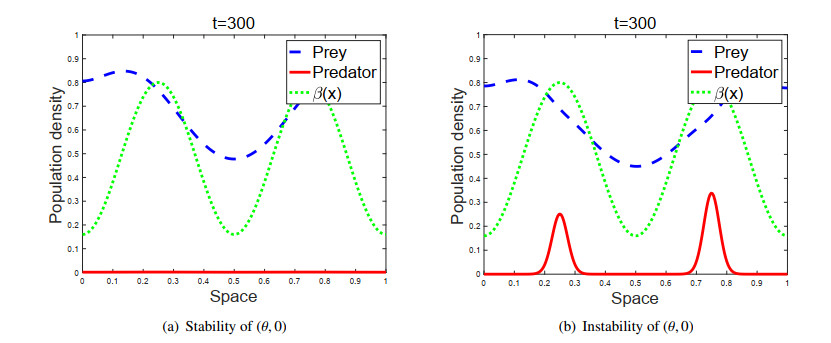
Citation: Kwangjoong Kim, Wonhyung Choi. Local dynamics and coexistence of predator–prey model with directional dispersal of predator[J]. Mathematical Biosciences and Engineering, 2020, 17(6): 6737-6755. doi: 10.3934/mbe.2020351
[1] | Yuxuan Zhang, Xinmiao Rong, Jimin Zhang . A diffusive predator-prey system with prey refuge and predator cannibalism. Mathematical Biosciences and Engineering, 2019, 16(3): 1445-1470. doi: 10.3934/mbe.2019070 |
[2] | Yun Kang, Sourav Kumar Sasmal, Komi Messan . A two-patch prey-predator model with predator dispersal driven by the predation strength. Mathematical Biosciences and Engineering, 2017, 14(4): 843-880. doi: 10.3934/mbe.2017046 |
[3] | Yong Luo . Global existence and stability of the classical solution to a density-dependent prey-predator model with indirect prey-taxis. Mathematical Biosciences and Engineering, 2021, 18(5): 6672-6699. doi: 10.3934/mbe.2021331 |
[4] | Yongli Cai, Malay Banerjee, Yun Kang, Weiming Wang . Spatiotemporal complexity in a predator--prey model with weak Allee effects. Mathematical Biosciences and Engineering, 2014, 11(6): 1247-1274. doi: 10.3934/mbe.2014.11.1247 |
[5] | Eric M. Takyi, Charles Ohanian, Margaret Cathcart, Nihal Kumar . Dynamical analysis of a predator-prey system with prey vigilance and hunting cooperation in predators. Mathematical Biosciences and Engineering, 2024, 21(2): 2768-2786. doi: 10.3934/mbe.2024123 |
[6] | Jin Zhong, Yue Xia, Lijuan Chen, Fengde Chen . Dynamical analysis of a predator-prey system with fear-induced dispersal between patches. Mathematical Biosciences and Engineering, 2025, 22(5): 1159-1184. doi: 10.3934/mbe.2025042 |
[7] | Lazarus Kalvein Beay, Agus Suryanto, Isnani Darti, Trisilowati . Hopf bifurcation and stability analysis of the Rosenzweig-MacArthur predator-prey model with stage-structure in prey. Mathematical Biosciences and Engineering, 2020, 17(4): 4080-4097. doi: 10.3934/mbe.2020226 |
[8] | Yuanfu Shao . Bifurcations of a delayed predator-prey system with fear, refuge for prey and additional food for predator. Mathematical Biosciences and Engineering, 2023, 20(4): 7429-7452. doi: 10.3934/mbe.2023322 |
[9] | Swadesh Pal, Malay Banerjee, Vitaly Volpert . Spatio-temporal Bazykin’s model with space-time nonlocality. Mathematical Biosciences and Engineering, 2020, 17(5): 4801-4824. doi: 10.3934/mbe.2020262 |
[10] | Eric Ruggieri, Sebastian J. Schreiber . The Dynamics of the Schoener-Polis-Holt model of Intra-Guild Predation. Mathematical Biosciences and Engineering, 2005, 2(2): 279-288. doi: 10.3934/mbe.2005.2.279 |
For decades, many researchers have studied and established mathematical models describing interactions among species. Initially, most studies primarily focused on various types of interaction mechanisms and models using random dispersal of species. The importance of species dispersal, which is one of the main factors involved in species behavior, led to the establishment of more realistic models for species dispersal, for example, self-cross diffusion [1,2,3,4,5,6,7,8,9,10], advection-diffusion [11,12,13,14,15,16,17,18,19,20], and other types of non-uniform dispersal [21,22,23,24,25,26,27,28] models. For the predator–prey interaction, a popular way of describing the dispersal mechanism of predators is using prey-taxis [29,30,31,32,33,34]. There are some predator dispersal types such as the cross diffusion [2,3,4,5,6] and starvation-driven diffusion [22,25]. However, most models describing the dispersal mechanism of predators were dependent on prey's population density.
In this paper, we examine the predator–prey model describing a situation wherein the predator dispersal depends on other environmental factors rather than prey density. Here, we consider the following model with a directional dispersal of a predator in a spatially heterogeneous environment:
{ut=μΔu+u(m(x)−u)−α(x)uvu+d(x)vvt=∇⋅(η∇v−χv∇β)+v(−c+β(x)uu+d(x)v)inΩ×(0,∞),∂u∂→n=η∂v∂→n−χv∂β∂→n=0on∂Ω×(0,∞),u(x,0)=u0(x)≥0,v(x,0)=v0(x)≥0inΩ, | (P) |
where Ω is a bounded convex domain in Rn. The zero-flux condition is provided on the smooth boundary ∂Ω, where →n is the outward unit normal vector on the boundary. The functions u(x,t) and v(x,t) represent the population densities of the prey and predator, respectively. m(x) is the growth rate of the prey depending on the location x∈Ω, and μ and η are the diffusion rates of the prey and predator, respectively. α(x) is a positive function representing the capturing rate. β(x) is proportional to α(x), β=kα, where k>0 is a conversion efficiency of food into offspring. A positive function d(x) is a measure of the interference for the predators during hunting the prey. c and χ are positive constants representing the death rate of the predator and taxis sensitivity coefficient, respectively. The reaction term of the predator represents a case wherein the predator is fed only using the prey. To describe the predator–prey interaction, a ratio-dependent functional response is adopted. By considering the spatially heterogeneous environment, we use the predator-dependent form, such as the ratio-dependent form, for the system [35,36,37].
The model (P) represents a situation wherein the predator dispersal is unaffected by the prey density. The evolution of prey's behavior and traits has helped them against the threats of the predators. Consequently, these evolved traits and behaviors, for example, nocturnality [38,39], camouflage and masquerade [40,41], or living underground [42], make it difficult for the predators to chase the prey directly. This implies that the prey density does not affect the predator dispersal anymore. In response to defensive mechanisms of the prey, predators not only memorize the location where the prey was detected before but also learn the locations to hunt the prey in their habitat efficiently. Some studies have investigated the searching mechanism depending on such spatial memory [43,44,45,46]. In [43], the artificial experiments using birds was introduced, which provided some evidence of such animal learning to find better locations (see [43] and references therein). Thus, we assume that the prey has evolved to avoid detection from the predator, and the predator disperses using a spatial memory from experiences about the good locations to forage efficiently.
Such predator dispersal is mathematically described using the taxis term −∇⋅(χv∇β). An attractant function β(x) includes factors such as an encounter with prey and attack success rates. That is, β(x) indicates which area has high or low hunting efficiency for predators. Thus, the taxis term represents that the predator moves toward locations with high foraging efficiency using the spatial memory about β, which is a kind of mnemotaxis [44,45]. The reaction–diffusion system with the taxis term has a property that v is concentrated around the local maximums of β [15,16]. This implies that the predator gathers near the locations that have a high hunting efficiency. Thus, the predators' directional dispersal toward the location with better hunting efficiency can be described using such type of taxis term.
