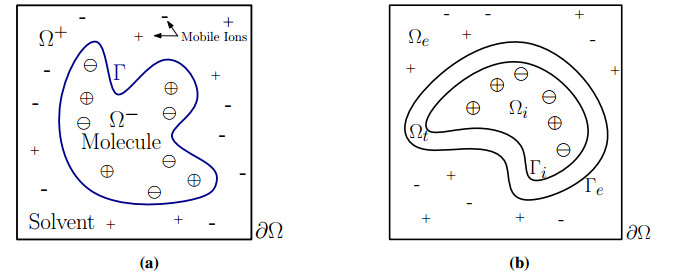
Numerical treatment of singular charges is a grand challenge in solving the Poisson-Boltzmann (PB) equation for analyzing electrostatic interactions between the solute biomolecules and the surrounding solvent with ions. For diffuse interface PB models in which solute and solvent are separated by a smooth boundary, no effective algorithm for singular charges has been developed, because the fundamental solution with a space dependent dielectric function is intractable. In this work, a novel regularization formulation is proposed to capture the singularity analytically, which is the first of its kind for diffuse interface PB models. The success lies in a dual decomposition – besides decomposing the potential into Coulomb and reaction field components, the dielectric function is also split into a constant base plus space changing part. Using the constant dielectric base, the Coulomb potential is represented analytically via Green's functions. After removing the singularity, the reaction field potential satisfies a regularized PB equation with a smooth source. To validate the proposed regularization, a Gaussian convolution surface (GCS) is also introduced, which efficiently generates a diffuse interface for three-dimensional realistic biomolecules. The performance of the proposed regularization is examined by considering both analytical and GCS diffuse interfaces, and compared with the trilinear method. Moreover, the proposed GCS-regularization algorithm is validated by calculating electrostatic free energies for a set of proteins and by estimating salt affinities for seven protein complexes. The results are consistent with experimental data and estimates of sharp interface PB models.
Citation: Siwen Wang, Emil Alexov, Shan Zhao. On regularization of charge singularities in solving the Poisson-Boltzmann equation with a smooth solute-solvent boundary[J]. Mathematical Biosciences and Engineering, 2021, 18(2): 1370-1405. doi: 10.3934/mbe.2021072
[1] | Reymundo Itzá Balam, Francisco Hernandez-Lopez, Joel Trejo-Sánchez, Miguel Uh Zapata . An immersed boundary neural network for solving elliptic equations with singular forces on arbitrary domains. Mathematical Biosciences and Engineering, 2021, 18(1): 22-56. doi: 10.3934/mbe.2021002 |
[2] | Hamid Mofidi . New insights into the effects of small permanent charge on ionic flows: A higher order analysis. Mathematical Biosciences and Engineering, 2024, 21(5): 6042-6076. doi: 10.3934/mbe.2024266 |
[3] | Peter W. Bates, Jianing Chen, Mingji Zhang . Dynamics of ionic flows via Poisson-Nernst-Planck systems with local hard-sphere potentials: Competition between cations. Mathematical Biosciences and Engineering, 2020, 17(4): 3736-3766. doi: 10.3934/mbe.2020210 |
[4] | Chuan Li, Mark McGowan, Emil Alexov, Shan Zhao . A Newton-like iterative method implemented in the DelPhi for solving the nonlinear Poisson-Boltzmann equation. Mathematical Biosciences and Engineering, 2020, 17(6): 6259-6277. doi: 10.3934/mbe.2020331 |
[5] | Jue Wu, Lei Yang, Fujun Yang, Peihong Zhang, Keqiang Bai . Hybrid recommendation algorithm based on real-valued RBM and CNN. Mathematical Biosciences and Engineering, 2022, 19(10): 10673-10686. doi: 10.3934/mbe.2022499 |
[6] | Haohao Xu, Yuchen Gong, Xinyi Xia, Dong Li, Zhuangzhi Yan, Jun Shi, Qi Zhang . Gabor-based anisotropic diffusion with lattice Boltzmann method for medical ultrasound despeckling. Mathematical Biosciences and Engineering, 2019, 16(6): 7546-7561. doi: 10.3934/mbe.2019379 |
[7] | Wenrui Li, Qimin Zhang, Meyer-Baese Anke, Ming Ye, Yan Li . Taylor approximation of the solution of age-dependent stochastic delay population equations with Ornstein-Uhlenbeck process and Poisson jumps. Mathematical Biosciences and Engineering, 2020, 17(3): 2650-2675. doi: 10.3934/mbe.2020145 |
[8] | Jin Li, Yongling Cheng, Zongcheng Li, Zhikang Tian . Linear barycentric rational collocation method for solving generalized Poisson equations. Mathematical Biosciences and Engineering, 2023, 20(3): 4782-4797. doi: 10.3934/mbe.2023221 |
[9] | Zijuan Wen, Meng Fan, Asim M. Asiri, Ebraheem O. Alzahrani, Mohamed M. El-Dessoky, Yang Kuang . Global existence and uniqueness of classical solutions for a generalized quasilinear parabolic equation with application to a glioblastoma growth model. Mathematical Biosciences and Engineering, 2017, 14(2): 407-420. doi: 10.3934/mbe.2017025 |
[10] | M. Botros, E. A. A. Ziada, I. L. EL-Kalla . Semi-analytic solutions of nonlinear multidimensional fractional differential equations. Mathematical Biosciences and Engineering, 2022, 19(12): 13306-13320. doi: 10.3934/mbe.2022623 |
Numerical treatment of singular charges is a grand challenge in solving the Poisson-Boltzmann (PB) equation for analyzing electrostatic interactions between the solute biomolecules and the surrounding solvent with ions. For diffuse interface PB models in which solute and solvent are separated by a smooth boundary, no effective algorithm for singular charges has been developed, because the fundamental solution with a space dependent dielectric function is intractable. In this work, a novel regularization formulation is proposed to capture the singularity analytically, which is the first of its kind for diffuse interface PB models. The success lies in a dual decomposition – besides decomposing the potential into Coulomb and reaction field components, the dielectric function is also split into a constant base plus space changing part. Using the constant dielectric base, the Coulomb potential is represented analytically via Green's functions. After removing the singularity, the reaction field potential satisfies a regularized PB equation with a smooth source. To validate the proposed regularization, a Gaussian convolution surface (GCS) is also introduced, which efficiently generates a diffuse interface for three-dimensional realistic biomolecules. The performance of the proposed regularization is examined by considering both analytical and GCS diffuse interfaces, and compared with the trilinear method. Moreover, the proposed GCS-regularization algorithm is validated by calculating electrostatic free energies for a set of proteins and by estimating salt affinities for seven protein complexes. The results are consistent with experimental data and estimates of sharp interface PB models.
At atomistic level of detail, biological processes involve charged objects such as proteins, DNAs and RNAs, immersed in an aquatic environment with mobile ions. To understand such processes, an electrostatic analysis is indispensable, which concerns interactions between solute macromolecules, the surrounding solvent molecules, and ions. Explicit solvent calculations are very expensive since they involve millions of water molecules, while implicit solvent models, such as the Poisson-Boltzmann (PB) equation [1,2,3], allow for fast analysis, by computing interactions via a mean force approach. Physically, by treating the macromolecule and water as dielectric continuum, the PB model combines the Gauss's law in electrodynamics with Boltzmann distribution in statistical thermodynamics. Mathematically, the PB equation is an elliptic partial differential equation (PDE) with singular source terms to account for partial charges contained in the macromolecule, and is defined on a domain consisting of solute and solvent regions.
In the classical PB model [1,2,3], a sharp interface is assumed as the solute-solvent boundary, and a low dielectric constant is assigned to the biomolecule, while the water phase is considered as a high dielectric constant medium. The shape of the interface is known as a molecular surface, and is usually modeled according to the protein structure, as well as effect of water penetration. The most commonly used models include Van der Waals (VdW) surface, solvent accessible surface (SAS) [4], solvent excluded surface (SES) [5], and Gaussian surface [6]. Nevertheless, a sharp interface introduces a discontinuity in the dielectric coefficient across the solute-solvent boundary, so that the PB potential solution loses its regularity too. This demands special handling in numerical solution of the PB equation [8,7]. Moreover, geometrical singularities could occur at sharp molecular surfaces [9,10].
It is known physically that the dielectric coefficient of a medium is determined by the polarizability of the medium in responding to local electrostatic field. Thus, for biological and chemical systems in molecular scales, the assumption of a sharp interface in the PB model as the solute-solvent boundary seems to be unphysical [11,12,13]. In particular, beginning with macromolecule interior and moving toward the molecular surface and further into the water phase, the polarizability constantly increases, but should not undergo a sharp jump [14]. This motivates the development of many PB models by treating the solute-solvent boundary as a smooth diffuse interface [9,10,15,16,17,18]. For example, in studying charged objects immersed in liquids, a smooth implicit solvent model has been developed by incorporating the structures of water dipoles and ions into mean field modeling, so that the effective dielectric coefficient becomes a smoothly variant function [15]. A popular mathematical formulation [9,10,16,17,18] for studying polar and non-polar interactions between the macromolecule and solvent is to construct a free energy functional by incorporating various contributions, such as electrostatic effects, protein dimensions, surface curvatures, etc. Then, the Euler-Lagrange variation of the free energy minimization will lead to a coupled PDE system, and the resulted PB equation naturally involves a diffuse interface type dielectric boundary.
This work focuses on numerical treatments of the singular sources in the PB equation, which take the form of a sum of the Dirac delta distributions in modeling of the partial charges located at the atomic centers of the biomolecules [1,2,3]. Mathematically, the electrostatic potential blows up at the atom centers too, which introduces a great difficulty to the theoretical analysis [19]. Numerically, the accurate treatment of singular charge sources in grid based computations is a significant issue for the PB equation with either sharp or diffuse interfaces.
The existing algorithms for the PB charge singularities in the classical sharp interface setting can be classified into direct and indirect approaches. The most commonly used direct approach is to distribute the point charges to their neighboring vertexes of the cube or element by means of a trilinear approximation [3]. Alternatively, in a finite element variational form, one can apply the definition of the delta function so that a point charge can be evaluated through the trial functions [20]. Being computationally efficient, these direct approaches usually involve a large approximation error. To improve the accuracy, the approximation of partial charges by Gaussian function or high order polynomials has also been considered in the PB literature [21,22]. Nevertheless, these methods involve a wide stencil of grid nodes for approximating a point charge. Numerically, when the point charge is near the molecular surface, it could happen that one will use nodes outside the molecular surface to approximate partial charges inside the protein. Recently, a second order accurate geometric discretization of the multidimensional Dirac delta distribution has been introduced in [23] for solving Poisson's equation, in which the approximation is confined within a simplex. Special treatments have been designed so that the second order accuracy is maintained even when the simplex is intersecting with the dielectric interface.
In an indirect approach for solving the PB equation with a sharp interface, the inaccurate discretization of the unbounded delta function by finite grid values is avoided. In the biophysics community, an induced charge method has been developed [24,25], in which the free energy is split into Coulomb, reaction field, and ionic solvent components. The reaction field effects due to a dielectric boundary can be physically reproduced by an appropriate mapping of induced polarization charge placed on the molecular surface, so that one can use real charges rather than their grid representation in calculating the free energy. In the mathematical literature, a series regularization methods [8,19,26,27,28,29,30,31,32,33] have been introduced for handling singular charges by directly manipulating the PDE. Typically, these methods involve a decomposition of the potential solution into two or three components with a singular one to capture the singularity. In most methods, this singular component is defined as the Coulomb potential and satisfies a Poisson's equation with the same singular sources, so that it can be analytically solved as Green's functions. In the range separated regularization method [26,31], the delta functions are further decomposed into local and global components. The singular component corresponding to the local singular sources can be accurately approximated by tensor discretizations. After removing the calculated singular component, other potential components in all regularizations become bounded so that their numerical computation becomes more accurate. Recently, a comparison of four popular regularizations was conducted in [34] to investigate an accuracy reduction issue. An accuracy recovery technique has been correspondingly designed so that all four methods yield the same high precision.
To the best of the authors' knowledge, only direct approaches have been implemented in the existing diffuse interface PB models, and indirect approaches have never been developed for handling charge sources, due to various challenges. In the induced charge method [24,25], the surface induced charges are defined on the sharp molecular surface. It is uncertain if this method can be generalized for a diffuse interface. For regularization methods, the difficulty is due to the space dependent dielectric function in the Laplacian operator of the PB equation. Mathematically, the fundamental solution that satisfies the Poisson's equation with such a Laplacian and singular sources is not analytically available, even though a semi-analytical method has been proposed to construct it for separable geometries via quasi-harmonic approximations [35]. Without an analytical representation of the singular potential, it is unclear on how to formulate a regularization for the PB equation with diffuse interfaces.
