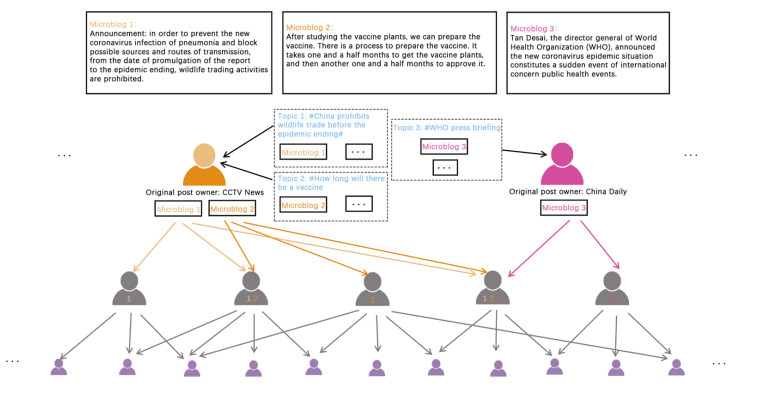
Citation: Fulian Yin, Jiahui Lv, Xiaojian Zhang, Xinyu Xia, Jianhong Wu. COVID-19 information propagation dynamics in the Chinese Sina-microblog[J]. Mathematical Biosciences and Engineering, 2020, 17(3): 2676-2692. doi: 10.3934/mbe.2020146
[1] | Fulian Yin, Hongyu Pang, Lingyao Zhu, Peiqi Liu, Xueying Shao, Qingyu Liu, Jianhong Wu . The role of proactive behavior on COVID-19 infordemic in the Chinese Sina-Microblog: a modeling study. Mathematical Biosciences and Engineering, 2021, 18(6): 7389-7401. doi: 10.3934/mbe.2021365 |
[2] | Fulian Yin, Xinyi Tang, Tongyu Liang, Yanjing Huang, Jianhong Wu . External intervention model with direct and indirect propagation behaviors on social media platforms. Mathematical Biosciences and Engineering, 2022, 19(11): 11380-11398. doi: 10.3934/mbe.2022530 |
[3] | Fulian Yin, Xueying Shao, Jianhong Wu . Nearcasting forwarding behaviors and information propagation in Chinese Sina-Microblog. Mathematical Biosciences and Engineering, 2019, 16(5): 5380-5394. doi: 10.3934/mbe.2019268 |
[4] | Xiaonan Chen, Suxia Zhang . An SEIR model for information propagation with a hot search effect in complex networks. Mathematical Biosciences and Engineering, 2023, 20(1): 1251-1273. doi: 10.3934/mbe.2023057 |
[5] | Hamdy M. Youssef, Najat A. Alghamdi, Magdy A. Ezzat, Alaa A. El-Bary, Ahmed M. Shawky . A new dynamical modeling SEIR with global analysis applied to the real data of spreading COVID-19 in Saudi Arabia. Mathematical Biosciences and Engineering, 2020, 17(6): 7018-7044. doi: 10.3934/mbe.2020362 |
[6] | Cheng-Cheng Zhu, Jiang Zhu . Spread trend of COVID-19 epidemic outbreak in China: using exponential attractor method in a spatial heterogeneous SEIQR model. Mathematical Biosciences and Engineering, 2020, 17(4): 3062-3087. doi: 10.3934/mbe.2020174 |
[7] | Ayako Suzuki, Hiroshi Nishiura . Transmission dynamics of varicella before, during and after the COVID-19 pandemic in Japan: a modelling study. Mathematical Biosciences and Engineering, 2022, 19(6): 5998-6012. doi: 10.3934/mbe.2022280 |
[8] | Haiyan Wang, Nao Yamamoto . Using a partial differential equation with Google Mobility data to predict COVID-19 in Arizona. Mathematical Biosciences and Engineering, 2020, 17(5): 4891-4904. doi: 10.3934/mbe.2020266 |
[9] | Peng An, Xiumei Li, Ping Qin, YingJian Ye, Junyan Zhang, Hongyan Guo, Peng Duan, Zhibing He, Ping Song, Mingqun Li, Jinsong Wang, Yan Hu, Guoyan Feng, Yong Lin . Predicting model of mild and severe types of COVID-19 patients using Thymus CT radiomics model: A preliminary study. Mathematical Biosciences and Engineering, 2023, 20(4): 6612-6629. doi: 10.3934/mbe.2023284 |
[10] | Sarafa A. Iyaniwura, Musa Rabiu, Jummy F. David, Jude D. Kong . Assessing the impact of adherence to Non-pharmaceutical interventions and indirect transmission on the dynamics of COVID-19: a mathematical modelling study. Mathematical Biosciences and Engineering, 2021, 18(6): 8905-8932. doi: 10.3934/mbe.2021439 |
The rapidly evolving novel coronavirus (COVID-19) has received considerable social attention. Major news items combined have generated quite strong fluctuations in public opinions. For example, when Nanshan Zhong, a well-known expert in infectious disease control, confirmed that the COVID-19 could be transmitted from human to human on January 20, 2020 [1], the outbreak-related topics grew exponentially and the personal protection equipment such as surgical masks was instantly in urgent need and in short supply [2]. Understanding how these emerging topics spread in social media to alter the public behaviors is important to help designing effective communication strategies for rapid implementation of public health interventions.
