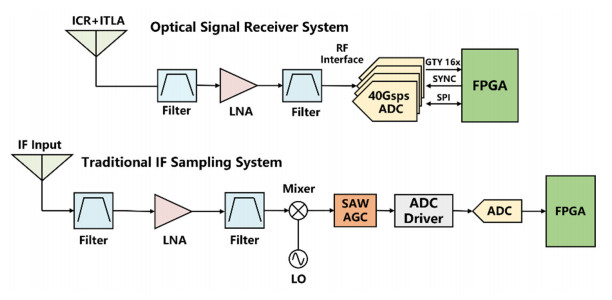
Citation: Youyi Yang, Yongzhen Pei, Xiyin Liang, Yunfei Lv. An optimal scheme to boost immunity and suppress viruses for HIV by combining a phased immunotherapy with the sustaining antiviral therapy[J]. Mathematical Biosciences and Engineering, 2020, 17(5): 4578-4608. doi: 10.3934/mbe.2020253
[1] | Alexej Tschumak, Frank Feldhoff, Frank Klefenz . The switching and learning behavior of an octopus cell implemented on FPGA. Mathematical Biosciences and Engineering, 2024, 21(4): 5762-5781. doi: 10.3934/mbe.2024254 |
[2] | Virginia González-Vélez, Amparo Gil, Iván Quesada . Minimal state models for ionic channels involved in glucagon secretion. Mathematical Biosciences and Engineering, 2010, 7(4): 793-807. doi: 10.3934/mbe.2010.7.793 |
[3] | Weibin Jiang, Xuelin Ye, Ruiqi Chen, Feng Su, Mengru Lin, Yuhanxiao Ma, Yanxiang Zhu, Shizhen Huang . Wearable on-device deep learning system for hand gesture recognition based on FPGA accelerator. Mathematical Biosciences and Engineering, 2021, 18(1): 132-153. doi: 10.3934/mbe.2021007 |
[4] | Mingwei Wang, Kaisheng Shi, Zhao Wang, Rui Yan, Tao Lei, Jiaqin Xiao . Multi-slot energy harvesting wireless communication in interference environment. Mathematical Biosciences and Engineering, 2021, 18(4): 4127-4145. doi: 10.3934/mbe.2021207 |
[5] | Wei Zhong, Li Mao, Wei Du . A signal quality assessment method for fetal QRS complexes detection. Mathematical Biosciences and Engineering, 2023, 20(5): 7943-7956. doi: 10.3934/mbe.2023344 |
[6] | Gianni Gilioli, Sara Pasquali, Fabrizio Ruggeri . Nonlinear functional response parameter estimation in a stochastic predator-prey model. Mathematical Biosciences and Engineering, 2012, 9(1): 75-96. doi: 10.3934/mbe.2012.9.75 |
[7] | János Végh, Ádám József Berki . Revisiting neural information, computing and linking capacity. Mathematical Biosciences and Engineering, 2023, 20(7): 12380-12403. doi: 10.3934/mbe.2023551 |
[8] | Bestami TAŞAR, Fatih ÜNEŞ, Ercan GEMİCİ . Laboratory and numerical investigation of the 2-array submerged vanes in meandering open channel. Mathematical Biosciences and Engineering, 2023, 20(2): 3261-3281. doi: 10.3934/mbe.2023153 |
[9] | Arni S. R. Srinivasa Rao, Kurien Thomas, Kurapati Sudhakar, Philip K. Maini . HIV/AIDS epidemic in India and predicting the impact of the national response: Mathematical modeling and analysis. Mathematical Biosciences and Engineering, 2009, 6(4): 779-813. doi: 10.3934/mbe.2009.6.779 |
[10] | Suqi Xue, Farong Gao, Xudong Wu, Qun Xu, Xuecheng Weng, Qizhong Zhang . MUNIX repeatability evaluation method based on FastICA demixing. Mathematical Biosciences and Engineering, 2023, 20(9): 16362-16382. doi: 10.3934/mbe.2023730 |
With the popularization of Internet, various new media promote the explosive development of the network industry. The transmission bandwidth requirements of metropolitan area networks and backbone networks continue to increase. Reference [1] proposes a method which includes two pilot scheduling schemes, fractional pilot reuse (FPR) and asynchronous fractional pilot scheduling (AFPS) scheme, which significantly mitigate the personal computer (PC) in the uplink time division duplex (TDD) massive multiple-input-multiple-output (MIMO) system. Reference [2] describes a machine learning and deep learning concepts in optical 5G network, they collect the data first, and operate the optimal weighted feature extraction (OWFE), then they finish the slicing classification. Their model can influence the provision of accurate 5G network slicing. In 5G communication and MIMO system, there are still many challenges to the deployment of transmission systems. In order to transfer the photoelectric conversion analog signals into the digital domain, ADC plays an important role.
The traditional intermediate frequency (IF) signal acquisition platform is shown in Figure 1. With the help of a software radio structure, the microwave signal received by the antenna is mixed, converted into an IF signal, and then it is sampled after the signal conditioning links such as amplification and filtering; however, for the 20 Gbps radio frequency signal, the IF method cannot directly sample the radio frequency signal, so the radio frequency (RF) signal sampling structure is created, which is shown in Figure 1. First, it receives the radio frequency signal, and passes through a filter to reduce noise. After filtering through a low noise amplifier (LNA), it enters the ADC to achieve analog-to-digital conversion. Compared with the traditional sampling method, the structure is simpler and it is more suitable for high-bandwidth signals.
In order to meet the requirements of RF signal sampling frequency, the sampling method of multi-channel time interleaving is proposed and widely used in ultra-wideband communication and high-speed serial communication. The structure and clock phase are shown in Figure 2, M channels sample alternately, and the sampling frequency becomes M times that of a single channel. Although the time interleaved technology increases the sampling frequency on the limited hardware resources, due to process issues and device aging, stress imbalance and temperature drift, etc., the gain, offset and delay of each channel will be randomly distributed (gain and offset are the values by which the input values are multiplied and then to which the input values are added, respectively), and such errors will be amplified several times under the influence of high speed, which seriously affect the accuracy of ADC, so ADC calibration becomes a key step in the process of digital signal.
