ=120 underlying an average period of recovery (or death) of 20 days. This value is in line with the reports of the World Health Organization that estimate the average time of recovery to be in the range of 2–3 weeks [36]. We can determine τ from Eq (2.5), and we find that
τ=ln(NfNi)Δt | (2.8) |
where Ni is the population on April 8 and Nf is the population on June 30, after the arrival of air passengers. The time Δt is the number of days of incoming flights. This corresponds to τ=2.91×10−5/day. From official data provided in [16], we also find that θ=0.0377 by taking the ratio of all positive cases among arriving travellers to the total number of incoming travellers until June 30, 2020.
Assuming that initially no SDM are applied, R0 represents the transmission of disease with no mitigation measures. In [35] they adopt R0=3.1, while in [37] they take values between 2.76 and 3.25, and [26] considers different values between 3 and 1.6. Recent studies reveal that R0 of COVID-19 can assume higher values up to 5.7 and 6.47 according to data analyzed from China [38,39]. After introduction of measures, Rt can assume values less than 1 in case extremely severe mitigation measures are applied [40]. It was well established that COVID-19 has higher R0 than other infections like SARS [41]. The estimation of R0 and Rt is essential for forecasting the spread, but their determination depends on the available data and the accuracy of the reporting of initial cases and dates.
We assume that the initial value of I is I0=16M, in line with the first initial case in Lebanon reported on February 21 and a gross population of 6 million inhabitants. We take E0=12I0 to account for the fact that the initial case registered had been in contact with many people on a flight from Iran which raises the number of initially exposed people. The passengers were unprotected as they were not aware of the presence of an infection among them until late during the flight [42]. To account for this fact, we took E0 to be triple the value used by [26] where they consider I0=110M with 33 initial cases in the United States whose population stands at around 330 millions, and E0=4I0 given 132 individuals were initially carrying but not contagious, acknowledging the considerable uncertainty related to initial cases in the US. This entails some uncertainty in the initial conditions of the spread due to the lack of readily available data.
By fitting the values of the reproductive number parameters in our model, we find that R0=5.6, R1=0.52 and R2=1.1 for t1=32 days, t2=63 days and t3=130 days respectively, provide the best numerical prediction to account for the actual reported cases. We assume that relaxation of measures would increase the reproductive rate, but that public awareness and remaining measures will keep it well below its initial value. Accordingly, we simulate four possible future scenarios with R3=1.1, 1.4, 1.7 and 2 after t3=130 days. Regarding τ, we inspect four possible cases for t>130 days, upon the opening of Beirut airport, and the expected increase in the number of travellers entering the country. We simulate the effect of τ multiplied by 2, 3 and 4 in comparison to the previous rate of arrivals, and its consequences on the cumulative number of infected cases.
In this section, we adopt an alternative approach, the RI model, which exploits the available daily data of infections and recoveries to forecast progression of the disease. Here we implement a variation of the repeated iterations method proposed in [29]. We denote the active infected daily values by Ii where i is the index of days and i∈[1,n]. We use the last m values of Ii to determine the average arithmetic gross rate in the last m days as
Ga=1mn∑i=n−m+1(IiIi−1−1) | (2.9) |
and the average geometric gross rate of the same set of data as
Gg=(IiIi−m)1m | (2.10) |
Ga and Gg indicate the average rate of increase in the number of current infections during the last m days, using arithmetic and geometric means. We forecast the progression of the number of infections accordingly as follows
Ii+1=Ii(1+Ga) | (2.11) |
using the arithmetic gross infection rate Ga or alternatively
Ii+1=IiGg | (2.12) |
using the geometric gross infection rate Gg.
To account for the removal of active cases, we define the death and recovery rates by p and 1−p respectively, the number of days need for recovery by h and the average number of days between infection and death by d. This implies that on day (i+1), the number of deaths will be p(Ii−d−Ii−d−1) where (Ii−d−Ii−d−1) represents the number of people who caught the virus d days ago. Similarly, the number of people recovered would be proportional to the number of people who caught the virus h days ago and is given by (1−p)(Ii−h−Ii−h−1). The values of p and h vary in the literature and in the available data from the country under consideration. To simulate the available data from Lebanon, we take p=0.04, h=20 days and d=24 days. h is in accordance with the average period of recovery taken in the STEIR model used before, and p and d are discussed in available literature [29].