To our best knowledge, there is no appropriate model framework that can be used to analyze multiple-information propagation during a major public health emergency. In consideration of the urgent need to develop theoretically sounding and practical useful technologies to help effective communications of public health interventions, we propose a multiple-information susceptible-discussing-immune model (M-SDI) based on the discussion quantity which under multiple related topics to analyze the public opinion propagation of the COVID-19. In particular, we consider the characteristic user behavior that users may participate in the discussion on different microblogs subordinate to different topics.
Sina-microblog is the most popular microblogging service in China [3] and public opinion concentrates outbreak on that platform. Figure 1 shows the whole process of COVID-19 information propagation in Sina-microblog. Many original post owners can post multiple microblogs related to the epidemic in one of multiple topics. Take CCTV News and China Daily for example. CCTV News reports on multiple topics, while China Daily focuses on a particular topic, and both of them can be discussed by users who are interested in these Weibos, and the relevant discussion can resume in the Sina-microblog later, leading to a multi-level information diffusion process. All users (discussants) can choose to discuss only one Weibo or multiple Weibos, and information propagates through one Weibo or multiple Weibos. This promotes the COVID-19 information dissemination rapidly.
Traditionally, researches of scholars on information mainly focused on single information, and the publication of a large number of papers began with the study of rumors. Considering rumors are similar to epidemiology in several ways, many scholars used susceptible-infected (SI) model [4,5], susceptible-infected-recovered (SIR) model [6,7], susceptible-infected-exposed-recovered (SEIR) model [8,9] and susceptible-infected-susceptible (SIS) [10] model to represent rumor propagation and address relevant issues. Then, classical models were improved to be more targeted and effective. In 2012, Zhao et al. [11] developed a new rumor spreading model called susceptible-infected-hibernator-removed (SIHR) model introducing a new kind of people-Hibernators in order to reduce the maximum rumor influence. In 2014, Zhao et al. [12] added the refutation mechanism in homogeneous social networks to the basic model, which could help authorities reduce the maximum influence of the rumor. In 2015, Zhang et al. [13] studied the cumulative effects of memory on rumor spreading and proposed a model that examined how the memory affected rate changes over time in an artificial network and a real social network. Chen et al. [14] studied the effect of the nodes' role in network on rumor's suppression. Zhang et al. [15] developed the dynamic 8-state ignorance-carrier-spreader-advocate-removal (ICSAR) rumor propagation model to study the function of each influencing factor, which could improve the efficiency of rumor refutation and make emergency plans. Huang et al. [16] constructed a model that took the impact of rumor refuting by the affected enterprise, a microblogging opinion leader and microblogging platform into account. Trpevski D et al. [17], Qian et al. [18], Wang et al. [19], Wang et al. [20], Cheng et al. [21], Liu et al. [22] also extended basic models to study the spread of rumors and had gotten substantial progress.
With the improvement of the academic level, researches about information dissemination are not restricted to rumors. In 2012, Xiong et al. [23] proposed a susceptible-contacted-infected-refractory (SCIR) diffusion model, which contained four possible states to characterize information propagation on online microblogs. Rui et al. [24] proposed a susceptible-potential-infective-removed (SPIR) model that analyzed the diffusion process based on the discrete-time to avoid repeatedly calculating susceptible nodes. And in 2019, we [25] proposed an epidemic model called susceptible-forwarding-immune (SFI) to capture a single information propagation trend in the Sina-microblog.
