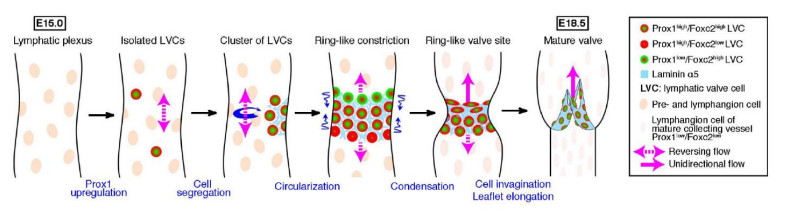
A majority of lymphatic valves tend to form in proximity to vessel junctions, and it is often proposed that disturbed flow at junctions creates oscillating shear stress that leads to accumulation of transcription factors which bring about valvogenesis at these sites. In images of networks of dorsal skin lymphatics from embryonic mice (day E16), we compared simulated fluid flow patterns and observed distributions of the transcription factor Prox1, which is implicated in valve formation. Because of creeping-flow conditions, flow across vessel junctions was not 'disturbed', and within a given vessel, shear stress varied inversely with local conduit width. Prox1 concentration was indeed localised to vessel end-regions, but over three networks was not consistently correlated with the vessel normalised-distance distribution of either fluid shear stress or shear-stress axial gradient. These findings do not support the presently accepted mechanism for the role of flow in valve localisation.
Citation: Christopher D. Bertram, Bernard O. Ikhimwin, Charlie Macaskill. Modeling flow in embryonic lymphatic vasculature: what is its role in valve development?[J]. Mathematical Biosciences and Engineering, 2021, 18(2): 1406-1424. doi: 10.3934/mbe.2021073
[1] | Benchawan Wiwatanapataphee, Yong Hong Wu, Thanongchai Siriapisith, Buraskorn Nuntadilok . Effect of branchings on blood flow in the system of human coronary arteries. Mathematical Biosciences and Engineering, 2012, 9(1): 199-214. doi: 10.3934/mbe.2012.9.199 |
[2] | Lijian Xu, Lekang Yin, Youjun Liu, Fuyou Liang . A computational study on the influence of aortic valve disease on hemodynamics in dilated aorta. Mathematical Biosciences and Engineering, 2020, 17(1): 606-626. doi: 10.3934/mbe.2020031 |
[3] | Aftab Ahmed, Javed I. Siddique . The effect of magnetic field on flow induced-deformation in absorbing porous tissues. Mathematical Biosciences and Engineering, 2019, 16(2): 603-618. doi: 10.3934/mbe.2019029 |
[4] | B. Wiwatanapataphee, D. Poltem, Yong Hong Wu, Y. Lenbury . Simulation of Pulsatile Flow of Blood in Stenosed Coronary Artery Bypass with Graft. Mathematical Biosciences and Engineering, 2006, 3(2): 371-383. doi: 10.3934/mbe.2006.3.371 |
[5] | Oualid Kafi, Nader El Khatib, Jorge Tiago, Adélia Sequeira . Numerical simulations of a 3D fluid-structure interaction model for blood flow in an atherosclerotic artery. Mathematical Biosciences and Engineering, 2017, 14(1): 179-193. doi: 10.3934/mbe.2017012 |
[6] | Fazal Abbas, Rangarajan Sudarsan, Hermann J. Eberl . Longtime behavior of one-dimensional biofilm models with shear dependent detachment rates. Mathematical Biosciences and Engineering, 2012, 9(2): 215-239. doi: 10.3934/mbe.2012.9.215 |
[7] | Li Cai, Yu Hao, Pengfei Ma, Guangyu Zhu, Xiaoyu Luo, Hao Gao . Fluid-structure interaction simulation of calcified aortic valve stenosis. Mathematical Biosciences and Engineering, 2022, 19(12): 13172-13192. doi: 10.3934/mbe.2022616 |
[8] | Fan He, Minru Li, Xinyu Wang, Lu Hua, Tingting Guo . Numerical investigation of quantitative pulmonary pressure ratio in different degrees of stenosis. Mathematical Biosciences and Engineering, 2024, 21(2): 1806-1818. doi: 10.3934/mbe.2024078 |
[9] | Alberto M. Gambaruto, João Janela, Alexandra Moura, Adélia Sequeira . Sensitivity of hemodynamics in a patient specific cerebral aneurysm to vascular geometry and blood rheology. Mathematical Biosciences and Engineering, 2011, 8(2): 409-423. doi: 10.3934/mbe.2011.8.409 |
[10] | Eric Salgado, Yanguang Cao . Pharmacokinetics and pharmacodynamics of therapeutic antibodies in tumors and tumor-draining lymph nodes. Mathematical Biosciences and Engineering, 2021, 18(1): 112-131. doi: 10.3934/mbe.2021006 |
A majority of lymphatic valves tend to form in proximity to vessel junctions, and it is often proposed that disturbed flow at junctions creates oscillating shear stress that leads to accumulation of transcription factors which bring about valvogenesis at these sites. In images of networks of dorsal skin lymphatics from embryonic mice (day E16), we compared simulated fluid flow patterns and observed distributions of the transcription factor Prox1, which is implicated in valve formation. Because of creeping-flow conditions, flow across vessel junctions was not 'disturbed', and within a given vessel, shear stress varied inversely with local conduit width. Prox1 concentration was indeed localised to vessel end-regions, but over three networks was not consistently correlated with the vessel normalised-distance distribution of either fluid shear stress or shear-stress axial gradient. These findings do not support the presently accepted mechanism for the role of flow in valve localisation.