The main goal of this paper to introduce the first regularization method to treat singular charges for diffuse interface PB models. Following our recent study of Poisson's equation with a diffuse interface [36], the main idea of our new approach is to decompose the inhomogeneous dielectric function into a constant base plus a space variant part. This allows us to capture the singular potential by considering a Poisson's equation with the constant base dielectric value, and analytically solve the fundamental solution as Green's functions. Through a simple PDE analysis, the other component, i.e., the reaction field potential, can be shown to be bounded. With a smooth dielectric setting and being free of singular sources, the reaction field potential can be accurately approximated by any numerical discretization.
The second goal of this work is to introduce a simple algorithm for generating a diffuse interface in dealing with complex protein systems. In principle, the proposed regularization method can be applied to all existing diffuse interface PB models [9,10,15,16,18,17]. Nevertheless, in order to validate our regularization in an independent setting, a Gaussian convolution surface (GCS) will be introduced together with a fast Fourier transform (FFT) implementation. The new algorithm can robustly handle various protein geometries, and is very efficient for large complex systems. The GCS algorithm will provide a smooth surface function to characterize the space, which takes a constant value of one and zero, respectively, in the solute and solvent domains. In a narrow transition layer at the solute-solvent boundary, the surface function decays smoothly from one to zero.
The rest of this paper is organized as follows. The proposed regularization method will be introduced in Section 2. Its numerical implementation will be discussed too. In Section 3, the details of the proposed GCS diffuse interface will be offered. Numerical tests will be carried out to examine the GCS model parameters. The validation of the proposed regularization based on analytical surface and GCS will be considered in Section 4 for several simple atomic systems. The biological applications to small macromolecules, large proteins, and binding protein complexes will be investigated in Section 5. Finally, this paper ends with a conclusion.
In this section, we will develop a regularization approach for treating singular charge sources of the Poisson-Boltzmann (PB) equation, where a smooth solute-solvent boundary is assumed to be given by a known diffuse interface. Numerical discretization of the PB equation and energy calculation will be discussed too.
In implicit solvent models, a macromolecule such as a protein is regarded as a solute, being immersed into an aqueous solvent. Consider a large enough cubic domain Ω⊂R3 that contains this three dimensional (3D) solute-solvent system. In a traditional two-dielectric PB model [1,2,3], the domain Ω is divided by a molecule surface Γ into two parts, namely the molecule domain Ω− and solvent domain Ω+, and the dielectric function ϵ(→r) is assumed to be a piecewise constant with ϵ=ϵ− in Ω− and ϵ=ϵ+ in Ω+. See Figure 1a for an illustration.
In this paper, we will focus on a smooth dielectric PB model with a diffuse interface, see Figure 1b. The domain Ω is separated into three regions: an interior domain Ωi, an exterior domain Ωe and a transition layer Ωt in between Ωi and Ωe. The dielectric function ϵ(→r) will take constant values in solute and solvent with ϵ(→r)=ϵi in Ωi and ϵ(→r)=ϵe in Ωe, with 0<ϵi<ϵe. In the smooth solute-solvent boundary Ωt, ϵ(→r) varies smoothly from ϵi to ϵe. Mathematically, the domain partition can be characterized by a level set or surface function S(→r), which equals to one and zero, respectively, in Ωi and Ωe. In Ωt, S(→r) monotonically decays from one to zero, so that S(→r) is at least a C2 continuous function over the entire domain Ω. See Figure 2a for an illustration. The dielectric function is then defined as [9,12]
ϵ(→r)=S(→r)ϵi+(1−S(→r))ϵe,for →r∈Ω, | (2.1) |
which is also at least C2 continuous, see Figure 2b. In the present study, we will take ϵi=1 for the protein and ϵe=80 for the water. The construction of the smeared surface function S(→r) for real proteins will be discussed in the next section. We assume S(→r) being given in the following discussion on regularization.
For the present setting, the nonlinear Poisson-Boltzmann (PB) equation for the electrostatic potential u(→r) can be expressed as [12]
−∇⋅(ϵ(→r)∇u(→r))+(1−S(→r))κ2sinh(u(→r))=ρ(→r),in Ω, | (2.2) |
in the dimensionless form. Here the source term is due to singular charges contained in the protein
ρ(→r)=4πec2kBTNm∑j=1qjδ(→r−→rj),in Ω, | (2.3) |
where δ(→r−→rj) is the Dirac delta distribution. Consequently, the solution u to Eq (2.2) shall be defined in a weak form. We refer to the references [19,32,37] for more details about the weak solution and well-posedness of the PB equation.
In this work, Nm is the total number of atoms in the solute molecule, kB is the Boltzmann constant, and T is the temperature. For each atom, a partial charge qj in terms of the fundamental charge ec is located at the atom center →rj. Since →rj∈Ωi for all j, ρ(→r) is only defined within in Ωi and S(→r) has actually been dropped in the source term, i.e., S(→r)ρ(→r)=ρ(→r) in Eq (2.2). With S(→r), the coefficient of the PB nonlinear term (1−S)κ2 is at least C2 continuous, where the modified Debye-Hückel parameter κ takes a constant value κ2=(2NAe2c100kBT)I=8.486902807Å−2I. Here NA is the Avogadro's Number and I is the molar ionic strength. On the outer boundary ∂Ω, a Dirichlet boundary condition can be assumed
u(→r)=ub(→r):=ec2kBTNm∑j=1qjϵe|→r−→rj|e−|→r−→rj|√κ2ϵe,on ∂Ω. | (2.4) |
We note that the potential u and its gradient ∇u are continuous everywhere in Ω, except at charge centers. We also note that the dimensionless potential u=ecϕkBT, where the original electrostatic potential ϕ has the unit kcal/mol/ec.
Following our recent study [36] on the regularization of the Poisson's equation with a diffuse interface, we propose to regularize the PB equation (2.2) through a dual decomposition strategy. The potential u is split into a Coulomb component uC and a reaction field component uRF with u(→r)=uC(→r)+uRF(→r) in Ω. The dielectric function is decomposed to be a constant base value plus space variant part ϵ(→r)=ϵi+ˆϵ(→r), with ˆϵ(→r)≥0 for →r∈Ω. Note that ˆϵ=0 in Ωi and ˆϵ=ϵe−ϵi in Ωe.
The key of regularization is to capture the solution singularity analytically by the Green's function. Similar to [36], we assume that the Coulomb potential uC satisfies a homogeneous Poisson's equation with the singular source ρ given by Eq (2.3),
{−ϵiΔuC(→r)=ρ(→r)in R3,uC(→r)=0as |→r|→∞. | (2.5) |
Then, the singular component uC is analytically given as the Green's function G(→r)
uC(→r)=G(→r):=e2ckBTNm∑j=1qjϵi|→r−→rj|. | (2.6) |
After removing uC, the reaction field potential uRF(→r) will be bounded everywhere inside Ω.
With the dual decomposition, the PB equation (2.2) can be expanded as
−∇⋅(ˆϵ∇uC))−∇⋅(ˆϵ∇uRF)−ϵiΔuC−ϵiΔuRF+(1−S)κ2sinh(uC+uRF)=ρ,in Ω. | (2.7) |
By subtracting Eq (2.5) from Eq (2.7), the PB equation is free of singular charges
−∇⋅(ˆϵ∇uC)−∇⋅(ˆϵ∇uRF)−ϵiΔuRF+(1−S)κ2sinh(uC+uRF)=0,in Ω. | (2.8) |
Since uC=G(→r) is known, we can move the first term to the right-hand side
−∇⋅(ˆϵ∇uRF)−ϵiΔuRF+(1−S)κ2sinh(uRF+G)=∇⋅(ˆϵ∇G),in Ω. | (2.9) |
This actually gives rise to a regularized PB equation for the reaction field component
−∇⋅(ϵ∇uRF)+(1−S)κ2sinh(uRF+G)=∇⋅(ˆϵ∇G),in Ω, | (2.10) |
where the gradient of Green's function is analytically given as
∇G(→r)=−e2ckBTNm∑j=1qj(→r−→rj)ϵi|→r−→rj|3. | (2.11) |
The regularized source ∇⋅(ˆϵ∇G) is smooth and non-vanishing only in Ωt. To see this, recall that ˆϵ=0 inside Ωi. Even though ∇G is singular at atom centers →rj in Ωi, the limit of ˆϵ∇G as →r goes to →rj exists and actually equals to zero. Thus, ˆϵ∇G is a smooth function in the entire domain Ω, so that the new source term can be rewritten as
∇⋅(ˆϵ∇G)=∇ˆϵ⋅∇G+ˆϵΔG,in Ω. | (2.12) |
Since ΔG=0 everywhere except at charge centers, whereas ˆϵ=0 inside Ωi including at charge centers, we have ˆϵΔG=0 for all →r∈Ω. Thus, this term can be dropped from the new source
∇⋅(ˆϵ∇G)=∇ˆϵ⋅∇G=∇ϵ⋅∇G,in Ω, | (2.13) |
where we have applied ∇ϵ=∇ˆϵ, because ϵ and ˆϵ differ by a constant ϵi. According to the definition of ϵ by Eq (2.1), ∇ϵ=0 in both molecule domain Ωi and solvent domain Ωe. So does ∇ϵ⋅∇G. In the transition layer Ωt, ∇G can be analytically calculated according to Eq (2.11), while ∇ϵ shall be smooth. Thus, the regularized source ∇ϵ⋅∇G is bounded and smooth in entire Ω, while being non-vanishing only in Ωt.
In summary, we propose a new regularized PB equation for the reaction field potential
{−∇⋅(ϵ∇uRF)+(1−S)κ2sinh(uRF+G)=∇ϵ⋅∇Gin Ω,uRF=ub(→r)−G(→r)on ∂Ω. | (2.14) |
We note that the decomposition of the dielectric function ϵ=ϵi+ˆϵ is used only in the derivation, and all computations can be carried out based on ϵ(→r) only. Since (1−S)=0 inside Ωi, the nonlinear term in Eq (2.14) is also vanishing in Ωi. This guarantees that the reaction field potential uRF will be bounded and smooth in Ω, and can be easily solved by any numerical method. Once uRF is computed from Eq (2.14), the potential solution of the PB equation (2.2) is simply given by u=uRF+G. Throughout this paper, the dielectric coefficients will be chosen as ϵi=1 and ϵe=80. The ionic strength will be taken as I=0.15 M unless specified otherwise.
When the electrostatic potential is weak, sinh(u) can be approximated by u, which yields a linearized PB (LPB) model. For simplicity, we will numerically validate the proposed regularization method by considering the following LPB equation with a diffuse interface
−∇⋅(ϵ(→r)∇u(→r))+(1−S(→r))κ2u(→r)=ρ(→r),in Ω, | (2.15) |
subject to the same source term (2.3) and boundary condition (2.4). It can be similarly derived that now the reaction field component satisfies the following regularized LPB equation
{−∇⋅(ϵ∇uRF)+(1−S)κ2uRF=∇ϵ⋅∇G−(1−S)κ2Gin Ω,uRF=ub(→r)−G(→r)on ∂Ω. | (2.16) |
Note that the second source term is vanishing inside Ωi due to (1−S) factor. In Ωt and Ωe, the Green's function G(→r) is bounded and can be directly computed by Eq (2.6).
Consider a uniform mesh partition of the computational domain Ω, with Nx, Ny, Nz being the number of the grid points in x, y, and z directions, respectively. Without the loss of generality, we assume the grid spacing h in all x, y, and z directions to be the same, i.e., h=Δx=Δy=Δz, with the unit Å. For a function f defined at a node (xi,yj,zk), we denote fi,j,k=f(xi,yj,zk). For real proteins, the surface function S(→r) has to be generated numerically. Thus, in the present numerical discretization, we will assume Si,j,k being known for all i, j, and k. Similarly, by using Eq (2.1), we have all nodal values ϵi,j,k. But we may not know S and ϵ values off-grid. In the source term, Gi,j,k and ∇Gi,j,k can be calculated analytically.
The central finite difference method is employed to solve the regularized LPB equation (2.16). For this purpose, we rewrite Eq (2.16) into its Cartesian component form
−ϵ(∂2uRF∂x2+∂2uRF∂x2+∂2uRF∂z2)−∂ϵ∂x∂uRF∂x−∂ϵ∂y∂uRF∂y−∂ϵ∂z∂uRF∂z+(1−S)κ2uRF=∂ϵ∂x∂G∂x+∂ϵ∂y∂G∂y+∂ϵ∂z∂G∂z−(1−S)κ2G. | (2.17) |
Since both surface function S and dielectric function ϵ are available only on grid nodes, derivatives of uRF and ϵ in Eq (2.17) will be discretized by using central differences involving nodal values. For example, we have
∂2uRF∂x2|i,j,k=(uRF)i−1,j,k−2(uRF)i,j,k+(uRF)i+1,j,kh2+O(h2), | (2.18) |
∂ϵ∂x|i,j,k=ϵi+1,j,k−ϵi−1,j,k2h+O(h2). | (2.19) |
In the present study, the band-width of Ωt will be taken as 3Å in protein studies, which guarantees a high accuracy in approximating ∇ϵ by Eq (2.19). After discretization, Eq (2.17) becomes a sparse linear system with dimension N3-by-N3, where N3=Nx×Ny×Nz. A biconjugate gradient iterative solver [38] is employed to solve this linear system for uRF(xi,yj,zk). The order of accuracy of the entire central difference discretization is two.
