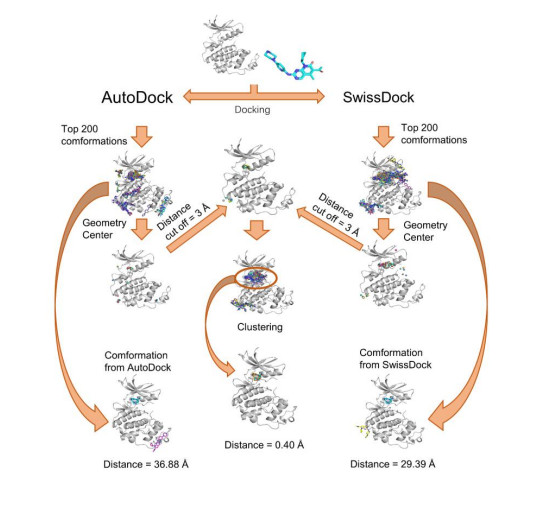
Citation: Linlu Song, Shangbo Ning, Jinxuan Hou, Yunjie Zhao. Performance of protein-ligand docking with CDK4/6 inhibitors: a case study[J]. Mathematical Biosciences and Engineering, 2021, 18(1): 456-470. doi: 10.3934/mbe.2021025
[1] | Hong Yuan, Jing Huang, Jin Li . Protein-ligand binding affinity prediction model based on graph attention network. Mathematical Biosciences and Engineering, 2021, 18(6): 9148-9162. doi: 10.3934/mbe.2021451 |
[2] | Jihong Yang, Hao Xu, Congshu Li, Zhenhao Li, Zhe Hu . An explorative study for leveraging transcriptomic data of embryonic stem cells in mining cancer stemness genes, regulators, and networks. Mathematical Biosciences and Engineering, 2022, 19(12): 13949-13966. doi: 10.3934/mbe.2022650 |
[3] | Jian-Di Li, Gang Chen, Mei Wu, Yu Huang, Wei Tang . Downregulation of CDC14B in 5218 breast cancer patients: A novel prognosticator for triple-negative breast cancer. Mathematical Biosciences and Engineering, 2020, 17(6): 8152-8181. doi: 10.3934/mbe.2020414 |
[4] | Guoxin Hou, Binbin Song . Gastric cancer patient with c-MET amplification treated with crizotinib after failed multi-line treatment: A case report and literature review. Mathematical Biosciences and Engineering, 2019, 16(5): 5923-5930. doi: 10.3934/mbe.2019296 |
[5] | Shun Li, Lu Yuan, Yuming Ma, Yihui Liu . WG-ICRN: Protein 8-state secondary structure prediction based on Wasserstein generative adversarial networks and residual networks with Inception modules. Mathematical Biosciences and Engineering, 2023, 20(5): 7721-7737. doi: 10.3934/mbe.2023333 |
[6] | Yu Jiang, Lijuan Lin, Huiming Lv, He Zhang, Lili Jiang, Fenfen Ma, Qiuyue Wang, Xue Ma, Shengjin Yu . Immune cell infiltration and immunotherapy in hepatocellular carcinoma. Mathematical Biosciences and Engineering, 2022, 19(7): 7178-7200. doi: 10.3934/mbe.2022339 |
[7] | Shuangshuang Wen, Peng Zhao, Siyu Chen, Bo Deng, Qin Fang, Jishi Wang . The impact of MCCK1, an inhibitor of IKBKE kinase, on acute B lymphocyte leukemia cells. Mathematical Biosciences and Engineering, 2024, 21(4): 5164-5180. doi: 10.3934/mbe.2024228 |
[8] | Shequn Gu, Jihui Luo, Wenxiu Yao . The regulation of miR-139-5p on the biological characteristics of breast cancer cells by targeting COL11A1. Mathematical Biosciences and Engineering, 2020, 17(2): 1428-1441. doi: 10.3934/mbe.2020073 |
[9] | Li-Bing Wang, Liang Feng, Jing He, Bo Liu, Jian-Guang Sun . MiR-125a-5p inhibits the proliferation and invasion of breast cancer cells and induces apoptosis by targeting GAB2. Mathematical Biosciences and Engineering, 2019, 16(6): 6923-6933. doi: 10.3934/mbe.2019347 |
[10] | Taniya Mukherjee, Isha Sangal, Biswajit Sarkar, Tamer M. Alkadash . Mathematical estimation for maximum flow of goods within a cross-dock to reduce inventory. Mathematical Biosciences and Engineering, 2022, 19(12): 13710-13731. doi: 10.3934/mbe.2022639 |
The cyclin-dependent kinases 4/6 (CDK4/6) is one of the most important regulators for the cell cycle. Aberrant activity of CDK4/6 may cause uncontrolled cell proliferation, leading to malignant tumor diseases. For example, breast cancer is often associated with the abnormal accumulation of CDK4/6 [1,2]. The identification of the inhibitors would be essential to breast cancer treatment.
The activation of CDK is a three-step process. In the inactive state, T-loop blocks at the entrance of the active site cleft. Thus, the first step is to form a CDK-Cyclin complex to decrease the T-loop flexibility for substrate binding. Second, the Cyclin protein can correctly position the essential amino-acid side chains for phosphorylation. Third, the active CDK-Cyclin complex performs specific functions. For example, the CDK4/6 complex inactivates the RB protein and releases the transcription factor E2F [3]. This process can trigger the up-regulation of E2F response genes, and thereby promoting cell proliferation during the G1/S transition of the cell cycle [4]. Therefore, the CDK4/6 inhibitor can inhibit the RB phosphorylation and arrest the cells in the G1 phase.