Given the aforementioned parameters, we define the recursive relation used in our forecast as follows
(Ii+1)net=Ii+1−p(Ii−d−Ii−d−1)−(1−p)(Ii−h−Ii−h−1) | (2.13) |
where (Ii+1)net is the net number of infected people on day (i+1). In every iteration, we use the available data up to the day under consideration, estimate the number of infections on the next day, and reevaluate Ga and Gg accordingly, hence recursively predicting the progression of the rate and the number of active infected cases. We will consider m=14 previous days, and forecast the next upcoming 14 days as well. The interesting feature of the RI model is that as new daily data is revealed, we update our daily future forecast, hence have a new 14 day future expectation every day.
The average gross rate G (the arithmetic rate Ga or the geometric rate Gg) is a dynamic quantity and it is contingent on the promptness and severity of public policies of isolation, curfews and social distancing as well as on the degree of individual commitment and personal preventive efforts e.g. sanitation, use of face masks, etc. For this end, we consider several scenarios to predict the progression of G in relation to SDM. Furthermore, we conduct the simulations using both Ga, Gg, the maximum value of G attained in the previous m days, and possible increases or decreases of those rates.
In the parameterization of the STEIR model based on the cases reported in Lebanon, we find that the initial value of the reproductive transmission factor is remarkably high at R0=5.6. However, it significantly decreases to R1=0.52 at t1=32 days, before rising moderately to R2=1.1 at t2=90 days following a partial relaxation of SDM. This parameterization provides an accurate fit of the registered cases for the first 130 days. In addition to the absence of any SDM, a relatively high value of R0 could be attributed to late reporting or under reporting of the early cases, hence the fast surge of registered results during the first few days of testing. R0 here reflects the fast pace of spread of the disease in the early days before implementing social distancing measures. The rapid fall of the rate from R0 to R1 occurs after the implementation and the social commitment to mitigation measures, hence the rapid decrease in the number of daily infections and the slow increase in the cumulative number of infections. The significantly low rate of R1 sharply diminishes the number of new infections, and the curve of the cumulative number of infections (Figure 2) starts flattening out slowly, before its new rise when the SDM are eased.
However, if the measures are relaxed at a time t3=130 days, we expect an increase in the reproductive rate from R2 to a another constant value R3. The exact value of R3 will still depend on the extent of relaxation and the public commitment to SDM. We consider here four possible values of R3 (Figure 3): continued partial mitigation measures with R3=R2=1.1, a weakly higher relaxation with R3=1.4, while more public social interaction and moderate relaxation has R3=1.7. Finally, we investigate R3=2 which corresponds to a wider relaxation of measures that yet coincides with self-awareness and individual efforts, hence preventing the return to the initial rate R0.
We find that the cumulative number of infections will rise again at a higher pace as depicted in Figure 4 even for the lowest increase in R3. The number of cumulative infections could reach a total varying between 4491 to more than 7042 infections in a period of 50 days, depending on the extent of relaxation. A constant level of SDM of R2 would lead to a total of 3697 infections over the same period. Moreover, we find that even for the low constant value of R3, an increase in the flow of incoming travelers would drive the number of infectious cases upward, depending on the rate of travel over the period of the posterior 50 days (Figure 5). More precisely, the simulation of the first 130 days with absolute closure of the airport and no incoming travelers shows that the cumulative number of infections would have reached 948 instead of the 1778 registered cases on June 30.
This also suggests that the disease can rapidly spread again once the measures are relaxed, at a pace contingent on the degree of relaxation of the measures. A combination of loosened measures and a higher rate of travel arrivals would trigger a stronger second phase of infection. These results are numerically specific to Lebanon, but they can be naturally generalized, and they suggest that a second outbreak of COVID-19 infections would be inevitable in absence or weakening of within as well as cross-country measures.