For a comparison, the traditional trilinear method [3] will also be employed to treat singular charges. In the trilinear method, for each charge qj in the source ρ located at →rj, one will find a cubic cell containing this charge. One will then distribute the charge qj into eight corner nodes of the cell. Denote Qi,j,k as the resulting fractional charge at grid point (xi,yj,zk). One can then directly discretize the LPB equation (2.15) by evaluating qj as eight Qi,j,k values in ρ, while the left hand side terms can be approximated by the same second order central differences. We denote the resulting potential of the trilinear method as uTL(xi,yj,zk). The trilinear distribution usually involves a huge error——much larger than the discretization error of the central difference.
The energy released when the solute molecule is dissolved in solvent is known as the free energy of solvation. The polar component of solvation free energy can be calculated in the PB model by computing the difference between total electrostatic free energy of the macromolecule in the solvent and in the vacuum [1,2,3]. For the linearized PB equation with a diffuse interface, i.e., Eq (2.15), this involves two numerical solutions in traditional methods, such as the trilinear method. One first solves Eq (2.15) for the water state to obtain u(→r). For the vacuum state, one solves a Poisson equation
−ϵiΔu0(→r)=ρ(→r), | (2.20) |
which is obtained by taking κ=0 in the PB equation (2.15) and boundary condition (2.4), and ϵi=ϵe=1 for defining ϵ in Eq (2.1). Here we denote the vacuum state solution as u0(→r). The polar solvation free energy or electrostatic free energy is then calculated as
E=12kBT∫ΩNm∑j=1qjδ(→r−→rj)(u(→r)−u0(→r))d→r=12kBTNm∑j=1qj(u(→rj)−u0(→rj)). | (2.21) |
We note that the solution of Eq (2.20) is actually the coulomb component uC, which is known analytically as the Green's function G. Nevertheless, in the trilinear method, one still numerically solvers Eq (2.20) for u0. Because the same source approximation is utilized in the water and vacuum states on the same mesh, the discretization error of trilinear distribution can be offset when evaluating uTL−u0 in Eq (2.21) for free energy calculation [3].
For the regularization approach, one does not need to solve Eq (2.20), and just solves the PB equation once in the water state for uRF. Then, the electrostatic free energy is computed as
E=12kBT∫ΩNm∑j=1qjδ(→r−→rj)(u(→r)−uC(→r))d→r=12kBTNm∑j=1qjuRF(→rj). | (2.22) |
Since the calculated potentials u, u0, and uRF are available only at grid nodes (xi,yj,zk), a linear interpolation is conducted to evaluate these potentials at charge centers →rj in Eqs (2.21) and (2.22). In the present study, the electrostatic free energy will be reported in the unit of kcal/mol.
In order to validate our regularization in an independent setting, we will introduce a simple algorithm in this section to compute the surface function S(→r). For any protein, we will determine Γi and Γe properly so that S(→r)=1 in Ωi and S(→r)=0 in Ωe, while S(→r) changes smoothly in Ωt. Our intention is not to introduce another molecular surface definition. Instead, our focus is on computational efficiency in treating large proteins, as well as numerical robustness in handling complex shapes in a discrete setting.
We first briefly review several commonly used molecular surfaces, see Figure 3a. For a protein with a total Nm atoms, we know the center →rl and radius rl of each atom. By representing each atom as a hard sphere, the Van der Waals (VdW) surface is simply defined as the smallest envelop enclosing the union of all spheres. The solute-accessible surface (SAS) and solute-excluded surface (SES) [4,5] are defined by rolling a probe sphere around the VdW surface, to mimic the solvent penetration by the water molecule. The probe radius rp will be fixed as 1.5Å in this study. The SAS is traced by the center of the probe, while the SES is traced by the inward-facing surface of the probe, see Figure 3a. However, these sharp interface molecular surface definitions are known to admit geometric singularities [9,10].
We propose a new algorithm to generate a diffuse interface type smooth molecular surface based on the SAS. We start by defining a Heaviside function H(→r) such that H=1 when →r is inside the SAS or on the SAS, and H=0 when →r is outside. A smeared surface function S(→r) can be obtained by convoluting H(→r) with a one-dimensional (1D) Gaussian kernel function K(x) defined as
K(x)=1σ√2πexp(−x22σ2). | (3.1) |
This normalized Gaussian function represents the probability density function of a normal distribution with a variance σ and the expected value being zero. The Gaussian convolution or filtering will be carried out in x, y, and z directions in a tensor product style. Thus, the convolution of H and K along a x grid line can be expressed as
(H∗K)(x)=∫∞−∞H(x−t)K(t)dt, | (3.2) |
where we have abused the notation by denoting H as a 1D function function H(x). In Figure 3b, such a 1D Heaviside function H(x) is shown in the top portion. The convoluted function shown in the bottom portion clearly involves a smooth transition from 1 to 0, corresponding to a smooth solute-solvent boundary.
Mathematically, the convolution equation (3.2) can be simply realized via the Fourier transform F, which transfers a physical space in x to a frequency space in ω,
F{H}(ω)=F{H(x)}=12π∫∞−∞H(x)eiωxdx. | (3.3) |
By the convolution theorem, we have
F{H(x)∗K(x)}=F{H(x)}×F{K(x)}. | (3.4) |
Thus, the convolution equation (3.2) can be carried out in three steps. First, both H(x) and K(x) are transformed into frequency space to obtain F{H} and F{K}. Second, a scalar product of two Fourier components produces the Fourier component F{H∗K}. Finally, an inverse Fourier transform yields the convoluted function (H∗K)(x). The resulting function after convoluting H(→r) with K(x) in x, y, and z directions is the desired smeared surface function S(→r), and the underlying diffuse interface will be called as the Gaussian convolution surface (GCS).
The initial setup of the GCS computation is illustrated in Figure 4a. Centered around the SAS, we aim to create a transition layer with the width being 3Å as the smooth solute-solvent boundary. This guarantees that the solute domain ˜Ωi inside the VdW surface ˜Γi has a fixed dielectric value ϵ=ϵi. Mathematically, we define an enlarged SAS by augmenting the atomic radii by 2rp=3Å, and denote it as ˜Γe. Outside ˜Γe, the dielectric value is fixed as ϵ=ϵe in the solvent domain ˜Ωe. Note that we have added a tilde in all notations because they are physically defined before the Gaussian filtering. After convolution, the actual domains and boundaries will be slightly different. We will discuss them in the next subsection.
In practice, the GCS is generated discretely based on a given uniform mesh with a spacing h. The fast Fourier transform (FFT) will be adopted to accelerate the computational speed. In such computation, one does not need to specify the exact locations of ˜Γi and ˜Γe. Instead, it is sufficient to generate nodal values of S(xi,yj,zk) for all grid nodes, which then can be utilized in the regularization discretization.
We first numerically determine the Heaviside function. Consider a protein with Nm atoms. For l=1,2,…Nm, the atom center (xl,yl,zl) and radius rl of the protein structure can be obtained from the Protein Data Bank (PDB) by using the PDB ID. Note that the SAS is actually a VdW surface by augmenting each atomic radius rl by the probe radius rp=1.5Å. We first initiate H=0 for all nodes (xi,yj,zk) in Ω. Then for each atom, we will search for nearby nodes. If the distance between the atom center and one node is close enough, i.e., √(xl−xi)2+(yl−yj)2+(zl−zk)2≤rl+rp, we set H(xi,yj,zk)=1.
A proper discrete sampling of Gaussian kernel K(x) is crucial for convoluting with the Heaviside function H(xi,yj,zk). In order to make sure that the width of the transition layer is around 3Å, we may control the window size of K(x) by adjusting σ. Another important factor is the width of the sampled Gaussian kernel, see Figure 4b. In the present study, we set the half-width to be
W=σ√−ln(2πτ2σ2), | (3.5) |
which is obtained by solving K(W)=τ, where τ is a tolerance measuring the height of the truncated Gaussian kernel above the x axis, see Figure 4b. The tolerance is fixed to be τ=0.0025 in this work. Then, the discrete kernel will be sampled uniformly in the interval [−W,W]. The total number of sampling is related to the probe radius rp and the mesh spacing h,
M=2⌊rph⌋+1, | (3.6) |
where ⌊⋅⌋ is the floor function. For example, for rp=1.5 and h=0.5, we have M=7. We then sample K(x) defined by Eq (3.1) by a uniform grid with M nodes over the interval [−W,W], see Figure 4b. The resulting vector is denoted as →K with →K∈RM.
The Gaussian convolution equation (3.2) will be carried out dimension by dimension. Without the loss of generality, let us discuss the filtering in the x direction with Nx grid nodes. The discrete Heaviside function is a vector of dimension Nx, and is denoted as →H. An illustration of →H and →K is shown in Figure 5, along with the convoluted vector. Note that by the definition of both Heaviside function H(→r) and surface function S(→r), a periodic boundary condition could be assumed for both functions over the domain Ω in any Cartesian direction. Thus, their discretized versions also satisfy the periodic boundary condition. Therefore, the convolution of two vectors →H and →K could be represented either as linear convolution or circular convolution. For instance, in case of linear convolution, let us formulate the convoluted vector (→H⋆→K) with its i-th element being
(→H⋆→K)[i]=M∑j=1→H[i+j−1]→K[j]. | (3.7) |
In order to make sure that the convoluted vector has dimension Nx, the discrete convolution equation (3.7) shall be defined for i=1,2,…Nx. This means that the dimension →H has to be extended to Nx+M−1. This is achieved by zero padding, i.e., one simply adds enough number of zeros at the end of vector →H. Moreover, in order to apply the FFT algorithm, the dimension of →H should be an integer power of 2. Thus, more zeros will be added into →H such that the dimension Nf is the least integer satisfying Nf>Nx+M−1 and Nf=2m for some integer m. A vector →H after zero padding is shown in the top portion of Figure 5. The vector →K is also padded with zeros such that its dimension is Nf as well, see the middle portion. By maintaining the same length for →H and →K, the discrete Fourier components of both vectors, which are obtained by the FFT, can be multiplied term-wisely. An inverse FFT will produce a vector of dimension Nf, as shown in the bottom part of Figure 5. Because the convolution result by the FFT is based on the circular convolution, the first (M−1)/2 points of this vector are 'corrupted' by circulation. Thus, we will skip the first (M−1)/2 points, and read the next Nx values to define a vector →S as the convoluted result. See Figure 5. We note that the complexity for the FFT Gaussian convolution along one grid line is O(NflogNf). The entire GCS algorithm involves the discrete Gaussian filtering in x, y and z directions, and for all grid lines. The overall complexity asymptotically equals to O(N3logN3), where N3=Nx×Ny×Nz is the the total degree of freedom (DOF). It is noted that since the width M of the Gaussian kernel K(x) is proportional to Nx, the direct convolution using Eq (3.7) will result in a complexity O(N23). This is much slower than the proposed FFT procedure.
At last, we need to process the generated discrete function S(xi,yj,zk) to cancel numerical artifacts. Even though the present Gaussian kernel is properly defined so that the convolution could be confined within a band of 3Å, the numerical value of S could be slightly less than 1 inside ˜Ωi, say 0.9999. In fact, such a long tail smoothing is because the Fourier component of the Gaussian function is not a band-limited function in the frequency domain. This is a well known drawback of the Gaussian filtering. To rectify this, we usually conduct a post-processing after convolutions. For any node (xi,yj,zk) inside ˜Γi, we simply reset S=1. Similarly, for any node (xi,yj,zk) outside ˜Γe, we will force S=0. The S values between two boundaries will not be changed. Because the long tail smearing is so weak, the rectified discrete function S(xi,yj,zk) are still sufficiently smooth for the regularization analysis.
Mathematically, the solute domain Ωi, transition region Ωt, solvent domain Ωe, and their boundaries Γi and Γe can be defined based on the final S(xi,yj,zk). For example, Γi and Γe could be represented as an isosurface S=1 and S=0, respectively. For most parts, these domains and boundaries will be close to their counterparts defined initially in Figure 4a. Nevertheless, the new boundaries Γi and Γe will become very smooth, which is different from ˜Γi and ˜Γe. Moreover, our GCS algorithm is designed to guarantee that ˜Ωi⊂Ωi and ˜Ωe⊂Ωe. Finally, we note that the explicit definition of domains and boundaries are actually unnecessary, because in our regularization computation, we just need to know nodal values of the GSC surface function S(xi,yj,zk).
In this subsection, we validate the GCS model numerically by considering some simple systems. In all tests, a cubic domain Ω=[−10,10]3 is employed. A uniform grid with mesh size N=Nx=Ny=Nz is used with a spacing h=20N−1.
