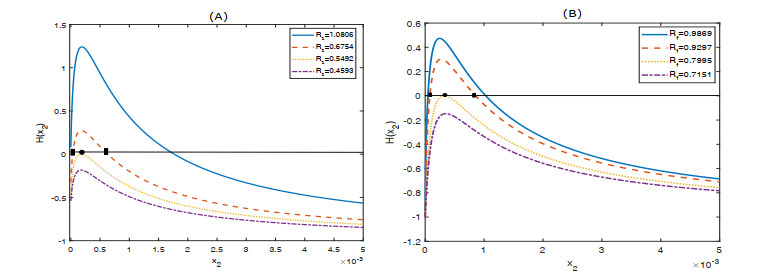
Citation: Yuyi Xue, Yanni Xiao. Analysis of a multiscale HIV-1 model coupling within-host viral dynamics and between-host transmission dynamics[J]. Mathematical Biosciences and Engineering, 2020, 17(6): 6720-6736. doi: 10.3934/mbe.2020350
[1] | Nara Bobko, Jorge P. Zubelli . A singularly perturbed HIV model with treatment and antigenic variation. Mathematical Biosciences and Engineering, 2015, 12(1): 1-21. doi: 10.3934/mbe.2015.12.1 |
[2] | Conrad Ratchford, Jin Wang . Multi-scale modeling of cholera dynamics in a spatially heterogeneous environment. Mathematical Biosciences and Engineering, 2020, 17(2): 948-974. doi: 10.3934/mbe.2020051 |
[3] | Huguette Laure Wamba Makeng, Ivric Valaire Yatat-Djeumen, Bothwell Maregere, Rendani Netshikweta, Jean Jules Tewa, Winston Garira . Multiscale modelling of hepatitis B virus at cell level of organization. Mathematical Biosciences and Engineering, 2024, 21(9): 7165-7193. doi: 10.3934/mbe.2024317 |
[4] | Churni Gupta, Necibe Tuncer, Maia Martcheva . Immuno-epidemiological co-affection model of HIV infection and opioid addiction. Mathematical Biosciences and Engineering, 2022, 19(4): 3636-3672. doi: 10.3934/mbe.2022168 |
[5] | Conrad Ratchford, Jin Wang . Modeling cholera dynamics at multiple scales: environmental evolution, between-host transmission, and within-host interaction. Mathematical Biosciences and Engineering, 2019, 16(2): 782-812. doi: 10.3934/mbe.2019037 |
[6] | Zhaohui Yuan, Xingfu Zou . Global threshold dynamics in an HIV virus model with nonlinear infection rate and distributed invasion and production delays. Mathematical Biosciences and Engineering, 2013, 10(2): 483-498. doi: 10.3934/mbe.2013.10.483 |
[7] | Jinhu Xu, Yicang Zhou . Bifurcation analysis of HIV-1 infection model with cell-to-cell transmission and immune response delay. Mathematical Biosciences and Engineering, 2016, 13(2): 343-367. doi: 10.3934/mbe.2015006 |
[8] | Xinli Hu, Wenjie Qin, Marco Tosato . Complexity dynamics and simulations in a discrete switching ecosystem induced by an intermittent threshold control strategy. Mathematical Biosciences and Engineering, 2020, 17(3): 2164-2178. doi: 10.3934/mbe.2020115 |
[9] | Lin Chen, Xiaotian Wu, Yancong Xu, Libin Rong . Modelling the dynamics of Trypanosoma rangeli and triatomine bug with logistic growth of vector and systemic transmission. Mathematical Biosciences and Engineering, 2022, 19(8): 8452-8478. doi: 10.3934/mbe.2022393 |
[10] | Ning Bai, Rui Xu . Mathematical analysis of an HIV model with latent reservoir, delayed CTL immune response and immune impairment. Mathematical Biosciences and Engineering, 2021, 18(2): 1689-1707. doi: 10.3934/mbe.2021087 |
A number of mathematical models of infectious diseases (e.g., human immunodeficiency virus (HIV)) have been studied at a single microscale or macroscale [1,2,3,4,5]. The single-scale models are proposed to either explore the within-host viral dynamics at the individual level and give guides for treatment strategies, or the between-host transmission dynamics at the population level to predict the future prevalence and suggest effective control measures. However, more evidence indicated that viral load at the individual level affects the progression of infection [6,7]. Laith J et al. found that a higher circulating viral load was positively related to a higher rate of host-to-host transmission [8]. This means that the dynamics at different scales are not independent, but interrelated. Obviously, this also complicates disease control. For example, implementing measures to change behavior patterns may not only affect the disease transmission at the population level, but even the probability of an individual receiving treatment, and further the within-host virus dynamics. Therefore, formulating a multiscale model that can combine the within-host and between-host scales and study the macro-micro interaction mechanism is significant for determining more effective strategies at different levels.
In recent years, many multiscale systems have been proposed in the area of mathematical biology [9,10,11,12,13]. Initially, some researchers built nested models to consider the evolution of the host and parasite by evolutionary dynamics [14,15]. Further, researchers applied coupled models to study infectious diseases. They integrated the within-host model into the epidemiological model by introducing the viral load-dependent transmission rate or disease-induced mortality rate to explore the potential effect of micro dynamics on the macro dynamics, design the coupled optimal scheme and provide the cost-effectiveness analysis [16,17,18]. Later, Feng et al. formulated a multiscale model with an environment compartment to investigate the effects of between-host dynamics on the viral progression within the hosts [19,20]. Taking contaminated environment as a coupling link, Wang et al. coupled the age-structured macro model and the micro within-host dynamics model through the bacteria-dependent indirect transmission [21], Sun et al. proposed a multiscale model with threshold control strategy to study the effect of threshold-dependent interventions on the spread of infectious disease [22], Xiao et al. linked the macroscale to the microscale in a spatiotemporal context to examine effects of an individual movement and spatial control measures on a disease outbreak [23].