Previous research suggested that CDK inhibitors can be categorized into three types (Table S1) [5,6]. The type Ⅰ inhibitors bind to the catalytic pocket in the active state [7,8,9,10]. The type Ⅱ inhibitors also bind to the catalytic pocket but in the inactive state, which is the 'DFG-out' conformation [11,12]. The gatekeeper residues of the catalytic pocket can provide selectivity for type Ⅰ and Ⅱ inhibitors. However, CDKs are highly conserved in both sequence and structure. Most inhibitors can affect the normal cell cycle process and have side effects consequences. The type Ⅲ inhibitors bind to the allosteric sites outside the catalytic pocket and do not compete with ATP binding [13,14]. These inhibitors have high specificity and fewer side effects. Currently, there are three highly selective CDK4/6 inhibitors approved by the Food and Drug Administration (FDA): Palbociclib (PD0332991), Ribociclib (LEE011), and Abemaciclib (LY2835219). These inhibitors have less toxicity to normal cells and side effects on hormone receptor (HR)-positive, human epidermal growth factor receptor 2 (HER2)-negative advanced breast cancer or metastatic breast cancer patients [15,16,17,18,19]. However, the existing inhibitors still have some side effects [19,20,21,22,23]. It is necessary to screen or design more CDK4/6 inhibitors computationally [24].
A ligand may interfere with the protein function by binding to a specific position on the protein. Docking is one of the most promising approaches to determine the protein-ligand complex structure computationally. Most of the docking approaches contain two steps: The first step is to perform a conformational search algorithm for structure sampling. The second step is to provide a scoring function for structure evaluation. Protein-ligand docking [25,26], based on the lock-and-key assumption, uses shape complementary and interface interactions to determine the complex conformation. The electrostatic, van der Waals interactions and hydrogen bonds are essential for the protein-ligand complex formation. The available docking methods can be categorized into shape complementary [27,28,29], Monte Carlo [30,31,32,33], genetic [34,35,36,37,38], and other approaches (Table S2) [39,40]. However, most of the traditional docking methods cannot generate the highly correct native-like protein-ligand conformations. Besides, it is still difficult to evaluate the sampling structures precisely. A new docking strategy is needed. An alternative to protein-ligand prediction is to incorporate the strategy and use the information as well.
In this article, we provide a multi-source information-based protein-ligand docking approach (pmDock). Compare to traditional docking methods, pmDock can obtain a more accurate protein-ligand conformation and improve the docking results. The case study of CDK4/6 inhibitor testing shows the overall performance is better than the original AutoDock and SwissDock methods. The binding residue and energy analysis shows that pmDock can provide more accurate protein-ligand interactions. Furthermore, we applied our method to screen some potential inhibitors for breast cancer. The results show that pmDock has a reliable accuracy for protein-ligand prediction.
To obtain a more precise docking result, we provide one hybrid docking approach by integrating multi-source information to effectively generate more native-like conformations and evaluate the structures more accurately (Figure 1).
AutoDock and SwissDock are the most widely used docking methods [41,42]. In the first step, AutoDock allows the receptor side chains and ligand to rotate the torsional angles for flexible docking [43]. SwissDock treats the receptor as rigid but splits the ligand into fragments. The fragments are then docked and reconstructed to the receptor based on the tree-based sampling algorithm [44].
In the second step, AutoDock uses a semi-empirical scoring function. It uses the Amber force field to evaluate the enthalpic contributions, empirical methods to calculate the solvation and entropy contributions [45]. The force field includes evaluations (V) and an estimate of the conformational entropy lost upon binding (△Sconf):
V=Wvdw∑i,j(Aijr12ij−Bijr6ij)+Whbond∑i,jE(t)(Cijr12ij−Dijr10ij) | (2.1) |
+Welec∑i,jqiqjε(rij)rij+Wsol∑i,j(SiVj+SjVi)e−(r2ij2σ2). |
where Wvdw, Whbond, Welec, Wsol are the weighting constants from dispersion/repulsion, hydrogen bonding, electrostatic, and desolvation obtained by experimental calibration. The first term is a typical 6–12 potential for dispersion/repulsion interactions, where the parameters A and B are taken from the Amber force field [46]. The second term is a directional H-bond term based on a 10–12 potential [47]. And the parameters C and D are assigned to give a maximal well depth of 5 kcal/mol at 1.9 Å for O-H and N-H and a depth of 1 kcal/mol at 2.5 Å for S-H. The directionality of the hydrogen bond interaction E(t) is dependent on the angle t away from ideal bonding geometry. The third item is the electrostatic interactions [48]. The last term is the desolvation potential based on the volume (V) of the atoms surrounding the given atom and is weighted by the solvation parameter (S). In contrast, the exponential term is based on distance [49]. And the distance weighting factor σ is set to 3.5 Å.
The term for the loss of torsional entropy upon binding (△Sconf) is directly proportional to the number of rotatable bonds in the molecule (Ntors):
△Sconf=WconfNtors. | (2.2) |
the Wconf is conformational entropy determined by experimental calibration. The numbers of rotatable bonds include all torsional degrees of freedom.
SwissDock uses the CHARMM force field [42,50,51] to evaluate the valence angle, dihedral angles, and improper angle [52]. Besides, SwissDock uses a harmonic model to consider the improper angle [53].