The Gaussian kernel in Eq (3.1) contains only one parameter: the variance σ, which controls the shape of Gaussian function. We first explore how σ2 influences the GCS by studying a one-atom model with radius R=2 and the atom center located at the origin (0,0,0). Because of a simple geometry, the boundaries Γi and Γe could be fixed in the one-atom model throughout the Gaussian filtering, and are chosen as spheres with radii ri=2 and re=5, respectively. In Figure 6, we plot S along a x grid line y=z=0 by considering different σ2 values and N=401 for one atom system. Due to the design of the GCS algorithm, all cases have the same S values for |→r|<ri=2 and |→r|>re=5. For the transition layer ri≤|→r|≤re, the GCS shape depends on σ2. In particular, as σ2 becomes smaller, the decay from S=1 to S=0 becomes steeper. It can be seen in Figure 6 that as σ2→0, the GCS curve has a tendency to converge to a Heaviside function with radius r=3.5. However, such a theoretical convergence is impossible to be realized numerically with a finite mesh size N=401. As can be seen in Figure 6b, the GCS surface is still quite smooth when σ2 is as small as 10−20. Moreover, a too small σ2 value is usually not welcomed in the PB simulations, because the central difference will yield a large numerical error in resolving a rapid ϵ change. On the other hand, when σ2 is too large, it can be seen that the decay becomes too slow in Figure 6b. The post-processing of S becomes critical in this case. Otherwise, one may concern about the smoothness of S near ri and re. For simplicity, a median value such as σ2=1 is what we recommend for general computations.
We next study a diatomic system with both radii being R=2 and centers located at (±3,0,0). In Figure 7, the GCS heat-map figures for the diatomic system are shown. By using N=401, the initial Heaviside function is plotted in part (a), while other parts use different σ2 values. Similar to the one atom case, σ2 controls the steepness of decay in the transition layer. Moreover, the smoothness of the GCS diffuse interface is well illustrated in Figure 7. For this diatomic system, the VdW surface ˜Γi consists of two isolated spheres, and the enlarged SAS ˜Γe looks like the SAS, i.e., it involves two geometrical singularities, see Figure 7a. Nevertheless, after Gaussian filtering, domains and boundaries become sufficiently smooth. In particular, the solute domain Ωi becomes a connected region with ˜Ωi⊂Ωi. For the solvent domain, we also have ˜Ωe⊂Ωe. In Ωt, S has a smooth decay from 1 to 0 in any direction.
To further illustrate the smoothness of the GCS model, we change the distance between two atom centers by fixing σ2=1 and other parameters, see Figure 8. In particular, six cases are considered with the atom centers being chosen as (±2,0,0), (±2.3,0,0), (±2.6,0,0), (±2.9,0,0), (±3.2,0,0), and (±3.5,0,0). In the first case, the VdW surface ˜Γi involves two touched spheres, while in the last case, the enlarged SAS ˜Γe also consists of two touched spheres. After Gaussian smoothing, a connected solute domain Ωi is resulted in the first five cases, while Ωi becomes two isolated spheres in the last case. In all cases, S is sufficiently smooth, especially near the origin in the last case. These qualitative studies indicate that the GCS model provides a suitable diffuse interface for the proposed regularization. In the rest of this paper, unless specified otherwise, we will fix σ2=1 in all GCS studies.
When N goes to ∞ or h→0, the GCS will approach certain limit. Nevertheless, a finite N has to be used in practice. We next explore how N influences the GCS, by considering the same one atom system studied above. In Figure 9a, we plot S under different grid sizes N=51,101,...401, along the x-axis with y=0 and z=0. Visually, all curves coincide with each other, except the one with N=51, which is a little bit away from the others. It gives us a rough idea that changing grid size will not cause much difference on the shape of GCS surfaces.
Two zoom-in plots are shown in Figure 9 to allow us to see some subtle changes. In particular, in Figure 9b, we focus on a region to the right of x=−re=−5 or in the orange box of part (a). In Figure 9c, details are shown for a region to the left of x=−ri=−2 or in the green box of part (a). In the transition layer [−re,−ri], curves with different N cross each other frequently. With a fine enough resolution in part (c), the curves are separated so that we can read the order of N values according the position of curves from top to bottom. The order is "51→101→251→301→151→351→401→201", which is also shown in the legend. For Figure 9b, the zoom-in resolution is insufficient, so that the curves are still clustered. The order from top to bottom is tentatively given as "401→151→351→201→301→101→251→51", and shown in the legend. We note that these two sequences are given roughly because the curves cross each other and change their relative positions frequently. What is interesting is that these two sequences have completely different orders. In general, this means that the GCS convergence with respect to N is not monotonic. Consider a "ground truth" curve as the asymptotic limit, the GCS curve will not converge to this limit from left, nor from right. Instead, the GCS curve will oscillate around the limit in a random fashion. In next section, we will show that the electrostatic free energy sensitively depends on the GCS curve near Γi, so that the energy convergence with respect to N is also oscillatory.
To comprehend such oscillations, two diagrams are considered in Figure 10, where a "ground truth" sharp interface is fixed. In each diagram, two uniform meshes are shown. The interface representation problem underlying this study can be formulated like this: based on each coarse mesh, the sharp interface is approximated by a reconstructed interface (not shown) by certain approach. Now without knowing the sharp interface explicitly, the convergence of this approach can only be assessed by examining two reconstructed interfaces, which, unfortunately, are affected by the particular locations of the grid nodes with respect to the sharp interface. In the top subfigure of Figure 10, grid nodes with two general N values, such as N=15 and N=21, are plotted. These nodes are mostly different, except for a few points. Consequently, the interfaces reconstructed based on two independent meshes are quite irrelevant. This is essentially why the convergence pattern in Figure 9 by using a general sequence of N is oscillatory.
This motivates us to study the convergence by considering a special sequence of N. In the bottom part of Figure 10, the mesh spacing is halved from N=11 to N=21. In this manner, the denser mesh keeps all original nodes of the coarser mesh, while adding more nodes. It is expected that the interface information contained in the reconstruction on the coarser mesh is preserved with newly added information. A better convergence pattern could be resulted.
In Figure 11, we display the GCS curves of one atom system by considering N=51,101,201, and 401. Similarly, two zoom-in plots are also given. It can be observed that the convergence is still not monotonic, but becomes more regular. In particular, if we take the GCS curve for N=401 as the reference solution, and measure the distance of other curves away from it. Such a distance obviously becomes smaller when N increases. Such a convergence can be illustrated visually and quantitatively. Qualitatively, we can take the surface function S for N=401 as the reference solution Sref. Note that all grid nodes of the other three mesh sizes are also nodes for N=401. This enables us to calculate the absolute difference |SN−Sref| point-wisely, where SN is the surface function under grid size N=51,101, or 201. Such differences are depicted in Figure 12 as surface plots on the plane z=0. The convergence is evidently. Quantitatively, we measure the differences by using the L2 norm: L2=√∑i,j,k|SN−Sref|2/N3, and the results are found to be 1.4E-2, 3.5E-3, and 6.9E-4, respectively, for N=51, 101, and 201. This is obviously a convergence on the order of O(h2). Therefore, in the following studies, when we examine the GCS convergence, we will take N=51,101,201, and 401.
In this section, we will validate the regularization method and the GCS model by solving the linearized PB (LPB) equation for several simple atomic systems. Comparison of the regularization method and the trilinear technique will be conducted too. In all cases, a cubic domain Ω=[−10,10]3 is partitioned by a uniform mesh with the spacing h=20N−1 in all directions. Here the length unit for h is Å.
Consider the one atom system studied in Section 3.3 with center at (0,0,0) and radius 2. We first define an analytical smeared surface to verify the proposed regularization method. The boundaries Γi and Γe are spheres with radii being ri=2 and re=5, respectively. In Ωi and Ωe, the level set function takes constant values S(→r)=si=1 and S(→r)=se=0, respectively. In the transition band ri<|→r|<re, it takes the form
S(→r)=se−si2tanh(k(|→r|−rire−ri−0.5)+1)+si. | (4.1) |
where the parameter k controls the steepness of transition. We choose k=6, which is large enough to ensure that S(→r) is a smooth function throughout the domain Ω. This diffuse interface will be called tanh-like surface in the following discussions.
We first demonstrate how error cancellation is so crucial for the trilinear method in calculating the electrostatic free energy. Consider a point charge q1=1 at the atom center (0,0,0). In Figure 13, the result of the regularization method is compared with that of the trilinear method in two subfigures. Line plots of potentials along a x line with y=0 and z=0 are shown. As mentioned in Section 2.4, the electrostatic free energy depends on u(→r)−G(→r). However, the trilinear method produces a huge error in approximating the singular source q1. Thus, the plot of uTL−G has a sharp spike near x=0. In fact, since G(→r) is undefined at x=0, in this plot, we re-defined the grid value G(0,0,0) as the average of six nearest neighboring nodes. For the regularization method, Green's function G is analytically excluded so that the reaction field potential uRF is free of singularity. Being invisible in Figure 13a, the difference between two potentials uTL−G and uRF is actually quite minor away from the point charge.
As suggested in Eq (2.21), in calculating the electrostatic free energy by the trilinear method, one will not use the Green's function directly. Instead, one numerically solves the Poisson's equation (2.20) to obtain an approximated Green's function u0. The plot of uTL−u0 against uRF in Figure 13b shows the power of error cancellation. The error of singular source approximation has been compensated, because the same approximation is invoked twice in uTL and u0. Consequently, uTL−u0 also yields a constant potential inside the sphere, and behaves similarly to the uRF. The height difference between uTL−u0 and uRF becomes smaller when N is larger.
We next examine the electrostatic free energies for the one atom system with one point charge at the its center and tanh-like surface. The numerical results of the trilinear and regularization methods are listed in Table 1 for various mesh sizes. For the regularization method, because the level function S(→r) is analytically defined for the tanh-like surface, all terms in the regularized source of Eq (2.16) can be computed analytically, including ∇ϵ. Thus, in this example, the numerical error of the regularization is solely from finite difference discretization of the PB equation. When h becomes smaller, the energy of the regularization method quickly approaches to a limit around −65.6 (kcal/mol). For the trilinear method, the charge distribution is actually not conducted, because for all odd N values, the charge center (0,0,0) is a grid node. Thus, one simply approximates the delta function by 1h3 on that node. Even though the error cancellation trick has been applied, the energy predicted by the trilinear method is still inaccurate. When h is as small as h=0.05, the energy is still not close to that of the regularization method. With large h values, the errors are significant.
Electrostatic free energy | |||||
One charge | Two charges | ||||
N | h | Trilinear | Regularization | Trilinear | Regularization |
11 | 2 | –175.3344 | –56.8468 | –414.6217 | –271.3451 |
21 | 1 | –94.2773 | –64.8951 | –486.4823 | –310.5806 |
31 | 0.6667 | –79.4891 | –63.9885 | –419.0835 | –300.3787 |
41 | 0.5 | –74.9363 | –64.7367 | –380.6996 | –303.0649 |
51 | 0.4 | –72.7830 | –65.1096 | –364.9318 | –305.7947 |
101 | 0.2 | –69.6594 | –65.4422 | –339.0436 | –305.1510 |
201 | 0.1 | –68.8730 | –65.6210 | –329.3492 | –304.1162 |
401 | 0.05 | –68.6046 | –65.6644 | –326.3818 | –303.7689 |
To fully illustrate the approximation error of the trilinear distribution, we next consider the same one atom system and tanh-like surface with two charges q1=q2=1 located at (±1.475,0,0). In this way, these two charges are not located on grid nodes for all tested N values, so that trilinear distributions are indeed carried out in all cases. The potentials generated by trilinear and regularization are depicted in Figure 14. It can be seen that the potential is no longer flat inside the sphere. We note that charge centers are close to the molecular surface in the present study, which mimics the usual protein problems. Due to an intensive interaction between charges and ∇ϵ in diffuse interface, the potential changes abruptly near the VdW surface x=±2. This produces a strong grid error, especially when h is large for the trilinear method. The regularization method performs robustly for this difficult problem with a fast convergence. As can be seen in Table 1, energies for h<1 are all around −303 and the relative difference between N=201 and N=401 is as small as 0.11%. The energies predicted by the trilinear method are not close to those of the regularization. In particular, taking the energy of the regularization at N=401 as a reference, the relative error of the trilinear method at h=0.5 is about 25.33%. Since h=0.5 is the mostly commonly used mesh spacing in real protein applications, the trilinear energies are simply unreliable.
To further compare the regularization with the trilinear method, their CPU time for solving the one-atom system is reported in Table 2. It can be seen that in most cases, the trilinear method demands more CPU time than that of the regularization, because it needs to additionally solve the Poisson equation (2.20) for calculating the electrostatic free energy. Here a biconjugate gradient iterative algorithm [38] is employed for solving both the PB and Poisson equations. For the regularization, the computation of the discrete source terms takes more time, so that for a large N like N=401, it becomes more expensive in the case of two charges.