Disturbed or oscillating flow has been proposed as an important trigger of lymphatic intravascular valvogenesis [1,2,3], but while there is support for this idea from cell cultures exposed to oscillating shear, direct evidence from in vivo is lacking. The idea arises both from the long-observed localisation of a majority of lymphatic valves to vessel bifurcations [4,5,6] and from detailed past work on blood flow at the human carotid arterial bifurcation [7,8,9,10,11,12], as summarised by Hahn & Schwartz [13]. According to the prevailing view, disturbed flow in the vicinity of embryonic lymphatic vessel junctions causes raised or oscillatory shear stress exerted by the fluid on the wall at those locations. This in turn is the trigger for the expression of several transcription factors2 involved in the creation of intravascular lymphatic valves, including Prox1, Foxc1, Foxc2, Cx37, Nfatc1 and Gata2 [1,14,15].
2 Transcription factors regulate gene expression, the use of information from a gene to synthesize a product such as a protein.
The gestation period of laboratory mice is approximately 20 days. Lymphatic intravascular valve formation starts between embryonic developmental days 15.5 and 16.5 with cell clusters expressing transcription factors [1,16]. The process of valvogenesis was divided into several postulated stages by Sabine et al. [1], as shown in Figure 1. Valve localisation is here shown as occurring when reversing flow, a feature of disturbed flow, causes isolated valve-forming cells to express Prox1 and Foxc2. Later, a cluster of such cells forms, and later still, the vessel becomes locally constricted, before the actual structure of a lymphatic valve forms. The whole process of building a valve is completed between embryonic days E15 and E18.5. In the later stages, the developing valve is itself responsible for changing the local vessel geometry and hence the flow. However, the site of the valve is determined before then, and any investigation of what determines valve location must look at the vessel geometry and flow that exists before the valve. Hence, we model the flow in networks of lymphatic vessels at embryonic day 16 (hereafter, E16), when valvogenic transcription factors have started to accumulate at what will eventually be valve sites but the vessel has not yet changed physically (Figure 1).
While disturbed flow certainly occurs at high flow speeds in large blood vessels where the Reynolds number is of order several hundred, this does not mean that the creeping lymph flow in tiny mouse lymphatic vessels, where the Reynolds number is small, is capable of exhibiting the same behaviour. To illustrate this, and to explain the concept of disturbed flow in general, Figure 2 shows simulations in two dimensions of flow through a channel having the shape of the human carotid bifurcation. The channel geometry precludes the spiraling flow that can occur in a tubular geometry, but in other respects the flow patterns depicted are those that occur in the real three-dimensional anatomy. In the upper panel, the Reynolds number of the flow is appropriate to blood flow through that particular vessel branching. On the upper wall of the bifurcation, the flow is seen to separate, i.e., there is a zone of recirculation, as outlined by the double arrow. The waveform of inlet arterial blood flow-rate along the common carotid is highly pulsatile. In consequence the separation zone varies in size and slightly in centre position during each cardiac cycle. As a result, there will be places at each end of the eddy where the wall experiences both positive and negative shear, varying cyclically. For our purpose this is a sufficient working definition of disturbed flow: still laminar, but leading to oscillatory wall shear in places.
In contrast, the lower panel of Figure 2 shows flow through the same geometry at a Reynolds number of 1. This typifies flow in mature collecting lymphatic vessels [17]; in embryonic lymphatics we can expect the Reynolds number to be one order of magnitude smaller again. The eddy does not occur, and (characterised by another dimensionless number, the Womersley number) the flow is also fluid-dynamically quasi-steady. The places along the wall that were previously subject to disturbed flow are now no longer distinguished from the rest. There may still be variations in the wall shear, if the inlet flow-rate varies with time, and even shear reversal if the inlet flow reverses itself, but in that case all parts of the wall will experience shear reversal. Disturbed flow is absent, but flow everywhere in the conduit may move back and forth under the impulsion of events elsewhere.
This realization was the starting point for this investigation. Previous work on valvogenesis in lymphatic vessels [1,18] has assumed the presence of locally disturbed flow at what will eventually become valve sites, whereas these universal fluid-mechanical considerations indicate that valve sites are unlikely to be so distinguished. Since embryonic lymphatics are impractical for direct flow measurement in situ, we devised a methodology which combined embryological lymphatic imaging, computational fluid dynamics (CFD) and assumptions about the circumstances of the flow. From the images we obtained the outlines of the vessels and the disposition of their networks, plus the local concentration everywhere of Prox13, a transcription factor implicated in valvogenesis. We assumed that all the imaged vessels lay in one plane, and we assumed that creeping flow through the vessel networks would have been impelled by linear pressure gradients of unknown orientation. We used CFD to simulate these flows and predict the spatial distributions of fluid shear stress, using the fact that, because of the low Reynolds number of the embryonic lymphatic flow, such distributions would be independent of the unknown actual flow magnitudes. We then devised methods to examine the correlation of Prox1 distribution and shear stress distribution across all vessels in each network, making use of vessel length normalisation to allow the combination of results from all the individual vessels of a network while still distinguishing vessel end-regions from mid-regions.