The arithmetic and geometric means proposed in equations (2.9) and (2.10) assume that all the SDM will remain unchanged over the m subsequent days under consideration. Nevertheless, this might not be the case. In order to take into account possible changes we consider the following scenarios that we simulate in Figure 6:
1. We determine the arithmetic and the geometric means of the last 14 days and the corresponding future forecasts are plotted in brown and black respectively. It is clear that with the rate infection registered in Lebanon, and with more people recovered, the number of currently infected people would slowly increase during the upcoming couple of weeks, and the geometric and arithmetic means considered lead to very similar forecasts.
2. The infection rate decreases by an absolute value of 2% and the number of recovered is also on the rise; hence, the total number of active cases decreases faster.
3. The rate of progression is defined as the maximum of the rates of increase recorded in the past fourteen days
Gmax=Max{Ggi−(m−1),Ggi−(m−2),....Ggi} |
The number of active cases would witness a steep increase despite of recoveries and deaths.
4. The mean rate of infection increases by an absolute rate of 1%, and the number of currently infected people would rise quickly despite recoveries or deaths from previous cases.
Similarly to the forecasts obtained using the STEIR model, the different scenarios are contingent on the imposed governmental measures as well as on public behavior and social distancing. If precautions are diminished, the number of infections will be on the rise again according to scenarios 3 or 4. The continued enforcement of measures would lead to scenario 1 or more optimistically scenario 2 in case of severe SDM and perhaps the resumption of a countrywide lockdown (Figure 6).
The low infection rate recorded in the second month of the COVID-19 spread in Lebanon could likely be attributed to public commitment to social distancing and the strict mitigation measures imposed. Another factor that could have affected this outcome is the number and extent of distribution of tests undertaken.
To address this issue, we compare the number of daily tests conducted per million residents in Lebanon, with that of several other countries that witnessed stronger spreads like Italy, USA, Iran and Turkey. We find that the percentage of people tested in Lebanon is similar to that in Iran, and tends to rise with time but it is significantly less than the other countries as shown in Figure 7. This suggests that the number of detected infections is possibly lower than the actual number of cases.
Furthermore, we check the ratio of the cumulative number of infections with respect to the cumulative numbers of tests conducted. This criterion would give the rate of infected out those of tested, hence eliminates the uncertainty related to under-testing. An examination of the publically available data reveals that the cumulative rate of infection among those who were tested in Lebanon was 1.37% on June 24th, 2020, compared to 8.17% in the USA, 4.63% in Italy, 6.21% in Turkey and 14.04% in Iran [2]. This is an assertion that the low rate of infection is more related to the mitigation measures applied in the country, as rates in more affected countries are larger by several orders of magnitude.
On the other hand, the relaxation of measures and the rise of the reproductive rate of infections due to increased interaction could reinstate a high rate of infections within a couple of weeks as forecasted in Figure 4 and Figure 6. This is confirmed by the simulations conducted based both on the STEIR and the RI models. The aforementioned models both forecast a resumption of a quick spread of the disease and an increase in the number of infected people once the SDM are reduced or abandoned, and once the inflow of travelers commences and increases. We note that the RI model, by construction, already accounts for all infected cases, whether residents or travellers, but it only allows a projection for a short term future behavior of infectious spread.
Our data also depicts that the mean age of infected individuals has fallen from 43.8 years to 36.7 years old between April 8 until June 30, 2020, which might have been generated by the travel inflows during this period.
Under all circumstances, the continued SDM and travel restrictions are essential to keep COVID-19 under control [43] until the introduction of effective medications or vaccines, which is estimated to take at least between 12 to 18 months [44], despite ongoing medical and clinical research around the globe [45].