CPU time (s) | |||||
One charge | Two charges | ||||
N | h | Trilinear | Regularization | Trilinear | Regularization |
11 | 2 | 6.230E−4 | 4.610E−4 | 7.490E−4 | 5.610E−4 |
21 | 1 | 9.498E−3 | 5.594E−3 | 1.096E−2 | 6.531E−3 |
41 | 0.5 | 1.921E−1 | 1.149E−1 | 2.134E−1 | 1.520E−1 |
51 | 0.4 | 4.537E−1 | 3.058E−1 | 1.026E+0 | 7.435E−1 |
101 | 0.2 | 1.248E+1 | 8.003E+0 | 1.379E+1 | 9.220E+0 |
201 | 0.1 | 1.845E+2 | 1.114E+2 | 2.205E+2 | 1.754E+2 |
401 | 0.05 | 3.641E+3 | 1.712E+3 | 2.564E+3 | 3.054E+3 |
For the one atom studies above, tanh-like smeared surface can be constructed analytically. However, such surface cannot be applied to more complicated molecules. In order to test the regularization method for real problems, we have introduced an efficient FFT algorithm to generate a Gaussian convolution surface (GCS). For simplicity, we will call the proposed regularization method and GCS model as the REG-GCS method. In this subsection, we first validate the REG-GCS algorithm for several atomic systems.
We first investigate the impact of the GCS model by considering again the one atom system. Here σ=1 is used. One point charge q1=1 will be assumed at (0,0,0), and is treated by the regularization method. Electrostatic free energies calculated based on both tanh-like and GCS surfaces are presented in Table 3. Obviously, the energy converges to a different limit when a different diffuse interface is employed. Another subtle difference can be seen in Figure 15a for the convergence lines with respect to all N values. For the tanh-like surface, the same analytical surface is utilized for all N values. Moreover, all source terms in the regularized PB equations are analytically calculated. Consequently, the convergence pattern of the regularization method for the tanh-like surface is superior---the energy converges monotonically and becomes almost flat for large N values. On the other hand, the GCS convergence line is oscillatory in Figure 15a. Moreover, the magnitude of such oscillation decays, but does not decay quickly when even larger N values are used. The convergence is impacted in two ways. First, the GCS surface is constructed based on a given h value and the fixed width re−ri=3. In other words, a different smeared surface is used for a different N. Thus, the energy convergence line includes not only the numerical convergence of the regularization method for solving the PB equation, but also the convergence of GCS surface. Second, with the level set function S being defined numerically, ∇ϵ in the regularized sources has to be approximated numerically, which further impact the convergence of the PB solver.
Electrostatic free energy | |||
N | h | tanh-like | GCS |
51 | 0.4 | –65.1096 | –67.3895 |
101 | 0.2 | –65.4422 | –67.7956 |
151 | 0.1333 | –65.5656 | –68.5860 |
201 | 0.1 | –65.6210 | –69.0848 |
251 | 0.08 | –65.6477 | –67.6126 |
301 | 0.0667 | –65.6423 | –68.1096 |
351 | 0.0571 | –65.6568 | –68.4918 |
401 | 0.05 | –65.6644 | –68.7645 |
In Section 3.3, we have discussed how grid size N influences the GCS model. The surface function S(→r) has been shown to oscillate with respect to N in the transition region, and the essential reason of such oscillation has been discussed. In particular, the GCS line plots near Γi roughly follow the order "51→101→251→301→151→351→401→201" as shown in Figure 9c, with some lines crossing each other. Since the zoom-in region is close to the charge center, we expect a correlation between the surface plot order and the sorted energy order. The energies in Table 3 can be re-ordered from large to small, which gives an order "51→251→101→301→351→151→401→201". By comparing these two orders, we found that they are mostly the same. There are only two tuples (101,251) and (351,151) in the GCS order that are switched in the energy order. This mismatch could be due to the selected window for the zoom-in plot. We note that the the GCS order is changing from place to place. So the present GCS order may have minor differences comparing with the surface plot sequences in the innermost transit region. Moreover, for these two tuples, their energy differences are about 0.1, which is less than the total average difference of the value about 0.3. For these reasons, the oscillatory energy convergence pattern is believed to be due to the GCS convergence.
Like we mentioned in the Section 3.3 about the GCS convergence, a monotonic convergence is unlikely when a general sequence of N is adopted. Nevertheless, if we choose a sequence of doubly refined meshes such as 51,101,201,401, the interface information contained in the coarse mesh will be inherited in the fine mesh. This will results a better convergence in the GCS surface construction, as well as the energy calculation. In Figure 15b, we limited ourselves to this special sequence of N, and plot energies of the tanh-like and GCS surfaces. It can be seen that the energy convergence for the GCS is still not monotonic. However, the magnitude of oscillation will decay quickly when h is further halved. In particular, we take the energy at N=401 as the reference value, and plot the absolute errors for N=51, 101, and 201 in Figure 15c. The self-convergence now becomes evidently and is monotonic. This agrees with the finding presented in Figure 11c for the GCS convergence by using the same sequence 51,101,201,401. In the following studies, we will test the convergence of the REG-GCS algorithm by only considering the doubly refined sequence N=51,101,201, and 401.
By using N=401, we next test how the free energy depends on the GCS parameter σ, by studying the same one-atom system. As we mentioned before, as σ→0, the GCS theoretically converges to a sharp interface. In this case, this is the SAS with radius r=3.5, whose analytical energy is known to be −46.8447 (kcal/mol). It is interesting to examine if the GCS energy could converge to −46.8447 (kcal/mol) as σ goes to zero. However, we should emphasize that such a σ convergence is numerically unreachable. As shown in Figure 6b, the GCS is still a smooth diffuse interface at σ=10−10, which is still far away from the Heaviside function, while an even smaller σ will be beyond the numerical resolution of the grid size N=401. In the present study, the electrostatic free energies obtained by taking σ to be 1,10−2,10−4,10−6,10−8, and 10−10 are plotted in Figure 16 against −log10σ. It can be seen that starting from −68.7645 (kcal/mol) at σ=1, the GCS free energy becomes closer to −46.8447 (kcal/mol) as σ becomes smaller, and shows a good tendency towards the sharp interface limit. In the rest of studies, we will fix σ=1 for simplicity.
We next study a diatomic system with two atoms of the same radius 2. At two centers (±10/3,0,0), two point charges q1=q2=1 are assumed. The surface plot of the potential uRF calculated by the REG-GCS method is shown in Figure 17a. Besides the surface plot over the plane z=0, the contour plot is also shown at the bottom of Figure 17a. It can be seen that uRF is no longer flat inside two atoms. The smoothness of the potential contour plot also indicates the smoothness of the GCS diffuse interface. The self-convergence analysis of the electrostatic free energy is depicted in Figure 17b. As above, we treat the energy at N=401 as exact, and calculate absolute errors for N=51, 101, and 201. Again, for this doubly refined sequence, the REG-GCS energy converges monotonically.
In this section, we carry out numerical experiments to test the performance of the proposed regularization method and GCS diffuse interface for solving real biological systems. Considering the LPB model, we will examine the convergence of the REG-GCS algorithm by studying small compounds and proteins. Salt affinities of protein complexes will also be studied. The dielectric coefficients are chosen as ϵi=1 and ϵe=80. The ionic strength is taken as I=0.15 M, except in studying the salt effect.
We first verify the numerical convergence of the proposed REG-GCS method by considering a set of 17 small compounds. The charges, atomic coordinates, and radii are defined based on a parameterization presented in [39]. The solvation free energies of this test set have been studied by using different methods in the literature [39,40]. We note that the present algorithm is designed to compute the polar component of the solvation free energy. Thus, without non-polar components [40], the present results cannot be directly compared with those in the literature. This test set is adopted in the present study, because of small sizes of these molecular systems. This allows to use dense meshes to verify the numerical convergence.
Since the physical dimensions of 17 compounds are different, we will adjust spacing h instead of mesh size N in the present study. For each compound, we will choose a fixed computational domain Ω and partition it into uniform meshes by using h=0.4,0.2,0.1 and 0.05Å. Note that h is halved in each refinement, in order to maintain the convergence pattern of the proposed method. The electrostatic free energies computed by the REG-GCS method are listed in Table 4. A clear convergent trend is seen in all compounds. To see the converging pattern better, we set the energy calculated by h=0.05Å (densest case) as the reference value for each molecule. The absolute errors of the other meshes are calculated and depicted in Figure 18. It can be seen that the absolute error approaches to zero in a monotonic manner. This agrees with our previous findings for atomic systems.
Compound | h=0.4 | h=0.2 | h=0.1 | h=0.05 |
glycerol triacetate | –5.5859 | –5.8435 | –6.2175 | –6.1240 |
benzyl bromide | –2.4127 | –2.5128 | –2.6296 | –2.5913 |
benzyl chloride | –2.5949 | –2.5944 | –2.7114 | –2.6707 |
m-bis(trifluoromethyl)benzene | –1.6184 | –1.6538 | –1.7213 | –1.7017 |
N, N-dimethyl-p-methoxybenzamide | –4.7921 | –4.9632 | –5.2105 | –5.1445 |
N, N-4-trimethylbenzamide | –4.0717 | –4.2108 | –4.4131 | –4.3589 |
bis-2-chloroethyl ether | –1.7917 | –1.8995 | –2.0339 | –1.9984 |
1, 1-diacetoxyethane | –4.0385 | –4.1670 | –4.3924 | –4.3299 |
1, 1-diethoxyethane | –1.6122 | –1.7166 | –1.8364 | –1.8095 |
1, 4-dioxane | –2.6011 | –2.6957 | –2.8760 | –2.8283 |
diethyl propanedioate | –3.8696 | –4.0384 | –4.2788 | –4.2165 |
dimethoxymethane | –1.8382 | –1.9678 | –2.1123 | –2.0750 |
ethylene glycol diacetate | –3.9456 | –4.1291 | –4.3829 | –4.3154 |
1, 2-diethoxyethane | –1.5662 | –1.6326 | –1.7431 | –1.7139 |
diethyl sulfide | –1.3971 | –1.4242 | –1.4866 | –1.4696 |
phenyl formate | –3.9594 | –4.0447 | –4.2625 | –4.2003 |
midazole | –6.0855 | –6.3074 | –6.6142 | –6.5268 |
We next examine the convergence by considering a protein with protein databank (PDB) ID: 1AHO. A fixed domain Ω=[0,60]3Å is partitioned into uniform meshes with a grid size N=Nx=Ny=Nz. By using N=51,101,201, and 401, the calculated electrostatic free energies are reported in Table 5. If we take the energy with N=401 as the reference, the absolute errors for N=51, 101, and 201, are 220.9509, 41.9946, and 32.7952, respectively, which shows a monotonic converge trend as well.
Surface generation | Solving PB Equation | |||||
N | h | Energy | CPU | Rate | CPU | Rate |
51 | 1.2 | –746.6443 | 0.244 | – | 168.315 | – |
101 | 0.6 | –567.6880 | 1.805 | 7.39 | 61.265 | 0.36 |
201 | 0.3 | –558.4886 | 14.359 | 7.95 | 555.374 | 9.07 |
401 | 0.15 | –525.6934 | 114.464 | 7.97 | 5777.622 | 10.40 |
The CPU time for generating the GCS surface and solving the LPB equation is also reported in Table 5. For each N, the majority of execution time was spent on solving the three-dimensional (3D) PDE by using a biconjugate gradient iterative algorithm [38]. When one halves the spacing, the degree of freedom (DOF) N3 increases by eight times. The CPU increment rate is calculated as the ratio of CPU time values between two mesh levels. It is interesting to note that the CPU increment rate of the GCS surface generation is about eight. This indicates the complexity of the proposed FFT Gaussian convolution algorithm being O(N3logN3) for a 3D DOF N3. For the numerical solution of the LPB equation, the CPU time at N=51 is larger than that at N=101 for this problem. This is because with a coarse spacing h=1.2Å, the finite difference discretization error is quite significant so that the biconjugate gradient solver takes too many iterations. When h is refined to h=0.6Å, the iterative solver actually converges faster so that the CPU time becomes short. When N is large enough, we can see the CPU increment rate is larger than 10, which is normal for the biconjugate gradient method and 3D problems.
We next visualize the GCS surface for the 1AHO. With the GCS diffuse interface, we generate three isosurfaces at S=0.1, S=0.5 and S=0.9. It can be seen in Figure 19 that these three surfaces are equivalent in a homological sense, and becomes bigger and smoother as S decreases. The electrostatic potential uRF computed by the REG-GCS algorithm is mapped onto three isosurfaces. Such potential plots can help us seeing the changing location of electrostatic potential and are helpful to the study of protein interactions. As expected, the intensity of the potential map is stronger when S is larger or closer to the VdW surface. The potential becomes weaker as S is approaching zero.