In recent years, scholars have mostly used equalization technology, adaptive blind correction, and random chopping sampling technology for calibrating the channel mismatch. Some works propose inter-channel equalization technology to solve the gain mismatch [3,4,5,6], they divide the convergence process into several phases and assist with a monotonicity detector to decide when to shift from one phase to the next phase. Selecting one of the channels as the reference channel will cause the channel to fail to perform self-calibration, and the calibration model lacks completeness, secondly, the addition of the reference channel makes the input impedance change, which is an obvious shortcoming for ultra-high-speed ADCs. The method proposed in [7] is to estimate the mismatch between channels, the technique is based on the discrete Fourier transform (DFT) for estimating and correcting gain mismatch and timing error in an M-ADC, which also describes the influence of these mismatches, however, the research does not propose a method to compensate the offset error, the technique is lack of integrity. In [8], an fast Fourier transform (FFT)-based method to evaluate and compensate offset and gain errors in time-interleaved ADC system is proposed with a known sinusoidal input. In [9], a digital background calibration method is proposed to calibrate the offset and gain mismatch as well as the timing error.
Reference [10] proposes a digital hybrid background calibration for time delay, with a 5 GS/s 29 mW TI successive-approximation-register (SAR) ADC, and the calibrated signal noise distortion rate (SNDR) reaches 48.5 dB (which is also called signal-to-noise-and-distortion ratio (SINAD) officially in the IEEE Standard, in order not to confuse the two definitions (SNDR and SINAD) later in the text, the subsequent exposition is consistent with the standard and is referred to as SINAD). They use a model of parameter mixed between channels, and estimate the time delay based on Nyquist frequency, the method is divided into 4 steps to achieve channel alignment. Finally, the calibration is completed in the order of offset and gain. However, the reason for the offset and gain mismatch is attributed to the delay in the article, after estimation and compensation, the offset and gain mismatch are resolved. For ultra-high-speed optical signal acquisition systems, the sources of mismatch are more complicated, and the effects of offset and gain need to be considered separately on the basis of completing channel alignment. Reference [11] divides the TI structure into two topological structures, which improves the convergence speed of time offset calibration, and SINAD can reach 54.2 dB; Reference [12] mainly introduces the calibration of bandwidth mismatch, based on the traditional method, constructs a kind of FIR filter to implement signal compensation. References [9] and [13] describe an algorithm for evaluating ADC performance, and comprehensively analyzes the impact of bandwidth mismatch on sampling. References [14,15] are different from the traditional background calibration, the calibration method of the digital-to-analog converter (DAC) in the feedback link is introduced, which further improves the calibration effect. References [16,17,18] analyze the influence of channel mismatch sampling, they use the channel cross feedback mechanism to equalize the mismatch, and design a peripheral delay circuit to compensate for the offset of the calibration clock, which eliminate the energy of harmonics, and improve SINAD; References [19,20,21,22] propose a background calibration method that does not require pre-emphasis based on a 65 nm, 6-bit, 16 GS/s TI ADC. The offset mismatch is reduced after digital calibration, the delay phase locked loop is used to generate 8 sampling interfaces as multi-phase clock generator; References [23,24,25] propose a blind method to estimate channel mismatch and timing skew, this method does not need to know the input signal, as long as it meets the required bandwidth, calibration can be done while ADC is converting, it can be applied to many environments. The above works are all carried out around the background calibration. Compared with the foreground calibration, the background calibration can better monitor the power and temperature of the system, besides, it will not affect the normal sampling of the ADC. 1241–2010 IEEE Standard in [26] provides the standard for terminology and test methods for analog-to-digital converters, which also includes the method to calculate the gain and offset mismatch.
Different from the previous research works, this article does not select an actual channel as the reference channel, which means all channels can be calibrated and there is no impedance mismatch. When every channel meets the standard (offset and gain error are 0), the calibration is completed, otherwise, calibrate it until convergence. We estimate the mismatch first and design a channel equalization structure to calibrate, such method can reduce the error and the signal quality is further improved. Besides, there are a variety of random noise in the complex transmission channel, traditional methods are not stable especially at a high sampling frequency, however, Monte Carlo method happens to be flexible to deal with these random issues, so the relative error of estimation is smaller and reduces the number of iterations. After calibration, the SFDR reaches 41.72 dB and the SINAD increases from 21.65 dB to 30.16 dB, the signal-to-noise ratio (SNR) improves from 21.91 dB to 30.58 dB and ENOB achieves 5.76 bits, such method shows a better performance than other methods in the same experiment condition.
The rest of this article is organized as follows: Section 2 describes the impact of mismatch on ADC. Section 3 reviews the accumulative average algorithm used in the channel equalization process. Section 4 describes the improvement of the channel equalization algorithm. Section 5 is the hardware implementation and experiment results compared with other art works. Section 6 draws the conclusion.
There are two versions to define the gain and offset: 1) (independently based) gain and offset are the values by which the input values are multiplied and then to which the input values are added, respectively, to minimize the mean squared deviation from the output values. 2) (terminal based) gain and offset are the values by which the input values are multiplied and then to which the input values are added, respectively, to cause the deviations from the output values to be zero at the terminal points, that is, at the first and last codes. In order to verify the derivation, we simulate the mismatch by a 4-channel 8-bit ADC with the sampling frequency at 40 GS/s, there are two types of input signals: the input frequency fin is 1.2 GHz and 12 GHz, both voltage of the input signal is 1 V.
Because the processing is performed in the digital domain, the processing process is based on the binary output, the input of the circuit is a standard sinusoidal signal, therefore, in the complete cycle, the average value of the output code is 0, compared the actual average value with 0 can get the offset mismatch. The same input voltage, output different codes, subtracting a calibrated offset mismatch from this code is the output result after calibration. The conversion structure is shown in Figure 3(a), each channel has an offset mismatch Oi [27], the mismatch cause the transfer curve to deviate from the ideal curve, as shown in Figure 3(b). At the same time, the temperature drift and stress of the chip will also affect it. A certain range of fluctuations are generated on the input analog voltage. Such fluctuations are randomly distributed among the sub-ADCs (SADCs), and there is a positive or negative shift.
Each channel has a different offset mismatch O1,O2,…,OM, since each channel works alternately, the offset mismatch can be seen as periodic noise, which can be expressed as:
{…,O1,O2,…,OM,O1,O2,…,OM,O1,O2,…} | (1) |
in the time domain, the noise sequence can be expressed as:
y(t)=M∑i=1Oi∞∑n=−∞δ(t−kTs−nMTs) | (2) |
where TS is the sampling period, in order to identify the influence of the harmonics caused by the offset mismatch, Fourier transform of Eq (1) can be represented as:
˜On=1MM−1∑i=−∞Oie−j(2πM)ni | (3) |
bring Eq (3) into the output signal:
Y(jω)=2πTS∞∑n=−∞˜Onδ(ω−2πnMTS) | (4) |
it can be seen from Eq (4) that the offset mismatch will produce harmonic components at i×fS/M, i=0,1,2,…,M−1, fS is the sampling frequency, since the DFT has symmetry, this article takes the spectrum of one side, and the harmonics of the other half are the same. When the ADC only has an offset mismatch, for example, simulate the offset in the 4-channel ADC, we set the root mean square (RMS) and the range of offset to 0.29 V and ±0.5 V respectively, Figure 4(a), (b) show the comparison between ideal and mismatch sampling sequence, the offset mismatch of each channel is different, and they all deviate from the ideal sampling point. As shown in Figure 4(c), (d), the spectrum contained M-1 harmonic components.