This paper presented two different models used in the simulation of the spread of infectious diseases which are the STEIR model and the RI model. We developed the STEIR model, a novel parameterization of the reproductive rate of infection, an improved RI model and adjusted all relevant parameters to fit the available data from Lebanon. We analyzed in detail the current spread and different forecasts for potential progressions. We found out that the rate of infection and the number of infected people fell down quickly due to the rapid fall in the reproductive number R due to strong mitigation measures. However, relaxing the measures, partially lifting the travel ban and the resumption of social activity and interaction would swiftly put the infections on a rapid rise again, thus reversing the temporary success in limiting the spread of COVID-19.
The results obtained can be further extended in different directions. At the theoretical level, the suggested models can be enhanced and generalized to include other within and cross-country features of the progression of pandemics. Furthermore, the findings can be exploited to draw policies and establish appropriate measures to limit the spread of the disease.
The authors have no acknowledgements.
The authors declare that they have no conflict of interests.
All data can be supplied by the authors upon request.
[1] | WHO Director-General's opening remarks at the media briefing on COVID-19, World Health Organization, 2020. Available from: https://who.int/dg/speeches/detail/who-director-general-s-opening-remarks-at-the-media-briefing-on-covid-19-11-march-2020. |
[2] | COVID-19 coronavirus pandemic 2020, Worldometer, 2020. Available from: https://www.worldometers.info/coronavirus. |
[3] | I. Bremmer, C. Kupchan, S. Rosenstein, The New York Times, 2020. Available from: https://www.nytimes.com/2020/05/04/opinion/coronavirus-sweden-herd-immunity.html. |
[4] | Q. Li, X. Guan, P. Wu, X. Wang, L. Zhou, Y. Tong, et al., Early transmission dynamics in Wuhan, China, of novel coronavirus-infected pneumonia, N. Engl. J. Med., 382 (2020), 1199-1207. |
[5] | T. Zhou, Q. Liu, Z. Yang, J. Lao, K. Yang, W. Bai, et al., Preliminary prediction of the basic reproduction number of the Wuhan novel coronavirus 2019-nCoV, J. Evid. Based Med., 13 (2020), 3-7. |
[6] | K. Biswas, A. Khaleque, P. Sen, Covid-19 spread: Reproduction of data and prediction using a SIR model on Euclidean network 2020, arXiv, arXiv:2003.07063. |
[7] | C. Yang, J. Yang, A mathematical model for the novel coronavirus epidemic in Wuhan, China, Math. Biosci. Eng., 17 (2020), 2708-2724. |
[8] | D. Fanelli, F. Piazza, Analysis and forecast of COVID-19 spreading in China, Italy and France, Chaos Soliton. Fract., 134 (2020), 109761. |
[9] | J. Taubenberger, D. Morens, 1918 Influenza: the Mother of All Pandemics, Emerging Infect. Dis., 12 (2006), 15-22. |
[10] | H. Philips, The Recent Wave of Spanish Flu Historiography, Soc. Hist. Med., 27 (2014), 789-808. |
[11] | Lebanon to default on debt for first time amid financial crisis, The Guardian, 2020. Available from: https://www.theguardian.com/world/2020/mar/07/lebanon-to-default-on-debt-for-first-time-amid-financial-crisis. |
[12] | Ministry of Public Health Republic of Lebanon, 2020. Available from: https://www.moph.gov.lb/en. |