We next apply the REG-GCS method for calculating electrostatic free energy of proteins by using a fixed mesh spacing h=0.5Å. For this purpose, a set of 21 proteins which have been studied in [11] are chosen. The results are listed in Table 6 under the name REG-GCS. In order to validate our new model, the rMIB algorithm [8] has also been employed to compute the energies. In solving the LPB equation with a sharp interface, the rMIB method treats the molecular surface by using a second order accurate interface algorithm, and uses a two-component regularization to remove charge singularities. Thus, the rMIB method is one of the most accurate algorithms for solving the PB equation [8]. Because of the difference between sharp interface and diffuse interface PB models, the energies of the rMIB method do not agree with those of the REG-GCS. However, certain correlation can be identified.
PDB-ID | Atom number | Total charge | rMIB | REG-GCS | REG-GCS-predict |
1AHO | 962 | 1 | –920.28 | –630.56 | –939.81 |
1C75 | 985 | –6 | –1522.18 | –1172.89 | –1488.11 |
1J0P | 1597 | 8 | –2435.43 | –1881.69 | –2355.66 |
1TG0 | 1029 | –12 | –2797.92 | –2436.10 | –2762.73 |
1X8Q | 2815 | –1 | –2544.05 | –1711.55 | –2501.48 |
1CBN | 639 | 0 | –379.55 | –243.23 | –468.69 |
1G6X | 888 | 6 | –1363.14 | –1069.62 | –1359.67 |
1IQZ | 1171 | –17 | –4210.73 | –3784.43 | –4147.89 |
1IUA | 1207 | –1 | –943.47 | –646.21 | –1019.01 |
1L9L | 1226 | 11 | –2896.89 | –2444.89 | –2822.62 |
1M1Q | 1265 | –7 | –2023.72 | –1548.87 | –1936.72 |
1NWZ | 1912 | –6 | –2086.55 | –1579.30 | –2134.99 |
1OK0 | 1076 | –5 | –1211.65 | –879.69 | –1218.51 |
1TQG | 1660 | –7 | –1736.90 | –1385.71 | –1876.02 |
1VB0 | 913 | 3 | –924.29 | –646.66 | –943.20 |
1VBW | 1056 | 8 | –1683.58 | –1311.53 | –1645.16 |
1W0N | 1756 | –5 | –1760.92 | –1273.34 | –1788.56 |
1X6X | 1732 | 0 | –1559.74 | –1108.59 | –1617.59 |
1XMK | 1268 | 1 | –1253.35 | –872.73 | –1261.35 |
1ZUU | 868 | 3 | –1285.57 | –1001.23 | –1286.09 |
1ZZK | 1252 | 1 | –1348.43 | –1005.53 | –1390.01 |
In Figure 20a, the free energies calculated by the rMIB and REG-GCS models are plotted against the number of atoms for 21 proteins. It can be seen that the difference between two models becomes larger as the atom number increases. In Figure 20b, such difference is shown against the atom number Nm. A linear trend is clearly behind the data points. This motivates us to construct a linear fitting model. Let us define the energy difference as D=E(rMIB)−E(REG-GCS), and assume that it is a linear function of Nm, i.e., the number of atoms. By using the least squares fitting, we have obtained D=−0.2594Nm−59.6969 (kcal/mol).
Consider that it takes a longer computational time by applying the rMIB model, we propose a simple formula to predict the free energy results of the sharp interface PB model by using our diffuse interface PB model. With the REG-GCS energy, we will predict the rMIB energy as E(rMIB)≈E(REG-GCS)−0.2594Nm−59.6969 (kcal/mol). The resulted energies are also shown in Table 6 under the name REG-GCS-predict. Obviously, these predictions are in good agreement with the original rMIB energies. Moreover, the predictions are plotted against the original rMIB energies in Figure 21. The Pearson correlation coefficient of the prediction results and actual rMIB results is found to be r=0.998. This means the prediction model is able to yield the rMIB energies almost precisely identical.
This present study demonstrates the consistency between the REG-GCS model and rMIB model. This is not surprising, because both models share many common counterparts, such as the linearized PB equation, two-component regularization, and second order finite difference discretization. From numerical point of view, the REG-GCS model is easier to implement.
We finally investigate the performance of the proposed REG-GCS model for the evaluation of the salt effect on the binding of ligands, proteins, peptides, etc.. By solving the LPB model, one can calculate the polar component of the binding energy (ΔE), which measures the difference in the electrostatic free energy between the protein complex and its monomers at a salt strength I.
ΔE(I)=EAB(I)−EA(I)−EB(I), | (5.1) |
where EAB(I), EA(I) and EB(I) are the electrostatic free energies of the complex AB, monomer A and monomer B, respectively, at a given ionic-strength I. The polar binding free energy can be further split into salt independent and dependent parts. The salt dependent part can be analyzed by calculating ΔE(I) based on some salt strength I and at the zero salt concentration. By taking difference of these two quantities, the salt dependent part of the binding free energy is defined as [41]
ΔΔE(I)=ΔE(I)−ΔE(0), | (5.2) |
in which the salt independent part in the binding free energy is canceled out. The binding affinity A (kcal/mol2) is then calculated as the slope ratio of the salt-dependent binding energy at certain salt strength I against the natural logarithm of I. Mathematically, the binding affinity A can be defined as
A=∂ΔΔE(lnI)∂(lnI), | (5.3) |
in which lnI is treated as the independent variable, and ΔΔE is regarded as a function of lnI. Numerically, the binding affinity A is estimated by calculating ΔΔE at several salt strengths of I or lnI, and conducting a least square fitting for the slope.
In this investigation, we tested the salt dependence of binding free energy on 7 protein-protein complexes that have been tested in [41,42]. CHARMM force field has been used. The results calculated by the proposed REG-GCS model with h=0.5Å are listed in Table 7. For each complex, several salt strengths of I are calculated within a range. For the E9Dnase-Im9 and Lactoglobulin dimer, the range is [0.02,0.08], while the other complexes use the range [0.02,0.6]. The experiment results of the binding affinity are known for these 7 complexes, and are also shown in the table. For a comparison, numerical predictions by using a sharp interface PB model [41] and a Gaussian dielectric smooth PB model [42] are also included. It can be seen that the salt effect is much weaker for the present diffuse interface PB model.
Binding affinity A | ||||||
Complex | PDB | Experimental | Ref. [41] | Ref. [42] | REG-GCS | REG-GCS-scaled |
E9Dnase-Im9 | 1EMV | 2.17 | 1.29 | 2.18 | 0.50 | 1.97 |
Barnase-Barstar | 1BRS | 0.96 | 0.67 | 1.86 | 0.2 | 0.81 |
Thrombin-Hirudin | 4HTC | 0.82 | 0.90 | 0.69 | 0.38 | 1.48 |
Tem_1-Blip | 1JTG | 0.40 | 0.38 | 0.06 | 0.08 | 0.33 |
Amy2-Basi | 1AVA | 0.35 | 0.37 | 0.29 | 0.086 | 0.34 |
Hemoglobin tetramer | 1A3N | 0.16 | 0.23 | -0.16 | 0.11 | 0.45 |
Lactoglobulin dimer | 1BEB | –1.62 | –0.82 | –1.71 | –0.27 | –1.05 |
In Figure 22, we plot the REG-GCS binding affinity A against the experimental value. With a weak salt effect, the range of the REG-GCS binding affinity is much shorter than that of the experimental data. Nevertheless, our results are actually highly related to the experimental results. The Pearson correlation coefficient between the binding affinities of the REG-GCS method and experimental ones is actually 0.9564.
Physically, the much weaker salt effect is due to the nature of the diffuse interface model. The ionic strength I determines the value of the modified Debye-Hückel parameter κ for the solvent. For sharp interface and Gaussian-dielectric PB models [41,42], the full strength of κ is applied quickly outside the VdW surface or in traditional solvent region. For the present GCS diffuse interface, the salt effect is loaded gradually with (1−S)κ in the transition band, and is fully operated after 3Å away from the VdW surface. Thus, the impact of changing I to the potential u becomes much insensitive in the diffuse interface model. This is essentially why the REG-GCS salt effect becomes weaker, but is still consistent with the experiment data.
An easy fix is carried out. Because of the underestimation, we will amplify the binding affinity of the REG-GCS by a constant m to give Am=mA, such that the scaled salt dependent part of the binding free energy mΔΔE(I) matches the experimental values in a least square sense. The best scaling factor is calculated as m=3.9201. The scaled binding affinity values are listed in Table 7 for the column REG-GCS-scaled. The mean square error equals to 0.13 for such scaled Am and experimental A values. In Figure 22, Am is also plotted against experimental A. Without any distortion, this scaling maintains the same Pearson correlation coefficient 0.9564. For both original and scaled REG-GCS results, we also fit them as straight lines in Figure 22. The corresponding slopes are 0.2075 and 0.8134, respectively. In summary, with an appropriate scaling to account for the hidden physical feature, the REG-GCS diffuse interface PB model produces decent estimates of the binding affinity. It is believed that the scaling factor should be related to the width of the GCS transition layer. This will be explored in a future work.
The main contribution of this work is the introduction of the first regularization method in the literature for treating charge singularities of the Poisson-Boltzmann (PB) model in a diffuse interface setting. Traditionally, only direct methods such as a trilinear distribution of the point charges are available for diffuse interface PB models [9,10,15,16,18,17]. However, by approximating the delta function by a finite quantity, the trilinear method produces a significant error at charge centers. Taking advantage of an error cancellation in calculating the electrostatic free energy, the trilinear method can produce bounded energy estimations. Nevertheless, the source approximation error is still large and makes this method unreliable for large spacing h. On the contrary, the proposed regularization method avoids singularity analytically, and produces a fast convergence in calculating free energies when the diffuse interface is analytically constructed.
In order to validate the proposed regularization for more general problems, we have also introduced a Gaussian convolution surface (GCS) to characterize a smooth solute-solvent boundary for proteins with complex geometries. Physically, beginning with macromolecule interior and moving into the water phase, the polarizability increases gradually, and should not undergo a sharp jump. In other words, the dielectric function ϵ will increase smoothly from ϵi to ϵe over a transition band. This is the physical justification of the diffuse interface modeling. Mathematically, the diffuse interface PB equation with a smooth ϵ function guarantees a better regularity for the potential u near the solute-solvent boundary, i.e., u is C∞ continuous in Ωt. For the sharp interface PB equation, u is merely C0 continuous, so that extra efforts are required for mathematical analysis and numerical computation. Computationally, based on the fast Fourier transform (FFT), the GCS generation is very efficient. In particular, the complexity of the GCS algorithm is O(N3), where N3 is the total degree of freedom. We note N3 value depends on the physical size of protein domain and mesh spacing h. This is different from the SES generation, which only depends on protein domain. Moreover, in testing the performance of the proposed regularization (REG) together with the GCS model, we have found that the energy convergence of the REG-GCS model is dominated by the GCS convergence, which is typically oscillatory for a sequence of general mesh size N. Nevertheless, for a doubly refined sequence of N, the energy convergence pattern becomes regular. We note that the bandwidth of Ωt should be large enough, such as 3Å in the present study. Otherwise, finite difference approximation may not able to capture the change of ∇ϵ in Ωt. Moreover, when the width shrinks or when σ goes to zero, the GCS surface function becomes a Heaviside function. In this case, the present GCS-REG model should not be applied. Instead, one should use various regularization schemes developed for the sharp interface PB model.
In our future studies, it is interested to apply the proposed regularization in other diffuse interface PB models [9,10,15,16,18,17]. Moreover, this study opens a new theoretical direction for developing regularization formulations for other heterogeneous dielectric PB models, such as the super Gaussian PB model [12]. The development of more accurate and efficient numerical algorithms for solving the regularized PB equation with a smooth dielectric profile will also be explored. The GCS model optimization by taking σ as a tunable parameter deserves further investigation.
Siwen Wang and Shan Zhao were supported by the National Science Foundation (NSF) under grant DMS-1812930. Emil Alexov was supported by the National Institutes of Health (NIH) under grants R01GM093937, R01GM125639 and P20GM121342.
All authors declare no conflicts of interest in this paper.