The gain mismatch is generally caused by the mismatch of the ADC reference voltage, capacitance or device, the amplitude of the output signal is inconsistent with the ideal case, it is just a ratio without unit. The ADC used in this article integrates 128 SADCs, each 8 SADCs are combined to form 16 channels. Due to the different positions of the SADCs on the chip, uneven temperature and unbalanced forces, each ADC has a randomly distributed gain mismatch. In an ideal case, the gain is 1, but due to the above reasons, the gain of each channel is randomly distributed within a certain range above or below 1. The mismatch model is shown in Figure 5(a), and Figure 5(b) shows a comparison between ideal transfer curve and actual curve with gain mismatch.
Each sub-channel ADC has a different gain (G1,G2,…,GM). As shown in Figure 5(a), due to the periodic alternating work of each channel ADC, the gain mismatch can be seen as the sequence noise, which can be expressed as:
{…,G1,G2,…,GM,G1,G2,…,GM,G1,G2,…} | (5) |
in time domain, Eq (5) is represented as:
a(t)=M−1∑i=0Gi∞∑n=−∞δ(t−kTS−nMTS) | (6) |
in order to facilitate analysis, Eq (6) is transformed into frequency domain after Fourier transform:
An=1MM−1∑i=0Gie−j(2πM)ni | (7) |
assume there is only a gain mismatch in the time-interleaved ADC system, the output sequence can be expressed as:
Y(jω)=1TS∞∑n=−∞AnX[j(ω−2πnMTS] | (8) |
gain mismatch will cause the nonlinearity of the time-interleaved ADC output, which appears as periodic noise in the time domain, and as high-energy harmonics at a fixed frequency in the frequency domain. The frequency position where the peak of the harmonic spectrum appears for:
fdis=±fin+iMfS,i=1,2,…,M−1 | (9) |
In the simulation with gain mismatch only, we set the RMS to 1.01, and the range of the gain mismatch is ±1.30, the sampling sequence is shown in Figure 6(a), (b). The inconsistency of the gains between the 4 channels causes the sampling point to deviate from the ideal sampling point. The mismatched spectrum is shown in Figure 6(c), (d). Large harmonic components are produced at the frequency ±fin+i×fs/M, which have an impact on the signal, different input frequencies lead to different harmonic positions within a limited spectrum.
The jitter of the clock causes delay in the sampling time of different channels, the clock diagram with time delay is shown in Figure 7(a), which make the actual sampling point deviate from the ideal sampling point shown in Figure 7(b).
The clock phase generated by the ideal clock is ϕi and the jitter is Δti, the sampling points are different from ideal case, the sequence can be expressed as:
y(t)=M−1∑i=0yi(t)=M−1∑i=0+∞∑k=−∞x(t−Δti)δ(t−(nM+i)TS),i=0,1,…,M−1 | (10) |
where yi(t) is the sampling sequence of channel i, x(t) is the input signal, and δ(⋅) is the sampling pulse sequence, δ(⋅) can be represented in frequency domain as:
+∞∑k=−∞δ(j(Ω−2πkMTS))e−jΩiTS | (11) |
As a result, y(t) can be represented in frequency domain as:
Y(jΩ)=1TS+∞∑k=−∞X(j(Ω−k2πMTS))M−1∑i=01Me−jki2πMe−jΩΔti=1TS+∞∑k=−∞α[k]X(j(Ω−k2πMTS)) | (12) |
where α[k] can be represented as:
α[k]=M−1∑i=01Me−jΩΔtie−jki2πM | (13) |
As we can know from Eq (13), the jitter of sampling clock can cause several harmonic components at ±fin±ifS/M(i=1,2,M−1) in the spectrum, in the simulation, we set the RMS to 14.53 ps, and the clock jitter range is ±1/fS, as shown in Figure 8(a), (b), the sampling points are different from the ideal case, the position of harmonic components are consistent with the above derivation, which are shown in Figure 8(c), (d).
The energy of the effective signal in the output spectrum can be represented as:
PS=2|α[0]|2=2|1MM−1∑i=0ejΩ0Δti|2 | (14) |
The noise power caused by clock jitter can be represented as:
PN=2M−1∑i=1|α[k]|2=2M−1∑i=0|α[k]|2−2|α[0]|2 | (15) |
According to the Parseval theory,
2M−1∑i=1|α[k]|2=21MM−1∑i=0|e−jΩ0Δti|2=2 | (16) |
As a result, the SNR can be represented as:
SNR=10log10(PSPN)=10log10(β21+β221−β21−β22) | (17) |
where β1=1M∑M−1i=0cos(Ω0Δti),β2=1M∑M−1i=0sin(Ω0Δti), Eq (17) shows that noise and input frequency can influence the SNR seriously.
According to the channel equalization method, we construct the offset mismatch calibration diagram shown in Figure 9. The model ACC & AVG indicates the accumulative average method. The specific process is:
● According to the accumulative average method, calculate the initial offset mismatch Oi(i=0,1,…,M−1) according to Eq (18), and obtain Do by taking the difference between Oi and Oref,
Oi=NM⋅(si+N/M∑n=1snM−i)−s0,i=0,1,…,M−1 | (18) |
D0=Oi−Oref,Oref=0V | (19) |
● where s is the actual sampling sequence, M is the number of channels, N is the total number of sampling points, and s0 is the direct current (DC) offset of the signal under ideal case, Oref=0.
● According to Eq (20), the offset correction amount Ocal,t is initialized, the result is stored in the register, and Ocal,t is updated according to Eq (21).
Ocal,1=μg×Do | (20) |
Ocal,t=μo×Do+Ocal,t−1 | (21) |
where μo is the calibration step size, which determines the number of iterations and calibration accuracy of the calibration algorithm.
● Calibrate the output data according to Ocal,t to get the updated sequence yout,i.
yout,i=sout,i−Ocal,t−1 | (22) |
Update the calibrated data to Do once in step 2 and repeat the above algorithm, until Oi approaches Oref and Ocal approaches a fixed value.