U(→R)=∑bondsKb(b−b0)2+∑anglesKθ(θ−θ0)2 | (2.3) |
+∑Urey−BradleyKUB(S−S0)2 |
+∑dihedralsKφ(1+cos(nφ−δ)) |
+∑impropersKω(ω−ω0)2 |
+∑non−bondedpairs{εminij[(Rminijrij)12−2(Rminijrij)6]+qiqj4ε0εrij} |
+∑residuesUCMAP(φ,ψ). |
where the potential energy, U(→R), is a sum over individual terms. The parameters Kb, Kθ, KUB and Kφ are their respective force constants, and the variables with a subscript of 0 are their equilibrium values [53]. The first term is bond (b). The second term is valence angles (θ). The third term is Urey−Bradley (UB, S). For three bonded atoms A-B-C, this term is a quadratic function of the distance S between atoms A and C [53]. The fourth term is dihedral angle (φ), n is the multiplicity or periodicity of the dihedral angle and, δ is the phase shift. The fifth item is improper angle(ω). The sixth is Non−bonded terms include Coulombic interactions between the point charges (qi and qj) and the Lennard-Jones (LJ) 6–12 term, which is used for the treatment of the core-core repulsion and the attractive van der Waals dispersion interaction. And εminij is represents the well depth, where i and j are the indices of the interacting atoms, rij is the interatomic distance, and Rminij is the distance at which the LJ term has its minimum [53]. The last term is backbone torsional correction (CMAP,φ,ψ), it is used to perform a numerical correction for the backbone [53].
To improve the docking accuracy, we integrated the AutoDock and SwissDock information in four steps. The detailed workflow of pmDock is as follows. First, we performed structure sampling by using AutoDock and SwissDock. AutoDock produces 2000 conformation samplings while SwissDock produces 5000 binding models. Second, we ranked the docking results and selected the top 200 docking results from both AutoDock and SwissDock. Third, we calculated the distances between the geometry center of the predicted ligands. We removed the ligand conformations in AutoDock (SwissDock) if the distance is larger than 3 Å to the conformations in SwissDock (AutoDock). Then, we further clustered the conformations using the K-Means algorithm [54,55]. The K-Means algorithm is mainly divided into the following steps: (a) Selects an initial K = 6 and initializes their respective cluster centers randomly. (b) Calculates the distances between the ligand geometry centers and cluster centers. The K-Means algorithm divides the ligands into the corresponding cluster according to the closest distance between the ligands and cluster centers. (c) Updates the cluster centers by the average positions of the ligands in each cluster. (d) Repeats the steps (b) and (c) until the sum of squared errors between the empirical average of a cluster and the points in the cluster is minimized among all K clusters:
J(c)=∑Kk=1∑xi∈ck||xi−μk||2 | (2.4) |
J(c) is the sum of the squared error over all K clusters. Let X={xi}, i=1,…,n be the set of n 3-dimensional points clustered into a set of K clusters. C={ck,k=1,…K}, μk is the mean of cluster ck. (e) Sorts the ligands according to the distances between the ligand positions and the cluster center.
The CDK homology sequences were extracted from ConSurf-DB [56,57]. The HMMER [58] is used to search for homologous sequences similar to the CDK structure. Then, the multiple sequence alignment of these homologous sequences is calculated by MAFFT [59]. The position-specific conservation scores of each amino acid position in the alignment were computed using the Rate4Site program [60]. The Rate4Site algorithm assigns a conservation level for reach amino acid using an empirical Bayesian inference. The continuous conservation scores are divided into a discrete scale of 9 grades. Grade 1 indicates the most variable positions. Grade 9 shows the most conserved positions.
We use the FDA approved CDK4/6 inhibitors as the case study dataset. These three inhibitors block the cell cycle progression by inhibiting the hyperphosphorylation of RB protein in sensitive breast cancer cells [2]. Here, we used the available CDK6-inhibitor complex structures to test the performance of pmDock. The inhibitors (Palbociclib, Abemaciclib, and Ribociclib) and corresponding CDK6 structures were extracted from the PDB database (PDB codes: 5L2I, 5L2S, and 5L2T) [61] (Table S3). We used OpenBabelGUI 2.4.1 [62] to convert the PDB files into mol2 files.
The performance of pmDock was tested against traditional AutoDock and SwissDock with the FDA approved breast cancer inhibitors Palbociclib (PD0332991), Ribociclib (LEE011), and Abemaciclib (LY2835219). The experimental CDK6-inhibitor complex structures are shown in Figure 2. These three inhibitors are ATP-competitive compounds to inhibit the CDK6 function and therefore interfere with tumor cells' growth [63]. We compared the three corresponding CDK6 structures (PDB codes: 5L2I, 5L2S, and 5L2T) (Figure S1). The three CDK6 structures' structural differences are very small, with an average RMSD of 0.27 ± 0.06 Å.
The workflow of the case study is divided into the following steps (Figure 3). First, we select the top 200 docking conformations according to the lowest binding energy in AutoDock and SwissDock calculations. We calculated the distances between the predicted ligands' geometry center and removed the conformations if the distance is larger than 3 Å. There are 240,323, and 204 predicted conformations for Palbociclib, Abemaciclib, and Ribociclib. Then, we calculate each ligand's geometric center and re-rank the docking results by using the K-Means clustering algorithm [54,55] (please see section 2.1 for detailed information). We compare the distance between the predicted ligand geometry centers and experiments. The results show that the distance accuracy of pmDock produces a substantial increase of 80 and 373% compare with AutoDock and SwissDock (Table S4). The pmDock is able to get more accurate docking results with the native-like geometry center as the crystal structures.