For the described dispersal mechanism of the predators, two situations can occur. The first situation wherein β is proportional to the resources of prey m (β=κm for some constant κ). This means that the predators move toward locations where the prey is abundant because the prey is distributed along with the distribution of resources. Mathematical and experimental works have been performed on a similar situation that the predators track the prey's resources instead of the prey density [47,48,49]. The second situation in which the distributions of β and m are different, i.e., β is not proportional to m. This situation can happen because some places are rich with prey but are easy to hide, making it difficult to hunt for predators, or because the predators memorized correctly, but the environment of prey's habitat has changed due to external factors. This situation seems to be disadvantageous to the predators. Thus, this paper aimed to show the effect of the directional movement of predators in both situations.
In our model, we let w=e−χβ(x)/ηv. Then, an alternative form of (P) is obtained as follows:
{ut=μΔu+u(m(x)−u)−α(x)uwu+d(x)weχβ(x)eχβ(x)/ηwt=ηΔw+χ∇β⋅∇w+w(−c+β(x)uu+d(x)weχβ(x)/η)inΩ×(0,∞),∂u∂→n=∂w∂→n=0on∂Ω×(0,∞),u(x,0)=u0(x)≥0,w(x,0)=w0(x)≥0inΩ, | (P') |
where w(x0)=e−χβ(x)/ηv0. Throughout this paper, we give assumptions on m, α and β:
m(x),α(x),β(x)andd(x)areinC2(ˉΩ),∫Ωm(x)dx>0 | (A) |
Under the assumption (A), the global existence and uniqueness of the classical solution to (P') follows from the Amann's results [50] for quasilinear parabolic equations. And (P') has one semi-trivial solution (θ,0), where θ is a unique positive steady state solution of
{μΔu+u(m(x)−u)=0inΩ,∂u∂→n=0on∂Ω. | (1.1) |
In this paper, we first investigate the local stability of the semi-trivial solution (θ,0), which is closely related to the survival of the predator. We also consider the elliptic system of (P'). We show that the elliptic system has a positive solution, called the coexistence steady-state, under the conditions for the instability of (θ,0). We also investigate the nonexistence of the positive solution.
The remaining part of this paper is organized as follows. In Section 2, we state the main theorems and give a biological explanation. The theorems address the stability of (θ,0) and the existence of a coexistence steady-state. Moreover, the effects of taxis sensitivity χ are observed. We provide the proofs of stability theorem using the principal eigenvalue analysis in Section 3. The existence of a coexistence steady-state is shown by the fixed-point index theory in Section 4. In Section 5, we present the numerical simulations for some cases. Section 6 summarizes the results obtained and concludes the paper.
First, we introduce the results for the stability of (θ,0). The instability of (θ,0) and the positivity of the prey density imply that the predator can invade the region. This implies that the predator can survive in the environment, even though this is rare. This allows us to examine whether the taxis is beneficial to the survival of the predator. As in [29], we can show the positivity of the prey density and lim inft→∞u(x,t)>0 if ∫Ωm(x)dx>∫Ωα(x)dx. Therefore, if we assume ∫Ωm(x)dx>∫Ωα(x)dx, the invasibility of the predator can be determined by the instability of (θ,0).
To study the stability of (θ,0), we consider the following linearized eigenvalue problem of (P') at (θ,0):
{μΔϕ+(m(x)−2θ)ϕ−α(x)eχβ(x)/ηψ=λϕinΩ,ηΔψ+χ∇β⋅∇ψ+(−c+β(x))ψ=λψinΩ,∂ϕ∂→n=∂ψ∂→n=0on∂Ω. | (2.1) |
We multiply eχβ/η by the second equation of Eq (2.1) and obtain
η∇⋅(eχβ/η∇ψ)+(−c+β(x))eχβ/ηψ=λeχβ/ηψ. | (2.2) |
Equation (2.2) is the weighted eigenvalue problem λeχβ/ηψ=Lψ, where Lψ=η∇⋅(eχβ/η∇ψ)+(−c+β(x))eχβ/ηψ. Since L is a self-adjoint, we have the following variational form of the principal eigenvalue:
λ1=supψ∈H1(Ω)∖{0},∫Ωeχβ/ηψ2=1∫Ω−ηeχβ/η|∇ψ|2+(−c+β(x))eχβ/ηψ2. |
We denote the principal eigenvalue of the operator L by λ1(L,χ). It is well-known that λ1(L,χ) and the local stability of (θ,0) have the following relation: If λ1(L,χ)>0, then (θ,0) is locally unstable; if λ1(L,χ)<0, then (θ,0) is locally stable (see [51]).
We define the ecological reproduction number R0 as
R0=supψ∈H1(Ω)∖{0}∫Ωβ(x)eχβ/ηψ2∫Ωηeχβ/η|∇ψ|2+ceχβ/ηψ2>0. | (2.3) |
Thus, we have the following lemma whose proof is analogous to the proof of Lemma 2, 3 in [52].
Lemma 2.1. R0>1 if and only if λ1(L,χ)>0.
Lemma 2.1 implies that (θ,0) is unstable if R0>1, and (θ,0) is locally asymptotically stable if R0<1. Then, R0 has a similar role as the basic reproduction number in epidemiology. This means that the survival of the predator is determined by the sign of R0−1.
Now, we introduce the results for the local stability of (θ,0) with an assumption on β, ∂β∂→n=0. For a function f, we denote the average of f in Ω by ¯f. We first present the stability result about the predator's death rate c when ∂β∂→n=0.
Theorem 2.2. Let η>0,χ≥0 be given. If ∂β∂→n=0, there exists ˜c∈(¯β−χ24η¯|∇β|2,maxˉΩβ) such that (θ,0) is unstable when c<˜c, and (θ,0) is locally asymptotically stable when c>˜c.
In Theorem 2.2, the sharp criteria for the local stability of (θ,0) about c is obtained, which represents invasibility of the predator. Given η>0 and χ≥0, there exists a threshold value ˜c such that λ1(L,χ)=0 for c=˜c and the sign of c−˜c determines the stability of (θ,0). From Theorem 2.2, (θ,0) is unstable for all η>0 when c<¯β and χ=0, but χ>0 gives a possibility (θ,0) to be stable if c∈(¯β−χ24η¯|∇β|,¯β). This means that the directional dispersal could be disadvantageous.
Next, we give the result for the local stability of (θ,0) about the death rate c and diffusion rate η of a predator.
Theorem 2.3. Suppose that ∂β∂→n=0. Let χ>0 and ξ>0 be given constants.
(i) If c>maxˉΩβ, then (θ,0) is locally asymptotically stable for all η>0.
(ii) If c<minˉΩβ, then (θ,0) is unstable for all η>0.
(iii) If c<¯β−14ξ¯|∇β|2, then (θ,0) is unstable when η>ξχ2.
(iv) Suppose that c∈(¯β−14ξ¯|∇β|2,maxˉΩβ). Then ˜η>0 exists such that if ˜η<ξχ2 then (θ,0) is locally asymptotically stable for η∈(˜η,ξχ2); and if ˜η>ξχ2 then (θ,0) is unstable for η∈(ξχ2,˜η).