Similar to the calibration of offset mismatch, the gain calibration is shown in Figure 10. The specific derivation process is presented as follows:
● According to the accumulative average, the gain mismatch Gi is initialized according to Eq (23), and the difference between Gi and Gref is expressed by Dg:
Gi=M∑NMn=1(s2nM−i+s2i)∑Mi=0∑N/Mn=1(s2nM−i+s2i) | (23) |
Dg=Gi−Gref,Gref=1 | (24) |
● Initialize the gain correction amount Gcal according to Eq (25), and update Gcal according to Eq (26):
Gcal=μgDg | (25) |
Gcal,t=μgDg+Gcal,t−1 | (26) |
where μg is the calibration step size, which determines the number of iterations and calibration accuracy of the calibration algorithm.
● Use Gcal to calibrate the signal to get the updated sequence yout,i:
yout,t=sout,i×(Gcal,t−1) | (27) |
● Update the calibrated data to Dg once in step 2 and repeat the above algorithm. Gi approaches Gref, Gcal approaches a fixed value, and the calibration is completed.
Monte Carlo method, also known as statistical simulation method, is a general term for ideas or methods, rather than algorithms in the strict sense [28,29]. The advantage of this method is that it can better deal with the randomness problem in the communication system: the mismatch of each channel is stable and unchanging, and the constant characteristics can be used to iterate; but the quantized noise is different in each measurement, and noise varies non-linearly with time, which is in line with the characteristics of probability and statistics. Follow the steps below to build a Monte Carlo model:
● Construct or describe the random process: The random noise generated by the mismatch allows the probabilistic process to be established, although each ADC has its own different true value of mismatch, but the result of each estimated mismatch is random.
● Sample from a known probability distribution: Because the estimated mismatch noise is different each time, the output result of ADCi is different, but the sequence noise is periodically randomly distributed and has regularity.
● Obtain estimation results.
The estimation diagram is shown in Figure 11. The input signal x(t) is sampled by ADC to obtain y[n], and y[n] is subjected to least square fitting. The gain mismatch gi, offset mismatch osi and time delay Δti are included in the parameters ˆA,ˆB,ˆC; the ideal sequence is interpolated by finite impulse response (FIR), after the mismatch model, the unknown mismatch Δti,gi,osi are added and the actual output sequence is equal, and the equation is constructed and solved using the equal relationship between them.
The specific solution process is as follows: the ideal input signal can be expressed as:
x(t)=acos(2πfint+ϕ)+C | (28) |
where α is the signal amplitude, fin is the signal frequency, ϕ is the phase, and C is the DC bias. The actual sampling sequence can be expressed as:
y[n]=Q[αcos(ωn+ϕ)+C+e[n]],n=0,…,N−1 | (29) |
where Q is the quantization parameter, Q=VFS2n, ω=2πfinfs, e(n) is the channel noise, and N is the number of sampling points. The actual output of ADCi is:
Yi=[y(i),y(i+1∗M),…,y(i+N128∗M)] | (30) |
There are many ways to estimate parameters. This article uses least square (LS) to complete fitting. By calculating the minimum value of Eq (31), the best estimation result is:
(E)2=N−1∑n=0(y[n]−ˆy[n])2 | (31) |
where y[n] is the actual sampling sequence, ˆy[n]=ˆαcos(ωn+ˆϕ)+ˆC=ˆAcos(ωn)+ˆBsin(ωn)+ˆC,ˆA=ˆαcos(ωn),ˆB=ˆαsin(ωn). The actual output after fitting is:
yi[n]=ˆAicos(ωin)+ˆBisin(ωin)+ˆCi,n=i,i+m,…,i+NM128 | (32) |
For the case of no mismatch between ADCs, the output ofADCi can actually be understood as the output of ADC0 obtained through n-fold interpolation [30,31]. Therefore, when performing LS fitting on an ideal channel, an ideal sequence can be obtained through an interpolation filter. This article uses Chebyshev I-type interpolation filtering to simulate two-channel ADC interpolation. First, ADC1 is twice interpolated, and the upper limit of passband lost is set Rp=0.1, the lower limit of stopband loss is Rs=1, and the normalized band angle frequency Wp=0.4, the stopband corner frequency Ws=0.6, after calculated through the above parameters, the filter order n=2 and the cutoff frequency fp=0.4, besides, as shown in Figure 12, the interpolated filter is compared to the initial sequence, the filter contains more points and the error would be reduced.
Fit the output data without mismatch according to the result of interpolation, and the fitted sequence of ideal ADCi can be represented as:
¯yi[n]=¯Aicos(ωin)+¯Bisin(ωin)+¯Ci,n=i,i+m,…,i+NM128 | (33) |
where ¯Ai=¯αicos(¯ϕi),¯Bi=¯αisin(¯ϕi).
In order to construct a set of equations, add mismatches to the ideal sequence according to Figure 13, add the delay Δti between ADCs, the offset mismatch osi and the gain mismatch gi one by one [11]. Due to the presence of noise and mismatch in the channel, the actual output sequence can be expressed as:
yi[n]=gi⋅x(nMTs+iTs+Δti)+osi,i=0,1,2,…,M−1 | (34) |
substituting the x(t) expression into Eq (34), yi(n) can be expressed as:
yi[n]=Pcos(ω0n)−Qsin(ω0n)+¯Ci+osiP(⋅)=[¯Aigicos(2πfinΔti)+¯Bigisin(2πfinΔti)]Q(⋅)=[¯Aigisin(2πfinΔti)−¯Bigicos(2πfinΔti)] | (35) |
Equations (35) and (32) both represent the actual output of ADCi, so the relationship between the ideal sine and the fitted actual sequence is:
[ˆAiˆBiˆCi]T=[¯Ai¯Bi¯Ci]T⋅¯E | (36) |
where ˆAiˆBiˆCi and ¯Ai¯Bi¯Ci are all known, matrix ¯E can be represented as:
[gicos(2πfinΔti)−gisin(2πfinΔti)0gisin(2πfinΔti)gicos(2πfinΔti)0001+osi/¯Ci] | (37) |
Solve the matrix equation and get the expressions of gi, Δti and osi:
{Δti=tan(ˆAi¯Bi−¯AiˆBiˆAi¯Ai−¯BiˆBi)/2πfingi=ˆAi¯Aicos(2πfinΔti)+¯Bisin(2πfinΔti)osi=ˆCi−¯Ci | (38) |
Pack the above algorithm into the model named Cali, replace the ACC & AVG module in the algorithm presented in Section 3, the detailed process is shown in Figure 14:
● The input signal enters M SADCs through time interleaving sampling, and the sampled sequence enters the Cali.