The inhibitor needs to interact with the critical residues to inhibit the CDK6 function. The prediction of the binding site can provide a better understanding of the protein structure and function [64,65,66,67]. Previous research showed Palbociclib and Abemaciclib bind to the residues of Tyr24, Lys43, Phe98, His100, Asp104, and Thr107 on CDK6 (Figure 4). The Ribociclib binds to the residues of Lys43, Phe98, His100, Asp104, and Thr107 on CDK6 [61]. The average distance of these interactions is 3.69 Å. Therefore, we use a distance of 4 Å to calculate the accuracy of the interactions between the ligands and the above-mentioned critical binding sites. The accuracy is defined as:
accuracy=∑Nn=0|TP||TP|+|FP|nN | (3.1) |
where N is the critical binding sites determined experimentally (Palbociclib = 6, Abemaciclib = 6, and Ribociclib = 5). The n is the number of binding sites. For Palbociclib and Abemaciclib n=0,1,2,…,6. And for Ribociclib n=0,1,2,…,5. TP (FP) represents true positives (false positives). For example, TP (FP) is the total number of ligands whose distance between two binding sites is less (larger) than 4 Å when n = 2.
In the AutoDock calculation, the interaction accuracy of Palbociclib, Abemaciclib, and Ribociclib are 0.78, 0.91, and 0.52 (Table S5A). In the SwissDock, the interaction accuracy of Palbociclib, Abemaciclib, and Ribociclib are 0.26, 0.52, and 0.19 (Table S5B). For pmDock, the accuracy values of Palbociclib, Abemaciclib, and Ribociclib are 0.77, 0.81, and 0.64 (Table S5C). The mean and standard deviation accuracy values of AutoDock, SwissDock, and pmDcok are 0.74 ± 0.20, 0.32 ± 0.17, and 0.74 ± 0.09, respectively (Figure 5a, Table S5D). The results indicate pmDcok can provide accurate interaction predictions with a small standard deviation.
We further calculated the binding energy between CDK6 and the three inhibitors. The experimental binding energies of Palbociclib, Abemaciclib, and Ribociclib are −10.0 kcal mole-1, −9.6 kcal mole-1, and −8.3 kcal mole-1, respectively. First, we compared the differences between the experiment and theoretical predictions. The mean and standard deviation of energy differences of AutoDock, SwissDock, and pmDock are 0.66 ± 0.29, 1.41 ± 0.47, and 0.75 ± 0.15 (Table S6), respectively. The binding energy differences of AutoDock and pmDock are relatively small. We also selected the top 200 pmDock prediction conformations using binding energy (Figure S2). The results show that the average binding energy of pmDock is closer to the experimental binding energy. The low energy difference and highest correlation show that pmDock is able to provide a more accurate structure evaluation.
We performed sequence conservation analysis to infer the structural or functional important residues. The evolutionary conservation scores were identified using the ConSurf-DB. The continuous conservation scores are divided into a discrete scale of 9 grades. Grade 1 indicates the most variable positions, and grade 9 indicates the most conserved positions. The residue conservations of CDK6 are listed in Table S7. There are four conserved residues in the binding sites: Tyr24 (8), Lys43 (9), Phe98 (7), and Asp104 (8). The two non-conserved residues in the binding sites are His100 (5) and Thr107 (5). We calculated the prediction probability of the critical conserved (non-conserved) residues with the following equation:
probability=∑Mm=0|TP||TP|+|FP|mM | (3.2) |
where M is the critical conserved residue (non-conserved residue) determined experimentally (Palbociclib = 4 (2), Abemaciclib = 4 (2), and Ribociclib = 3(2)). The m is the number of conserved residues (non-conserved residue). For Palbociclib and Abemaciclib m=0,1,2,…,4.(0,1,2.). For Ribociclib m=0,1,2,3.(0, 1, 2.). TP (FP) represents true (false) positives. For example, TP (FP) is the number of correct ligands whose distance between two conserved residues (non-conserved residues) is less (larger) than 4 Å when m = 2. The correct ligand refers to the ligand whose distance from the native ligand is less than 4 Å in the previous description.
AutoDock, SwissDock, and pmDock can predict the conserved critical binding residues with a probability of 0.87 ± 0.15, 0.72 ± 0.04, and 0.83 ± 0.12, respectively (Figure 5b, Table S8D). For non-conserved residues, the probability of AutoDock, SwissDock, and pmDock are 0.80 ± 0.18, 0.38 ± 0.06, and 0.70 ± 0.18, respectively (Figure 5b, Table S9D). The evolutionary information needs to be considered in the docking algorithm.
To further validate the accuracy, we calculated the positive predictive value and RMSD. Figure S3a shows that the PPV of pmDock has increased by 9 and 121% compared with original AutoDock and SwissDock. We also performed clustering calculations for pmDock results. The RMSDs of average conformations for the first clusters in pmDock (3.57Å) are much smaller than AutoDock (4.84 Å) and SwissDock (12.37 Å) (shown in Figure S3b). Together, the results demonstrate that the pmDock approach is useful for further studies. We also performed the MM-GBSA calculation to predict the binding energy of the pmDock docking conformations. We filtered out the conformations less than 0 kcal mole-1. Figure S4 shows the energy versus distance accuracy plot of the (a) Palbociclib, (b) Abemaciclib, and (c) Ribociclib predictions. The results show pmDock can provide native-like predictions.