But in complex social networks, it is rare for single information to exist independently. In other words, multiple information propagation is more common. In 2018, Zan et al. [26] studied the double rumors spreading with different launch time and introduced two kinds of model: double-susceptible-infected-recovered (DSIR) model and comprehensive-DSIR (C-DSIR) model, which focused on the interaction from old rumor to new rumor and the propagation of two rumors posted successively. By investigating states-vectors expressions and attraction of different rumors, they provided the double-rumors dissemination mechanism finally.
Different from previous work, in this paper, we not just consider the behavior of users under one information or two information. Particularly, we focus on the feature that users may re-enter the next related information under different topic after finishing a discussion of one certain information. By analyzing multiple-information propagation mechanism about COVID-19, we can distinctly predict evolution of public opinion.
Since the outbreak of COVID-19, around 4000 major topics appeared in Sina-microblog and the representative topics included #Latest outbreak map#, #Real-time information on joint epidemic prevention and control#, #Wuhan diary#, etc. Figure 2 shows the cumulative discussion quantity of 4059 topics from December 31, 2019 to February 27, 2020, where the ordinate is the logarithm of the cumulative discussion quantity. It can be roughly seen that during the period from December 31, 2019 to January 15-17, 2020, there was only a certain amount of hot topics of COVID-19, however, from January 18-20, 2020, the hot topics about the epidemic kept emerging dramatically.
Table 1 gives the final cumulative discussion quantity of 35 typical topics as of February 27, 2020, among which, #Cheer up! Wuhan# has the highest discussion quantity of 355.26×105. And some of the hot topics like #Nationally confirmed cases of novel coronavirus pneumonia#, #Doctor Wenliang Li passed away# have a discussion quantity over 10×105. Since each hot topic is a part of the entire epidemic event, we pay attention to the total number of the entire COVID-19 information propagation, as shown in Table 2, where 'C' denotes the sum of cumulative discussion. We removed part of data caused by fans of stars to ensure the objectivity and avoid the effect of "water army".
Date | Typical hot topics | Discussion(*105) |
2019.12.31 | #Pneumonia of unknown cause was found in Wuhan# | 1.01 |
2020.1.20 | #Nanshan Zhong confirms the human-to-human transmission of the new coronavirus pneumonia# | 3.89 |
2020.1.21 | #291 cases of novel coronavirus pneumonia have been confirmed in China# | 1.48 |
2020.1.22 | #Nationally confirmed cases of novel coronavirus pneumonia# | 29.03 |
#The COVID-19 is not resistant to alcohol and high temperatures# | 4.97 | |
2020.1.23 | #Cheer up! Wuhan# | 355.26 |
#Wuhan Sealed off# | 6.07 | |
2020.1.25 | #Latest outbreak map# | 25.21 |
2020.1.26 | #5 million people left Wuhan# | 4.31 |
#Wuhan# | 5.41 | |
#Wuhan diary# | 43.69 | |
2020.1.28 | #Real-time information on joint epidemic prevention and control# | 3.71 |
2020.1.29 | #The outbreak is still spreading# | 2.49 |
2020.1.30 | #The World Health Organization# | 2.23 |
2020.1.31 | #Shuanghuanglian can inhibit the new coronavirus# | 5.41 |
#The charity worker Han Hong# | 3.11 | |
2020.2.1 | #Doctor Wenliang Li# | 2.00 |
2020.2.2 | #Huoshenshan hospital completed# | 3.01 |
2020.2.4 | #Hand-written encouragement relay# | 135.28 |
#There is no spring that does not come# | 4.03 | |
2020.2.6 | #Vice governor of Hubei responds to citizens' asking for help online# | 3.92 |
2020.2.7 | #Doctor Wenliang Li is still under rescue# | 4.40 |
#Doctor Wenliang Li passed away# | 13.79 | |
#Wuhan central hospital# | 2.32 | |
2020.2.8 | #Record the anti-epidemic time# | 3.29 |
2020.2.9 | #CCTV Lantern Festival special program# | 2.71 |