It should be noted that most of the above-mentioned multiscale models were formulated by linking viral dynamics to between-host transmission dynamics, which can not reflect the influence of transmission of disease on viral loads within the hosts. It is worth noting that for some environmentally-driven infectious disease such as Toxoplasma gondii, there have been models successfully coupling the macro level to the microscale [19,20,21]. However, for some infectious diseases such as HIV that the virus can be spread by direct contact with infected individuals, how to construct a bridge which can couple the epidemiological model to the virus dynamics model and study the interactions between macro and micro level remain unclear and fall within the scope of our study.
The main purpose of this study is to propose a multiscale model which can bidirectionally couple the within-host viral dynamics and between-host transmission dynamics, and then analyze the dynamic behaviors of the full system. Note that most existing coupled models provided that between-host transmission rate is bilinear incidence, which is inconsistent with the fact that one can only contact with limited persons in a certain time. Hence, we include the standard incidence in our study. Specifically, in this article, we first formulate a multiscale model with standard incidence in section 2. In section 3 and section 4, we analyze the dynamic behaviors of the fast subsystem and slow subsystem, which can help us understand the dynamics of the full system. In section 5, we discuss the interactions of within-host and between-host dynamics by a series of numerical simulations. Finally, the main conclusions are highlighted.
Here, we take HIV viral dynamics and transmission dynamics as an example to illustrate how we form the links on macro and micro levels. The micro system is embedded into the macro system by incorporating the viral load-dependent transmission and disease-induced mortality. The virus shed by the contacted infections, depending on the contact rate and prevalence, enter into the within-host viral system due to close contact and act as a bridge such that between-host system is coupled into the within-host system, inspired by the ideas by Kostova [24], Bhattacharya and Maia Martcheva [25]. In order to investigate the impact of macro epidemic dynamics on micro virus dynamics and vice verse, we proposed a multiscale model, motivated by the sexual contact transmission of HIV [26]. The model equations are as follows.
{dSdt=Π−c1β1(V)SNI−c2β2(V)SNA−μS,dIdt=c1β1(V)SNI+c2β2(V)SNA−(μ+ξ+α1(V))I,dAdt=ξI−(μ+α2(V))A,dTdt=λ−k(1−η)TV−dT,dT∗dt=k(1−η)TV−δT∗,dVdt=c1p1IN+c2p2AN+N1δT∗−cV, | (2.1) |
where S(t),I(t),A(t) are the number of susceptibles, HIV-positive individuals without clinical manifestation and AIDS patients who have developed one or more opportunistic infections regardless of their CD4 count, N(t)=S(t)+I(t)+A(t). T(t),T∗(t), V(t) denote the densities of healthy T cells, infected T cells and viral load, respectively. λ,k,d represent the recruitment, per-capita infection rate, per-capital mortality of healthy cells, δ is the per-capital mortality of infected cells. η is the drug efficacy (0≤η≤1). For HIV infection, it represents the effectiveness of reverse transcriptase (RT) inhibitors. N1 is the virus production rate by an infected cell, c is the clearance rate of virus within host. c1,c2 represent the average number of contacts with HIV-positive individuals and AIDS patients who have developed clinical symptoms, p1,p2 denote the amount of virus released by HIV infections and AIDS patients at each contact. Π,μ denote recruitment rate and natural death rate of hosts. ξ is the transfer rate from HIV-positive stage to the onset of opportunistic infections. β1(V),β2(V) are the transmission probability of HIV-positive individuals and AIDS patients, α1(V),α2(V) are the corresponding disease-related mortality. They are dependent on the viral loads, such as β(V)=aV, or β(V)=aV1+bV, or β(V)=aVq, q<1 with
β(0)=0,β(V)≥0,β′(V)>0,β″(V)≤0. |
Since the system (2.1) contains the dynamics on different time-scales, i.e., within-host dynamics on a fast time-scale and between-host dynamics on a slow time-scale, it is a challenge to directly study the multiscale system. For this problem, some studies analyzed the full system on two time-scales, i.e., fast system and slow system [27,28]. The fast system is obtained by assuming that the slow variables are all constants and the slow system is defined by assuming that the fast system will tend to the stable equilibrium very quickly. This methods allows us to derive the analytical results and reveal some meaningful phenomena of the full system from the analyses of the fast and slow subsystems.
For the within-host system to have a meaningful couple with the between-host system, we focus on the case I,A>0 in the following analysis. We first introduce a slow time-scale τ=ϵt with 0<ϵ≪1 and let
Π=ϵˉΠ,μ=ϵˉμ,ξ=ϵˉξ,α1(V)=ϵˉα1(V),α2(V)=ϵˉα2(V),β1(V)=ϵ¯β1(V),β2(V)=ϵ¯β2(V). |
Then the system (2.1) can be written as
{dSdt=ϵ[ˉΠ−c1ˉβ1(V)SNI−c2ˉβ2(V)SNA−ˉμS],dIdt=ϵ[c1ˉβ1(V)SNI+c2ˉβ2(V)SNA−(ˉμ+ˉξ+ˉα1(V))I],dAdt=ϵ[ˉξI−(ˉμ+ˉα2(V))A],dTdt=λ−k(1−η)TV−dT,dT∗dt=k(1−η)TV−δT∗,dVdt=c1p1IN+c2p2AN+N1δT∗−cV. |
Setting ϵ=0 and ddt=⋅, we get the fast subsystem
{˙T=λ−k(1−η)TV−dT,˙T∗=k(1−η)TV−δT∗,˙V=c1p1ˆIˆN+c2p2ˆAˆN+N1δT∗−cV, | (3.1) |
where ˆI,ˆA are considered as constants. Let E∗f=(˜T∗,˜V,˜T) denote a positive equilibrium of system (3.1). It is obvious that for ˆI>0, the fast subsystem (3.1) has no disease-free equilibrium. In addition, we can get the following result.
Theorem 1 For ˆI>0, the fast subsystem (3.1) has unique positive equilibrium E∗f, which is globally asymptotically stable.