CDK4/6 targeted therapy is one important treatment for HR+/HER2- advanced or metastatic breast cancer [68,69,70]. Computational molecular docking is able to speed up the early-stage development of new drugs. Generally, docking is mainly divided into two steps: structure sampling and scoring function. We have used a multi-source information-based docking approach to improve the prediction accuracy. In the case study of Palbociclib-CDK6, Abemaciclib-CDK6, and Ribociclib-CDK6, pmDock performs consistently better than the traditional AutoDock and SwissDock methods. Therefore, we can use pmDock to screen promising inhibitors that potentially target CDK6. We used the three CDK4/6 inhibitors (Palbociclib, Abemaciclib, and Ribociclib) as references to screen all the non-CDK inhibitors in the ChEMBL DB. The Palbociclib and Ribociclib have three important chemical groups: 3N-pyridine, exocyclic NH of the side chain, and carbonyl. For Abemaciclib, there are two critical chemical groups: exocyclic NH of the side chain and carbonyl (Figure 6) [61,71]. We have screened three potential compounds: CHEMBL272332 [47], CHEMBL205409 [48,49,50] and CHEMBL257665 [47]. These compounds are screened by SwissSimilarity [51] from ChEMBL DB [52,53]. We can obtain their SDF files directly from the ChEMBL DB. We also convert their SDF files into PDB and mol2 files by OpenBabelGUI 2.4.1. The predicted compounds CHEMBL272332 and CHEMBL257665 have the same chemical groups as the reference inhibitors. But CHEMBL205409 only has one similar chemical group: exocyclic NH of the side chain (Figure 7). The similarity scores of CHEMBL272332, CHEMBL205409, and CHEMBL257665 to Palbociclib, Abemaciclib, and Ribociclib are 0.77, 0.41, and 0.22, respectively (Table S10A). The predicted binding probability of CHEMBL272332, CHEMBL205409, and CHEMBL257665 are 1.00, 0.64, and 1.00, respectively (Table S10B). We will conduct biological activity experiments to explore the above compounds' CDK6 inhibition effects in the future.
Here, we provide one multi-source information-based protein-ligand docking approach. The hybrid method is based on two well-known docking methods, AutoDock and SwissDock. Combining these two methods allows us to generate better prediction conformations compared to the original docking methods. The prediction results of pmDock are not very impressive compare to AutoDock, but pmDock can filter out more accurate native-like conformations. Together, pmDock can be implemented for virtual screening and evaluation for the early drug discovery development stage. In the future, we will incorporate additional features as the dynamical motions and co-evolution information to enhance the prediction accuracy.
This work is supported by the NSFC under Grant No. 11704140 (YZ), self-determined research funds of CCNU from the colleges' basic research and operation of MOE CCNU20TS004 (YZ), and Zhongnan Hospital of Wuhan University Science, Technology and Innovation Seed Fund, Project znpy2019068 (JH).
All authors declare no conflicts of interest in this paper.
[1] | S. Pernas, S. M. Tolaney, E. P. Winer, S. Goel, CDK4/6 inhibition in breast cancer: current practice and future directions, Ther. Adv. Med. Oncol., 10 (2018), 1758835918786451. |
[2] |
H. Xu, S. Yu, Q. Liu, X. Yuan, S. Mani, R. G. Pestell, et al., Recent advances of highly selective CDK4/6 inhibitors in breast cancer, J. Hematol. Oncol., 10 (2017), 97. doi: 10.1186/s13045-017-0467-2
![]() |
[3] | S. F. Dowdy, M. Kaulich, Abstract 1304: Cyclin D:Cdk4/6 activates RB by mono-phosphorylation during early G1 phase, Cancer Res., 74 (2014), 1304–1304. |
[4] | A. N. Omstead, D. Matsui, J. E. Kosovec, S. A. Martin, B. A. Jobe, Antitumor efficacy of CDK 4/6 dual inhibitor, abemaciclib, in an esophageal adenocarcinoma model, J. Clin. Oncol., 35 (2017), e15598–e15598. |
[5] |
M. Tutone, A. M. Almerico, Recent advances on CDK inhibitors: An insight by means of in silico methods, Eur. J. Med. Chem., 142 (2017), 300–315. doi: 10.1016/j.ejmech.2017.07.067
![]() |
[6] |
S. Müller, A. Chaikuad, N. S. Gray, S. Knapp, The ins and outs of selective kinase inhibitor development, Nat. Chem. Biol., 11 (2015), 818–821. doi: 10.1038/nchembio.1938
![]() |
[7] | P. Ayaz, D. Andres, D. A. Kwiatkowski, C. C. Kolbe, P. Lienau, G. Siemeister, et al., Conformational adaption may explain the slow dissociation kinetics of roniciclib (BAY 1000394), a yype I CDK inhibitor with kinetic selectivity for CDK2 and CDK9, ACS Chem. Biol., (2016), acschembio.6b00074. |