[13] | A. Tuite, I. Bogoch, R. Sherbo, A. Watts, D. Fisman, K. Khan, Estimation of COVID-2019 burden and potential for international dissemination of infection from Iran, preprint, 2020. Available from: https://www.medrxiv.org/content/10.1101/2020.02.24.20027375v1. |
[14] | Z. Zhuang, S. Zhao, Q. Lin, P. Cao, Y. Lou, L. Yang, et al., Preliminary estimation of the novel coronavirus disease (COVID-19) cases in Iran: A modelling analysis based on overseas cases and air travel data, Int. J. Infect. Dis., 94 (2020), 29-31. |
[15] | D. Gavlak, Latest in a series of crises: Lebanon grapples with COVID-19, National Catholic Reporter, 2020. Available from: https://www.ncronline.org/news/world/latest-series-crises-lebanon-grapples-covid-19. |
[16] | Disaster Risk Management Unit., 2020. Available from: http://drm.pcm.gov.lb. |
[17] | S. Bocaletti, V. Latora, Y. Moreno, M. Chavez, D. Hwang, Complex networks: structure and dynamics, Phys. Rep., 424 (2006), 175-308. |
[18] | R. Pastor-Satorras, C. Castellano, P. Van Mieghem, A. Vespignani, Epidemic processes in complex networks, Rev. Mod. Phys., 87 (2015), 925-979. |
[19] | M. Batista, fitVirusCOVID19, MIT Central File Exchange, 2020. Available from: https://www.mathworks.com/matlabcentral/fileexchange/74658-fitviruscovid19. |
[20] | J. Lou, When Will COVID-19 End? Data-Driven Prediction, Data-Driven Innovation Lab, 2020. Available from: https://ddi.sutd.edu.sg/when-will-covid-19-end/. |
[21] | C. Sun, Y. Hsieh, Global analysis of an SEIR model with varying population size and vaccination, Appl. Math. Model., 34 (2010), 2685-2697. |
[22] |
D. Helbing, D. Brockmann, T. Chadefaux, K. Donnay, U. Blanke, O. Woolley-Meza, et al., Saving Human Lives: What Complexity Science and Information Systems can Contribute, J. Stat. Phys., 158 (2015), 735-781. doi: 10.1007/s10955-014-1024-9
![]() |
[23] | R. Anderson, H. Heesterbeek, D. Klinkenberg, D. Hollingsworth, How will countrybased mitigation measures influence the course of the COVID-19 epidemic?, Lancet, 395 (2020), 931- 934. |
[24] | B. McCloskey, A. Zumla, G. Ippolito, L. Blumberg, P. Arbon, A. Cicero, et al., Mass gathering events and reducing further global spread of COVID-19: a political and public health dilemma, Lancet, 395 (2020), 1096-1099. |
[25] | M. Kraemer, C. Yang, B. Gutierrez, C. Wu, B. Klein, D. Pigott, et al., The effect of human mobility and control measures on the COVID-19 epidemic in China, Science, 368 (2020), 493-497. |
[26] | A. Atkeson, What will be the economic impact of COVID-19 in the US? Rough estimates of disease scenarios, National Bureau of Economic Research, 2020. Available from: https://www.nber.org/papers/w26867. |
[27] | L. Graef, L. Wang, J. Karsai, Global Dynamics of a SEIR model with varying total population size, Math. Bio., 160-2 (1999), 191-213. |
[28] | L. Peng, W. Yang, D. Zhang, C. Zhuge, L. Hong, Epidemic analysis of COVID-19 in China by dynamical modeling, preprint, arXiv:2002.06563. |
[29] | M. Perc, N. Miksic, M. Slavinec, A. Stozer, Forecasting COVID-19, Front. Phys., 8 (2020), 127. |
[30] | H. Ritchie, E. Ortiz-Ospina, D. Beltekian, E. Mathieu, J. Hasell, B. Macdonald, et al., Statistics and Research Coronavirus Disease (COVID-19), Our World in Data, 2020. Available from: https: //www.ourworldindata.org/coronavirus. |