● The offset Oi of channel i is obtained by least square fitting and Monte Carlo estimation.
● The initial value of the offset calibration amount Ocal,t=μoDo.
● Compensate the sampling sequence according to Ocal,t, yout,i=sout,i−Ocal,t.
● Perform fitting and mismatch estimation on the compensated sequence, then update the mismatch Oi through the estimated result ESTi=[gi,osi,Δti], the ESTi is calculated by Eq (38).
● Update the offset mismatch calibration amount Ocal,t=μoDo+Ocal,t−1.
● Compensate the sampling sequence again, yout,i=sout,i−Ocal,t;
● Repeat the above steps until Oi,Do approach 0, Ocal,t approach a constant value.
The above-mentioned calibration steps are calibrated ADCi to ADCM.
Similar to calibrate the offset mismatch, calibrate the gain mismatch according to the diagram shown in Figure 15:
● The analog signal is input to M SADCs for interleaving sampling, and the sampling sequence output by ADCi enters the model Cali.
● Fit the sequence and estimate the initial value Gi of the gain mismatch.
● The initial value of the gain calibration amount Gcal,t=μgDg;
● Compensate the sampling sequence according to Gcal,t, yout,i=sout,i×(Gcal,t−1);
● Perform fitting and mismatch estimation on the compensated sequence, and update the gain mismatch Gi through the estimation result ESTi=[gi,osi,Δti];
● Update gain mismatch calibration amount Gcal,t=μgDg+Gcal,t−1;
● Compensate the sampling sequence again, yout,i=sout,i×(Gcal,t−1).
Repeat the above steps until Gi,Dg approach 0, and Gcal,t approach a constant value. The above-mentioned calibration procedure is calibrated according to from ADCi to ADCM.
Compared with the inter-channel equalization algorithm proposed in Section 3, the reference channel set in this article is not a channel in the actual ADC, but an ideal ADC with offset mismatch Oref=0V and gain mismatch Gref=1. In the calibrated process, all M channels are compensated, and the final sequence is close to the ideal value. The new reference channel does not occupy more logic resources and consume more power consumption; in addition, the initial mismatch will also be compensated in an iterative manner. Therefore, the accuracy requirements of this article are not as strict as those mentioned in [7], and the accuracy of calibration mainly depends on the calibration factor μ. This method reduces the number of estimates and the amount of calculation.
The layout design and power consumption of the ADC are shown in Figure 16(a), and the size is 21 mm×21 mm. The ADC is calibrated using the method in Section 4. The signal supply source is shown in Figure 16(b). The signal generator from Rohde Schwarz is used to input a 1.2 GHz and 5 dBm sinusoidal signal and enter the experimental platform through the RF interface. The actual platform is shown in Figure 16(c): The daughter card in the lower right corner is equipped with a 16-channel 8-bit ADC with a sampling frequency of 40 GS/s, and the reference voltage Vref is 1.2 V, which is connected to the motherboard through the FPGA mezzanine card (FMC) interface, and the FMC connector establishes a connection with the FPGA through the gigabit transmitter in Y-version (GTY) high-speed port. The test environment and related indicators are shown in Tables 1 and 2:
Hardware Configuration | Amount | Remark |
Sampling Clock | 1 | fS>15 GHz |
Input Signal | 1 | fin>1 GHz |
High Frequency Cables | 2 | / |
Balun | 1 | / |
ADC Test Evaluation Board | 1 | 40 GS/s 8-bit |
FPGA | 1 | Xilinx Virtex |
Computer | 1 | / |
Parameter | Index |
Resolution | 8-bit |
Sampling Frequency | 40 GS/s |
Number of Channels | 16 |
Analog Input Bandwidth | 1.2 GHz |
SFDR | 32 dB |
ENOB | 4.5 bits |
Package | Flip Chip Ball Grid Array (FC BGA) 256 |
Size | 21 mm×21 mm×2.53 mm |
Pitch | 1.27 mm |
Output Interface | (Current Mode Logic) CML |
Static Level | 1C |
On the premise that the channel is aligned, the offset mismatch is calibrated. The spectrum before and after calibration is shown in Figure 17. The harmonic components are suppressed and the SFDR is improved. The other parameters are shown in Table 3.
Testing Parameters | Before Calibration | After Calibration | Units |
SINAD | 21.65 | 30.16 | dB |
SNR | 21.91 | 30.58 | dB |
SFDR | 34.51 | 40.72 | dB |
ENOB | 4.21 | 5.76 | bits |
The experimental results show that the offset calibration algorithm is effective. The mismatch between channels is compensated.
After 100 iterations of the algorithm, the relationship between related parameters and the number of iterations is shown in Figure 18. Within the first 20 calibration iterations, the rate of change of the parameters is larger and the convergence speed is faster. After 50 iterations, it is basically stable. The offset mismatch Oi of each SADC approaches Oref, and the difference Do between the two approaches 0. The calibration value Ocal approaches a constant value, and the value is written into the M registers of the FPGA. The subsequent sampling sequence directly uses the register value, the result is compensated without iteration.
Compensate the sampling sequence according to the calibration algorithm of gain mismatch, and the spectrum is shown in Figure 19. The comparison before and after shows that the harmonic components are reduced after the algorithm. The test parameters are shown in Table 4. The experimental results show the algorithm in Section 4 is also effective in compensating for gain mismatch.
Testing Parameters | Before Calibration | After Calibration | Units |
SINAD | 21.00 | 29.68 | dB |
SNR | 21.05 | 30.05 | dB |
SFDR | 33.53 | 40.72 | dB |
ENOB | 3.90 | 5.63 | bits |
Gain is the ratio of the output signal to the input signal without unit, so there is no unit of the relevant parameters. Similar to the offset calibration, after 100 iterations of the algorithm, the relationship between the relevant parameters and the number of iterations is shown in Figure 20, the calibration parameters within 20 times have a large change rate and a fast convergence speed. All parameters converge around 50 iterations, for each SADC, its gain mismatch Gi approaches Gref, and the difference Dg approaches 0. The calibration amount Gcal approaches a constant value, and this value is written into the M registers of the FPGA. The subsequent sampling sequence directly uses Gcal to compensate the signal without calibration.
The relationship curve between μ (μo and μg) and the number of iterations is shown in Figure 21. Although μ is less than 1, the number of iterations decreases with the increase of μ, which reduces the amount of calculation, but it affects the accuracy of calibration. When it is greater than 1, the number of iterations will increase accordingly. Therefore, setting μ appropriately can balance the calculation complexity and the calculation accuracy.