[8] | T. Dale, P. A. Clarke, C. Esdar, D. Waalboer, O. Adeniji-Popoola, M. J. Ortiz-Ruiz, et al., A selective chemical probe for exploring the role of CDK8 and CDK19 in human disease, Nat. Chem. Biol., 2015. |
[9] | M. Schreuer, V. Kruse, Y. Jansen, B. Neyns, COMBI-rechallenge: a phase II clinical trial on dabrafenib plus trametinib in BRAFV600-mutant melanoma patients who previously experienced progression on BRAF(+MEK)-inhibition, Ann. Oncolo., 27 (2016). |
[10] | R. B. Corcoran, G. S. Falchook, J. R. Infante, O. Hamid, W. A. Messersmith, E. L. Kwak, et al., BRAF V600 mutant colorectal cancer (CRC) expansion cohort from the phase I/II clinical trial of BRAF inhibitor dabrafenib (GSK2118436) plus MEK inhibitor trametinib (GSK1120212), J. Clin. Oncol., 2012. |
[11] |
T. Wang, Z. Yang, Y. Zhang, W. Yan, F. Wang, L. He, et al., Discovery of novel CDK8 inhibitors using multiple crystal structures in docking-based virtual screening, Eur. J. Med. Chem., 129 (2017), 275–286. doi: 10.1016/j.ejmech.2017.02.020
![]() |
[12] |
S. E. Dixon-Clarke, S. N. Shehata, T. Krojer, T. D. Sharpe, F. Von Delft, K. Sakamoto, et al., Structure and inhibitor specificity of the PCTAIRE-family kinase CDK16, Biochem. J., 474 (2017), 699–713. doi: 10.1042/BCJ20160941
![]() |
[13] |
N. Canela, M. Orzaez, R. Fucho, F. Mateo, R. Gutierrez, A. Pineda-Lucena, et al., Identification of an hexapeptide that binds to a surface pocket in cyclin A and inhibits the catalytic activity of the complex cyclin-dependent kinase 2-cyclin A, J. Biol. Chem., 281 (2006), 35942–35953. doi: 10.1074/jbc.M603511200
![]() |
[14] | Orzáez, Guevara, Sancho, Pérez-Payá, Intrinsic caspase-8 activation mediates sensitization of erlotinib-resistant tumor cells to erlotinib/cell-cycle inhibitors combination treatment, Cell Death Dis., 2012. |
[15] |
R. S. Finn, A. Aleshin, D. J. Slamon, Targeting the cyclin-dependent kinases (CDK) 4/6 in estrogen receptor-positive breast cancers, Breast Cancer Res.: BCR, 18 (2016), 17. doi: 10.1186/s13058-015-0661-5
![]() |
[16] |
T. Otto, P. Sicinski, Cell cycle proteins as promising targets in cancer therapy, Nat. Rev. Cancer, 17 (2017), 93–115. doi: 10.1038/nrc.2016.138
![]() |
[17] | L. Spring, A. Bardia, S. Modi, Targeting the cyclin D-cyclin-dependent kinase (CDK) 4/6-retinoblastoma pathway with selective CDK 4/6 inhibitors in hormone receptor-positive breast cancer: rationale, current status, and future directions, Discovery Med., 21 (2016), 65. |
[18] |
M. W. Landis, B. S. Pawlyk, T. Li, P. Sicinski, P. W. Hinds, Cyclin D1-dependent kinase activity in murine development and mammary tumorigenesis, Cancer Cell, 9 (2006), 13–22. doi: 10.1016/j.ccr.2005.12.019
![]() |
[19] | B. Laderian, T. Fojo, CDK4/6 inhibition as a therapeutic strategy in breast cancer: palbociclib, ribociclib, and abemaciclib, Semin. Oncol., (2018), S0093775418300812. |
[20] | S. Parylo, A. Vennepureddy, V. Dhar, P. Patibandla, A. Sokoloff, Role of cyclin-dependent kinase 4/6 inhibitors in the current and future eras of cancer treatment, J. Oncol. Pharm. Pract., (2018), 107815521877090. |
[21] | A. Patnaik, L. S. Rosen, S. M. Tolaney, A. W. Tolcher, J. W. Goldman, L. Gandhi, et al., Efficacy and safety of abemaciclib, an inhibitor of CDK4 and CDK6, for patients with breast cancer, non–small cell lung cancer, and other solid tumors, Cancer Discovery, (2016), 740–753. |
[22] | H. Wang, K. Wang, Z. Guan, Y. Jian, Y. Jia, F. Kashanchi, et al., Computational study of non-catalytic T-loop pocket on CDK proteins for drug development, Chin. Phys. B, 2017. |
[23] | H. W. Wang, Z. Y. Guan, J. D. Qiu, Y. Jia, C. Zeng, Y. J. Zhao, Novel method to identify group-specific non-catalytic pockets of human kinome for drug design, RSC Adv., 4 (2020). |
[24] | Y. Zhao, H. Chen, C. Du, Y. Jian, H. Li, Y. Xiao, et al., Design of tat-activated CDK9 inhibitor, Int. J. Peptide Res. Therapeutics, 25 (2018), 807–817. |
[25] |
A. M. Almerico, M. Tutone, A. Lauria, 3D-QSAR pharmacophore modeling and in silico screening of new Bcl-xl inhibitors, Eur. J. Med. Chem., 45 (2010), 4774–4782. doi: 10.1016/j.ejmech.2010.07.042
![]() |
[26] |
A. M. Almerico, M. Tutone, A. Lauria, Receptor-guided 3D-QSAR approach for the discovery of c-kit tyrosine kinase inhibitors, J. Mol. Model., 18 (2012), 2885–2895. doi: 10.1007/s00894-011-1304-0
![]() |
[27] | Z. Shentu, M. A. Hasan, C. Bystroff, M. J. Zaki, Context shapes: Efficient complementary shape matching for protein-protein docking, Proteins-Struct. Funct. Bioinformatics, 70 (2010), 1056–1073. |