3 Prox stands for Prospero-related homeobox; Prox1 is a protein of homeodomain structure encoded by a gene having the same name.
In principle there can also be flow-derived signals other than shear stress magnitude which could determine the distribution of valve-forming transcription factors. In the context of blood vessels, where localisation of atherosclerosis has been a strong driver of investigations, much attention has also been paid to shear stress gradients. In cell culture, Dolan et al. [19] showed that positive shear stress gradients hindered endothelial cell alignment with flow while negative gradients with comparable shear stress levels promoted it. Dolan et al. [20] found differential gene expression by endothelial cells under positive and negative streamwise gradients of high wall shear stress. Seeking to explain how these effects might be mediated, Dolan et al. [21] suggested that a positive gradient of wall shear stress along a vessel would act to stretch the endothelium locally, and vice versa. However, this mechanism has not been examined numerically, and it is hard to see how the net stretch arising from fluid shear stress variation over the length of an endothelial cell would be significant relative to the effects of shear stress itself. In the lymphatic context, Surya et al. [22] found that the duration of individual calcium-ion pulses in lymphatic endothelial cells increased when a shear-stress gradient was present in vitro, while the same group found that a shear-stress gradient caused lymphatic endothelial cells to orientate perpendicular to the flow, also in vitro [23]. Thus, the theoretical possibility of shear-stress gradient involvement has to be entertained, and accordingly we have here examined localised distribution of both shear stress and its axial gradient.
The rest of this paper is structured as follows. Methods are described in section 2 in two sub-sections, one dealing with the processing applied to stained vessel-network images, the other, the further processing applied to data from those images once reduced to values averaged across vessel diameters. The results of the investigation are given in section 3, and discussed in section 4. Finally, the overall conclusions are restated briefly in section 5.
We obtained images of E164 mouse dorsal skin lymphatic networks separately stained for the presence of the transcription factors Prox1, Lyve1 and Vegfr35; the images were produced by Dr Amélie Sabine at University of Lausanne using standard biological methods [24]. Starting from the image formed by overlay of all three stainings (Figure 3), we manually segmented (delineated the silhouette of) each of the vessels to create two-dimensional (2D) networks (Figure 4). Images having 512 × 512 pixels of three different skin areas were processed; these will be referred to as images (or networks) 1, 2 and 3 in what follows (B1-3 in plot surtitles).
4 There is uncertainty in staging embryonic development, both because the exact time when it starts is not known and because embryos in the same litter can develop at different speeds. Therefore the most precise statement possible is that embryos were collected between E16.0 and E16.5 (Sabine, personal communication).
5 Lyve1 stands for lymphatic vessel endothelial hyaluronan receptor 1, Vegfr3 for vascular endothelial growth factor receptor 3.
The vessel network outlines so created were used as the rigid bounding walls for a simulation of steady creeping laminar flow across the network in the plane of the 2D image. The term creeping implies that the Reynolds number ρ−ud/μ of the simulated flow was everywhere < < 1; here ρ = fluid density, d = local vessel diameter, −u = axial velocity averaged over the cross-section, and μ = fluid viscosity. Under these circumstances, the flow patterns are fully dominated by viscous forces, and self-similar both spatially and temporally as the actual flow-rate is varied. We have no information on the actual flow-rate in each vessel in vivo; we simply assume such conditions to have existed, on the grounds of what is known about lymphatic flow in mature animals, plus the certainty that flow would have been minimal in these barely formed vessels at this early stage of development. Even though we do not know the actual flow patterns, as long as the assumption of sufficiently low Reynolds number is correct, we can be confident on fundamental fluid-mechanical grounds that the simulated patterns, i.e., flow velocity fields, are the same as those which would have existed in the unknown 'real' flow, except for a constant of proportionality which applies across the whole image. The same reason justifies simulating steady flow, in that, while the actual flow may have varied in time, it would at all times have been fluid-dynamically quasi-steady.
However, a second radical step has been taken in the process. The assumptions built into the 2D flow simulation convert the outlines of the vessels to channels of nominally infinite depth normal to the plane of the image. The original biological vessels would have been basically tubular, albeit irregular in shape. The biological laboratory processes leading to the stained image necessarily flatten these tubes, such that the segmented vessel outlines theoretically have a width which is half the vessel circumference (noting that there is no guarantee that the vessels would have been distended to circular cross-section by positive transmural pressure in vivo). This means that the vessel outlines may be up to a factor of π/2 wider than their original diameter, relative to the distances between vessels.
Flow is simulated through this network of channels by finite-element solution of the equations of steady mass and momentum conservation
˜∇⋅˜u=0 |
ρ(˜u⋅˜∇)˜u=−˜∇p+μ∇2˜u |
where, in (x,y)-space, ˜∇=(∂/∂x,∂/∂y), ˜u=(u,v), u(x,y) and v(x,y) are orthogonal velocity components, and p(x,y) is pressure; u=v=0, i.e., the no-slip condition, is imposed at all channel walls. If the Reynolds number were higher, such that the inertia of the fluid significantly influenced the flow, conversion to channel flow would give rise to significant changes in the flow patterns, for instance abolishing any prospect of streamlines spiraling along the vessel. However, in the context of creeping (fully viscous) flow, the channel flow and the tube flow are qualitatively similar.