Proof. Let the right sides of system (3.1) be equal to 0 and then we get ˜T∗=λ−d˜Tδ,˜V=(c1p1ˆI/ˆN+c2p2ˆA/ˆN)+N1(λ−d˜T)c and ˜T satisfying
a0˜T2+a1˜T+a2=0, |
where a0=k(1−η)N1d,a1=−[k(1−η)(N1λ+c1p1ˆI/ˆN+c2p2ˆA/ˆN)+cd],a2=cλ.
Solving the above equation, we get
˜T1=−a1+√a21−4a0a22a0,˜T2=−a1−√a21−4a0a22a0 |
with a21−4a0a2>0. Obviously, ˜T1>˜T2>0. For a positive equilibrium, it should satisfy ˜T∗,˜V>0, which corresponds to the condition ˜T<λd. It is easy to verify ˜T2<λd<˜T1. Hence, the system (3.1) has unique positive equilibrium E∗f=(˜T∗,˜V,˜T) with ˜T=˜T2. This completes the proof.
In the following, we verify that E∗f is globally asymptotically stable. Formulating the following Lyapunov function
W(T,T∗,V)=(T−˜T−˜TlnT˜T)+(T∗−˜T∗−˜T∗lnT∗˜T∗)+1N1(V−˜V−˜VlnV˜V), |
then the derivative of W(t) is
dWdt=(1−˜TT)[λ−k(1−η)TV−dT]+(1−˜T∗T∗)[k(1−η)TV−δT∗]+1N1(1−˜VV)(c1p1ˆIˆN+c2p2ˆAˆN+N1δT∗−cV)=d˜T(2−T˜T−˜TT)+δ˜T∗(3−˜TT−˜VT∗˜T∗V−˜T∗TV˜T˜VT∗)+c1p1ˆI/ˆN+c2p2ˆA/ˆNN1(2−V˜V−˜VV)≤0 |
and for all T,T∗,V>0, dWdt=0 holds only at E∗f. By LaSalle's invariant principle, E∗f is globally asymptotically stable.
Writing system (2.1) with respect to the slow time-scale τ, we have
{dSdτ=ˉΠ−c1ˉβ1(V)SNI−c2ˉβ2(V)SNA−ˉμS,dIdτ=c1ˉβ1(V)SNI+c2ˉβ2(V)SNA−(ˉμ+ˉξ+ˉα1(V))I,dAdτ=ˉξI−(ˉμ+ˉα2(V))A,ϵdTdτ=λ−k(1−η)TV−dT,ϵdT∗dτ=k(1−η)TV−δT∗,ϵdVdτ=c1p1IN+c2p2AN+N1δT∗−cV. |
Letting ϵ=0, ddτ=′, and dropping the bar for convenience, we obtain the slow subsystem
{S′=Π−c1β1(˜V)INS−c2β2(˜V)ANS−μS,I′=c1β1(˜V))INS+c2β2(˜V)ANS−(μ+ξ+α1(˜V))I,A′=ξI−(μ+α2(˜V))A, | (4.1) |
where ˜V(S,I,A) is the steady state of the fast subsystem. The initial condition for system (4.1) is S0>0, I0 or A0>0.
By using the next generation method [29], we can calculate the basic reproduction number of the slow system (4.1) as follows
Rs=(μ+α2(˜V(0)))c1β1(˜V(0))+ξc2β2(˜V(0))(μ+ξ+α1(˜V(0)))(μ+α2(˜V(0))), |
where
˜V(0)=limI,A→0˜V(S,I,A)=N1λc(1−1Rf), |
with Rf>1. Here, Rf=λN1k(1−η)cd represents the basic reproduction number of isolated within-host system.
In the following, we first analyze the stability of disease-free equilibrium for the slow subsystem (4.1).
Theorem 2 The disease-free equilibrium Es0=(Πμ,0,0) of slow subsystem (4.1) is locally asymptomatically stable (LAS) for Rs<1 and unstable for Rs>1.
Proof. The characteristic equation of Jacobian matrix at Es0 is
(χ+μ){χ2+[2μ+ξ+α1(˜V(0))+α2(˜V(0))−c1β1(˜V(0))]χ+[(μ+ξ+α1(˜V(0)))(μ+α2(˜V(0)))−(μ+α2(˜V(0)))c1β1(˜V(0))−ξc2β2(˜V(0))]}=0. |
It is easy obtained
χ1=−μ,χ2χ3=(μ+ξ+α1(˜V(0))(μ+α2(˜V(0)))(1−Rs),χ2+χ3=(μ+ξ+α1(˜V(0)))(Rs−1−μ+α2(˜V(0))μ+ξ+α1(˜V(0))−ξc2β2(˜V(0))(μ+ξ+α1(˜V(0)))(μ+α2(˜V(0)))). |
Obviously, for Rs<1, χ2+χ3<0 and χ2χ3>0, that is χ2<0,χ3<0. Thus, the disease-free equilibrium is locally asymptomatically stable. For Rs>1, χ2χ3<0, i.e., there is a positive solution for above characteristic equation. This demonstrates the disease-free equilibrium is unstable. Hence, we obtain the Theorem 2.
If there exists the equilibrium for the slow subsystem, we let E∗s=(˜S,˜I,˜A) denote a positive equilibrium of system (4.1). For the existence and stability of the endemic equilibrium, it is always difficult to be analyzed theoretically with viral load-dependent transmission rate, disease-induced mortality rate and standard incidence, simultaneously. Hence, we provide partial analytic proofs for the case where βi(V) satisfies the properties as mentioned in the Section 2 and αi(V)=αi (i=1,2). Then we extend the results with numerical simulations.