In order to verify the advantages proposed in this article, a series of comparative experiments are completed under the same conditions, the details are as follows.
The initial mismatch must be estimated before the calibration, the initial estimation accuracy affects the subsequent operations, we evaluate relative error after the first estimation, it is an average relative error of all SADCs, its unit is %, which can be represented as:
δ=Xi−ˉX¯X×100% | (39) |
where δ is the relative error, Xi is the estimated mismatch, and ¯X is the conventional true value of the mismatch after enough experiments. We compare the relative error with the accumulative average method and method proposed in 1241–2010 IEEE standard [26], in the standard, gain G and offset VOS are represented as:
{G=Q(2N−1)(∑2N−1k=1kT[k]−2N−1∑2N−1k=1T[k])(2N−1)∑2N−1k=1T2[k]−(∑2N−1k=1T[k])2VOS=T[1]+Q(2N−1−1)−G2N−12N−1∑k=1T[k] | (40) |
where Q is the ideal width of a code bin, that is, the full-scale range divided by the total number of codes, N is the resolution of ADC, T[k] is the input value corresponding to the transition between codes k and k−1. Table 5 shows the comparisons of relative errors, references [3] and [4] represent the accumulative and average method, the Monte Carlo method in this article shows smaller relative error and higher estimation accuracy than others.
Parameter (Gain/Offset) | This Work | [26] | [3] & [4] | Units |
Relative Error | 0.01/0.09 | 3.69/4.92 | 3.1/6.47 | % |
After estimating the initial mismatch, we also use the method presented in [3] and [4] to calibrate the ADC in the same experiment condition, the testing parameters are compared in Table 6, the proposed method has better calibration performance, higher accuracy and faster convergence than [3] and [4], the signal quality improves more and the harmonic components caused by the channel mismatch are suppressed.
Parameters (Gain/Offset) | This Work | [3] & [4] | Units |
SINAD | 29.68/30.16 | 23.72/24.48 | dB |
SNR | 30.05/30.58 | 23.81/24.59 | dB |
SFDR | 40.7182/40.7187 | 37.56/37.46 | dB |
ENOB | 5.63/5.76 | 5.08/4.75 | bits |
Number of Iterations | 28/52 | 76/95 | times |
Furthermore, we verify the comparability of the two methods based on the measurement uncertainty uA, which can be represented as:
uA(x)=√∑ni=1(xi−¯x)2n(n−1) | (41) |
where x is the measured quantity, ¯x is the average of x, n is the number of measurements, the uA of SINAD, SNR, SFDR and ENOB are listed in Table 7, which shows the possibility to compare this work with [3] and [4].
uA (Gain/Offset) | This Work | [3] & [4] | Units |
SINAD | 0.0064/0.0044 | 0.0067/0.0076 | dB |
SNR | 0.0077/0.0053 | 0.0080/0.0088 | dB |
SFDR | 0.0530/0.0455 | 0.0530/0.0532 | dB |
ENOB | 2.03×10−4/1.48×10−4 | 2.14×10−4/2.44×10−4 | bits |
Some art works only present the method to calibrate gain mismatch, so we compare the gain error with other art works, different from the relative error, the gain error eg is calculated after calibration and it is an absolute error, which can be represented as:
eg=∑Mi=1(Gi−Gref)M | (42) |
where Gi is the gain of SADCi after calibration, M is the number of channels, this error represents the degree to which the calibrated signal is close to the ideal signal, which is also one of the indicators for judging the performance of calibration. As shown in Table 8, the gain error is smaller than previous works, shows better signal quality after calibration.
This article proposes a method that uses Monte Carlo to estimate the mismatch between ADC channels and combines the channel equalization method to calibrate the offset and gain mismatch. Such method does not need an actual channel as the reference, it is a global calibration, and there are no additional circuits. Compared with the accumulative average method, Monte Carlo method is more stable in the face of random noise. It can flexibly grasp the dynamic changes of transmitted data and does not require additional front-end processing circuits; besides, the estimation is more accurate compared to just average the mismatch, and high-precision estimation shortens convergence time. At a sampling frequency of 40 GS/s, a 16-channel TI ADC reaches an SFDR of 41 dB and an SNR of 30 dB, increases the ENOB from 4.06 bits to 5.68 bits, the performance is better than previous works (including IEEE Standard) at the same experiment condition. The results verify the novelty of the method, and highlight the advantages compared to other works in the literature, based on the high accuracy of estimation, this work increases the convergence speed, and saves more power consumption for the subsequent operations, such as frame synchronization, modulation format recognition and carrier recovery. As a result, it is an effective method in ADC calibration.
The authors would like to acknowledge the Natural Science Foundation of Hunan Province (Grant No. 2018JJ3607), Natural Science Foundation of China (Grant No. 51575517), and National Technology Foundation Project (Grant No. 181GF22006) and Acela Micro Co., Ltd to provide fund and technical support for conducting experiments.
We declare that we have no financial and personal relationships with other people or organizations that can inappropriately influence our work, there is no professional or other personal interest of any nature or kind in any product, service and/or company that could be construed as influencing the position presented in, or the review of, the manuscript entitled.