To complete the simulation, pressure or flow-rate boundary conditions (BCs) must be imposed wherever there is an open inlet or outlet, i.e., wherever any network vessel enters or leaves an edge of the image, or terminates within the image. In separate analyses, terminations within the image were assumed to be either genuine cul-de-sacs, or vessels that continued out of the image plane (and therefore demanded appropriate BCs)6.
6 Both explanations are entirely plausible, because cul-de-sacs are quite common in developing vessels of both blood capillary and lymphatic phenotype, and all initial lymphatic networks begin with blind-ended twig vessels. Kelly-Goss et al. [25] have also documented the existence of entirely isolated lymphatic island vessels.
For the BCs, flow was assumed to be impelled by relative tissue motions giving rise to extrinsic pressure gradients imposed over a length scale much larger than the size of the image (1.53 mm side length). This allowed the assumption that pressure varied linearly across the image. Since the predominant direction in which lymph would have flowed through the network in vivo is not known, 2D flow patterns were computed for both vertical and horizontal imposed pressure gradients, as shown schematically on the right of Figure 5, using the finite-element software ADINA-CFD (ADINA R & D, Inc., Watertown MA). We further assumed for these simulations that all vessels had impermeable walls, on the basis that mature secondary valves are mostly found in collecting lymphatics which are impermeable. With the actual flow speeds unknown, the pressure gradient magnitude was set to yield flow with low Reynolds number everywhere (as argued above, this state certainly characterises these embryonic vessels).
The velocity distributions were used in post-processing in ADINA to compute a scalar output measure of the fluid shear stress distribution everywhere in the vessels, namely the maximum shear stress, defined as the largest of the shear stress tensor components. From these computed shear stress patterns, diameter-averaged (i.e., averaged across the vessel at each axial location) fluid shear stress vs. axial position was calculated in all network vessels, using custom software created in Matlab (The Mathworks, Inc., Natick MA). The analysis was conducted for 12 flows (3 embryo skin lymphatic images7, ×2 orthogonal directions of applied pressure gradient, ×2 assumptions about internal vessel ends, i.e., either cul-de-sacs or in/outlets). For each vessel, diameters were constructed normal to the local centre-line (Figure 6), extending as far as the limits of the vessel outline (Figures 4 and 6), giving the whole-network set of diameters shown in Figure 7. The image quantity determining pixel intensity was then averaged over the pixels forming each diametral line. This method was applied to two separate images for each analysis, one showing the distribution of intensity of Prox1 staining (Figure 7), and the other, the distribution of maximum fluid shear stress, again rendered as a monochromatic intensity (Figure 8).
7 In fact network 3 was segmented with internal in/outlets twice, once excluding (network 3m) and the second time including (network 3x) faintly imaged vessels, giving a total of 14 flows analysed.
As segmented with internal cul-de-sacs, networks 1 to 3 had 163, 92 and 86 vessels; re-segmented with internal in/outlets by a different author, networks 1 and 2 had 176 (as shown in Figure 6) and 94 vessels. As mentioned above, network 3 was segmented with internal in/outlets twice, once excluding (network 3m) and the second time including (network 3x) faintly imaged vessels, causing the segmentation to yield either 94 or 113 vessels. Because of the low Reynolds number, the shear stress (SS)8 distribution at all locations along all vessels/channels reflected essentially fully developed laminar flow velocity profiles, with SS maximal at each side and varying with axial position along a given vessel inversely with the local channel width (Figure 8). Because the flow was 2D, there were no circumferential secondary-flow velocity components; such flows were also proscribed by the low Reynolds number. Also because of the latter, flow across vessel junctions had no special characteristics such as disturbed flow.
8 In this paper, henceforth SS or the words shear stress will be used to mean specifically the maximum fluid shear stress as defined earlier (largest component of the local fluid shear stress tensor).
Network SS distributions with orthogonal pressure gradients showed that some vessels were favoured by flow irrespective of impulsion direction (cf. left and right panels of Figure 8). For all three imaged networks, a majority of vessels showed positive correlation between SS distributions for left/right and top/bottom pressure gradients: 79.9, 75.8 and 75.0% of vessels for networks 1-3 respectively when segmented with internal cul-de-sacs. Irrespective of the direction of application of the pressure gradient, high SS tended to occur where vessels were locally narrow.
Axial shear-stress gradient (SSG) was also calculated from the diameter-averaged SS vs. vessel position data, by differencing successive SS data along each vessel. The profiles of Prox1, SS and SSG vs. position for each vessel were smoothed by moving-average (MA) filtering. Several different lengths (as defined by n1, the either-side number of included pixels) of MA window were computed in each case.