2020.2.10 | #Fight against the epidemic clocking action# | 104.38 |
2020.2.12 | #Xinjiang doctors in Wuhan# | 1.68 |
2020.2.13 | #How to save masks scientifically# | 20.48 |
2020.2.14 | #Valentine's day under the epidemic# | 38.93 |
2020.2.15 | #The PK game of cooking food at home# | 20.75 |
2020.2.19 | #Easy epidemic prevention station# | 13.99 |
2020.2.21 | #Epidemic prevention and anti-epidemic east in action# | 6.24 |
2020.2.23 | #It depends on immunity# | 3.54 |
2020.2.25 | #The smile under a mask# | 18.26 |
Date | 2019.12.31 | 2020.1.1 | 2020.1.2 | 2020.1.3 | 2020.1.4 | 2020.1.5 | 2020.1.6 |
C (*105) | 0.87 | 0.96 | 1.09 | 1.18 | 1.23 | 1.35 | 1.43 |
Date | 2020.1.7 | 2020.1.8 | 2020.1.9 | 2020.1.10 | 2020.1.11 | 2020.1.12 | 2020.1.13 |
C (*105) | 1.44 | 1.49 | 1.68 | 1.72 | 1.77 | 1.78 | 1.80 |
Date | 2020.1.14 | 2020.1.15 | 2020.1.16 | 2020.1.17 | 2020.1.18 | 2020.1.19 | 2020.1.20 |
C (*105) | 1.84 | 2.04 | 2.15 | 2.26 | 3 | 4 | 14 |
Date | 2020.1.21 | 2020.1.22 | 2020.1.23 | 2020.1.24 | 2020.1.25 | 2020.1.26 | 2020.1.27 |
C (*105) | 34 | 76 | 238 | 319 | 378 | 434 | 470 |
Date | 2020.1.28 | 2020.1.29 | 2020.1.30 | 2020.1.31 | 2020.2.1 | 2020.2.2 | 2020.2.3 |
C (*105) | 503 | 543 | 578 | 624 | 696 | 756 | 787 |
Date | 2020.2.4 | 2020.2.5 | 2020.2.6 | 2020.2.7 | 2020.2.8 | 2020.2.9 | 2020.2.10 |
C (*105) | 847 | 902 | 993 | 1121 | 1173 | 1211 | 1253 |
Date | 2020.2.11 | 2020.2.12 | 2020.2.13 | 2020.2.14 | 2020.2.15 | 2020.2.16 | 2020.2.17 |
C (*105) | 1294 | 1336 | 1390 | 1438 | 1481 | 1523 | 1557 |
Date | 2020.2.18 | 2020.2.19 | 2020.2.20 | 2020.2.21 | 2020.2.22 | 2020.2.23 | 2020.2.24 |
C (*105) | 1590 | 1617 | 1639 | 1662 | 1688 | 1714 | 1742 |
Date | 2020.2.25 | 2020.2.26 | 2020.2.27 | ||||
C (*105) | 1775 | 1798 | 1821 |
Figure 3 shows the trend of cumulative discussion in Table 2. Public opinion is closely related to the development of the epidemic. When COVID-19 first appeared in Hubei Province, China, there was quite limited attention and discussion about the epidemic. Since January 19, 2020, infections have been reported in other provinces, and the discussion on COVID-19 has gradually increased. By January 23, 2020, 830 confirmed cases had been reported across the country. From that day on, the epidemic broke out rapidly throughout China, and the public opinion ushered in a large-scale outbreak. The cumulative discussion quantity rose swiftly from 7600, 000 on January 22, 2020 to 2, 3800, 000 on January 23, 2020. Since then, the cumulative discussion quantity of COVID-19 has continued rising as the epidemic has continued intensifying.
The whole public opinion can be divided into two major phases: the partial hot discussion phase from December 31, 2019 to January 17, 2020 and the intense outbreak phase from January 17, 2020 on. Each major phase also included several minor stages. In the partial hot discussion phase, the development of public opinions could be divided into 3 small stages: the first stage, from December 31, 2019 to January 8, 2020, had a linear growth and stabilized later; the second stage, from January 8, 2020 to January 14, 2020, broke out in the rapid growth on January 9, 2020 and then increased slowly; and the third short stage, from January 15, 2020 to January 17, 2020, kept a relatively high speed of growth. The phase of intense explosion could also be divided into four small stages: from January 17, 2020 to January 22, 2020 in the first stage, the popularity of public opinion grew at a faster rate than the whole partial hot discussion phase; after the explosion on January 22, 2020 that entered the second stage, the speed slowed down but still grew at a higher rate than the first stage; after a smaller acceleration in January 31, 2020, the public opinion entered the third stage, which first has a lower speed than the second stage but then has a great increase on February 7, 2020 that entered the fourth stage, from February 7, 2020 until now, had a linear growth.