[1] | J. Henkel, Attacking AIDS With a 'Cocktail' Therapy, Fda Consum., 33 (1999), 12-17. |
[2] | A. S. Perelson, P. W. Nelson, Mathematical Analysis of HIV-1 Dynamics in Vivo, Siam Rev., 41 (1999), 3-44. |
[3] | V. D. Martino, T. Thevenot, J. F. Colin, N. Boyer, M. Martinot, F. D. Michele, et al., Influence of HIV infection on the response to interferon therapy and the long-term outcome of chronic hepatitis B, Gastroenterology, 123 (2002), 1812-1822. |
[4] | D. Lamarre, A. Daniel, C. Paul, M. Bailey, P. Beaulieu, G. Bolger, et al., An NS3 protease inhibitor with antiviral effects in humans infected with hepatitis C virus, Nature, 426 (2003), 186-189. |
[5] | E. D. Clercq, Antiviral drugs in current clinical use, J. Clin. Virol., 30 (2004), 0-133. |
[6] | A. Bouhnik, M. Preau, E. Vincent, M. P. Carrieri, H. Gallais, G. Gallais, et al., Depression and clinical progression in HIV-infected drug users treated with highly active antiretroviral therapy, Antivir. Ther., 10 (2005), 53-61. |
[7] | M. Perreau, R. Banga, G. Pantaleo, Targeted Immune Interventions for an HIV-1 Cure, Trends Mol. Med., 23 (2017), 945-961. |
[8] | T. Bruel, B. F. Guivel, S. Amraoui, M. Malbec, L. Richard, K. Bourdic, et al., Elimination of HIV-1-infected cells by broadly neutralizing antibodies, Nat. Commun., 7 (2016), 10844. |
[9] | M. N. Wykes, S. R. Lewin, Immune checkpoint blockade in infectious diseases, Nat. Rev. Immunol., 18 (2017), 91-104. |
[10] | R. M. Ruprecht, Anti-HIV Passive Immunization: New Weapons in the Arsenal, Trends Microbiol., 25 (2017), 954-956. |
[11] | N. Seddiki, Y. Levy Therapeutic HIV-1 vaccine: time for immunomodulation and combinatorial strategies, Curr. Opin. HIV AIDS., 13 (2018), 119-127. |
[12] | A. L. Gill, S. A. Green, S. Abdullah, C. L. Saout, S. Pittaluga, H. Chen, et al., Programed death-1/programed death-ligand 1 expression in lymph nodes of HIV infected patients, AIDS., 30 (2016), 2487-2493. |
[13] | J. Arthos, C. Cicala, E. Martinelli, K. Macleod, D. Van Ryk, D. Wei, et al., HIV-1 envelope protein binds to and signals through integrin alpha4beta7, the gut mucosal homing receptor for peripheral T cells, Nat. Immunol., 9 (2008), 301-309. |
[14] | K. A. O'Connell, J. R. Bailey, J. N. Blankson, Elucidating the elite: mechanisms of control in HIV-1 infection, Trends Pharmacol. Sci., 30 (2009), 631-637. |
[15] | E. S. Rosenberg, M. Altfeld, S. H. Poon, M. N. Phillips, B. M. Wilkes, R. L. Eldridge, et al., Immune control of HIV-1 after early treatment of acute infection, Nature, 407 (2000), 523-526. |
[16] | S. Liu, X. Lu, Y. Chen, B. Li, A delayed HIV-1 model with virus waning term, Math. Biosci. Eng., 13 (2016), 135-157. |
[17] | N. L. Komarova, E. Barnes, P. Klenerman, D. Wodarz, Boosting immunity by antiviral drug therapy: a simple relationship among timing, efficacy, and success, Proc. Natl. Acad. Sci. USA, 100 (2003), 1855-1860. |
[18] | D. D. Richman, D. Havlir, J. Corbeil, D. Looney, D. Pauletti, Nevirapine resistance mutations of human immunodeficiency virus type 1 selected during therapy, J. Virol., 68 (1994), 1660-1666. |
[19] | D. R. Bangsberg, F. M. Hecht, E. D. Charlebois, A. R. Zolopa, M. Holodniy, L. Sheiner, et al., Adherence to protease inhibitors, HIV-1 viral load, and development of drug resistance in an indigent population, AIDS., 14 (2000), 357-366. |
[20] | B. J. Epstein, Drug Resistance among Patients Recently Infected with HIV, N. Engl. J. Med., 347 (2002), 1889-1890. |
[21] | M. S. Hirsch, H. F. Gunthard, J. M. Schapiro, V. Brun, S. M. Hammer, V. A. Johnson, et al., Antiretroviral Drug Resistance Testing in Adult HIV-1 Infection: 2008 Recommendations of an International AIDS Society-USA Panel, Clin. Infect. Dis., 347 (2008), 266-285. |
[22] | The Wistar Institute, Interferon Decreases HIV-1 Viral Levels and Controls Virus after Stopping Antiretroviral Therapy in Patients, 2012 Conference on Retroviruses and Opportunistic Infections, 2012. Available from: https://www.positivelypositive.ca/hiv-aids-news/Interferon_decreases_HIV-1_levels.html. |
[23] | M. A. Nowak, S. Bonhoeffer, A. M. Hill, R. Boehme, H. C. Thomas, H. Mcdade, et al., Viral dynamics in hepatitis B virus infection, Proc. Natl. Acad. Sci. USA, 93 (1996), 4398-4402. |
[24] | S. Bonhoeffer, R. M. May, G. M. Shaw, M. A. Nowak, Virus dynamics and drug therapy, Proc. Natl. Acad. Sci. USA, 94 (1997), 6971-6976. |
[25] | D. Wodarz, J. P. Christensen, A. R. Thomsen, et al., The importance of lytic and nonlytic immune responses in viral infections, Trends in Immunol., 23 (2002), 194-200. |
[26] | C. Bartholdy, J. P. Christensen, D. Wodarz, A. R. Thomsen, Persistent Virus Infection despite Chronic Cytotoxic T-Lymphocyte Activation in Gamma Interferon-Deficient Mice Infected with Lymphocytic Choriomeningitis Virus, J. Virol., 74 (2002), 10304-10311. |
[27] | Y. Pei, C. Li, X. Liang, Optimal therapies of a virus replication model with pharmacological delays based on RTIs and PIs, J. Phys. A Math. Theor., 50 (2017), 455601. |
[28] | H. Shu, L. Wang, Joint impacts of therapy duration, drug efficacy and time lag in immune expansion on immunity boosting by antiviral therapy, J. Biol. Sys., 25 (2017), 105-117. |
[29] | H. Shu, L. Wang, J. Watmough, Sustained and transient oscillations and chaos induced by delayed antiviral immune response in an immunosuppressive infection model, J. Math. Biol., 68 (2014), 477-503. |
[30] | M. A. Nowak, C. R. Bangham, Population Dynamics of Immune Responses to Persistent Viruses, Science, 272 (1996), 74-79. |