The computed patterns of SS distribution (Figure 8) or SSG distribution were then compared with the intensity distribution of Prox1 staining, in terms of profiles of Prox1 and SS (or SSG) vs. axial position along each vessel, with or without MA-smoothing (see the left panel of Figure 9 for an example). The extent of the correlation between the Prox1 profile and the SS profile in individual vessels varied greatly, as is shown by the histogram of correlation coefficients (right panel of Figure 9); in a substantial minority of the vessels, the two profiles were anti-correlated. Similarly, because of this great variability, the correlation between diameter-averaged Prox1 and diameter-averaged SS over all axial locations in all vessels in a network was low for all three networks (not shown).
Further progress demanded a method of distinguishing, across all vessels, the junction regions of a vessel from other parts. We now consider network 2 (see Figure 10), as it has the fewest vessels of the three images available and so provides greater clarity in the following explanation. Normalised axial position along a vessel was defined by dividing axial position by the vessel length. For all vessels, the profiles of intensity (Prox1 and SS or SSG) were then plotted vs. normalised position (0 to 1) as in Figure 11. Vessels joining two junctions occupy the whole span of normalised length, and are plotted in black in Figure 11. Because a significant fraction of all the vessels in a network image did not join two junctions, it was necessary to organize a rational way to include such vessels in the analysis. If a vessel did not end at a junction, it was allocated to one or other half of the normalized vessel length, as shown in Figure 11. For instance, a vessel which entered at the left-hand edge of the image (red circle in Figure 10) was treated as half of a vessel extending further to the left, and plotted between normalised positions 0.5 and 1 (red line in Figure 11). Similarly, for the segmentation with internal in/outlets, a vessel with a within-image apparent termination was treated as half of a vessel extending further but above or below the imaged plane. In such cases, the relative positions of the pixels at each end of the vessel determined whether it was allocated to left-continuing (red circle, red line) or right-continuing (blue square, blue line) half-vessels. For want of a viable alternative, within-image terminations viewed as cul-de-sacs were similarly treated, even though the whole vessel was deemed to be visible.
The diameter-averaged (and optionally MA-smoothed) data for all vessels were then deposited into 50 bins of normalised position along the vessel. The average of all data (Prox1 intensity, or SS, or SSG) in each bin was found, and second-order polynomials were fitted to these averages as functions of normalised position. Finally, the extent of the correlation between the bin-averaged Prox1 data and the bin-averaged SS (or SSG) data was found.
For each network, orientation of applied pressure gradient, and intra-image termination assumption, the whole of the above analysis was carried out for several different values of the MA-smoothing parameter n1. The final correlations between binned Prox1 and SS data, or Prox1 and SSG data, first increased with window length, then flattened out, then tended to decrease again if n1 was excessive. The results reported below are for the correlation that reached the greatest significance (smallest p-value, indicating least likely to occur by chance) in each case, i.e., over all values of n1 and both left-right and top-bottom pressure-gradient application.
With position normalised as described above, all inter-vessel junctions in a network image occur at normalised positions 0 and 1. As shown for network 1 in the left panel of Figure 12, the height of Prox1 intensity bins averaging all vessels was greater near normalised positions 0 and 1 than near normalised position 0.5 (the mid-point of all complete vessels, and the assumed mid-point of all incomplete vessels). Correspondingly, the fitted second-order polynomial displays a minimum near normalised position 0.5, and is high at 0 and 1. This reflects the known fact that Prox1 is localized to junction regions at day E16. The same result was achieved in all three networks analysed (Figure 12).
The more interesting distributions are those of the computed shear stress (SS) and shear stress gradient (SSG). In network 1, the spatial distribution of smoothed SS (left panel, Figure 13) was qualitatively similar to that of Prox1 (left panel, Figure 12), i.e., when averaged across all vessels in the network, shear stress was consistently high at or near vessel junctions. The resemblance between these distributions suggests what is shown explicitly in the right panel of Figure 13, namely, that in network 1 the along-vessel distribution of SS was highly significantly (p < 1.6 x 10−9) correlated with that of Prox1 intensity.
However, this concordance did not hold up across all three networks. In network 2, Prox1 was not significantly correlated with either SS or SSG. There was still a tendency for shear stress to increase near vessel junctions (Figure 14, left panel), but it was outweighed by high shear stress occurring near the middle of vessels. The reason for this was that many vessels in network 2 narrowed at intermediate points along their length. Consequently, the only correlation between shear stress and Prox1 distributions that reached significance was a negative one (see Table 1A): low shear stress correlated with high Prox1, and vice versa.
(A) shear stress | |||||
image | segm. | p/g dir. | n1 | r2 | p |
1 | cul-de-sac | LR | 4 | 0.2195 | ** |
in/outlets | UD | 10 | 0.5354 | *** | |
2 | cul-de-sac | LR | 4 | 0.1823 | ** |
in/outlets | LR | 8 | 0.0693 | - | |
3 | cul-de-sac | LR | 6 | 0.0505 | - |
3m | in/outlets | UD | 4 | 0.0830 | * |
3x | in/outlets | LR | 6 | 0.0178 | - |
(B) shear stress gradient | |||||
image | segm. | p/g dir. | n1 | r2 | p |
1 | cul-de-sac | LR | 0 | 0.0099 | - |
in/outlets | UD | 2 | 0.0128 | - | |
2 | cul-de-sac | LR | 0 | 0.0102 | - |
in/outlets | LR | 0 | 0.0244 | - | |
3 | cul-de-sac | LR | 4 | 0.3334 | *** |
3m | in/outlets | UD | 2 | 0.0994 | * |
3x | in/outlets | UD | 4 | 0.1684 | ** |
In network 3, the correlation of Prox1 intensity with raised shear stress barely reached significance; with vertical (U-D) pressure-gradient, the correlation attained a minimally significant p-value of 0.0424 before returning to insignificance again as the extent of moving-average filtering was increased. Instead, Prox1 intensity was very significantly correlated with the spatial distribution of smoothed shear stress gradient, for all three segmentations of this image (Figure 15; see also Table 1B). The significance of the correlation varied with the segmentation, being greatest (least likely to arise by chance, p = 1.13 × 10−5) when internal cul-de-sacs were assumed.