Theorem 2.3 gives sufficient conditions of the stability of (θ,0) for the predators' diffusion rate η when c is in a proper region. A positively given ξ plays a role for obtaining the sufficient conditions for the stability. For example, we suppose that c and χ such that c<¯β−14ξ1¯|∇β|2 and c∈(¯β−14ξ2¯|∇β|2,maxˉΩβ) for some ξ1>ξ2. Then, we obtain from Theorem 2.3 that (θ,0) is unstable for η>ξ1χ2. Moreover, (θ,0) is unstable for η∈(ξ2χ2,˜η(ξ2)) if ~η(ξ2)>ξ2χ2, and (θ,0) is locally asymptotically stable for η∈(˜η,ξ2χ2) if ˜η<ξ2χ2.
Now, we investigate the effect of taxis χ for fixed c and η.
Theorem 2.4. Suppose that β has at least one isolated global maximum point and maxˉΩβ>c. For given η>0, there exists ˜χ>0 such that if χ≥˜χ then (θ,0) is unstable.
From Theorem 2.4, we can compare the stability of (θ,0) with or without the predator's directional movement. Without the taxis (χ=0), the predator cannot survive when they are rare for (c,η)∈(¯β,maxˉΩβ)×(η∗,∞) for some η∗, which will be immediately shown by lemma in Section 3. However, Theorem 2.4 shows that a large taxis χ>0 makes the predators invade a region. This implies that the strong taxis for predators gives a survival advantage to them compared with a random dispersal.
Next, we give the final result for the stability of (θ,0) when β(x)=κm(x) for x∈Ω. Consider the following equation Aχ2+Bχ+C=0, where
A=−κ24η∫Ω|∇m|2θ2dx,B=κ∫Ωθ∇θ⋅∇mdx,C=∫Ω−η|∇θ|2+(κm−c)θ2dx. | (2.4) |
If B is positive and B2−4AC>0, the equation has two real roots χ1<χ2 such that χ2 is positive.
Theorem 2.5. Suppose that m is positive, β=κm for some constant κ>0 and maxˉΩβ>c. If B2−4AC>0, (θ,0) is unstable when χ∈(max{χ1,0},χ2).
Remark that β(x)=κm(x) implies that the predator moves toward the favorable habitat of the prey. Because the prey has a property to be distributed according to m(x), such movement of the predator allows them to go to an environment where the prey population is sufficient. Moreover, as the assumption β(x)=κm(x) gives two effects, which are taxis toward the location with an abundance of prey and high hunting efficiency, the fitness of the predator increases. Therefore, a proper taxis sensitivity provides a survival advantage to the predator without the assumption on β in Theorem 2.4 for which predators cannot survive without taxis (Theorem 2.5).
Consider the steady-state of the system (P'):
{μΔu+u(m(x)−u)−α(x)uwu+d(x)weχβ(x)/ηeχβ(x)/η=0ηΔw+χ∇β⋅∇w+w(−c+β(x)uu+d(x)weχβ(x)/η)=0inΩ,∂u∂→n=∂w∂→n=0on∂Ω. | (2.5) |
In Eq (2.5), the ratio-dependent functional response is not defined at (u,w)=(0,0). Since
lim(u,w)→(0,0)uweχβ(x)/ηu+d(x)weχβ(x)/η=0, |
we can extend the domain of ud(x)weχβ(x)/ηu+d(x)weχβ(x)/η to {(u,w):u≥0,w≥0}, so that (0,0) becomes a trivial solution of Eq (2.5).
Let a positive solution of Eq (2.5) be a coexistence steady-state. Then, we have the following results for the existence of the coexistence steady-state of Eq (2.5), which is proven using the fixed-point index theory.
Theorem 2.6. Let η>0 and χ≥0 be given. If ∂β∂→n=0, there exists ˜c∈(¯β−χ24η¯|∇β|2,maxˉΩβ) such that Eq (2.5) has at least one coexistence steady state if and only if c<˜c.
Theorem 2.7. Suppose that ∂β∂→n=0. Let χ>0 and ξ>0 be given constants.
(i) If c<¯β−14ξ¯|∇β|2, then Eq (2.5) has at least one coexistence steady state when η>ξχ2.
(ii) If c<minˉΩβ, then Eq (2.5) has at least one coexistence steady state for all η>0.
(iii) Suppose that c∈(¯β−14ξ¯|∇β|2,maxˉΩβ). Then ˜η>0 exists such that if ˜η<ξχ2 then Eq (2.5) has at least one coexistence steady state for η∈(ξχ2,˜η).
Theorem 2.8. Suppose that β has at least one isolated global maximum point and maxˉΩβ>c. For given η>0, there exists ˜χ>0 such that if χ≥˜χ then Eq (2.5) has at least one coexistence steady state.
Theorem 2.9. Suppose that m is positive, β=κm for some constant κ>0 and maxˉΩβ>c. If B2−4AC>0 for given A,B and C as Eq (2.4), then Eq (2.5) has at least one coexistence steady state when χ∈(max{χ1,0},χ2).
It is noteworthy that the conditions for the existence of coexistence steady-state are closely related to the sign of λ1(L,χ), which will result in the proof of the above theorems. From the results in subsection 2.1, Eq (2.5) has more chance to have the coexistence steady-state when taxis sensitivity χ is properly chosen.
In this section, we prove Theorem 2.2–2.5. Recall that the principal eigenvalue of (2.2) is defined by
λ1(L,χ)=supψ∈H1(Ω)∖{0},∫Ωeχβ/ηψ2=1∫Ω−ηeχβ/η|∇ψ|2+(−c+β(x))eχβ/ηψ2. | (3.1) |
We assume that ∂β∂→n=0 and consider φ=eχβ/2ηψ. Since ψ∈H1(Ω) and β∈C2(ˉΩ), φ is also in H1(Ω). In addition, a mapping ψ↦eχβ/2ηψ is bijective. Thus, if we take ψ=e−χβ/2ηφ and take supremum over φ∈H1(Ω), we can rewrite the (3.1) as
λ1(L,χ)=supφ∈H1(Ω)∖{0},||φ||2=1∫Ω−η|∇φ|2+χφ∇φ⋅∇β+(−χ24η|∇β|2+β(x)−c)φ2. | (EV1) |
Then, by Green's identity and the assumption of β, we have
λ1(L,χ)=supφ∈H1(Ω)∖{0},||φ||2=1∫Ω−η|∇φ|2+(−χ2Δβ−χ24η|∇β|2+β(x)−c)φ2. | (EV2) |
It can be easily shown from (EV2) that λ1(L,χ) is the principal eigenvalue of
{ηΔφ+(−χ2Δβ−χ24η|∇β|2+β(x)−c)=λφinΩ,∂φ∂→n=0on∂Ω. |
Let ψ1 be the principal eigenfunction with respect to λ1(L,χ). From (EV2), we obtain
λ1(L,χ)=∫Ω−η|∇φ1|2+(−χ2Δβ−χ24η|∇β|2+(β(x)−c))φ21, |
where φ1=eχβ/2ηψ1. Since the principal eigenfunction is unique, φ1 is the principal eigenfunction with respect to λ1(ηΔ−χ2Δβ−χ24η|∇β|2+(β(x)−c)). Therefore, λ1(L,χ)=λ1(ηΔ−χ2Δβ−χ24η|∇β|2+(β(x)−c)). We will utilize the forms (EV1) and (EV2) in proving our results.
Before proving the theorems, we introduce some properties of the principal eigenvalue. The first property is well-known; the proof is omitted here (See [53]).
Lemma 3.1. Let λ1(ηΔ+g(x)) be the principal eigenvalue of
{ηΔψ+g(x)ψ=λψinΩ,∂ψ∂→n=0on∂Ω. | (3.2) |
Then,
(i) λ1(ηΔ+g(x)) is monotone decreasing function to η;
(ii) λ1(ηΔ+˜g(x))≥λ1(ηΔ+g(x)) for a function ˜g(x)≥g(x);
(iii) limη→0λ1(ηΔ+g(x))=maxx∈ˉΩg(x);
(iv) limη→∞λ1(ηΔ+g(x))=¯g.