Let ˜x1=c1p1˜I˜N+c2p2˜A˜N, then the equilibrium of the fast subsystem can be written as
˜T∗=λ−d˜Tδ,˜V=˜x1+N1(λ−d˜T)c,˜T=(k(1−η)˜x1+k(1−η)N1λ+cd)−√(k(1−η)˜x1+k(1−η)N1λ+cd)2−4N1dk(1−η)λc2N1dk(1−η). |
Let ˜x2=c1β1(˜V)˜I˜N+c2β2(˜V)˜A˜N, then the equilibrium E∗s of the slow subsystem can be written as
˜I=Π~x2(μ+ξ+α1)(μ+~x2),˜A=ξ˜Iμ+α2,˜S=Π−(μ+ξ+α1)˜Iμ,˜N=˜I+˜A+˜S=Π{(μ+α2)(μ+ξ+α1)(μ+~x2)−[ξα2+α1(μ+α2)]~x2}μ(μ+α2)(μ+ξ+α1)(μ+~x2). |
Further, ˜x1 can be described by ˜x2, that is
˜x1=[(μ+α2)c1p1+ξc2p2]μ˜x2(μ+α2)(μ+ξ+α1)(μ+˜x2)−[ξα2+α1(μ+α2)]~x2. |
Thus, ˜T=˜T(˜x2),˜T∗=˜T∗(˜x2),˜V=˜V(˜x2). Using the equation ˜x2=c1β1(˜V)˜I˜N+c2β2(˜V)˜A˜N, we obtain
˜x2=[(μ+α2)c1β1(˜V)+ξc2β2(˜V)]μ˜x2(μ+α2)(μ+ξ+α1)(μ+˜x2)−[ξα2+α1(μ+α2)]~x2. |
Let
H(x2)=μ[(μ+α2)c1β1(˜V(x2))+ξc2β2(˜V(x2))](μ+α2)(μ+ξ+α1)(μ+x2)−[ξα2+α1(μ+α2)]x2−1, | (4.2) |
then ˜x2 is the solution of equation H(x2)=0. In the following, we only need to identify the number of zeros of H(x2) for x2≥0 by examining the properties of the function H. It can be verified that
H(0)=[(μ+α2)c1β1(˜V(0))+ξc2β2(˜V(0))](μ+α2)(μ+ξ+α1)−1=Rs−1={<0,for Rf>1,Rs<1,>0,for Rf>1,Rs>1,−1,for Rf<1, |
and H(∞)<0.
The derivative of H(x2) is
H′(x2)=μG(x2){(μ+α2)(μ+ξ+α1)(μ+x2)−[ξα2+α1(μ+α2)]x2}2, |
where
G(x2)=[(μ+α2)c1β′1(˜V)+ξc2β′2(˜V)]{(μ+α2)(μ+ξ+α1)(μ+x2)−[ξα2+α1(μ+α2)]x2}˜V′(x2)−[(μ+α2)c1β1(˜V)+ξc2β2(˜V)]μ(μ+ξ+α2). | (4.3) |
We find that the sign of H′(x2) can be determined by G(x2). So, we first analyze the properties of the function G(x2).
G′(x2)=[(μ+α2)c1β″1(˜V)+ξc2β″2(˜V)]{(μ+α2)(μ+ξ+α1)(μ+x2)−[ξα2+α1(μ+α2)]x2}˜V′2(x2)+[(μ+α2)c1β′1(˜V)+ξc2β′2(˜V)]{(μ+α2)(μ+ξ+α1)(μ+x2)−[ξα2+α1(μ+α2)]x2}˜V″(x2)<0, |
with
˜V′(x2)=12cx′1(x2)(1+k(1−η)x1+k(1−η)N1λ+cd√(k(1−η)x1+k(1−η)N1λ+cd)2−4N1dk(1−η)λc)>0, |
˜V″(x2)=12c{(1+k(1−η)x1+k(1−η)N1λ+cd√(k(1−η)x1+k(1−η)N1λ+cd)2−4N1dk(1−η)λc)x″1+[1−(k(1−η)x1+k(1−η)N1λ+cd)2(k(1−η)x1+k(1−η)N1λ+cd)2−4N1dk(1−η)λc]k(1−η)x′21√(k(1−η)x1+k(1−η)N1λ+cd)2−4N1dk(1−η)λc}<0, |
x′1(x2)=μ2(μ+α2)(μ+ξ+α1)[c1p1(μ+α2)+c2p2ξ]{(μ+α2)(μ+ξ+α1)(μ+x2)−[ξα2+α1(μ+α2)]x2}2>0,x″1(x2)=−2μ3(μ+ξ+α2)(μ+α2)(μ+ξ+α1)[c1p1(μ+α2)+c2p2ξ]{(μ+α2)(μ+ξ+α1)(μ+x2)−[ξα2+α1(μ+α2)]x2}3<0. |
The result indicates that the function G(x2) is a monotone decreasing function and G(∞)<0. Thus, if G(0)>0, then with x2 increasing, the function H′(x2)>0 firstly and then H′(x2)<0, which means that the function H(x2) increases firstly and then decreases. In this case, if H(0)<0 and Hmax>0, we can obtain function H(x2) has two positive equilibriums; if H(0)<0 and Hmax=0 or only H(0)>0, there is unique positive equilibrium. If G(0)<0, then the function H(x2) always decreases. In this case, if H(0)<0, then there is no positive equilibrium; if H(0)>0, then there is only one positive equilibrium. In summary, we give the following results.
Theorem 3 Let H=H(x2),G=G(x2) be the function defined in (4.2) and (4.3), Hmax=maxx2 H(x2),
(1) For Rs<1,Rf>1;
(a) If G(0)>0 and Hmax>0, there exists two positive equilibriums E1∗s,E2∗s;
(b) If G(0)>0 and Hmax=0, there is only one positive equilibrium E∗s;
(c) If G(0)≤0 or Hmax<0, there is no positive equilibrium;
(2) For Rs>1,Rf>1, there is only one positive equilibrium E∗s;
(3) For Rf<1;
(a) If G(0)>0 and Hmax>0, there exists two positive equilibriums E1∗s,E2∗s;
(b) If G(0)>0 and Hmax=0, there is only one positive equilibrium E∗s;
(c) If G(0)≤0 or Hmax<0, there is no positive equilibrium.