The division of the public opinion of COVID-19 helps us understand the trend caused by the entire epidemic hot topics. For the two large stages and seven small stages, Table 3 gives the urgent hot topics that caused the key time points. The urgent hot topics lead to a wide range of people's attention and reflection, which generated an explosion of discussion quantity.
Date | Urgent hot topics |
2019.12.31 | #Unexplained pneumonia found in Wuhan# |
2019.1.9 | #The pathogen of unexplained pneumonia in Wuhan is a novel coronavirus# |
2019.1.15 | #Wuhan pneumonia does not rule out the possibility of limited human-to-human transmission# |
2019.1.18 | #5 rumors of viral pneumonia in Wuhan# |
2019.1.23 | #Wuhan Sealed off# |
2020.2.1 | #Shuanghuanglian can inhibit the COVID-19# |
2020.2.7 | #Doctor Wenliang Li passed away# |
The propagation of an event like COVID-19 on Sina-microblog accompanied by the post of multiple information within multiple topics is normal behavior. In order to more clearly show the propagation process of the event, a schematic diagram is shown in Figure 4 Taking the propagation of three Weibos under three topics as an example, the user's overall state of integrated information propagation is given.
Discussing is a kind of behavior that reflects users' participation in information propagation, which includes posting a new Weibo (like Twit in Twitter), forwarding that or giving some comments. The Weibos posted by original post owners, such as the red nodes, and the Weibo can be discussed separately by single spreaders with interest in which users can participate in the discussion about the outbreaks, such as the black nodes. Especially, some co-spreaders between different topics or different Weibos discuss the related information successively they contacted because of the correlation about the event, such as the green nodes. Of course, there will also be many users who contact information choose to be silent (un-spread node) because they do not want to discuss in the COVID-19 event, such as the yellow node. In real-world, the number of topics and Weibos cannot be clearly calculated.
The discussion quantity of the whole COVID-19 is composed of many topics with multiple Weibos. Different from the traditional public hot events, the outbreak is causing great public concern. With the continuous development of the COVID-19, there is a high level of repetition in the public discussion on different topics. In addition to the public's repeated reading on different topics of the outbreak, users will also make subjective decisions on whether or not to discuss each Weibo in the topic. As discussion is a measure of information dissemination, in this paper, we build the multiple-information susceptible-discussing-immune (M-SDI) dynamics model with considering the "re-discussing" on the impact of public opinion propagation.
The propagation dynamics model based on discussion quantity of COVID-19 constructed in this paper is shown in Figure 5. Here, we only consider the accessible population in the process of information propagation, and only pay attention to the information diffusion caused by users' discussing behavior. It is assumed that all users that can be contacted in the whole process of the event developing are in a closed environment, and the total number of users (N) remains unchanged. At any time, each individual in the crowd may be in one of the following three states: the susceptible state (S), the discussing state (D) and the immune state (I), where the D state is consisted of many topics related to the COVID-19.
The susceptible users can contact one Weibo of one topic with an average exposure rate β and discuss it with the discussion probability p to become discussing users, those who keep silence in the event and go straight to the immune state are with direct immunity probability q. The discussing users can become immune users who inactive to the event with an average inactive rate α, with 1/α being the average duration where a D-user remains active in contacting.
The core of our model is to study the role of repeated discussing through exposures to different Weibos in different topics about COVID-19. Hence, we use the parameter θ to describe the "re-discussing" probability for a discussing user who can return a new round of susceptible state of COVID-19.