Lemma 3.2. Let λ1(ηΔ+g(x)) be the principal eigenvalue of (3.2).
(i) If g(x)<0, then λ1(ηΔ+g(x))<0.
(ii) If ¯g>0, then λ1(ηΔ+g(x))>0.
(iii) If ∫Ωg(x)dx<0 and g(x) is positive somewhere, then there exists ˜η>0 such that λ1(ηΔ+g(x))≥0 if and only if η≤˜η. The equality holds when η=˜η.
Proof. (i) is obvious by the definition of the principal eigenvalue.
(ii) Suppose ∫Ωg(x)dx>0. From the definition of λ1(ηΔ+g(x)),
λ1(ηΔ+g(x))=supψ∈H1(Ω)∖{0},||ψ||2=1∫Ω−η|∇ψ|2+g(x)ψ2dx≥¯g>0. |
(iii) Let S:={x:g(x)>0}≠∅. Then, we can choose a test function ˜ψ with ||˜ψ||2=1 whose support is in S. Then, we obtain
λ1≥∫Ω−η|∇˜ψ|2+g(x)˜ψ2dx=∫S−η|∇˜ψ|2+g(x)˜ψ2dx>0 |
for sufficiently small η. Since λ1(ηΔ+g(x)) is monotone decreasing function to η and limη→∞λ1(ηΔ+g(x))=¯g<0, there exists ˜η such that if η>˜η, then λ1(ηΔ+g(x))<0, and if η<˜η, then λ1(ηΔ+g(x))>0, which is a desired result.
Now, we first give the proof Theorems 2.2 and 2.3.
Proof of Theorem 2.2. From the definition of the principal eigenvalue (3.1), we have
λ1(L,χ)=supψ∈H1(Ω)∖{0},∫Ωeχβ/ηψ2=1∫Ω−ηeχβ/η|∇ψ|2+(−c+β(x))eχβ/ηψ2<0 |
for c>maxˉΩβ.
Next, from (EV2) and ∂β∂→n=0, we obtain
λ1(L,χ)=λ1(ηΔ−χ2Δβ−χ24η|∇β|2+(β(x)−c))=∫Ω−η|∇φ1|2+(−χ2Δβ−χ24η|∇β|2+(β(x)−c))φ21, |
where φ1 is the principal eigenfunction with respect to λ1(ηΔ−χ2Δβ−χ24η|∇β|2+(β(x)−c)). Also, by the Green's first identity, we have
∫ΩΔβ(x)dx=∫∂Ω∂β∂→ndS=0. | (3.3) |
It follows from Eq (3.3) and Lemma 3.2 that
λ1(ηΔ−χ2Δβ−χ24η|∇β|2+(β(x)−c))>0forc<¯β−χ24η¯|∇β|2. |
Also, since Eq (3.1) and (EV2) are equivalent form of the principal eigenvalue,
λ1(ηΔ−χ2Δβ−χ24η|∇β|2+(β(x)−c))<0forc>maxˉΩβ. |
By Lemma 3.1 (ii), λ1(ηΔ−χ2Δβ−χ24η|∇β|2+(β(x)−c)) is monotone decreasing function to c. Then, we can find ˜c∈(¯β−χ24η¯|∇β|2,maxˉΩβ) such that λ1(ηΔ−χ2Δβ−χ24η|∇β|2+(β(x)−c))=0. By the monotonicity of λ1(L,χ) to c, (θ,0) is unstable when c<˜c, and (θ,0) is locally asymptotically stable when c>˜c.
Proof of Theorem 2.3. As in the proof of Theorem 2.2, it suffices to check the sign of λ1(L,χ). From the form Eq (3.1), (i) and (ii) are immediately obtained.
For (iii), we denote λ1(ηΔ−χ2Δβ−χ24η|∇β|2+(β(x)−c)) by ˜λ. Consider an eigenvalue problem as follows:
{ηΔφ+(−χ2Δβ−14ξ|∇β|2+(β(x)−c))φ=λφinΩ,∂φ∂→n=0on∂Ω. | (3.4) |
Then, the principal eigenvalue of Eq (3.4) can be written by
λ1(ηΔ−χ2Δβ−14ξ|∇β|2+(β(x)−c))=supφ∈H1(Ω)∖{0},||φ||2=1∫Ω−η|∇φ|2+(−χ2Δβ−14ξ|∇β|2+(β(x)−c))φ2, |
From Lemma 3.1 (ii),
˜λ>λ1(ηΔ−χ2Δβ−14ξ|∇β|2+(β(x)−c))if and only if η>ξχ2. | (3.5) |
Then, the results (iii) follows from Lemma 3.2.
(iv) Assume that c∈(¯β−14ξ¯|∇β|2,maxˉΩβ). By Lemma 3.2 (iii), there exists ˜η>0 depending on ξ and χ such that λ1(ηΔ−χ2Δβ−14ξ|∇β|2+(β(x)−c))<0 if and only if η>˜η. For ˜η<ξχ2, (˜η,ξχ2) is a non-empty interval. Then, it follows form (3.5) that ˜λ<0 when η∈(˜η,ξχ2), and thus (θ,0) is locally asymptotically stable. Similarly, for ˜η>ξχ2, (θ,0) is unstable when η∈(ξχ2,˜η).
Next, we prove Theorem 2.4.
Proof of Theorem 2.4. It can be proved similarly to Theorem 4.4 in [15]. For reader's convenience, we give the proof here. Since the principal eigenvalue of (2.2) is
λ1(L,χ)=supψ∈H1(Ω)∖{0},∫Ωeχβ/ηψ2=1∫Ω−ηeχβ/η|∇ψ|2+(−c+β(x))eχβ/ηψ2, |
we only need to find a function Ψ with ∫Ωeχβ/ηΨ2=1 such that
∫Ωηeχβ/η|∇Ψ|2<∫Ω(−c+β(x))eχβ/ηΨ2. | (3.6) |
From the assumption, a point x0∈ˉΩ exists satisfying β(x0)=maxˉΩβ and β(x0)−c≥δ for some δ>0. Let R1>0 be small such that β(x)−c≥12δ in BR1(x0)∩Ω, where BR1(x0) is a ball of radius R1 and centered at x0. We define β1=max(BR1(x0)∖BR1/2(x0))∩Ωβ and β2=minBR2(x0)∩Ωβ for R1,R2>0. Since the global maximum point is isolated,
β2≥12[β1+β(x0)]>β1 |
for sufficiently small R2 satisfying R2≤R1/2.
Define a function Φ∈C1(ˉΩ) as
Φ={1in BR1/2(x0)∩Ω,∈[0,1]in (BR1(x0)∖BR2(x0))∩Ω,0otherwise. |
If we put Ψ:=Φ/(∫Ωeχβ/ηΦ2)1/2 in Eq (3.6), the left-hand side becomes
∫Ωηeχβ/η|∇Ψ|2=η∫(BR1(x0)∖BR2(x0))∩Ωeχβ/η|∇Ψ|2≤C1∫(BR1(x0)∖BR2(x0))∩Ωeχβ/η≤C1|Ω|e(χ/η)β1, |
where C1 satisfies ||∇Ψ||∞≤C1. The right-hand side of Eq (3.6) is
∫Ω(−c+β(x))eχβ/ηΨ2=∫BR1(x0)∩Ω(−c+β(x))eχβ/ηΨ2≥e(χ/η)β2∫BR2(x0)∩Ω(−c+β(x))≥C2e(χ/η)β2 |
for some positive constant C2. Since C1 and C2 is independent of χ, Eq (3.6) holds if we choose χ large enough. Hence, we have a desired result.