[31] | W. Kermack, A. McKendrick, A contribution to the mathematical theory of epidemics, Proc. R. Soc. A, 115 (1927), 700-721. |
[32] | R. May, R. Anderson, Infectious diseases of humans: dynamics and control, Oxford University Press, Oxford, 1994. |
[33] | V. Capasso, Mathematical Structure of Epidemic Systems, Springer, Berlin, 1993.34. E. Vynnycky, R. White, An Introduction to Infectious Disease Modelling, Oxford University Press, Oxford, 2010. |
[34] | 35. H. Wang, Z. Wang, Y. Dong, R. Chang, C. Xu, X. Yu, et al., Phase-adjusted estimation of the number of Coronavirus Disease 2019 cases in Wuhan, China, Cell Discov., 6 (2020), 10. |
[35] | 36. Report of the WHO-China Joint Mission on Coronavirus Disease 2019 (COVID-19), World Health Organization, 2020. Available from: https://www.who.int/docs/default-source/coronaviruse/who-china-joint-mission-on-covid-19-final-report.pdf. |
[36] | 37. A. Remuzzi, G. Remuzzi, COVID-19 and Italy: what next?, Lancet, 395 (2020), 1225-1228. |
[37] | 38. S. Sanche, Y. Lin, C. Xu, E. Romero-Severson, N. Hengartner, R. Ke, High contagiousness and rapid spread of severe acute respiratory syndrome coronavirus 2, Emerg. Infect. Dis., 26 (2020), 1470-1477. |
[38] | 39. B. Tang, X. Wang, Q. Li, N. Bragazzi, S. Tang, Y. Xiao, et al., Estimation of the Transmission Risk of the 2019-nCoV and Its Implication for Public Health Interventions, J. Clin. Med., 9 (2020), 462. |
[39] | 40. J. Ma, J. Dushoff, B. Bloker, D. Earn, Estimating initial epidemic growth rates, Bull. Math. Biol., 76 (2014), 245-260. |
[40] | 41. Y. Liu, A. Gayle, A. Wilder-Smith, J. Rocklov, The reproductive number of COVID-19 is higher compared to SARS coronavirus, J. Travel Med., 27 (2020), taaa021. |
[41] | 42. N. Houssari, Lebanon confirms first coronavirus case as death toll hits 4 in Iran, Arab News, |
[42] | 2020. Available from: https://www.arabnews.com/node/1631166/middle-eastr. |
[43] | S. Momtazmanesg, H. Ochs, L. Uddin, M. Perc, J. Routes, D.N. Vieiera, et al., All together to Fight COVID-19, Am. J. Trop. Med. Hyg., 102-6 (2020), 1181-1183. |
[44] | N. Akpan, Why a coronavirus vaccine could take way longer than a year, National Geographic, 2020. Available from: https://nationalgeographic.com/science/2020/04/why-coronavirus-vaccine-could-take-way-longer-than-a-year. |
[45] | N. Lurie, M. Saville, R. Hatchett, J. Halton, Developing Covid-19 Vaccines at Pandemic Speed, N. Engl. J. Med., 382 (2020) 1969-1973. |
1. | Omar El Deeb, Spatial Autocorrelation and the Dynamics of the Mean Center of COVID-19 Infections in Lebanon, 2021, 6, 2297-4687, 10.3389/fams.2020.620064 | |
2. | A.R. Appadu, A.S. Kelil, Y.O. Tijani, Comparison of some forecasting methods for COVID-19, 2021, 60, 11100168, 1565, 10.1016/j.aej.2020.11.011 | |
3. | Jacob Burns, Ani Movsisyan, Jan M Stratil, Renke Lars Biallas, Michaela Coenen, Karl MF Emmert-Fees, Karin Geffert, Sabine Hoffmann, Olaf Horstick, Michael Laxy, Carmen Klinger, Suzie Kratzer, Tim Litwin, Susan Norris, Lisa M Pfadenhauer, Peter von Philipsborn, Kerstin Sell, Julia Stadelmaier, Ben Verboom, Stephan Voss, Katharina Wabnitz, Eva Rehfuess, International travel-related control measures to contain the COVID-19 pandemic: a rapid review, 2021, 14651858, 10.1002/14651858.CD013717.pub2 | |