To demonstrate that there are corresponding parameter values which can satisfy all conditions described in Theorem 3, we plot the curve H(x2) for different parameter sets to show the three cases in which H has 0, 1 or 2 zeros, that is the slow subsystem may have 0, 1 or 2 interior equilibriums. In Figure 1(A), we fix most parameters and change Rs by parameter c2. It shows for 0.5492<Rs<1, the function H(x2) has two zeros meaning there are two interior equilibriums for slow subsystem, but for Rs<0.5492, there is no positive equilibrium. This result demonstrates Rs=0.5492 is a threshold which determines the number of positive equilibrium. In Figure 1(B), we discuss the case of Rf<1. Most parameters have the same values as those in Figure 1(A) except N1. We change Rf by parameter N1. It shows Rf=0.7995 is the lower bound such that the slow subsystem has two interior equilibriums.
In the following analysis, we choose βi(V)=aiV,αi(V)=αi,i=1,2. Then, the Jacobian matrix of the slow subsystem (4.1) at E∗s=(˜S,˜I,˜A) is
J(E∗s)=(−(u1+u2)−μ−(u3+u4)−(u5+u6)u1+u2u3+u4−(μ+ξ+α1)u5+u60ξ−(μ+α2)), |
where
u1+u2=(μ+ξ+α1)˜I(˜V′S˜V+1˜S−1˜N),u3+u4=(μ+ξ+α1)˜I(˜V′I˜V−1˜N)+c1a1˜V˜S˜N,u5+u6=(μ+ξ+α1)˜I(˜V′A˜V−1˜N)+c2a2˜V˜S˜N. |
Let ˜y=1+k(1−η)˜x1+k(1−η)N1λ+cd√(k(1−η)˜x1+k(1−η)N1λ+cd)2−4N1dk(1−η), then
˜V′S=−12c˜N˜x1˜y,˜V′I=12c˜N(c1p1−˜x1)˜y,˜V′A=12c˜N(c2p2−˜x1)˜y. |
Being similar to above description, ˜I,˜S,˜N,˜x1 can be written as the functions of ~x2. The characteristic equation of Jacobian matrix at E∗s is
z3+j2z2+j1z+j0=0, |
where
j0=(μ+ξ+α1)(μ+α2)(μ+u1+u2)−μ(μ+α2)(u3+u4)−μξ(u5+u6),j1=(2μ+ξ+α1+α2)(μ+u1+u2)−(2μ+α2)(u3+u4)−ξ(u5+u6)+(μ+ξ+α1)(μ+α2),j2=3μ+ξ+α1+α2+u1+u2−u3−u4. |
According to Routh-Hurwitz criterion, we need to identify the sign of j0, j1 and j1j2−j0. Specifically, if j0<0, E∗s is unstable, whereas if j0>0 and j1>0,j1j2−j0>0, E∗s is locally stability. It is very difficult to theoretically judge the sign of ji (i=1,2,3), due to the complexity of these functions, so we conduct a large number of numerical simulations. We discover that the Routh-Hurwitz criterion is only satisfied at the larger equilibrium for Rs<1,Rf>1 and is always satisfied at the unique equilibrium for Rs>1,Rf>1. We plot the equilibria and the corresponding stability for different cases in Figure 2: blue dashed line represents unstable equilibriums and black solid line denotes stable equilibriums and give the following conjectures: (1) for Rs<1,Rf>1, if G(0)>0 and Hmax>0, the larger positive equilibrium is locally asymptotically stable, the other is unstable; (2) for Rs>1,Rf>1, the unique positive equilibrium is locally asymptotically stable; (3) for Rf<1, if G(0)>0 and Hmax>0, the larger positive equilibrium is locally asymptotically stable, the other is unstable.
We note that the existence of multiple endemic states and their stability may indicate the possibility of a backward bifurcation for our coupled system. In this section, we provide the detailed numerical studies about the parameter regions in which the bifurcation may occur and simulations illustrating the bistability of the disease-free equilibrium and endemic state. We plot the fraction of infected individuals at the positive steady state ˜I/˜N as a function of Rs and Rf, where the solid and dashed line correspond to the stable and unstable equilibrium, separately. Figure 2(A) shows when Rs<1, there is a lower bound Rsc for Rs, above which the system has two positive equilibriums: one is stable, another is unstable and below which the system has no positive equilibria. This denotes the threshold, which governs the eradication of disease, is Rs=Rsc=0.5492. In other words, the infectious disease can only be controlled until Rs<Rsc. This highlights the challenges in the control of disease. Similar to Figure 2(A), Figure 2(B) shows there exists a stable equilibria and an unstable equilibria for Rf∈(Rfc,1), which demonstrates a backward bifurcation can occur for Rf<1. This result suggests that contact with infected individuals may cause the virus to persist in the population, although the virus can be cleared in the isolated individuals.
In Section 3 and 4, we analyze the dynamics of fast and slow subsystems, which are useful to obtain the theoretical results and reveal some meaningful phenomena of the full system. To confirm the analytical results, particularly the threshold condition for various dynamic behaviors of the full system, we plot the solution curve of the full system. Figure 3(A)-(B) show the time series of fraction of infected individuals for the case Rs<1,Rf>1 and Rf<1, separately. We observe there exists bistable attractors for these two cases. The solutions converge to the disease-free equilibrium with lower initial values of I(0), whereas the solutions converge to the interior equilibrium with higher initial values of I(0). Figure 3(C)-(D) plot the time series of fraction of infected individuals and viral load for the case Rs>1,Rf>1, in which the system has a unique interior equilibrium. The result shows the two time scales for the fast variable V and slow variable I/N. Specifically, the fast variable V quickly approaches the value around its equilibrium, followed by the convergence of the slow variable I/N at a slow rate. What's more, it should be noted that for the case α1(V)=α1V,α2(V)=α2V with bilinear incidence, the system may appear richer dynamics except for the backward bifurcation. Specifically, given suitable parameter values for the case Rs>1,Rf>1, we plot the phase trajectories of the between-host system and the within-host system as shown in Figure 4, in which we can observe the appearance of a stable limit cycle for the full system.