Finally, we assume that β(x)=κm(x) for x∈Ω, which is the assumption in Theorem 2.5. Before proving the theorem, we give a known result in [14].
Lemma 3.3 (Cantrell et al. [14]). The integral ∫Ωθ∇θ⋅∇mdx is positive if Ω⊆R is an interval or if Ω⊆Rn is convex.
Then, we give the proof of Theorem 2.5.
Proof of Theorem 2.5. Let λ1(L,χ) be the principal eigenvalue with the form (EV1). Since β(x)=κm(x) for x∈Ω,
λ1(L,χ)=supφ∈H1(Ω)∖{0},||φ||2=1∫Ω−η|∇φ|2+κχφ∇φ⋅∇m+(−κ2χ24η|∇m|2+κm−c)φ2≥∫Ω−η|∇θ|2+κχθ∇θ⋅∇m+(−κ2χ24η|∇m|2+κm−c)θ2/∫Ωθ2dx=(Aχ2+Bχ+C)/∫Ωθ2dx, |
where A,B and C are given as Equation (2.4). Since B2−4AC>0, A<0 and B>0 by Lemma 3.3, the equation has two real roots χ1 and χ2 with at least one positive solution, say χ2. Then, Aχ2+Bχ+C>0 for χ1<χ<χ2. which implies (θ,0) is unstable for χ∈(max{χ1,0},χ2). We note that B2−4AC cannot be positive for c>maxˉΩβ, because (θ,0) is locally asymptotically stable (λ1<0) when c>maxˉΩβ. Hence, Theorem 2.5 is valid for c<maxˉΩβ.
In this section, we present the proofs of Theorems 2.6–2.8 which are about the existence and non-existence of the coexistence steady-states of Eq (2.5). Before proving the theorems, we introduce the fixed-point index theory.
Fixed-point index theory
Let E be a real Banach space and W⊂E a closed convex set. W is called a total wedge if αW⊂W for all α≥0 and ¯W∪(−W)=E. A wedge is said to be a cone if W∩(−W)={0}. For y∈W, we define Wy={x∈E:y+γx∈Wfor someγ>0} and Sy={x∈¯Wy:−x∈¯Wy}. Then, ¯Wy is a wedge containing W, y and −y, while Sy is a closed subspace of E containing y.
Let T be a compact linear operator on E satisfying T(¯Wy)⊂¯Wy. We say that T has property α on ¯Wy if there are t∈(0,1) and w∈¯Wy∖Sy such that w−tTw∈Sy. Let F:W→W is a compact operator with a fixed-point y∈W and F is Fréchet differentiable at y. Let L=F′(y) be the Fréchet derivative of F at y. Then, L maps ¯Wy into itself. For an open subset U⊂W, define indexW(F,U)=index(F,U,W)=degW(I−F,U,0), where I is the identity map. If y is an isolated fixed-point of F, then the fixed-point index of F at y in W is defined by indexW(F,y)=index(F,y,W)=index(F,U(y),W), where U(y) is a small open neighborhood of y in W.
The following theorem can be obtained from the results of [54,55,56].
Theorem 4.1. Assume that I−L is invertible on ¯Wy.
(i) If L has property α on ¯Wy, then indexW(F,y)=0.
(ii) If L does not have property α on ¯Wy, then indexW(F,y)=(−1)σ, where σ is the sum of multiplicities of all the eigenvalues of L which are greater than 1.
Lemma 4.2. Any coexistence state (u,w) of system (2.5) has an a priori estimate
u(x)≤Q1,w(x)≤Q2. |
where Q1=maxˉΩm,Q2=Q1maxˉΩβcminˉΩd.
Proof. Let (u,w) be a positive solution of (2.5). Since −μΔu≤u(m(x)−u), we obtain u≤maxˉΩm by maximum principle for elliptic.
Let w(x0)=maxˉΩw and h=χ/η. Then, by maximum principle for elliptic equation,
0≤β(x0)u(x0)u(x0)+d(x0)ehβ(x0)w(x0)−c≤maxˉΩβQ1Q1+minˉΩdw(x0)−c. |
Since
cminˉΩdw(x0)≤cQ1+cminˉΩdw(x0)≤maxˉΩβQ1, |
it implies that
maxˉΩw≤maxˉΩβcminˉΩdQ1. |
Notation 4.3.
(i) X:=C1N(ˉΩ)⨁C1N(ˉΩ) where C1N(ˉΩ):={ϕ∈C1(ˉΩ):∂ϕ∂→n=0on∂Ω}.
(ii) Q:=max{Q1,Q2}+1.
(iii) D:=DQ⨁DQ where DQ={ϕ∈C1N(ˉΩ):ϕ<QonˉΩ}.
(iv) W:=D0⨁D0 where D0={ϕ∈C1N(ˉΩ):0≤ϕonˉΩ}.
(v) D′:=D∩W.
For τ∈[0,1], define a positive compact operator Fτ:X→X by Fτ(u,w)=(−DΔ+P)−1[PI+τG](u,w)T where
G(u,w)T=(mu−u2−αueχβ/ηwu+d(x)eχβ/ηwχ∇β⋅∇w+βueχβ/ηwu+d(x)eχβ/ηw−cw),D=(μ00η). |
and P is a positive constant such that (2Q+maxΩαminΩd+maxΩβminΩd+c)Q+χ‖∇β‖∞‖∇w‖∞<P. Fτ is positive and compact operator. We denote F=F1. Then, (P') has a positive solution if and only if F has a positive fixed-point.
For the operator F, we first find the result for the index values, indexW(F,D′) and indexW(F,(0,0)). The following lemmas can be proved by similar argument to [57]; the proofs are omitted here.
Lemma 4.4. indexW(F,D′)=1.
Lemma 4.5. indexW(F,(0,0))=0.
Next, we calculate the indexW(F,(θ,0)). Remark that the principal eigenvalue λ1(L,χ) of Eq (2.2) is real, and the corresponding principal eigenfunction Ψ is positive. Since the principal eigenpair (λ1(L,χ),Ψ) satisfies Eq (2.2), (λ1(L,χ),Ψ) also satisfies the second equation of Eq (2.1):
λ1(L,χ)Ψ=ηΔΨ+χ∇β⋅∇Ψ+(−c+β(x))ΨinΩ. | (4.1) |
Lemma 4.6. If λ1(L,χ)>0, then indexW(F,(θ,0))=0.
Proof. By calculation, we have
¯W(θ,0)=C1N(¯Ω)⨁D0,S(θ,0)=C1N(¯Ω)⨁{0}. |
Define
L:=F′(θ,0)=(−DΔ+P)−1(m−2θ+P−α0χ∇β⋅∇+β−c+P). |
We first claim that I−L is invertible. Let L(ϕ,ψ)T=(ϕ,ψ)T∈¯W(θ,0).
{−μΔϕ=(m−2θ)ϕ−αψ−ηΔψ=χ∇β⋅∇ψ+(β−c)ψinΩ,∂ϕ∂→n=∂ψ∂→n=0on∂Ω. | (4.2) |
For ψ≥0, multiply the second equation of Eq (4.2) by eχβ/ηΨ and integrate over Ω, where Ψ is a principal eigenfunction of λ1(L,χ). Then, we obtain
0=∫Ωeχβ/ηΨ[ηΔψ+χ∇β⋅∇ψ]+eχβ/η(β−c)Ψψ=∫ΩΨ∇⋅[ηeχβ/η∇ψ]+eχβ/η(β−c)Ψψ=∫Ωψ∇⋅[ηeχβ/η∇Ψ]+eχβ/η(β−c)Ψψ=∫Ωeχβ/ηψ[ηΔΨ+χ∇β⋅∇Ψ+(β−c)Ψ]=λ1(L,χ)∫Ωeχβ/ηΨψ≥0, |
which implies ψ≡0. The last equality holds from Eq (4.1).