The notion that oscillatory shear stress is involved in triggering the accumulation of valve-forming transcription factors in developing lymphatic vessels is directly supported only by evidence from cell culture experiments. As applied in vivo, it depends on the untested hypothesis of 'disturbed' flow at junctions, using ideas taken from large-vessel blood flow mechanics [26]. As outlined in the Introduction, the hypothesis is fatally weakened by considering the mechanics of flow in embryonic lymphatic vessels. The flow is viscous-dominated to an extent which precludes localised disturbed flow as a feature of how the fluid navigates the network junctions. Reversing flow may well exist, since at the time-point in question valves do not yet exist to enforce one-way flow, and there is negligible lymph transport by intrinsic pumping (the combination of contraction of muscular vessel walls and effective operation of valves). Such lymph transport as there is will be the result of relative motions of surrounding tissues, and is equally likely in either direction. But the reversals will occur everywhere; they will not be confined to junction regions. Reversing flow in this sense is perfectly compatible with the fluid-dynamic definition of quasi-steady flow. Accordingly we have simulated only quasi-steady viscous flow, which sets up flow patterns which are reversible; they are unchanged by a change of sign. What this means for the present investigation is that shear stress distributions will not vary qualitatively or geographically as the magnitude of flow varies with time. The exclusion of oscillatory shear stress as a localisation factor throws attention back onto the spatial variation of shear stress magnitude.
The fact that in all three networks Prox1 was found localised to vessel junctions, in agreement with known lymphatic biology, shows that the analysis scheme devised and used here has the power to discern from the images whether a given variable is globally higher or lower in the vicinity of vessel junctions. By the prevailing view, and taking into account the above ruling-out of oscillation, a similar localisation of raised shear stress to vessel junctions should have been found consistently in all three networks. However, this was not the case. We compared diameter-averaged fluid shear stress (and its axial gradient) vs. axial position in all network vessels with the corresponding Prox1 staining intensity data. Neither shear stress nor shear stress gradient was consistently correlated with Prox1. This suggests that Prox1 expression in valve-forming regions is in reality independent of both shear stress and shear stress gradient. It has long been suspected that Prox1 localisation is not directly controlled by flow; Sabine et al. [1] found that reversing flow in vitro upregulated Foxc2, and attributed the expression of Prox1 to unknown factors. However, Prox1 and Foxc2 co-localise very precisely at valve locations, so the findings here in relation to Prox1 apply also to Foxc2, and still leave the nature of the putative flow signal that is mechano-sensed uncertain.
In drawing conclusions from this study, one must bear in mind the rather large assumptions inherent in the methodology:
● reduction of 3D networks of vessels, imaged in 2D, to 2D channel networks,
● linear variation of in/outlet pressure with distance across the network,
● low-Reynolds-number quasi-steady flow,
● vessels which appear/disappear mid-image all assumed either cul-de-sacs or in/outlets,
● all vessels have impermeable walls.
Furthermore, at this time the analysis has been applied to only three small windows of murine dorsal skin. Clearly more data are needed, and we are in process of obtaining same through new collaborators. Thus this report must be viewed as preliminary only. Ideally, the subject should be reinvestigated using 3D data on the network architecture, vessel dimensions, and valve-forming transcription factor distribution, and with measurement of actual lymph flow velocities. However, at the present time these conditions are unobtainable, and the methods applied here provide the only evidence in existence so far from in vivo on the subject of valve localisation during valvogenesis.
Because of creeping-flow conditions in peripheral lymphatic vessels at the time when valves first start to form, flow across vessel junctions is not 'disturbed', contrary to the prevailing view. Over three different vessel networks at this developmental time-point, we found that the concentration of Prox1, a transcription factor involved in valvogenesis, was indeed localised to vessel end-regions, but was not consistently correlated with the vessel normalised-distance distribution of either fluid shear stress or shear-stress axial gradient. These findings raise doubt about the prevailing consensus on the role of flow in determining the eventual locations of intravascular lymphatic valves.
BI was supported by a scholarship from NIH grant U01-HL-123420, which also funded the research. The embryo mouse dorsal skin images were prepared and supplied by Dr Amélie Sabine and Professor Tatiana Petrova (Department of Oncology, Centre Hospitalier Universitaire Vaudois and University of Lausanne, Epalinges, Switzerland), whom we also thank for many useful discussions.
The authors have no conflict of interest to declare.