4. | Omar El Deeb, Maya Jalloul, Asep K. Supriatna, Efficacy versus abundancy: Comparing vaccination schemes, 2022, 17, 1932-6203, e0267840, 10.1371/journal.pone.0267840 | |
5. | Jonathan Santos Apolonio, Ronaldo Teixeira da Silva Júnior, Beatriz Rocha Cuzzuol, Glauber Rocha Lima Araújo, Hanna Santos Marques, Isadora de Souza Barcelos, Luana Kauany de Sá Santos, Luciano Hasimoto Malheiro, Vinícius Lima de Souza Gonçalves, Fabrício Freire de Melo, Syndemic aspects between COVID-19 pandemic and social inequalities, 2022, 12, 2222-0682, 350, 10.5662/wjm.v12.i5.350 | |
6. | Xinyu Bai, Shaojuan Ma, Stochastic dynamical behavior of COVID-19 model based on secondary vaccination, 2022, 20, 1551-0018, 2980, 10.3934/mbe.2023141 | |
7. | Jianhui Xu, Yingbin Deng, Ji Yang, Wumeng Huang, Yingwei Yan, Yichun Xie, Yong Li, Wenlong Jing, Effect of Population Migration and Socioeconomic Factors on the COVID-19 Epidemic at County Level in Guangdong, China, 2022, 10, 2296-665X, 10.3389/fenvs.2022.841996 | |
8. | Lama Bou-Karroum, Joanne Khabsa, Mathilda Jabbour, Nadeen Hilal, Zeinab Haidar, Pamela Abi Khalil, Rima Abdul Khalek, Jana Assaf, Gladys Honein-AbouHaidar, Clara Abou Samra, Layal Hneiny, Sameh Al-Awlaqi, Johanna Hanefeld, Fadi El-Jardali, Elie A. Akl, Charbel El Bcheraoui, Public health effects of travel-related policies on the COVID-19 pandemic: A mixed-methods systematic review, 2021, 83, 01634453, 413, 10.1016/j.jinf.2021.07.017 | |
9. | Abbas Hoballah, Rana El Haidari, Ghina Siblany, Fadi Abdel Sater, Samir Mansour, Hamad Hassan, Linda Abou-Abbas, SARS-CoV-2 antibody seroprevalence in Lebanon: findings from the first nationwide serosurvey, 2022, 22, 1471-2334, 10.1186/s12879-022-07031-z | |
10. | M. El Sayed, M. A. El Safty, M. K. El-Bably, Topological approach for decision-making of COVID-19 infection via a nano-topology model, 2021, 6, 2473-6988, 7872, 10.3934/math.2021457 | |
11. | Yoriyuki Yamagata, Shunki Takami, Keisuke Yamazaki, Tomoki Nakaya, Masaki Onishi, 2022, Individual-based epidemiological model of COVID19 using location data, 978-1-6654-8045-1, 4434, 10.1109/BigData55660.2022.10020242 | |
12. | Nancy Fayad, Walid Abi Habib, Ahmed Kandeil, Rabeh El-Shesheny, Mina Nabil Kamel, Youmna Mourad, Jacques Mokhbat, Ghazi Kayali, Jimi Goldstein, Jad Abdallah, SARS-CoV-2 Variants in Lebanon: Evolution and Current Situation, 2021, 10, 2079-7737, 531, 10.3390/biology10060531 | |
13. | Omar El Deeb, Khalid Hattaf, Samer A. Kharroubi, Editorial: Modeling of COVID-19 and other infectious diseases: Mathematical, statistical and biophysical analysis of spread patterns, 2023, 9, 2297-4687, 10.3389/fams.2023.1178479 | |
14. | Omar El Deeb, Antoine Matar, Impact of segregation and integration on infection dynamics: analysis of a Cholera model in a two-population system, 2024, 99, 0031-8949, 105016, 10.1088/1402-4896/ad72b4 | |
15. | Tawil Samah, Identifying health research in the era of COVID-19: A scoping review, 2023, 11, 2050-3121, 10.1177/20503121231180030 | |
16. | Samah Tawil, Shafiul Haque, Pascale Salameh, Mental Health During COVID-19: An Evaluation of Academic Universities’ Contribution to Existing Research, 2024, Volume 17, 1178-2390, 2053, 10.2147/JMDH.S439983 | |
17. | Diane Nauffal, Samah Tawil, Resilience in crisis: A study of Lebanese student engagement across economic, political, and pandemic turmoil, 2024, 0033-1538, 10.1007/s11125-024-09707-7 |