[31] | J. E. Schmitz, M. J. Kuroda, S. Santra, V. G. Sasseville, M. A. Simon, M. A. Lifton, et al., Control of Viremia in Simian Immunodeficiency Virus Infection by CD8+ Lymphocytes, Science, 283 (1999), 857-860. |
[32] | C. R. Bangham, The immune response to HTLV-I, Curr. Opin. Immunol., 12 (2000), 397-402. |
[33] | R. J. De Boer, A. S. Perelson, Target Cell Limited and Immune Control Models of HIV Infection: A Comparison, J. Theor. Biol., 190 (1998), 201-214. |
[34] | A. Pugliese, A. Gandolfi, A Simple Model of Pathogen Immune Dynamics Including Specific and Non-Specific Immunity, Math. Biosci., 214 (2008), 73-80. |
[35] | A. Fenton, J. Lello, M. B. Bonsall, Pathogen responses to host immunity: the impact of time delays and memory on the evolution of virulence, Proc. Biol. Sci., 273 (2006), 2083-2090. |
[36] | N. Mcdonald, Time lags in biological models, Springer Science & Business Media, 1978. |
[37] | S. Wain-Hobson, Virus Dynamics: Mathematical Principles of Immunology And Virology, Nat. Med., 410 (2001), 412-413. |
[38] | L. Rong, A. S. Perelson, Modeling HIV persistence, the latent reservoir, and viral blips, J. Theor. Biol., 260 (2009), 308-331. |
[39] | J. K. Hale, S. M. Verduyn Lunel, Introduction to Functional Differential Equations, Introduction to functional differential equations, 1993. |
[40] | E. Beretta, Y. Kuang, Geometric stability switch criteria in delay differential systems with delay dependent parameters, SIAM J. Math. Anal., 33 (2002), 1144-1165. |
[41] | K. L. Cooke, S. Busenberg, Vertically transmitted diseases, Vertically Transmitted Diseases, 1993. |
[42] | B. D. Hassard, N. D. Kazarinoff, Theory and applications of Hopf bifurcation, CAMBRIDGE UNIV. PR., 1981. |
[43] | S. Bonhoeffer, M. Rembiszewski, G. M. Ortiz, D. F. Nixon, Risks and benefits of structured antiretroviral drug therapy interruptions in HIV-1 infection, AIDS., 14 (2000), 2313-2322. |
[44] | L. B. Rong, A. S. Perelson, Modeling HIV persistence, the latent reservoir, and viral blips, J. Theor. Biol., 260 (2009), 308-331. |
[45] | S. Wang, F. Xu, L. Rong, Bistability analysis of an HIV model with immune response, J. Biol. Sys., 25 (2017), 677-695. |
[46] | B. Ingalls, M. Mincheva, M. R. Roussel, Parametric Sensitivity Analysis of Oscillatory Delay Systems with an Application to Gene Regulation, Bull. Math. Biol., 79 (2017), 1539-1563. |
[47] | T. Ma, Y. Pei, C. Li, M. Zhu, Periodicity and dosage optimization of an RNAi model in eukaryotes cells, BMC Bioinformatics, 20 (2019), 340. |
1. | Meng Li, Fangxu Lv, Mingche Lai, Xuqiang Zheng, Heng Huang, Xingyun Qi, Geng Zhang, A fully digital timing background calibration algorithm based on first-order auto-correlation for time-interleaved ADCs, 2024, 152, 18792391, 106330, 10.1016/j.mejo.2024.106330 |
Hardware Configuration | Amount | Remark |
Sampling Clock | 1 | fS>15 GHz |
Input Signal | 1 | fin>1 GHz |
High Frequency Cables | 2 | / |
Balun | 1 | / |
ADC Test Evaluation Board | 1 | 40 GS/s 8-bit |
FPGA | 1 | Xilinx Virtex |
Computer | 1 | / |
Parameter | Index |
Resolution | 8-bit |
Sampling Frequency | 40 GS/s |
Number of Channels | 16 |
Analog Input Bandwidth | 1.2 GHz |
SFDR | 32 dB |
ENOB | 4.5 bits |
Package | Flip Chip Ball Grid Array (FC BGA) 256 |
Size | 21 mm×21 mm×2.53 mm |
Pitch | 1.27 mm |
Output Interface | (Current Mode Logic) CML |
Static Level | 1C |
Testing Parameters | Before Calibration | After Calibration | Units |
SINAD | 21.65 | 30.16 | dB |
SNR | 21.91 | 30.58 | dB |
SFDR | 34.51 | 40.72 | dB |
ENOB | 4.21 | 5.76 | bits |
Testing Parameters | Before Calibration | After Calibration | Units |
SINAD | 21.00 | 29.68 | dB |
SNR | 21.05 | 30.05 | dB |
SFDR | 33.53 | 40.72 | dB |
ENOB | 3.90 | 5.63 | bits |
Hardware Configuration | Amount | Remark |
Sampling Clock | 1 | fS>15 GHz |
Input Signal | 1 | fin>1 GHz |
High Frequency Cables | 2 | / |
Balun | 1 | / |
ADC Test Evaluation Board | 1 | 40 GS/s 8-bit |
FPGA | 1 | Xilinx Virtex |
Computer | 1 | / |
Parameter | Index |
Resolution | 8-bit |
Sampling Frequency | 40 GS/s |
Number of Channels | 16 |
Analog Input Bandwidth | 1.2 GHz |
SFDR | 32 dB |
ENOB | 4.5 bits |
Package | Flip Chip Ball Grid Array (FC BGA) 256 |
Size | 21 mm×21 mm×2.53 mm |
Pitch | 1.27 mm |
Output Interface | (Current Mode Logic) CML |
Static Level | 1C |
Testing Parameters | Before Calibration | After Calibration | Units |
SINAD | 21.65 | 30.16 | dB |
SNR | 21.91 | 30.58 | dB |
SFDR | 34.51 | 40.72 | dB |
ENOB | 4.21 | 5.76 | bits |
Testing Parameters | Before Calibration | After Calibration | Units |
SINAD | 21.00 | 29.68 | dB |
SNR | 21.05 | 30.05 | dB |
SFDR | 33.53 | 40.72 | dB |
ENOB | 3.90 | 5.63 | bits |
Parameter (Gain/Offset) | This Work | [26] | [3] & [4] | Units |
Relative Error | 0.01/0.09 | 3.69/4.92 | 3.1/6.47 | % |
Parameters (Gain/Offset) | This Work | [3] & [4] | Units |
SINAD | 29.68/30.16 | 23.72/24.48 | dB |
SNR | 30.05/30.58 | 23.81/24.59 | dB |
SFDR | 40.7182/40.7187 | 37.56/37.46 | dB |
ENOB | 5.63/5.76 | 5.08/4.75 | bits |
Number of Iterations | 28/52 | 76/95 | times |
uA (Gain/Offset) | This Work | [3] & [4] | Units |
SINAD | 0.0064/0.0044 | 0.0067/0.0076 | dB |
SNR | 0.0077/0.0053 | 0.0080/0.0088 | dB |
SFDR | 0.0530/0.0455 | 0.0530/0.0532 | dB |
ENOB | 2.03×10−4/1.48×10−4 | 2.14×10−4/2.44×10−4 | bits |
This Work | [7] | [32] | [6] | [33] | [34] | |
eg | 0.0018 | 0.01 | 0.04 | −0.015 | −0.02 | 0.05 |