[1] |
A. Sabine, Y. Agalarov, H. M-E. Hajjami, M. Jaquet, R. Hägerling, C. Pollmann, et al., Mechanotransduction, PROX1, and FOXC2 cooperate to control connexin37 and calcineurin during lymphatic-valve formation, Dev. Cell, 22 (2012), 430-445. doi: 10.1016/j.devcel.2011.12.020
![]() |
[2] |
L. Planas-Paz, E. Lammert, Mechanical forces in lymphatic vascular development and disease, Cell. Mol. Life Sci., 70 (2013), 4341-4354. doi: 10.1007/s00018-013-1358-5
![]() |
[3] |
D. Choi, E. Park, E. Jung, B. Cha, S. Lee, J. Yu, et al., Piezo1 incorporates mechanical force signals into the genetic program that governs lymphatic valve development and maintenance, JCI Insight, 4 (2019), e125068-1-e125068-15. doi: 10.1172/jci.insight.125068
![]() |
[4] |
O. F. Kampmeier, The genetic history of the valves in the lymphatic system of man. Am. J. Anat., 40 (1928), 413-457. doi: 10.1002/aja.1000400302
![]() |
[5] |
E. Bazigou, J. T. Wilson, J. E. Moore jr., Primary and secondary lymphatic valve development: molecular, functional and mechanical insights, Microvasc. Res., 96 (2014), 38-45. doi: 10.1016/j.mvr.2014.07.008
![]() |
[6] |
D. T. Sweet, J. M. Jiménez, J. Chang, P. R. Hess, P. Mericko-Ishizuka, J. Fu, et al., Lymph flow regulates collecting lymphatic vessel maturation in vivo, J. Clin. Invest., 125 (2015), 2995-3007. doi: 10.1172/JCI79386
![]() |
[7] |
B. K. Bharadvaj, R. F. Mabon, D. P. Giddens, Steady flow in a model of the human carotid bifurcation. Part I——Flow visualization, J. Biomech., 15 (1982), 349-362. doi: 10.1016/0021-9290(82)90057-4
![]() |
[8] |
B. K. Bharadvaj, R. F. Mabon, D. P. Giddens, Steady flow in a model of the human carotid bifurcation. Part Ⅱ——Laser Doppler anemometer measurements, J. Biomech., 15 (1982), 363-378. doi: 10.1016/0021-9290(82)90058-6
![]() |
[9] |
D. N. Ku, D. P. Giddens, Pulsatile flow in a model carotid bifurcation, Arteriosclerosis, 3 (1983), 31-39. doi: 10.1161/01.ATV.3.1.31
![]() |
[10] |
D. N. Ku, D. P. Giddens, Laser Doppler anemometer measurements of pulsatile flow in a model carotid bifurcation, J. Biomech., 20 (1987), 407-421. doi: 10.1016/0021-9290(87)90048-0
![]() |
[11] |
D. N. Ku, D. P. Giddens, D. J. Phillips, D. E. Strandness jr., Hemodynamics of the normal human carotid bifurcation: in vitro and in vivo studies, Ultrasound Med. Biol., 11 (1985), 13-26. doi: 10.1016/0301-5629(85)90003-1
![]() |
[12] |
D. N. Ku, D. P. Giddens, C. K. Zarins, S. Glagov, Pulsatile flow and atherosclerosis in the human carotid bifurcation, Arteriosclerosis, 5 (1985), 293-302. doi: 10.1161/01.ATV.5.3.293
![]() |
[13] |
C. Hahn, M. A. Schwartz, Mechanotransduction in vascular physiology and atherogenesis, Nat. Rev. Mol. Cell Bio., 10 (2009), 53-62. doi: 10.1038/nrm2596
![]() |
[14] |
J. Kazenwadel, K. L. Betterman, C. E. Chong, P. H. Stokes, Y. K. Lee, G. A. Secker, et al., GATA2 is required for lymphatic vessel valve development and maintenance, J. Clin. Invest., 125 (2015), 2979-2994. doi: 10.1172/JCI78888
![]() |
[15] |
P. R. Norden, A. Sabine, Y. Wang, C. S. Demir, T. Liu, T. V. Petrova, et al., Shear stimulation of FOXC1 and FOXC2 differentially regulates cytoskeletal activity during lymphatic valve maturation, eLife, 9 (2020), e53814-1-e53814-35. doi: 10.7554/eLife.53814
![]() |
[16] |
C. Norrmén, K. I. Ivanov, J. Cheng, N. Zangger, M. Delorenzi, M. Jaquet, et al., FOXC2 controls formation and maturation of lymphatic collecting vessels through cooperation with NFATc1, J. Cell Biol., 185 (2009), 439-457. doi: 10.1083/jcb.200901104
![]() |
[17] |
J. E. Moore jr., C. D. Bertram, Lymphatic system flows, Annu. Rev. Fluid Mech., 50 (2018), 459-482. doi: 10.1146/annurev-fluid-122316-045259
![]() |
[18] | A. Sabine, T. V. Petrova, Interplay of mechanotransduction, FOXC2, connexins, and calcineurin signaling in lymphatic valve formation. In: Developmental Aspects of the Lymphatic Vascular System (eds. F Kiefer, S Schulte-Merker) Advances in Anatomy, Embryology and Cell Biology, vol 214. Springer-Verlag, Wien, (2014), pp 67-80.doi:10.1007/978-3-7091-1646-3_6 |