Since λ1(μΔ+(m−θ))=0 with the corresponding principal eigenfunction θ, λ1(μΔ+(m−2θ))<0 by Lemma 3.1 (ii). We multiply the first equation of Eq (4.2) by ϕ and integrate over Ω. Then, we have
0=∫Ωαϕψ=∫Ω−μ|∇ϕ|2+(m−2θ)ϕ2≤λ1(μΔ+(m−2θ))∫Ωϕ2≤0. |
Hence, ϕ≡0 and I−L is invertible.
Next, we show that L has a property α. Let r be the spectral radius of(−ηΔ+P)−1(χ∇β⋅∇+β−c+P). Then, the assumption λ1(L,χ)>0 implies that r>1. Then, there exist corresponding eigenfunction ξ exists in D0∖{0}. Take t=1/r∈(0,1), then
(ϕψ)−tL(ϕψ)=(00)∈S(θ,0)holdsfor(ϕψ)=(0ξ)∈¯W(θ,0)∖S(θ,0). |
Hence, L has property α. By Theorem 4.1 (i), we have indexW(F,(θ,0))=0.
Now, we give the proofs of Theorems 2.6–2.9. Theorems 2.7–2.9 can be proved in a similarly manner; we only give the proof of Theorem 2.6.
Proof of Theorem 2.6. Suppose that ∂β∂→n=0. From Theorem 2.2, there exists ˜c∈(¯β−χ24η¯|∇β|2,maxˉΩβ). Then, it follows from Lemma 4.4–4.6 that
1=indexW(F,D′)≠indexW(F,(0,0))+indexW(F,(θ,0))=0. |
Hence, Eq (2.5) has at least one coexistence state for c<˜c.
Next, we assume that c≥˜c. Suppose that (u,w) be the positive solution of Eq (2.5). Then, (u,w) satisfies
ηΔw+χ∇β⋅∇w+w(−c+β(x)uu+d(x)weχβ(x)/η)=0. | (4.3) |
Let ˜w:=w/(∫Ωeχβ/ηw2)1/2. If we multiply Eq (4.3) by eχβ/η˜w/(∫Ωeχβ/ηw2)1/2 and integrate over Ω, we have
0=∫Ω−ηeχβ/η|∇˜w|2+(β(x)uu+d(x)weχβ/η−c)eχβ/η˜w2=∫Ω−ηeχβ/η|∇˜w|2+(β(x)−c)eχβ/η˜w2+(β(x)uu+d(x)weχβ/η−β(x))eχβ/η˜w2≤λ1(L,χ)+∫Ω(β(x)uu+d(x)weχβ/η−β(x))eχβ/η˜w2. | (4.4) |
The last inequality holds by the definition of the principal eigenvalue λ1(L,χ). From Eq (4.4), we obtain
λ1(L,χ)≥∫Ω(β(x)−β(x)uu+d(x)weχβ/η)eχβ/η˜w2>0, |
which is a contradiction because λ1(L,χ)≤0 under the assumption c≥˜c. Hence, there is no positive solution of Eq (2.5) if c≥˜c.
In this section, we present some numerical simulations to verify the mathematical results of this paper. Throughout the simulations, the following parameters and functions are used:
β(x)=0.8α(x),d(x)=0.5+0.1sin(2πx),μ=0.01,u0(x)=0.5+0.2sin(2πx),v0(x)=0.2+0.1sin(2πx),Ω=[0,1]. |
We used the upwind scheme with the finite difference method, and the toleration of iteration is selected as 10−6. The simulation results presented show the important role of directional dispersal with taxis compared to the random dispersal when c,β and η are given.
Figure 1 shows that a large χ gives the predator with directional dispersal a survival advantage when c∈(¯β,maxˉΩβ). Theorem 2.4 implies that the (θ,0) is locally asymptotically stable when χ=0 for any given c and η, but a large χ allows (θ,0) to be unstable. This means that the strong taxis of predator can make them survive under the condition in which the predator with random dispersal cannot survive. Figure 1 shows that such dispersal makes the predator and prey coexist in the environment. It is noteworthy that, as can be seen in Figure 1, the density of the predator is concentrated on the local maximum of β, which is a feature of the species with directional dispersal.
In Figure 2, the case that β(x)=κm(x) is considered. If β(x) has at least one isolated global maximum point, we have a same result in Theorem 2.4 and Figure 1. Thus, we give β(x) as a combination of smoothstep function for Figure 2, which is the case when β has no isolated global maximum point on ¯Ω. Figure 2 shows that the properly chosen taxis sensitivity χ>0 can make the predator survive under the condition when the predator without taxis cannot survive. This represents the result of Theorem 2.5 that the directional dispersal is beneficial for the predator's survival when χ is in a proper region regardless of the assumption on β. This is a natural ecological result because the taxis of the predators not only makes them move towards the region with high hunting efficiency, but also the prey's favorable habitats where the density of the prey is large.
In this paper, we suggested the predator–prey model describing the phenomena that the predator moves with taxis toward the region with a high hunting efficiency. For the predator–prey model, most of the studies dealing with taxis have considered the prey-taxis [29,30,31,32,33,34]. Authors in [29] adopted the ratio-dependent functional responses with a spatially heterogeneous environment, which are the same as our model, and they showed that the prey taxis is always beneficial for the predator's survival. However, the predators cannot chase the prey directly because the model we established describes the situation that prey has evolved to avoid detection from the predator. Thus, we considered other type of predators' dispersal mechanism with taxis toward the location where the hunting efficiency is high using the spatial memory [43,44,45,46]. There are two cases of the rate at which predators capture the prey: proportional (β=κm) or not proportional (β≠κm) to the resources of prey. For the first case, a similar idea is introduced in [47,48,49] that the predators move in the direction of high prey's resources instead of tracking the prey directly, and [47] established the mathematical model of such dispersal and obtained some mathematical results. The other case can be followed from the prey's defensive mechanisms or environmental change from other external factors. This could make the predators go to a region with low prey density, which seems not helpful for the predators. In this paper, we investigated whether the defensive mechanism of the predators is advantageous to them for both cases.
Through this article, we determined the criteria and sufficient conditions for the local stability of the semi-trivial solution, which is closely related to the survival of the predators. Furthermore, the conditions for the existence of coexistence steady-state were obtained. We studied the stability and coexistence conditions for the three parameters: the death rate, diffusion, and taxis sensitivity. Finally, the obtained results were verified by using numerical simulations. From a biological perspective, the results for stability indicate that, when the predators have the spatial memory where the location is good to forage efficiently, the taxis toward the location could give a greater chance of invasion than when they disperse randomly (Theorems 2.2, 2.4 and 2.5). This implies that the directional movement toward the region with high foraging efficiency increases the fitness of predators. Consequently, such dispersal helps the predators to invade a region. If β has at least one isolated global maximum point, the dispersal is advantageous to the predator's survival when taxis is strong even though β is not proportional to m (Theorem 2.4). This represents that the dispersal mechanism also affects to the predators fitness regardless the distributions of β and m. When β has no isolated global maximum, the proper taxis sensitivity gives survival advantage to the predators (Theorem 2.5). This means that the properly chosen taxis sensitivity helps predators survive regardless of assumption on β when the predators have directional movement toward the location with abundant prey and high hunting efficiency (β is proportional to m). Moreover, as a dictum in ecology that "invasibility implies coexistence, " the taxis influences on the coexistence of predator and prey. This dispersal of predators could give them a greater possibility to coexist in the ecosystem than random dispersal (Theorems 2.6, 2.8 and 2.9). Thus, we conclude that the dispersal considered in this work plays a vital role in the survival of the predator and the coexistence in the predator–prey system.