In the following, we will discuss the interactions of within-host and between-host dynamics. To explore the influence of macro transmission dynamics on the micro virus dynamics, we plot the viral load at the equilibrium ˜V versus the fraction of infected individuals at equilibrium ˜I/˜N, contact rate c2 and viral releasing rate per contact p2. Figure 5(A) shows that ˜V is an increasing function of ˜I/˜N, which demonstrates the more infected individuals in the population, the higher the viral loads. In addition, as Rf increases, ˜V also increases. Figure 5(B) gives the variation in the number and the size of equilibrium ˜V with contact rate c2 increasing. For the case Rf=0.8582<1, there exists a critical level ˉc2=13.6 for contact rate c2, above which there are two positive equilibriums: one is stable, the other is unstable and below which there is no positive equilibria. Further, for c2>ˉc2, the larger the contact rate is, the higher the stable equilibrium level of viral loads is. The results suggest that for the coupled within-host dynamic model, Rf=1 is not a threshold to govern the eradication of virus in the hosts. Contact with infected individuals may cause the virus to persist in the population, even if the virus can not be persistent in the isolated individuals. Note that for the case Rf=1.1013>1, there is no positive equilibrium ˜V for c2<5.3. This result implies that having fewer contacts with infected individuals, the virus will eventually be eliminated at the population level even if there are some individuals who carry virus for long time. Figure 5(C) shows that as viral releasing rate p2 increases, the value of stable equilibrium ˜V also increases in the case Rf>1. This demonstrates that the final viral loads may tend to high level if contacts occur with infected individuals whose virus replication is in the active period. The results suggest that limiting contact with infected individuals by some protective measures, especially with those whose virus replication is active, is effective to control virus at a low level.
To investigate the influence of micro parameters on the macro disease transmission, we plot the prevalence at equilibrium ˜I/˜N against drug efficacy η under two cases: case 1): p1=40,p2=50 and case 2): p1=p2=0. In particular, the case 2) with p1=p2=0 means decoupling the macro dynamics from micro model. With assumption αi(V)=αi, Figure 5(D) shows that increasing treatment efficacy η leads to the decline of the prevalence ˜I/˜N. Specifically, ˜I/˜N declines relatively slow when increasing the drug effectiveness initially until a critical level above which the prevalence begins to decline quickly. This indicates that relatively low treatment efficacy is not enough to effectively control the infectious disease. Compared with case 1) p1=40,p2=50, the same drug efficacy leads to a lower disease prevalence for the case 2). Furthermore, the threshold of disease elimination for drug efficacy also reduces from η=0.55 to η=0.47 in the absence of macro to micro coupling. This demonstrates that ignoring the impact of macro transmission dynamics on micro virus dynamics may underestimate the transmission of diseases in the population and consequently the requirement of drug effectiveness.
Generally, for controlling infectious disease, we should implement measures to reduce the basic reproduction number and consequently the new infections. In this model, we can easily observe that Rs decreases with the value of macro parameters ai, ci (i=1,2) decreasing and the value of micro parameter η increasing. However, the existence of backward bifurcation makes the control and elimination of infectious diseases more complicated. To explore the interactions between microscale and macroscale with Rs≤Rsc, we plot the trends of contact rate c2, transmission coefficient a1 and drug effectiveness η at surface Rs=Rsc in Figure 6. If the values of these three parameters are below this surface (i.e., Rs<Rsc), then there is no positive equilibrium and the disease can die out. Otherwise, the disease may be persistent. Furthermore, Figure 6 shows that as η increases, the parameter region of (a1,c2) in which disease doesn't outbreak enlarges. This demonstrates with high drug efficacy, the control measures with relatively low intensity can still eliminate the disease. However, for small drug effectiveness η, in order to eliminate the disease, we should not only reduce the infection rate, but also keep the contact rate at a low level.
Multiscale systems can simultaneously describe the virus dynamics at the individual level and the transmission dynamics at the population level and have become a research focus in recent years. However, there are many challenges to constitute the linkage from the macroscale to the microscale. In this study, we formulate a multiscale model which can link the slow dynamics for disease transmission to the fast dynamics for viral progression, in order to examine the interactions of between-host dynamics and within-host viral dynamics. In fact, coupling the macro dynamics to micro models has been successfully proposed in environmentally driven infections. But, for some infectious disease such as HIV, the virus can be transmitted by direct contact with infected individuals, which may affect the generation rate of virus in microscopic level. Hence, in our model, the influx of viruses from infected individuals to the within-host system, depending on the contact rate and prevalence, becomes a bridge such that between-host dynamics is coupled to the within-host viral dynamics. What's more, the micro system is embedded into the macro system by introducing the viral load-dependent transmission rate and disease-induced mortality rate.
It is worth noting that the factors such as drug efficacy, individual differences and person-to-person contact influence the within-host dynamics, thereby affecting the value of viral load at the equilibrium, which in turn affects the spread of the disease at the population level. Therefore, it is extremely necessary to formulate a coupled model to reflect this feedback mechanism. Moreover, due to the fact that one can only contact a limited number of individuals per unit time, we choose the standard incidence instead of mass action in the model, which is a advantage compared with most existing coupled models. Of course, this also increases the difficulty of theoretical analysis.
We analyze the formulated model by studying the dynamics of the system on different time-scales, i.e., fast subsystem and slow subsystem. We prove that if I>0, the within-host system globally converges to the unique positive equilibrium, which is independent on the threshold Rf obtained from the isolated microscopic model. For the slow subsystem, we find that the system can have zero, one or two interior equilibria, which depends on the magnitudes of Rs and Rf. Specifically, for Rs<1,Rf>1 or Rf<1, there exists a locally stable positive equilibrium and an unstable positive equilibrium. For Rs>1,Rf>1, there is only one locally stable interior equilibria. This demonstrates that the backward bifurcation may occur for the coupled system and Rs=1 can not act as the threshold for infection control. Numerical simulations suggest that the full system exhibits the similar dynamic behaviors to the slow subsystem. For Rs<1,Rf>1 or Rf<1, whether the disease dies out or persists depends on the initial condition.