In particular, each user may have a unique state, that is, at the same time, each user can be only one of the susceptible, discussing or immune states. We obtain the following M-SDI dynamics model:
S′(t)=−βS(t)D(t)+(1−p−q)βS(t)D(t)+θαD(t), | (4.1) |
D′(t)=pβS(t)D(t)−αD(t), | (4.2) |
I′(t)=qβS(t)D(t)+(1−θ)αD(t). | (4.3) |
where ′=d/dt is the derivative with respect to t. The behavior transformation and state transition of the masses can also be interpreted as follow:
Discussing: Since an active discussing user will contact an average number of βN users per time and the probability of a contacted user is a susceptible user is S(t)/N, among which pβN will choose to discuss the Weibo subjectively. Hence, the number of new discussing users is pβN(S(t)/N)D(t)=pβS(t)D(t). Direct immune: Some susceptible users will not participate into discussing and enter the immune state directly because they want to keep silence in the event, and the number of new discussing users is qβS(t)D(t). Accordingly, there are still (1−p−q)βS(t)D(t) users who have not experienced state transition. They may simply be not interested in the Weibo they have contacted and will remain in a susceptible state waiting for the next Weibo about COVID-19. Timeout immune: The average number of inactive users will be αD(t) per time, among which θαD(t) will back to the susceptible state where exposures to another Weibo within same topic or with other topics can start a new round of discussing, and (1−θ)αD(t) will go to the immune state directly out of an active period.
The Sina-microblog provides a piece of important information directly is the number of cumulative discussing population within a topic about COVID-19, and we calculate the sum of the whole event shown in Table 3, given by
C(t)=∫t0pβS(t)D(t)dt. | (4.4) |
The corresponding differential equation can be expressed as:
C′(t)=pβS(t)D(t). | (4.5) |
Considering the initial condition: D0=C0, I0=0 and S0=N−D0. From Eqs.4.3 and Eqs.4.5 it follows I(t) and C(t) are increasing since I′(t)=qβS(t)D(t)+(1−θ)αD(t)>0, C′(t)=pβS(t)D(t)>0, therefore the final states are I∞=limt→∞I(t)<N, C∞=limt→∞C(t)<N, D(t) tends to 0 (F∞=0) and S∞=N−I∞. Here C∞ is the final size of the COVID-19 discussing.
Public opinion discussion reproduction ratio ℜ0:
We define the reproduction ratio ℜ0(t) to describe the outbreak of public opinion at each time t. The outbreak of discussion on COVID-19 at time t is given by D′(t)=pβS(t)D(t)−αD(t)>0, and the population will never take off since D′(t)=pβS(t)D(t)−αD(t)<0. Then we deduce
ℜ0(t)=pβS(t)α | (4.6) |
as the discussion reproduction ratio. The ℜ0(t) denotes the number of D-population generated by topics about COVID-19 during an active period, which is determined by the average exposures rate β, the average inactive rate α, the discussing probability p and the susceptible users S(t). When ℜ0(t)<1 the D-population of the event will decline which implies the propagation can never take off. And when ℜ0(t)>1, it means the D-population grows exponentially initially.
We have divided the development of COVID-19 information dissemination into several stages as shown in Figure 3 which are affected by some urgent hot topics shown in Table 2. Although we cannot control the occurrence of emergency incidents, it is very important that, in each period of a stage, we can predict the trend of public opinion based on the existing data before the emergency comes.
To use our M-SDI model to explore some distinctions of qualitative behaviors for prediction, we use the LS method to estimate the model parameters and the initial susceptible population. The vector can be set as Θ=(β,p,q,α,θ,S0), and the corresponding numerical calculation based on the parameter vector for C(t) is denoted by fC(k,Θ). The LS error function
LS=∑Tk=0|fC(k,Θ)−Ck|2 | (5.1) |
is used in our calculation, where Ck denotes the actual cumulative number of discussions given in Table 2, and k=0,1,2... is the sampling time, once a day here. In order to predict the public opinion trend at various stages earlier, we estimate the parameters of our M-SDI model with at least 3-4 days' data. In particular, we increase the sampling frequency when 3-4 sampling points cannot meet the parameters estimating conditions. We use DEDiscover software to solve this LS problem.
Figure 6 gives the numerical experiment results for prediction at each phase. The partial hot discussion phase from December 31, 2019 to January 17, 2020 has the number of cumulative discussion quantity in the order of one hundred thousand as shown in Figure 6(a)-6(c), and the intense outbreak phase from January 17, 2020 until now is nearly tens of millions shown in Figure 6(d)-6(g), where the red star denotes the actual cumulative discussion quantity we use to estimate the parameters for prediction, the pink circle denotes the actual cumulative discussion quantity we want to predict, and the blue line denotes the predicted results.