[19] |
J. M. Dolan, H. Meng, S. Singh, R. Paluch, J. Kolega, High fluid shear stress and spatial shear stress gradients affect endothelial proliferation, survival, and alignment, Ann. Biomed. Eng., 39 (2011), 1620-1631. doi: 10.1007/s10439-011-0267-8
![]() |
[20] |
J. M. Dolan, H. Meng, F. J. Sim, J. Kolega, Differential gene expression by endothelial cells under positive and negative streamwise gradients of high wall shear stress, Am. J. Physiol. Cell Physiol., 305 (2013), C854-C866. doi: 10.1152/ajpcell.00315.2012
![]() |
[21] |
J. M. Dolan, J. Kolega, H. Meng, High wall shear stress and spatial gradients in vascular pathology: a review, Ann. Biomed. Eng., 41 (2013), 1411-1427. doi: 10.1007/s10439-012-0695-0
![]() |
[22] |
V. N. Surya, E. Michalaki, G. G. Fuller, A. R. Dunn, Lymphatic endothelial cell calcium pulses are sensitive to spatial gradients in wall shear stress, Mol. Biol. Cell, 30 (2019), 923-931. doi: 10.1091/mbc.E18-10-0618
![]() |
[23] |
E. Michalaki, V. N. Surya, G. G. Fuller, A. R. Dunn, Perpendicular alignment of lymphatic endothelial cells in response to spatial gradients in wall shear stress, Commun. Biol., 3 (2020), 57-1-57-9. doi: 10.1038/s42003-019-0732-8
![]() |
[24] | A. Sabine, M. J. Davis, E. Bovay, T. V. Petrova, Characterization of mouse mesenteric lymphatic valve structure and function. In: Lymphangiogenesis (eds. G Oliver, M Kahn). Methods in Molecular Biology, vol 1846. Humana Press, New York, (2018), pp 97-129.doi:10.1007/978-1-4939-8712-2_7 |
[25] |
M. R. Kelly-Goss, E. R. Winterer, P. C. Stapor, M. Yang, R. S. Sweat, W. B. Stallcup, et al., Cell proliferation along vascular islands during microvascular network growth, BMC Physiol., 12 (2012), 7-1-7-9. doi: 10.1186/1472-6793-12-7
![]() |
[26] |
J. Chiu, S. Chien, Effects of disturbed flow on vascular endothelium: pathophysiological basis and clinical perspectives, Physiol. Rev., 91 (2011), 327-387. doi: 10.1152/physrev.00047.2009
![]() |
1. | Francine Blei, Update April 2021, 2021, 19, 1539-6851, 189, 10.1089/lrb.2021.29102.fb | |
2. | Christopher D. Bertram, Charlie Macaskill, Fluid‐Dynamic Modeling of Flow in Embryonic Tissue Indicates That Lymphatic Valve Location Is Not Consistently Determined by the Local Fluid Shear or Its Gradient, 2024, 31, 1073-9688, 10.1111/micc.12873 |
(A) shear stress | |||||
image | segm. | p/g dir. | n1 | r2 | p |
1 | cul-de-sac | LR | 4 | 0.2195 | ** |
in/outlets | UD | 10 | 0.5354 | *** | |
2 | cul-de-sac | LR | 4 | 0.1823 | ** |
in/outlets | LR | 8 | 0.0693 | - | |
3 | cul-de-sac | LR | 6 | 0.0505 | - |
3m | in/outlets | UD | 4 | 0.0830 | * |
3x | in/outlets | LR | 6 | 0.0178 | - |
(B) shear stress gradient | |||||
image | segm. | p/g dir. | n1 | r2 | p |
1 | cul-de-sac | LR | 0 | 0.0099 | - |
in/outlets | UD | 2 | 0.0128 | - | |
2 | cul-de-sac | LR | 0 | 0.0102 | - |
in/outlets | LR | 0 | 0.0244 | - | |
3 | cul-de-sac | LR | 4 | 0.3334 | *** |
3m | in/outlets | UD | 2 | 0.0994 | * |
3x | in/outlets | UD | 4 | 0.1684 | ** |
(A) shear stress | |||||
image | segm. | p/g dir. | n1 | r2 | p |
1 | cul-de-sac | LR | 4 | 0.2195 | ** |
in/outlets | UD | 10 | 0.5354 | *** | |
2 | cul-de-sac | LR | 4 | 0.1823 | ** |
in/outlets | LR | 8 | 0.0693 | - | |
3 | cul-de-sac | LR | 6 | 0.0505 | - |
3m | in/outlets | UD | 4 | 0.0830 | * |
3x | in/outlets | LR | 6 | 0.0178 | - |
(B) shear stress gradient | |||||
image | segm. | p/g dir. | n1 | r2 | p |
1 | cul-de-sac | LR | 0 | 0.0099 | - |
in/outlets | UD | 2 | 0.0128 | - | |
2 | cul-de-sac | LR | 0 | 0.0102 | - |
in/outlets | LR | 0 | 0.0244 | - | |
3 | cul-de-sac | LR | 4 | 0.3334 | *** |
3m | in/outlets | UD | 2 | 0.0994 | * |
3x | in/outlets | UD | 4 | 0.1684 | ** |