We numerically examine the synergistic impact between the viral dynamics at the individual level and transmission dynamics at the population level. On the one hand, we observe increasing the drug effectiveness can decrease the prevalence of disease. Previous multiscale models that did not consider the impact of macroscale on microscale may overestimate the control effect of drug treatment on disease transmission. On the other hand, the contact rate may induce the occurrence of backward bifurcation and then affect the number and size of equilibria of viral dynamic model. Contact with infected individuals may cause the virus to persist in the population, even if the virus can not be persistent in the isolated individuals. This emphasizes limiting contact with infected individuals is effective in eliminating virus or controlling virus at a low level at the population level. The existence of backward bifurcation also illustrates the coupled system has more complicated dynamics, and consequently results in the elimination of disease more complex. The suggested control measures may include both the reduction in contact rates or transmission rate at the population level and the increase in drug efficacy at the individual level, and using these control measures together can effectively control the diseases.
In summary, we developed a novel bidirectional coupled model with standard incidence and investigated the synergistic impact between the macro transmission dynamics and micro virus dynamics, which is a advantage compared to most existing multiscale models. We derived some interesting results from this model. The coupled system may appear the backward bifurcation and Hopf bifurcation under certain conditions. Especially, contact rate or drug efficacy is essential to induce these complex dynamic behaviors. The conclusions improve our understanding for prevention and control of infectious disease, which can not be obtained from the pure between-host transmission or within-host viral dynamic models. However, due to the complexity of coupled system, it is challenging to include all progression and transmission routes of infection in the model while providing a complete theoretical analysis.
Although this article is mainly discussed in the context of HIV, the approaches we used are able to be applied more generally in other infectious diseases. Moreover, it should be noted we study the rich dynamics that may occur in our proposed model by theoretical analysis and numerical simulations. However, due to the lack of reliable information on parameter values such as p1,p2, our simulations remain more qualitative. For example, the critical level of contact rate c2 with two positive equilibria may be larger than the real situation. Hence, it is necessary to further discuss the possible dynamic phenomena and the corresponding parameter region in practical application. We leave it for future work.
This work is supported by the National Natural Science Foundation of China (NSFC, 11631012).
All authors declare no conflicts of interest in this paper.
[1] | R. M. Anderson, R. M. May, O. U. P. (OUP), Infectious diseases of human: dynamics and control, 1992. |
[2] | O. Diekmann, J. Heesterbeek, Mathematical epidemiology of infectious diseases: Model building, analysis and interpretation, Wiley Series in Mathematical and Computational Biology, Chichester, Wiley. |
[3] |
A. S. Perelson, P. W. Nelson, Mathematical Analysis Of HIV-1 Dynamics In Vivo, Siam. Rev., 41 (1999), 3-44. doi: 10.1137/S0036144598335107
![]() |
[4] |
P. Song, Y. Lou, Y. Xiao, A spatial seirs reaction-diffusion model in heterogeneous environment, J. Differ. Equations, 267 (2019), 5084-5114. doi: 10.1016/j.jde.2019.05.022
![]() |
[5] | S. Wain-Hobson, Virus dynamics: Mathematical principles of immunology and virology, Nat. Med., 410 (2001), 412-413. |
[6] | L. J. Abu-Raddad, R. V. Barnabas, H. Janes, H. A. Weiss, J. G. Kublin, I. M. Longini, et al., Have the explosive HIV epidemics in sub-Saharan Africa been driven by higher community viral load?, AIDS, 27 (2013), 2494-2496. |
[7] |
D. Wilson, M. Law, A. E. Grulich, D. A. Cooper, J. M. Kaldor, Relation between HIV viral load and infectiousness: a model-based analysis, The Lancet, 372 (2008), 314-320. doi: 10.1016/S0140-6736(08)61115-0
![]() |
[8] |
T. C. Quinn, M. J. Wawer, N. Sewankambo, D. Serwadda, R. H. Gray, Viral load and heterosexual transmission of human immunodeficiency virus type 1, New. Engl. J. Med., 342 (2000), 921-929. doi: 10.1056/NEJM200003303421303
![]() |
[9] | L. M. Childs, F. E. Moustaid, Z. Gajewski, S. Kadelka, R. Nikinbeers, J. W. Smith, et al., Linked within-host and between-host models and data for infectious diseases: a systematic review, PeerJ, 7 (2019), e7057. |
[10] | N. Dorratoltaj, R. Nikinbeers, S. M. Ciupe, S. Eubank, K. Abbas, Multi-scale immunoepidemiological modeling of within-host and between-host HIV dynamics: systematic review of mathematical models, PeerJ, 5 (2017), e3877. |
[11] |
A. Gandolfi, A. Pugliese, C. Sinisgalli, Epidemic dynamics and host immune response: a nested approach, J. Math. Biol., 70 (2015), 399-435. doi: 10.1007/s00285-014-0769-8
![]() |
[12] | W. Garira, A primer on multiscale modelling of infectious disease systems, Infect. Dis. Model., 3 (2018), 176-191. |
[13] |
W. Garira, A complete categorization of multiscale models of infectious disease systems, J. Biol. Dyn., 11 (2017), 378-435. doi: 10.1080/17513758.2017.1367849
![]() |