[1] |
N. A. Baker, D. Sept, S. Joseph, M. J. Holst, J. A. McCammon, Electrostatics of nanosystems: application to microtubules and the ribosome, Proc. Natl. Acad. Sci., 98 (2001), 10037–10041. doi: 10.1073/pnas.181342398
![]() |
[2] |
B. Honig, A. Nicholls, Classical electrostatics in biology and chemistry, Science, 268 (1995), 1144–1149. doi: 10.1126/science.7761829
![]() |
[3] |
A. Nicholls, B. Honig, A rapid finite difference algorithm, utilizing successive over-relaxation to solve the Poisson-Boltzmann equation, J. Comput. Chem., 12 (1991), 435–445. doi: 10.1002/jcc.540120405
![]() |
[4] | B. Lee, F. M. Richards, Interpretation of protein structure: estimation of static accessibility, J. Molecular Bio., 55 (1973), 379–400. |
[5] | F. M. Richards, Areas, volumes, packing and protein structure, Ann. Rev. Biophys. Bioeng., 6 (1977), 151–176. |
[6] |
J. A. Grant, B. Pickup, A Gaussian description of molecular shape, J. Phys. Chem., 99 (1995), 3503–3510. doi: 10.1021/j100011a016
![]() |
[7] | S. Ahmed-Ullah, S. Zhao, Pseudo-transient ghost fluid methods for the Poisson-Boltzmann equation with a two-component regularization, Appl. Math. Comput., 380 (2020), 125267. |
[8] |
W. Geng, S. Zhao, A two-component matched interface and boundary (MIB) regularization for charge singularity in implicit solvent, J. Comput. Phys., 351 (2017), 25–39. doi: 10.1016/j.jcp.2017.09.026
![]() |
[9] |
P. Bates, G.W. Wei, S. Zhao, Minimal molecular surfaces and their applications, J. Comput. Chem., 29 (2008), 380–391. doi: 10.1002/jcc.20796
![]() |
[10] |
P. Bates, Z. Chen, Y. H. Sun, G. W. Wei, S. Zhao, Geometric and potential driving formation and evolution of biomolecular surfaces, J. Math. Biol., 59 (2009), 193–231. doi: 10.1007/s00285-008-0226-7
![]() |
[11] |
C. Arghya, Z. Jia, L. Li, S. Zhao, E. Alexov, Reproducing the ensemble average polar solvation energy of a protein from a singlestructure: Gaussian-based smooth dielectric function for macromolecular modeling, J. Chem. Theor. Comput., 14 (2018), 1020–1032. doi: 10.1021/acs.jctc.7b00756
![]() |
[12] |
T. Hazra, S. Ahmed-Ullah, S. Wang, E. Alexov, S. Zhao, A super-Gaussian Poisson-Boltzmann model for electrostatic solvation free energy calculation: smooth dielectric distribution for protein cavities and in both water and vacuum states, J. Math. Bio., 79 (2019), 631–672. doi: 10.1007/s00285-019-01372-1
![]() |
[13] |
L. Li, C. Li, Z. Zhang, E. Alexov, On the dielectric "constant" of proteins: smooth dielectric function formacromolecular modeling and its implementation in DelPhi, J. Chem. Theory Comput., 9 (2013), 2126–2136. doi: 10.1021/ct400065j
![]() |
[14] |
A. Chakravorty, S. Pandey, S. Pahari, S. Zhao, E. Alexov, Capturing the effects of explicit waters in implicit electrostatics modeling: Qualitative justification of Gaussian-based dielectric models in DelPhi, J. Chem. Inf. Modeling, 60 (2020), 2229–2246. doi: 10.1021/acs.jcim.0c00151
![]() |
[15] |
A. Abrashkin, D. Andelman, H. Orland, Dipolar Poisson-Boltzmann equation: ions and dipoles close to charge interfaces, Phy. Rev. Lett., 99 (2007), 077801. doi: 10.1103/PhysRevLett.99.077801
![]() |
[16] |
L. T. Cheng, J. Dzubiella, J. A. McCammon, B. Li, Application of the level-set method to the solvation of nonpolar molecules, J. Chem. Phys., 127 (2007), 084503. doi: 10.1063/1.2757169
![]() |
[17] |
S. Dai, B. Li, J. Liu, Convergence of phase-field free energy and boundary force for molecular solvation, Arch. Ration. Mech. Anal., 227 (2018), 105–147. doi: 10.1007/s00205-017-1158-4
![]() |
[18] |
Y. Zhao, Y. Y. Kwan, J. Che, B. Li, J. A. McCammon, Phase-field approach to implicit solvation of biomolecules with Coulomb-field approximation, J. Chem. Phys., 139 (2013), 024111. doi: 10.1063/1.4812839
![]() |
[19] |
L. Chen, M. J. Holst, J. Xu, The finite element approximation of the nonlinear Poisson-Boltzmann equation, SIAM J. Numer. Anal., 45 (2007), 2298–2320. doi: 10.1137/060675514
![]() |
[20] |
W. Deng, J. Xu, S. Zhao, On developing stable finite element methods for pseudo-time simulation of biomolecular electrostatics, J. Comput. Appl. Math., 330 (2018), 456–474. doi: 10.1016/j.cam.2017.09.004
![]() |
[21] |
J. A. Grant, B. Pickup, A. Nicholls, A smooth permittivity function for Poisson-Boltzmann solvation methods, J. Comput. Chem., 22 (2001), 608–640. doi: 10.1002/jcc.1032
![]() |
[22] | M. J. Schnieders, N. A. Baker, P. Ren, J. W. Ponder, Polarizable atomic multipole solutes in a Poisson-Boltzmann continuum, J. Chem. Phys., 126 (2002), 124114. |
[23] |
R. Egan, F. Gibou, Geometric discretization of the multidimensional Dirac delta distribution - Application to the Poisson equationwith singular source terms, J. Comput. Phys., 346 (2017), 71–90. doi: 10.1016/j.jcp.2017.06.003
![]() |
[24] | W. Rocchia, E. Alexov, B. Honig, Extending the applicability of the nonlinear Poisson-Boltzmann equation: Multiple dielectric constants and multivalent ions, J. Phys. Chem. B, 105 (2001), 6507–6514. |
[25] | W. Rocchia, S. Sridharan, A. Nicholls, E. Alexov, A. Chiabrera, B. Honig, Rapid grid-based construction of the molecular surface and the use of induced surface charge to calculate reaction field energies: applications to the molecular systems and geometric objects, J. Comput. Chem., 23 (2002), 128–137. |
[26] | P. Benner, V. Khoromskaia, B. Khoromskij, C. Kweyu, M. Stein, Computing electrostatic potentials using regularization based on the range-separated tensor format, preprint, arXiv: 1901.09864v1. |
[27] |
Q. Cai, J. Wang, H. K. Zhao, R. Luo, On removal of charge singularity in Poisson-Boltzmann equation, J. Chem. Phys., 130 (2009), 145101. doi: 10.1063/1.3099708
![]() |
[28] | I. L. Chern, J. G. Liu, W. C. Wang, Accurate evaluation of electrostatics for macromolecules in solution, Methods Appl. Anal., 10 (2003), 309–328. |
[29] |
W. Geng, S. Yu, G. W. Wei, Treatment of charge singularities in implicit solvent models, J. Chem. Phys., 127 (2007), 114106. doi: 10.1063/1.2768064
![]() |
[30] |
M. Holst, J. A. McCammon, Z. Yu, Y. C. Zhou, Y. Zhu, Adaptive finite element modeling techniques for the Poisson-Boltzmann equation I, Commun. Comput. Phys., 11 (2012), 179–214. doi: 10.4208/cicp.081009.130611a
![]() |
[31] |
B. Khoromskij, Range-separated tensor decomposition of the discretized Dirac delta and elliptic operator inverse, J. Comput. Phys., 401 (2020), 108998. doi: 10.1016/j.jcp.2019.108998
![]() |
[32] |
D. Xie, New solution decomposition and minimization schemes for Poisson-Boltzmann equation in calculation of biomolecular electrostatics, J. Comput. Phys., 275 (2014), 294–309. doi: 10.1016/j.jcp.2014.07.012
![]() |
[33] | Z. Zhou, P. Payne, M. Vasquez, N. Kuhn, M. Levitt, Finite-difference solution of the Poisson-Boltzmann equation: complete elimination of self-energy, J. Comput. Chem., 11 (1996), 1344–1351. |
[34] | A. Lee, W. Geng, S. Zhao, Regularization methods for the Poisson-Boltzmann equation: comparison and accuracy recovery, J. Comput. Phys., in press, 2021. |
[35] |
C. Xue, S. Deng, Unified construction of Green's functions for Poisson's equation in inhomogeneous media with diffuse interfaces, J. Comput. Appl. Math., 326 (2017), 296–319. doi: 10.1016/j.cam.2017.06.007
![]() |
[36] |
S. Wang, A. Lee, E. Alexov, S. Zhao, A regularization approach for solving Poisson's equation with singular charge sources and diffuse interfaces, Appl. Math. Lett., 102 (2020), 106144. doi: 10.1016/j.aml.2019.106144
![]() |
[37] | M. Holst, The Poisson–Boltzmann Equation: Analysis and Multilevel Numerical Solution, Ph.D thesis, UIUC, 1994. |
[38] | W. H. Press, S. A. Teukolsky, W. T. Vetterling, B. P. Flannery, Numerical Recipes in Fortran: The Art of Scientific Computing, 2nd edition, Cambridge University Press, 1992. |
[39] | A. Nicholls, D. L. Mobley, P. J. Guthrie, J. D. Chodera, V. S. Pande, Predicting small-molecule solvation free energies: An informal blind test for computational chemistry, J. Med. Chem. 51 (2008), 769–-779. |
[40] |
S. Zhao, Operator splitting ADI schemes for pseudo-time coupled nonlinear solvation simulations, J. Comput. Phys., 257 (2014), 1000–1021. doi: 10.1016/j.jcp.2013.09.043
![]() |
[41] |
C. Bertonati, B. Honig, E. Alexov, Poisson-Boltzmann calculations of nonspecific salt effects on protein-protein binding free energies, Biophy. J., 92 (2007), 1891–1899. doi: 10.1529/biophysj.106.092122
![]() |
[42] | J. Zha, L. Li, A. Chakravorty, and E. Alexov, Treating ion distribution with Gaussian-based smooth dielectric function in DelPhi, J. Comput. Chem., 38, 1974-1979, (2017). |
1. | In Kwon, Gwanghyun Jo, Kwang-Seong Shin, A Deep Neural Network Based on ResNet for Predicting Solutions of Poisson–Boltzmann Equation, 2021, 10, 2079-9292, 2627, 10.3390/electronics10212627 | |
2. | Azzam Alfarraj, Guo-Wei Wei, Geometric algebra generation of molecular surfaces, 2022, 19, 1742-5662, 10.1098/rsif.2022.0117 | |
3. | Siwen Wang, Yuanzhen Shao, Emil Alexov, Shan Zhao, A regularization approach for solving the super-Gaussian Poisson-Boltzmann model with heterogeneous dielectric functions, 2022, 464, 00219991, 111340, 10.1016/j.jcp.2022.111340 | |
4. | Yuanzhen Shao, Mark McGowan, Siwen Wang, Emil Alexov, Shan Zhao, Convergence of a diffuse interface Poisson-Boltzmann (PB) model to the sharp interface PB model: A unified regularization formulation, 2023, 436, 00963003, 127501, 10.1016/j.amc.2022.127501 | |