[28] | D. W. Ritchie, Evaluation of protein docking predictions using Hex 3.1 in CAPRI rounds 1 and 2, Proteins: Struct., Funct., Bioinformatics, 2003. |
[29] |
K. Wiehe, B. Pierce, J. Mintseris, W. W. Tong, R. Anderson, R. Chen, et al., ZDOCK and RDOCK performance in CAPRI rounds 3, 4, and 5, Proteins-Struct. Funct. Bioinformatics, 60 (2005), 207–213. doi: 10.1002/prot.20559
![]() |
[30] | A. Caflisch, P. Niederer, M. Anliker, Monte Carlo docking of oligopeptides to proteins, Proteins-Struct. Funct. Bioinformatics, 13 (2010), 223–230. |
[31] | T. N. Hart, R. J. Read, A multiple-start Monte Carlo docking method, J. Mol. Graphics, 13 (2010), 206–222. |
[32] | P. Reigan, W. Guo, D. Siegel, D. Ross, Molecular docking studies investigating the interaction of a series of benzoquinone ansamycin Hsp90 inhibitors with NAD(P)H: quinone oxidoreductase 1 (NQO1), Cancer Res., 66 (2006), 457–457. |
[33] |
C. M. Venkatachalam, X. Jiang, T. Oldfield, M. Waldman, LigandFit: a novel method for the shape-directed rapid docking of ligands to protein active sites, J. Mol. Graphics Model., 21 (2003), 289–307. doi: 10.1016/S1093-3263(02)00164-X
![]() |
[34] | L. Kang, H. L. Li, H. L. Jiang, X. C. Wang, An improved adaptive genetic algorithm for protein–ligand docking, J. Comput. Aided Mol. Des., 23 (2009), 1–12. |
[35] |
F. Sterberg, G. M. Morris, M. F. Sanner, A. J. Olson, D. S. Goodsell, Automated docking to multiple target structures: Incorporation of protein mobility and structural water heterogeneity in AutoDock, Protns Struct. Funct. Bioinformatics, 46 (2002), 34–40. doi: 10.1002/prot.10028
![]() |
[36] |
G. Jones, P. Willett, R. C. Glen, A. R. Leach, R. Taylor, Development and validation of a genetic algorithm for flexible docking, J. Mol. Biol., 267 (1997), 727–748. doi: 10.1006/jmbi.1996.0897
![]() |
[37] |
H. Jing, X. Zhou, X. Dong, J. Cao, H. Zhu, J. Lou, et al., Abrogation of Akt signaling by Isobavachalcone contributes to its anti-proliferative effects towards human cancer cells, Cancer Lett., 294 (2010), 167–177. doi: 10.1016/j.canlet.2010.01.035
![]() |
[38] |
H. Li, C. Li, C. Gui, X. Luo, K. Chen, J. Shen, et al., GAsDock: a new approach for rapid flexible docking based on an improved multi-population genetic algorithm, Bioorg. Med. Chem. Lett., 14 (2004), 4671–4676. doi: 10.1016/j.bmcl.2004.06.091
![]() |
[39] | G. Culletta, A. M. Almerico, M. Tutone, Comparing molecular dynamics-derived pharmacophore models with docking: a study on CDK-2 inhibitors, Chem. Data Collect., 2020. |
[40] | P. N. Sekhar, Software for molecular docking: a review, Biophys. Rev., 9 (2016), 91–102. |
[41] |
Z. Bikadi, E. Hazai, Application of the PM6 semi-empirical method to modeling proteins enhances docking accuracy of AutoDock, J. Cheminformatics, 1 (2009), 1–16. doi: 10.1186/1758-2946-1-1
![]() |
[42] | A. Grosdidier, V. Zoete, O. Michielin, SwissDock, a protein-small molecule docking web service based on EADock DSS, Nucleic Acids Res., 39 (2011), W270–W277. |
[43] | G. M. Morris, R. Huey, W. Lindstrom, M. F. Sanner, A. J. Olson, AutoDock4 and AutoDockTools4: Automated docking with selective receptor flexibility, J. Comput. Chem., 30 (2010), 2785–2791. |
[44] |
A. Grosdidier, V. Zoete, O. Michielin, Fast docking using the CHARMM force field with EADock DSS, J. Comput. Chem., 32 (2011), 2149–2159. doi: 10.1002/jcc.21797
![]() |
[45] | R. Huey, G. M. Morris, A. J. Olson, D. S. Goodsell, A semi-empirical free energy force field with charge-based desolvation, J. Comput. Chem., 28 (2010), 1145–1152. |
[46] |
S. J. Weiner, P. A. Kollman, D. A. Case, U. C. Singh, C. Ghio, G. Alagona, et al., A new force field for molecular mechanical simulation of nucleic acids and proteins, J. Am. Chem. Soc., 106 (1984), 765–784. doi: 10.1021/ja00315a051
![]() |
[47] |
P. J. Goodford, A computational procedure for determining energetically favorable binding sites on biologically important macromolecules, J. Med. Chem., 28 (1985), 849–857. doi: 10.1021/jm00145a002
![]() |
[48] | E. L. Mehler, T. Solmajer, Electrostatic effects in proteins: comparison of dielectric and charge models, Protn. Eng., (1991), 903–910. |
[49] |
G. M. Verkhivker, D. Bouzida, D. K. Gehlhaar, P. A. Rejto, S. Arthurs, A. B. Colson, et al., Deciphering common failures in molecular docking of ligand-protein complexes, J. Comput.-Aided Mol. Des., 14 (2000), 731–751. doi: 10.1023/A:1008158231558
![]() |