[14] | M. A. Gilchrist, D. Coombs, Evolution of virulence: Interdependence, constraints, and selection using nested models, Theor. Popul. Biol., 69 (2006), 145-153. |
[15] |
M. Park, C. Loverdo, S. J. Schreiber, J. O. Lloydsmith, Multiple scales of selection influence the evolutionary emergence of novel pathogens, Philos. T. R. Soc. B., 368 (2013), 20120333. doi: 10.1098/rstb.2012.0333
![]() |
[16] |
M. Shen, Y. Xiao, L. Rong, Global stability of an infection-age structured HIV-1 model linking within-host and between-host dynamics, Math. Biosci., 263 (2015), 37-50. doi: 10.1016/j.mbs.2015.02.003
![]() |
[17] |
M. Shen, Y. Xiao, L. Rong, L. A. Meyers, Conflict and accord of optimal treatment strategies for HIV infection within and between hosts, Math. Biosci., 309 (2019), 107-117. doi: 10.1016/j.mbs.2019.01.007
![]() |
[18] |
M. Shen, Y. Xiao, L. Rong, G. Zhuang, Global dynamics and cost-effectiveness analysis of HIV pre-exposure prophylaxis and structured treatment interruptions based on a multi-scale model, Appl. Math. Model., 75 (2019), 162-200. doi: 10.1016/j.apm.2019.05.024
![]() |
[19] | Z. Feng, X. Cen, Y. Zhao, J. Velasco-Hernandez, Coupled within-host and between-host dynamics and evolution of virulence, Math. Biosci., 270 (2015), 204-212. |
[20] | Z. Feng, J. Velasco-Hernandez, B. Tapia-Santos, A mathematical model for coupling within-host and between-host dynamics in an environmentally-driven infectious disease, Math. Biosci., 241 (2013), 49-55. |
[21] |
X. Wang, S. Tang, A multiscale model on hospital infections coupling macro and micro dynamics, Commun. Nonlinear. Sci., 50 (2017), 256-270. doi: 10.1016/j.cnsns.2017.03.009
![]() |
[22] |
X. Sun, Y. Xiao, Multiscale system for environmentally-driven infectious disease with threshold control strategy, Int. J. Bifurcat. Chaos, 28 (2018), 1850064. doi: 10.1142/S0218127418500645
![]() |
[23] |
Y. Xiao, C. Xiang, R. Cheke, S. Tang, Coupling the macroscale to the microscale in a spatiotemporal context to examine effects of spatial diffusion on disease transmission, B. Math. Biol., 82 (2020), 1-27. doi: 10.1007/s11538-019-00680-3
![]() |
[24] |
S. Bhattacharya, M. Martcheva, An immuno-eco-epidemiological model of competition, J. Biol. Dyn., 10 (2016), 314-341. doi: 10.1080/17513758.2016.1186291
![]() |
[25] |
T. Kostova, Persistence of viral infections on the population level explained by an immunoepidemiological model, Math. Biosci., 206 (2007), 309-319. doi: 10.1016/j.mbs.2005.08.003
![]() |
[26] |
E. C. Manda, F. Chirove, Modelling coupled within host and population dynamics of and HIV infection, J. Math. Biol., 76 (2018), 1123-1158. doi: 10.1007/s00285-017-1170-1
![]() |
[27] |
X. Cen, Z. Feng, Y. Zhao, Emerging disease dynamics in a model coupling within-host and between-host systems, J. Theor. Biol., 361 (2014), 141-151. doi: 10.1016/j.jtbi.2014.07.030
![]() |
[28] |
B. Boldin, O. Diekmann, Superinfections can induce evolutionarily stable coexistence of pathogens, J. Math. Biol., 56 (2008), 635-672. doi: 10.1007/s00285-007-0135-1
![]() |
[29] |
P. Dreessche, J. Watmough, Reproduction numbers and sub-threshold endemic equilibria for compartmental models of disease transmission, Math. Biosci., 180 (2002), 29-48. doi: 10.1016/S0025-5564(02)00108-6
![]() |
1. | Qian Lin, Bin Deng, Jia Rui, Song-Bai Guo, Qingqing Hu, Qiuping Chen, Chi Tang, Lina Zhou, Zeyu Zhao, Shengnan Lin, Yuanzhao Zhu, Meng Yang, Yao Wang, Jingwen Xu, Xingchun Liu, Tianlong Yang, Peihua Li, Zhuoyang Li, Li Luo, Weikang Liu, Chan Liu, Jiefeng Huang, Min Yao, Mengni Nong, Liping Nong, Jinglan Wu, Na Luo, Shihai Chen, Roger Frutos, Shixiong Yang, Qun Li, Jing-An Cui, Tianmu Chen, Epidemiological Characteristics and Transmissibility of Human Immunodeficiency Virus in Nanning City, China, 2001–2020, 2021, 9, 2296-2565, 10.3389/fpubh.2021.689575 | |
2. | Xue-Zhi Li, Shasha Gao, Yi-Ke Fu, Maia Martcheva, Modeling and Research on an Immuno-Epidemiological Coupled System with Coinfection, 2021, 83, 0092-8240, 10.1007/s11538-021-00946-9 | |
3. | James W.G. Doran, Robin N. Thompson, Christian A. Yates, Ruth Bowness, Mathematical methods for scaling from within-host to population-scale in infectious disease systems, 2023, 45, 17554365, 100724, 10.1016/j.epidem.2023.100724 | |
4. | Hui Wu, Yafei Zhao, Xinjian Xu, Jie Lou, Modeling and analysis of a two-strain immuno-epidemiological model with reinfection, 2025, 81, 14681218, 104188, 10.1016/j.nonrwa.2024.104188 | |
5. | Joshua C. Macdonald, Hayriye Gulbudak, Brianna Beechler, Erin E. Gorsich, Simon Gubbins, Eva Pérez-Martin, Anna E. Jolles, Within-Host Viral Growth and Immune Response Rates Predict Foot-and-Mouth Disease Virus Transmission Dynamics for African Buffalo, 2024, 204, 0003-0147, 133, 10.1086/730703 | |
6. | Qigui Yang, Jiabing Huang, A STOCHASTIC MULTI-SCALE COVID-19 MODEL WITH INTERVAL PARAMETERS, 2024, 14, 2156-907X, 515, 10.11948/20230298 | |
7. | Miao Wang, Yaping Wang, Lin Hu, Linfei Nie, Analysis of a Delayed Multiscale AIDS/HIV-1 Model Coupling Between-Host and Within-Host Dynamics, 2024, 13, 2075-1680, 147, 10.3390/axioms13030147 | |
8. | A. Hoyer-Leitzel, S.M. Iams, A.J. Haslam-Hyde, M.L. Zeeman, N.H. Fefferman, An immuno-epidemiological model for transient immune protection: A case study for viral respiratory infections, 2023, 8, 24680427, 855, 10.1016/j.idm.2023.07.004 | |
9. | Raul Isea, Anubha Bajaj, A General Approach to Modeling Covid-19, 2023, 2, 26432811, 1, 10.14302/issn.2643-2811.jmbr-23-4556 |