At the first partial hot discussion phase, the topic #Unexplained pneumonia found in Wuhan# attracted public attention and the discussion quantity of COVID-19 began to increase. We predict the public opinion trend of COVID-19 with the data from December 31, 2019 to January 3, 2020 and it achieves a good data fitting with the actual data until January 7, 2020, as shown in Figure 6(a1). Thus, we increase the data to January 7, 2020 and predict again, fortunately, we get a satisfactory result until the end of this stage, as shown in Figure 6(a2). After the second parameter estimation, the first stage ends quickly since the topic #The pathogen of unexplained pneumonia in Wuhan is a novel coronavirus# exploded on January 9, 2020. We use three days′ data until January 10, 2020 to realize the third prediction, as shown in Figure 6(b1), and have the next three days′ good data fitting. The fourth prediction shown in Figure 6(b2) is not meaningful because the stage finished after this prediction. Similarly, there is a turning point in the third stage since the topic #Wuhan pneumonia does not rule out the possibility of limited human-to-human transmission# exploded on January 15, 2020, our M-SDI model also has a good result although this period is short, as shown in Figure 6(c1)-(c2).
The second intense outbreak phase is the most important period in COVID-19 public opinion propagation. The first stage only existed six days with the evoke of #rumors of viral pneumonia in Wuhan# in January 18, 2020. During this stage, our M-SDI model use only three days' data until January 19, 2020 to realize a good prediction until the end of this stage, as shown in Figure 6(d1). The second prediction in this intense outbreak phase also has no use (as shown in Figure 6(d2))with the outbreak of #Wuhan Sealed off# on January 23, 2020. This is the topic that has caused the most public opinion so far and the public opinion with different topics has continued until now. We use the data between January 22, 2020 and January 24, 2020 to estimate the parameters and predict the trend of public opinion of the next four days, as shown in Figure 6(e1). In addition, we increase the data until January 28, 2020 to predict the rest of the public opinion trend in this stage, as shown in Figure 6(e2). With two prediction, our M-SDI model can well predict most of the data in this important period. We use the data between January 31, 2020 and February 2, 2020 to realize a good prediction until the end of this stage, as shown in Figure 6(f1). Then we increase the data until February 5, 2020 to predict the rest of the public opinion trend in this stage, as shown in Figure 6(f2). This stage ends since the topic #Doctor Wenliang Li passed away# exploded on February 7, 2020, then we use the data between February 6, 2020 and February 8, 2020 to realize a good prediction until the end of this stage, as shown in Figure 6(g1). In addition, we increase the data until February 11, 2020 to predict the rest of the public opinion trend in this stage, and we can also have a good data fitting results as shown in Figure 6(g2).
Figure 7 shows the whole prediction of discussion quantity for COVID-19, where the red star denotes the actual number of cumulative discussion quantity and the blue triangle denotes the predicted results with our M-SDI model. At partial hot discussion phase, we can make a good prediction of the development process at seven days which are January 4-6, 8, 11-13, 17, 2020. And at the intense outbreak phase, we have a good prediction at eleven days which are January 20-22, 25-31, February 3-6, 9-now, 2020. It can be concluded that, without the occurrence of emergency incidents, our M-SDI model can well predict the trend of public opinion based on the existing data for the whole COVID-19 public hot events.
From data fitting, more public opinion properties can also be obtained. Table 4 gives the results of estimated parameters about the influencing factors according to different time periods. From the results of parameter estimation, it can be seen that at the early stage of partial hot discussion phase (Figure 6(a)), although there are many susceptible users exposed, they keep silence to the topics about the novel coronavirus, so the average probability of entering the discussion state p after exposure is small, and the probability of directly entering the immune state q is large, but once the user enters the discussion state, it will be active for a long time. In the next two stages (Figure 6(b), (c)), the parameter p increased and the cumulative number of discussions continued to increase, but there was no sharp outbreak.