[50] | B. R. Brooks, R. E. Bruccoleri, B. D. Olafson, D. J. States, M. Karplus, CHARMM: A program for macromolecular energy, minimization, and dynamics calculations, J. Comput. Chem., 4 (2010), 187–217. |
[51] |
A. Grosdidier, V. Zoete, O. Michielin, Fast docking using the CHARMM force field with EADock DSS, J. Comput. Chem., 32 (2011), 2149–2159. doi: 10.1002/jcc.21797
![]() |
[52] |
H. J. C. Berendsen, J. R. Grigera, T. P. Straatsma, The missing term in effective pair potentials, J. Phys. Chem., 91 (1987), 6269–6271. doi: 10.1021/j100308a038
![]() |
[53] |
B. R. R. Brooks, C. L. B. Brooks, A. D. Mackerell, L. Nilsson, M. J. Karplus, CHARMM: the biomolecular simulation program, J. Comput. Chem., 30 (2009), 1545. doi: 10.1002/jcc.21287
![]() |
[54] | J. A. Hartigan, M. A. Wong, A K-Means clustering algorithm, Appl. Stats., 28 (1979). |
[55] |
A. K. Jain, Data clustering: 50 years beyond K-means, Pattern Recognit. Lett., 31 (2010), 651–666. doi: 10.1016/j.patrec.2009.09.011
![]() |
[56] | A. B. Chorin, G. Masrati, A. Kessel, A. Narunsky, ConSurf‐DB: An accessible repository for the evolutionary conservation patterns of the majority of PDB proteins, Protein Sci., 29 (2020). |
[57] |
O. Goldenberg, E. Erez, G. Nimrod, N. Ben-Tal, The ConSurf-DB: pre-calculated evolutionary conservation profiles of protein structures, Nucleic Acids Res., 37 (2009), D323–D327. doi: 10.1093/nar/gkn822
![]() |
[58] |
M. Jaina, R. D. Finn, S. R. Eddy, B. Alex, P. Marco, Challenges in homology search: HMMER3 and convergent evolution of coiled-coil regions, Nucleic Acids Res., 41 (2013), e121–e121. doi: 10.1093/nar/gkt263
![]() |
[59] |
K. Kazutaka, D. M. Standley, MAFFT multiple sequence alignment software version 7: improvements in performance and usability, Mol. Biol. Evol., 30 (2013), 772–780. doi: 10.1093/molbev/mst010
![]() |
[60] | P. Tal, R. E. Bell, M. Itay, G. Fabian, B. T. Nir, Rate4Site: an algorithmic tool for the identification of functional regions in proteins by surface mapping of evolutionary determinants within their homologues, Bioinformatics, (2002), S71. |
[61] |
P. Chen, N. V. Lee, W. Hu, M. Xu, B. W. Murray, Spectrum and degree of CDK drug interactions predicts clinical performance, Mol. Cancer Therapeutics, 15 (2016), 2273. doi: 10.1158/1535-7163.MCT-16-0300
![]() |
[62] |
N. M. O'Boyle, M. Banck, C. A. James, C. Morley, G. R. Hutchison, Open babel: an open chemical toolbox, J. Cheminformatics, 3 (2011), 33. doi: 10.1186/1758-2946-3-33
![]() |
[63] |
H. Wang, J. Qiu, H. Liu, Y. Xu, Y. Jia, Y. Zhao, HKPocket: human kinase pocket database for drug design, BMC Bioinformatics, 20 (2019), 617. doi: 10.1186/s12859-019-3254-y
![]() |
[64] | K. Wang, Y. Jian, H. Wang, C. Zeng, Y. Zhao, RBind: computational network method to predict RNA binding sites, Bioinformatics, 34 (2018). |
[65] |
Y. Jian, X. Wang, J. Qiu, H. Wang, Z. Liu, Y. Zhao, C. Zeng, DIRECT: RNA contact predictions by integrating structural patterns, BMC Bioinformatics, 20 (2019), 497. doi: 10.1186/s12859-019-3099-4
![]() |
[66] |
H. Wang, Y. Zhao, RBinds: a user-friendly server for RNA binding site prediction, Comput. Struct. Biotechnol. J., 18 (2020), 3762–3765. doi: 10.1016/j.csbj.2020.10.043
![]() |
[67] |
H. Wang, Y. Zhao, Methods and applications of RNA contact prediction, Chin. Phys. B, 29 (2020), 108708. doi: 10.1088/1674-1056/abb7f3
![]() |
[68] | K. Rascon, G. Flajc, C. De Angelis, X. Liu, M. V. Trivedi, E. Ekinci, Ribociclib in HR+/HER2- advanced or metastatic breast cancer patients, Ann. Pharmacotherapy, 2019. |
[69] | R. J. Cersosimo, Cyclin-dependent kinase 4/6 inhibitors for the management of advanced or metastatic breast cancer in women, (2019), 1183–1202. |
[70] |
A. F. D. Groot, C. J. Kuijpers, J. R. Kroep, CDK4/6 inhibition in early and metastatic breast cancer: A review, Cancer Treatment Rev., 60 (2017), 130–138. doi: 10.1016/j.ctrv.2017.09.003
![]() |
[71] |
M. Poratti, G. Marzaro, Third-generation CDK inhibitors: A review on the synthesis and binding modes of Palbociclib, Ribociclib and Abemaciclib, Eur. J. Med. Chem., 172 (2019), 143–153. doi: 10.1016/j.ejmech.2019.03.064
![]() |
![]() |
![]() |
1. | Shangbo Ning, Huiwen Wang, Chen Zeng, Yunjie Zhao, Prediction of allosteric druggable pockets of cyclin-dependent kinases, 2022, 23, 1467-5463, 10.1093/bib/bbac290 | |
2. | Xiaoman Zhao, Xue Wang, Zhou Jin, Rujing Wang, A normalized differential sequence feature encoding method based on amino acid sequences, 2023, 20, 1551-0018, 14734, 10.3934/mbe.2023659 |