This research was supported by Basic Science Research Program through the National Research Foundation of Korea (NRF) funded by the Ministry of Science, ICT & Future Planning (NRF-2015R1A2A2A01007013; NRF-2018R1C1B5039938).
All authors declare no conflicts of interest in this paper.
[1] | M. Iida, M. Mimura, H. Ninomiya, Diffusion, cross-diffusion and competitive interaction, J. Math. Biol., 53 (2006), 617-641. |
[2] |
W. Ko, K. Ryu, On a predator-prey system with cross diffusion representing the tendency of predators in the presence of prey species, J. Math. Anal. Appl., 341 (2008), 1133-1142. doi: 10.1016/j.jmaa.2007.11.018
![]() |
[3] |
T. Kadota, K. Kuto, Positive steady states for a prey-predator model with some nonlinear diffusion terms, J. Math. Anal. Appl., 323 (2006), 1387-1401. doi: 10.1016/j.jmaa.2005.11.065
![]() |
[4] | K. Kuto, A strongly coupled diffusion effect on the stationary solution set of a prey-predator model, Adv. Differential. Equ., 12 (2007), 145-172. |
[5] | K. Kuto, Y. Yamada, Coexistence problem for a prey-predator model with density-dependent diffusion, Nonlinear Anal.-Theor., 71 (2009), e2223-e2232. |
[6] | K. Kuto, Y. Yamada, Limiting characterization of stationary solutions for a prey-predator model with nonlinear diffusion of fractional type, Differ. Integral. Equ., 22 (2009), 725-752. |
[7] |
Y. Lou, W. Ni, Diffusion vs cross-diffusion: an elliptic approach, J. Differ. Equations, 154 (1999), 157-190. doi: 10.1006/jdeq.1998.3559
![]() |
[8] |
K. Ryu, I. Ahn, Positive steady-states for two interacting species models with linear self-cross diffusions, Discrete Contin. Dyn-A, 9 (2003), 1049. doi: 10.3934/dcds.2003.9.1049
![]() |
[9] |
K. Ryu, I. Ahn, Coexistence theorem of steady states for nonlinear self-cross diffusion systems with competitive dynamics, J. Math. Anal. Appl., 283 (2003), 46-65. doi: 10.1016/S0022-247X(03)00162-8
![]() |
[10] |
N. Shigesada, K. Kawasaki, E. Teramoto, Spatial segregation of interacting species, J. Theor. Biol., 79 (1979), 83-99. doi: 10.1016/0022-5193(79)90258-3
![]() |
[11] | I. Averill, K. Lam, Y. Lou, The role of advection in a two-species competition model: a bifurcation approach, volume 245. American Mathematical Society, 2017. |
[12] |
X. Chen, K. Lam, Y. Lou, Dynamics of a reaction-diffusion-advection model for two competing species, Discrete Contin. Dyn. S., 32 (2012), 3841-3859. doi: 10.3934/dcds.2012.32.3841
![]() |
[13] |
C. Cosner, Reaction-diffusion-advection models for the effects and evolution of dispersal, Discrete Contin. Dyn-A, 34 (2014), 1701-1745. doi: 10.3934/dcds.2014.34.1701
![]() |
[14] |
R. S. Cantrell, C. Cosner, Y. Lou, Movement toward better environments and the evolution of rapid diffusion, Math. Biosci., 204 (2006), 199-214. doi: 10.1016/j.mbs.2006.09.003
![]() |
[15] |
R. S. Cantrell, C. Cosner, Y. Lou, Advection-mediated coexistence of competing species, P. Roy. Soc. Edinb. A., 137 (2007), 497-518. doi: 10.1017/S0308210506000047
![]() |
[16] |
R. S. Cantrell, C. Cosner, Y. Lou, Approximating the ideal free distribution via reaction-diffusion- advection equations, J. Differ. Equations, 245 (2008), 3687-3703. doi: 10.1016/j.jde.2008.07.024
![]() |
[17] |
C. Cosner, Y. Lou, Does movement toward better environments always benefit a population?, J. Math. Anal. Appl., 277 (2003), 489-503. doi: 10.1016/S0022-247X(02)00575-9
![]() |
[18] |
K. Kuto, T. Tsujikawa, Limiting structure of steady-states to the lotka-volterra competition model with large diffusion and advection, J. Differ. Equations, 258 (2015), 1801-1858. doi: 10.1016/j.jde.2014.11.016
![]() |
[19] |
K.-Y. Lam, W.-M. Ni, Advection-mediated competition in general environments, J. Differ. Equations, 257 (2014), 3466-3500. doi: 10.1016/j.jde.2014.06.019
![]() |
[20] |
K.-Y. Lam, W. M. Ni, Limiting profiles of semilinear elliptic equations with large advection in population dynamics, Discrete Contin. Dyn. Syst., 28 (2010), 1051-1067. doi: 10.3934/dcds.2010.28.1051
![]() |
[21] |
E. Cho, Y.-J. Kim, Starvation driven diffusion as a survival strategy of biological organisms, B. Math. Biol., 75 (2013), 845-870. doi: 10.1007/s11538-013-9838-1
![]() |
[22] |
W. Choi, S. Baek, I. Ahn, Intraguild predation with evolutionary dispersal in a spatially heterogeneous environment, J. Math. Biol., 78 (2019), 2141-2169. doi: 10.1007/s00285-019-01336-5
![]() |
[23] |
W. Choi, I. Ahn, Strong competition model with non-uniform dispersal in a heterogeneous environment, Appl. Math. Lett., 88 (2019), 96-102. doi: 10.1016/j.aml.2018.08.014
![]() |
[24] |
W. Choi, I. Ahn, Non-uniform dispersal of logistic population models with free boundaries in a spatially heterogeneous environment, J. Math. Anal. Appl., 479 (2019), 283-314. doi: 10.1016/j.jmaa.2019.06.027
![]() |
[25] |
W. Choi, I. Ahn, Predator-prey interaction systems with non-uniform dispersal in a spatially heterogeneous environment, J. Math. Anal. Appl., 485 (2020), 123860. doi: 10.1016/j.jmaa.2020.123860
![]() |
[26] |
Y.-J. Kim, O. Kwon, F. Li, Evolution of dispersal toward fitness, B. Math. Biol., 75 (2013), 2474- 2498. doi: 10.1007/s11538-013-9904-8
![]() |
[27] |
Y.-J. Kim, O. Kwon, F. Li, Global asymptotic stability and the ideal free distribution in a starvation driven diffusion, J. Math. Biol., 68 (2014), 1341-1370. doi: 10.1007/s00285-013-0674-6
![]() |
[28] |
Y.-J. Kim, O. Kwon, Evolution of dispersal with starvation measure and coexistence, B. Math. Biol., 78 (2016), 254-279. doi: 10.1007/s11538-016-0142-8
![]() |
[29] |
W. Choi, I. Ahn, Effect of prey-taxis on predator's invasion in a spatially heterogeneous environment, Appl. Math. Lett., 98 (2019), 256-262. doi: 10.1016/j.aml.2019.06.021
![]() |
[30] |
S. Wu, J. Shi, B. Wu, Global existence of solutions and uniform persistence of a diffusive predator- prey model with prey-taxis, J. Differ. Equations, 260 (2016), 5847-5874. doi: 10.1016/j.jde.2015.12.024
![]() |
[31] |
H. Jin, Z. Wang, Global stability of prey-taxis systems, J. Differ. Equations, 262 (2017), 1257- 1290. doi: 10.1016/j.jde.2016.10.010
![]() |
[32] |