5. | Shan Zhao, Idowu E. Ijaodoro, Mark McGowan, Emil Alexov, Calculation of electrostatic free energy for the nonlinear Poisson-Boltzmann model based on the dimensionless potential, 2024, 497, 00219991, 112634, 10.1016/j.jcp.2023.112634 | |
6. | Mauricio Guerrero‐Montero, Michał Bosy, Christopher D. Cooper, Some Challenges of Diffused Interfaces in Implicit‐Solvent Models, 2025, 46, 0192-8651, 10.1002/jcc.70036 |
Electrostatic free energy | |||||
One charge | Two charges | ||||
N | h | Trilinear | Regularization | Trilinear | Regularization |
11 | 2 | –175.3344 | –56.8468 | –414.6217 | –271.3451 |
21 | 1 | –94.2773 | –64.8951 | –486.4823 | –310.5806 |
31 | 0.6667 | –79.4891 | –63.9885 | –419.0835 | –300.3787 |
41 | 0.5 | –74.9363 | –64.7367 | –380.6996 | –303.0649 |
51 | 0.4 | –72.7830 | –65.1096 | –364.9318 | –305.7947 |
101 | 0.2 | –69.6594 | –65.4422 | –339.0436 | –305.1510 |
201 | 0.1 | –68.8730 | –65.6210 | –329.3492 | –304.1162 |
401 | 0.05 | –68.6046 | –65.6644 | –326.3818 | –303.7689 |
CPU time (s) | |||||
One charge | Two charges | ||||
N | h | Trilinear | Regularization | Trilinear | Regularization |
11 | 2 | 6.230E−4 | 4.610E−4 | 7.490E−4 | 5.610E−4 |
21 | 1 | 9.498E−3 | 5.594E−3 | 1.096E−2 | 6.531E−3 |
41 | 0.5 | 1.921E−1 | 1.149E−1 | 2.134E−1 | 1.520E−1 |
51 | 0.4 | 4.537E−1 | 3.058E−1 | 1.026E+0 | 7.435E−1 |
101 | 0.2 | 1.248E+1 | 8.003E+0 | 1.379E+1 | 9.220E+0 |
201 | 0.1 | 1.845E+2 | 1.114E+2 | 2.205E+2 | 1.754E+2 |
401 | 0.05 | 3.641E+3 | 1.712E+3 | 2.564E+3 | 3.054E+3 |
Electrostatic free energy | |||
N | h | tanh-like | GCS |
51 | 0.4 | –65.1096 | –67.3895 |
101 | 0.2 | –65.4422 | –67.7956 |
151 | 0.1333 | –65.5656 | –68.5860 |
201 | 0.1 | –65.6210 | –69.0848 |
251 | 0.08 | –65.6477 | –67.6126 |
301 | 0.0667 | –65.6423 | –68.1096 |
351 | 0.0571 | –65.6568 | –68.4918 |
401 | 0.05 | –65.6644 | –68.7645 |
Compound | h=0.4 | h=0.2 | h=0.1 | h=0.05 |
glycerol triacetate | –5.5859 | –5.8435 | –6.2175 | –6.1240 |
benzyl bromide | –2.4127 | –2.5128 | –2.6296 | –2.5913 |
benzyl chloride | –2.5949 | –2.5944 | –2.7114 | –2.6707 |
m-bis(trifluoromethyl)benzene | –1.6184 | –1.6538 | –1.7213 | –1.7017 |
N, N-dimethyl-p-methoxybenzamide | –4.7921 | –4.9632 | –5.2105 | –5.1445 |
N, N-4-trimethylbenzamide | –4.0717 | –4.2108 | –4.4131 | –4.3589 |
bis-2-chloroethyl ether | –1.7917 | –1.8995 | –2.0339 | –1.9984 |
1, 1-diacetoxyethane | –4.0385 | –4.1670 | –4.3924 | –4.3299 |
1, 1-diethoxyethane | –1.6122 | –1.7166 | –1.8364 | –1.8095 |
1, 4-dioxane | –2.6011 | –2.6957 | –2.8760 | –2.8283 |
diethyl propanedioate | –3.8696 | –4.0384 | –4.2788 | –4.2165 |
dimethoxymethane | –1.8382 | –1.9678 | –2.1123 | –2.0750 |
ethylene glycol diacetate | –3.9456 | –4.1291 | –4.3829 | –4.3154 |
1, 2-diethoxyethane | –1.5662 | –1.6326 | –1.7431 | –1.7139 |
diethyl sulfide | –1.3971 | –1.4242 | –1.4866 | –1.4696 |
phenyl formate | –3.9594 | –4.0447 | –4.2625 | –4.2003 |
midazole | –6.0855 | –6.3074 | –6.6142 | –6.5268 |
Surface generation | Solving PB Equation | |||||
N | h | Energy | CPU | Rate | CPU | Rate |
51 | 1.2 | –746.6443 | 0.244 | – | 168.315 | – |
101 | 0.6 | –567.6880 | 1.805 | 7.39 | 61.265 | 0.36 |
201 | 0.3 | –558.4886 | 14.359 | 7.95 | 555.374 | 9.07 |
401 | 0.15 | –525.6934 | 114.464 | 7.97 | 5777.622 | 10.40 |
PDB-ID | Atom number | Total charge | rMIB | REG-GCS | REG-GCS-predict |
1AHO | 962 | 1 | –920.28 | –630.56 | –939.81 |
1C75 | 985 | –6 | –1522.18 | –1172.89 | –1488.11 |
1J0P | 1597 | 8 | –2435.43 | –1881.69 | –2355.66 |
1TG0 | 1029 | –12 | –2797.92 | –2436.10 | –2762.73 |
1X8Q | 2815 | –1 | –2544.05 | –1711.55 | –2501.48 |
1CBN | 639 | 0 | –379.55 | –243.23 | –468.69 |
1G6X | 888 | 6 | –1363.14 | –1069.62 | –1359.67 |
1IQZ | 1171 | –17 | –4210.73 | –3784.43 | –4147.89 |
1IUA | 1207 | –1 | –943.47 | –646.21 | –1019.01 |
1L9L | 1226 | 11 | –2896.89 | –2444.89 | –2822.62 |
1M1Q | 1265 | –7 | –2023.72 | –1548.87 | –1936.72 |
1NWZ | 1912 | –6 | –2086.55 | –1579.30 | –2134.99 |
1OK0 | 1076 | –5 | –1211.65 | –879.69 | –1218.51 |
1TQG | 1660 | –7 | –1736.90 | –1385.71 | –1876.02 |
1VB0 | 913 | 3 | –924.29 | –646.66 | –943.20 |
1VBW | 1056 | 8 | –1683.58 | –1311.53 | –1645.16 |
1W0N | 1756 | –5 | –1760.92 | –1273.34 | –1788.56 |
1X6X | 1732 | 0 | –1559.74 | –1108.59 | –1617.59 |
1XMK | 1268 | 1 | –1253.35 | –872.73 | –1261.35 |
1ZUU | 868 | 3 | –1285.57 | –1001.23 | –1286.09 |
1ZZK | 1252 | 1 | –1348.43 | –1005.53 | –1390.01 |
Binding affinity A | ||||||
Complex | PDB | Experimental | Ref. [41] | Ref. [42] | REG-GCS | REG-GCS-scaled |
E9Dnase-Im9 | 1EMV | 2.17 | 1.29 | 2.18 | 0.50 | 1.97 |
Barnase-Barstar | 1BRS | 0.96 | 0.67 | 1.86 | 0.2 | 0.81 |
Thrombin-Hirudin | 4HTC | 0.82 | 0.90 | 0.69 | 0.38 | 1.48 |
Tem_1-Blip | 1JTG | 0.40 | 0.38 | 0.06 | 0.08 | 0.33 |
Amy2-Basi | 1AVA | 0.35 | 0.37 | 0.29 | 0.086 | 0.34 |
Hemoglobin tetramer | 1A3N | 0.16 | 0.23 | -0.16 | 0.11 | 0.45 |
Lactoglobulin dimer | 1BEB | –1.62 | –0.82 | –1.71 | –0.27 | –1.05 |
Electrostatic free energy | |||||
One charge | Two charges | ||||
N | h | Trilinear | Regularization | Trilinear | Regularization |
11 | 2 | –175.3344 | –56.8468 | –414.6217 | –271.3451 |
21 | 1 | –94.2773 | –64.8951 | –486.4823 | –310.5806 |
31 | 0.6667 | –79.4891 | –63.9885 | –419.0835 | –300.3787 |
41 | 0.5 | –74.9363 | –64.7367 | –380.6996 | –303.0649 |
51 | 0.4 | –72.7830 | –65.1096 | –364.9318 | –305.7947 |
101 | 0.2 | –69.6594 | –65.4422 | –339.0436 | –305.1510 |
201 | 0.1 | –68.8730 | –65.6210 | –329.3492 | –304.1162 |
401 | 0.05 | –68.6046 | –65.6644 | –326.3818 | –303.7689 |
CPU time (s) | |||||
One charge | Two charges | ||||
N | h | Trilinear | Regularization | Trilinear | Regularization |
11 | 2 | 6.230E−4 | 4.610E−4 | 7.490E−4 | 5.610E−4 |
21 | 1 | 9.498E−3 | 5.594E−3 | 1.096E−2 | 6.531E−3 |
41 | 0.5 | 1.921E−1 | 1.149E−1 | 2.134E−1 | 1.520E−1 |
51 | 0.4 | 4.537E−1 | 3.058E−1 | 1.026E+0 | 7.435E−1 |
101 | 0.2 | 1.248E+1 | 8.003E+0 | 1.379E+1 | 9.220E+0 |
201 | 0.1 | 1.845E+2 | 1.114E+2 | 2.205E+2 | 1.754E+2 |
401 | 0.05 | 3.641E+3 | 1.712E+3 | 2.564E+3 | 3.054E+3 |
Electrostatic free energy | |||
N | h | tanh-like | GCS |
51 | 0.4 | –65.1096 | –67.3895 |
101 | 0.2 | –65.4422 | –67.7956 |
151 | 0.1333 | –65.5656 | –68.5860 |
201 | 0.1 | –65.6210 | –69.0848 |
251 | 0.08 | –65.6477 | –67.6126 |
301 | 0.0667 | –65.6423 | –68.1096 |
351 | 0.0571 | –65.6568 | –68.4918 |
401 | 0.05 | –65.6644 | –68.7645 |
Compound | h=0.4 | h=0.2 | h=0.1 | h=0.05 |
glycerol triacetate | –5.5859 | –5.8435 | –6.2175 | –6.1240 |
benzyl bromide | –2.4127 | –2.5128 | –2.6296 | –2.5913 |
benzyl chloride | –2.5949 | –2.5944 | –2.7114 | –2.6707 |
m-bis(trifluoromethyl)benzene | –1.6184 | –1.6538 | –1.7213 | –1.7017 |
N, N-dimethyl-p-methoxybenzamide | –4.7921 | –4.9632 | –5.2105 | –5.1445 |
N, N-4-trimethylbenzamide | –4.0717 | –4.2108 | –4.4131 | –4.3589 |
bis-2-chloroethyl ether | –1.7917 | –1.8995 | –2.0339 | –1.9984 |
1, 1-diacetoxyethane | –4.0385 | –4.1670 | –4.3924 | –4.3299 |
1, 1-diethoxyethane | –1.6122 | –1.7166 | –1.8364 | –1.8095 |
1, 4-dioxane | –2.6011 | –2.6957 | –2.8760 | –2.8283 |
diethyl propanedioate | –3.8696 | –4.0384 | –4.2788 | –4.2165 |
dimethoxymethane | –1.8382 | –1.9678 | –2.1123 | –2.0750 |
ethylene glycol diacetate | –3.9456 | –4.1291 | –4.3829 | –4.3154 |
1, 2-diethoxyethane | –1.5662 | –1.6326 | –1.7431 | –1.7139 |
diethyl sulfide | –1.3971 | –1.4242 | –1.4866 | –1.4696 |
phenyl formate | –3.9594 | –4.0447 | –4.2625 | –4.2003 |
midazole | –6.0855 | –6.3074 | –6.6142 | –6.5268 |
Surface generation | Solving PB Equation | |||||
N | h | Energy | CPU | Rate | CPU | Rate |
51 | 1.2 | –746.6443 | 0.244 | – | 168.315 | – |
101 | 0.6 | –567.6880 | 1.805 | 7.39 | 61.265 | 0.36 |
201 | 0.3 | –558.4886 | 14.359 | 7.95 | 555.374 | 9.07 |
401 | 0.15 | –525.6934 | 114.464 | 7.97 | 5777.622 | 10.40 |
PDB-ID | Atom number | Total charge | rMIB | REG-GCS | REG-GCS-predict |
1AHO | 962 | 1 | –920.28 | –630.56 | –939.81 |
1C75 | 985 | –6 | –1522.18 | –1172.89 | –1488.11 |
1J0P | 1597 | 8 | –2435.43 | –1881.69 | –2355.66 |
1TG0 | 1029 | –12 | –2797.92 | –2436.10 | –2762.73 |
1X8Q | 2815 | –1 | –2544.05 | –1711.55 | –2501.48 |
1CBN | 639 | 0 | –379.55 | –243.23 | –468.69 |
1G6X | 888 | 6 | –1363.14 | –1069.62 | –1359.67 |
1IQZ | 1171 | –17 | –4210.73 | –3784.43 | –4147.89 |
1IUA | 1207 | –1 | –943.47 | –646.21 | –1019.01 |
1L9L | 1226 | 11 | –2896.89 | –2444.89 | –2822.62 |
1M1Q | 1265 | –7 | –2023.72 | –1548.87 | –1936.72 |
1NWZ | 1912 | –6 | –2086.55 | –1579.30 | –2134.99 |
1OK0 | 1076 | –5 | –1211.65 | –879.69 | –1218.51 |
1TQG | 1660 | –7 | –1736.90 | –1385.71 | –1876.02 |
1VB0 | 913 | 3 | –924.29 | –646.66 | –943.20 |
1VBW | 1056 | 8 | –1683.58 | –1311.53 | –1645.16 |
1W0N | 1756 | –5 | –1760.92 | –1273.34 | –1788.56 |
1X6X | 1732 | 0 | –1559.74 | –1108.59 | –1617.59 |
1XMK | 1268 | 1 | –1253.35 | –872.73 | –1261.35 |
1ZUU | 868 | 3 | –1285.57 | –1001.23 | –1286.09 |
1ZZK | 1252 | 1 | –1348.43 | –1005.53 | –1390.01 |
Binding affinity A | ||||||
Complex | PDB | Experimental | Ref. [41] | Ref. [42] | REG-GCS | REG-GCS-scaled |
E9Dnase-Im9 | 1EMV | 2.17 | 1.29 | 2.18 | 0.50 | 1.97 |
Barnase-Barstar | 1BRS | 0.96 | 0.67 | 1.86 | 0.2 | 0.81 |
Thrombin-Hirudin | 4HTC | 0.82 | 0.90 | 0.69 | 0.38 | 1.48 |
Tem_1-Blip | 1JTG | 0.40 | 0.38 | 0.06 | 0.08 | 0.33 |
Amy2-Basi | 1AVA | 0.35 | 0.37 | 0.29 | 0.086 | 0.34 |
Hemoglobin tetramer | 1A3N | 0.16 | 0.23 | -0.16 | 0.11 | 0.45 |
Lactoglobulin dimer | 1BEB | –1.62 | –0.82 | –1.71 | –0.27 | –1.05 |