Y. Tao, Global existence of classical solutions to a predator-prey model with nonlinear prey-taxis, Nonlinear Anal.-Real., 11 (2010), 2056-2064. doi: 10.1016/j.nonrwa.2009.05.005
![]() |
[33] |
X. He, S. Zheng, Global boundedness of solutions in a reaction-diffusion system of predator-prey model with prey-taxis, Appl. Math. Lett., 49 (2015), 73-77. doi: 10.1016/j.aml.2015.04.017
![]() |
[34] |
C. Li, X. Wang, Y. Shao, Steady states of a predator-prey model with prey-taxis, Nonlinear Anal.- Theor., 97 (2014), 155-168. doi: 10.1016/j.na.2013.11.022
![]() |
[35] | P. A. Abrams, L. R. Ginzburg, The nature of predation: prey dependent, ratio dependent or neither?, Trends Ecol. Evol., 15 (2000), 337-341. |
[36] |
R. Arditi, L. R. Ginzburg, Coupling in predator-prey dynamics: ratio-dependence, J. Theor. Biol., 139 (1989), 311-326. doi: 10.1016/S0022-5193(89)80211-5
![]() |
[37] |
C. Cosner, D. L. DeAngelis, J. S. Ault, D. B. Olson, Effects of spatial grouping on the functional response of predators, Theor. Popul. Biol., 56 (1999), 65-75. doi: 10.1006/tpbi.1999.1414
![]() |
[38] |
J. M. Culp, N. E. Glozier, G. J. Scrimgeour, Reduction of predation risk under the cover of darkness: avoidance responses of mayfly larvae to a benthic fish, Oecologia, 86 (1991), 163-169. doi: 10.1007/BF00317527
![]() |
[39] |
F. Mougeot, V. Bretagnolle, Predation risk and moonlight avoidance in nocturnal seabirds, J. Avian. Biol., 31 (2000), 376-386. doi: 10.1034/j.1600-048X.2000.310314.x
![]() |
[40] | T. Caro, Antipredator defenses in birds and mammals, University of Chicago Press, 2005. |
[41] | H. B. Cott, Adaptive coloration in animals, 1940. |
[42] |
J. M. Hemmi, Predator avoidance in fiddler crabs: 1. escape decisions in relation to the risk of predation, Anim. Behav., 69 (2005), 603-614. doi: 10.1016/j.anbehav.2004.06.018
![]() |
[43] | W. J. Bell, Searching behaviour: the behavioural ecology of finding resources, Springer Science & Business Media, 2012. |
[44] |
S. Benhamou, Spatial memory and searching efficiency, Anim. Behav., 47 (1994), 1423-1433. doi: 10.1006/anbe.1994.1189
![]() |
[45] |
S. Benhamou, Bicoordinate navigation based on non-orthogonal gradient fields, J. Theo. Biol., 225 (2003), 235-239. doi: 10.1016/S0022-5193(03)00242-X
![]() |
[46] | W. F. Fagan, M. A. Lewis, M. Auger-Meth ′ e, T. Avgar, S. Benhamou, G. Breed, et al., Spatial ′ memory and animal movement, Ecol. Lett., 16 (2013), 1316-1329. |
[47] |
S. M. Flaxman, Y. Lou, Tracking prey or tracking the prey's resource? mechanisms of movement and optimal habitat selection by predators, J. Theor. Biol., 256 (2009), 187-200. doi: 10.1016/j.jtbi.2008.09.024
![]() |
[48] |
S. M. Flaxman, Y. Lou, F. G. Meyer, Evolutionary ecology of movement by predators and prey, Theor. Ecol., 4 (2011), 255-267. doi: 10.1007/s12080-011-0120-6
![]() |
[49] | A. M. Kittle, M. Anderson, T. Avgar, J. A. Baker, G. S. Brown, J. Hagens, et al., Landscape-level wolf space use is correlated with prey abundance, ease of mobility, and the distribution of prey habitat, Ecosphere, 8 (2017), e01783. |
[50] | H. Amann, Nonhomogeneous linear and quasilinear elliptic and parabolic boundary value problems, In Function spaces, differential operators and nonlinear analysis, pages 9-126. Springer, 1993. |
[51] | R. S. Cantrell, C. Cosner, Spatial ecology via reaction-diffusion equations, John Wiley & Sons, 2004. |
[52] |
L. J. S. Allen, B. M. Bolker, Y. Lou, A. L. Nevai, Asymptotic profiles of the steady states for an sis epidemic reaction-diffusion model, Discrete Contin. Dyn. Syst. Ser. A, 21 (2008), 1. doi: 10.3934/dcds.2008.21.1
![]() |
[53] |
X. He, W.-M. Ni, Global dynamics of the lotka-volterra competition-diffusion system: Diffusion and spatial heterogeneity i, Commun. Pur. Appl. Math., 69 (2016), 981-1014. doi: 10.1002/cpa.21596
![]() |
[54] |
E. N. Dancer, On the indices of fixed points of mappings in cones and applications, J. Math. Anal. Appl., 91 (1983), 131-151. doi: 10.1016/0022-247X(83)90098-7
![]() |
[55] |
L. Li, Coexistence theorems of steady states for predator-prey interacting systems, T. Am. Math. Soc., 305 (1988), 143-166. doi: 10.1090/S0002-9947-1988-0920151-1
![]() |
[56] | M. Wang, Z. Li, Q. Ye, Existence of positive solutions for semilinear elliptic system, In Qualitative aspects and applications of nonlinear evolution equations, 1991. |
[57] |
K. Ryu, I. Ahn, Positive solutions for ratio-dependent predator-prey interaction systems, J. Differ. Equations, 218 (2005), 117-135. doi: 10.1016/j.jde.2005.06.020
![]() |
1. | Rina Su, Chunrui Zhang, The generation mechanism of Turing-pattern in a Tree-grass competition model with cross diffusion and time delay, 2022, 19, 1551-0018, 12073, 10.3934/mbe.2022562 | |
2. | Wonhyung Choi, Kwangjoong Kim, Inkyung Ahn, Predation-induced dispersal toward fitness for predator invasion in predator–prey models, 2023, 17, 1751-3758, 10.1080/17513758.2023.2166133 | |
3. | Toan D. Ha, Vyacheslav G. Tsybulin, Pavel A. Zelenchuk, How to model the local interaction in the predator–prey system at slow diffusion in a heterogeneous environment?, 2022, 52, 1476945X, 101026, 10.1016/j.ecocom.2022.101026 | |
4. | T. D. Ha, V. G. Tsybulin, Multistability for a Mathematical Model of the Dynamics of Predators and Preys in a Heterogeneous Area, 2022, 68, 2413-3639, 509, 10.22363/2413-3639-2022-68-3-509-521 | |
5. | Wonhyung Choi, Kwangjoong Kim, Inkyung Ahn, Predator-prey models with prey-dependent diffusion on predators in spatially heterogeneous habitat, 2023, 525, 0022247X, 127130, 10.1016/j.jmaa.2023.127130 | |
6. | T. D. Ha, V. G. Tsybulin, Multistability for a Mathematical Model of the Dynamics of Predators and Prey in a Heterogeneous Area, 2024, 282, 1072-3374, 417, 10.1007/s10958-024-07185-y | |
7. | P. A. Zelenchuk, V. G. Tsybulin, Mathematical model of ideal free distribution in the predator-prey system, 2023, 69, 2949-0618, 237, 10.22363/2413-3639-2023-69-2-237-249 | |
8. | P. A. Zelenchuk, V. G. Tsybulin, Mathematical Model of Ideal Free Distribution in the Predator–Prey System, 2024, 1072-3374, 10.1007/s10958-024-07445-x |