β | p | q | α | θ | S0 | |
Figure 6(a1) | 0.1118 | 0.0082 | 0.6604 | 1.3443×10−6 | 0.8016 | 125.9721×105 |
Figure 6(a2) | 0.3078 | 0.0268 | 0.4592 | 2.1700×10−9 | 0.2390 | 14.9323×105 |
Figure 6(b1) | 0.0257 | 0.4352 | 0.0068 | 1.2132 | 0.9997 | 16.0828×105 |
Figure 6(b2) | 0.9032 | 0.0481 | 0.6979 | 0.2236 | 0.9867 | 4.1934×105 |
Figure 6(c1) | 0.0045 | 0.1619 | 0.0113 | 0.6268 | 0.9972 | 181.2324×105 |
Figure 6(c2) | 0.0155 | 0.3977 | 0.9945 | 0.4330 | 0.0036 | 19.6208×105 |
Figure 6(d1) | 0.0346 | 0.0275 | 4.0345×10−4 | 1.0443×10−5 | 0.4732 | 648.2643×105 |
Figure 6(d2) | 0.0110 | 0.0042 | 4.3398×10−6 | 2.3233×10−5 | 0.9729 | 15567×105 |
Figure 6(e1) | 0.0432 | 0.1485 | 0.0956 | 0.4879 | 0.9808 | 307.3187×105 |
Figure 6(e2) | 0.4196 | 0.5003 | 0.1059 | 0.8724 | 0.9337 | 94.0182×105 |
Figure 6(f1) | 0.0020 | 0.2662 | 0.1001 | 0.6349 | 0.7655 | 198.4130×105 |
Figure 6(f2) | 0.1086 | 0.7769 | 1.2098×10−13 | 0.0897 | 1.0000 | 8.9430×105 |
Figure 6(g1) | 0.0047 | 0.1183 | 0.0441 | 1.5000 | 0.9937 | 10.0017×105 |
Figure 6(g2) | 0.2737 | 0.4832 | 0.4094 | 0.1000 | 0.9925 | 116.2541×105 |
Entering the early stage of intense outbreak phase (Figure 6(d)), the probability of direct immune q after contacting the information has been greatly reduced, indicating that most users participate in the discussion, and the active time 1/α has increased, leading that public opinion enter a rapid outbreak phase. Then in the second stage (Figure 6(e)), the parameter p is higher than the previous stage and the parameter θ has maintained a high value nearly 1, which indicates that users participate in the discussion with a high probability and they will enter susceptible state of another information with a high probability, therefore, public opinion continues to spark a trend in this stage. At the sixth stage (Figure 6(f)), the parameter p is higher than the previous stage, which indicates that users participate in the discussion with a high probability. Therefore, public opinion continues to explode in this stage. At the last stage, (Figure 6(g)), with the development of the COVID-19, the parameter p reduced and the active time 1/α has decreased. Hence, public opinion about the COVID-19 will grow steadily.
Throughout the entire process, the important parameter θ remains at a high value in most stages, indicating that the public users will participate in the discussion of another Weibo or topic with a high probability after participating in the discussion of one Weibo or topic during the develop of COVID-19. Hence, the probability of repeated discussions is at a high level.
Throughout the entire public opinion development process of COVID-19, Figure 8 gives the prediction results in the future which uses the entire data at the intense outbreak phase and Table 5 shows some important values of parameter estimation. Unfortunately, with the development of the epidemic, Figure 8 shows that the public opinion will continue to erupt in a long time.
Name | Estimated Value | Standard Error | CI Low Bound | CI High Bound | p-value | t-statistic | Min | Max |
S0(*105) | 168.8749 | 8.7633 | 151.1021 | 186.6476 | 1.4913×10−20 | 19.2707 | 1.0000 | 2.0000×104 |
α | 0.9792 | 0.5558 | -0.1481 | 2.1064 | 0.0866 | 1.7617 | 0.0000 | 1.50000 |
β | 0.0179 | 0.0049 | 0.0080 | 0.0278 | 7.8418×10−4 | 3.6681 | 0.0000 | 1.0000 |
p | 0.5756 | 0.1799 | 0.2107 | 0.9405 | 0.0029 | 3.1990 | 0.0000 | 1.0000 |
q | 0.0017 | 0.0318 | -0.0628 | 0.0661 | 0.9588 | 0.0520 | 0.0000 | 1.0000 |
θ | 0.9776 | 0.0660 | 0.8439 | 1.1114 | 6.7306×10−17 | 14.8202 | 0.0000 | 1.0000 |
In addition, Table 6 gives the results of the reproduction ratio ℜ0 at each time. When ℜ0>1 on a certain day, it means that public opinion will continue to explode. When ℜ0<1, it means the D-population of the event will decline. The results show that in the early stage of COVID-19, it has the greatest reproduction ratio ℜ0=1.7769 and breaks out quickly. With the development of the epidemic, ℜ0 gradually decreases and stabilizes around 0.97, which indicates that in the future, the information on COVID-19 will continue to erupt slowly until it stabilizes if there is no violent information outbreak, which verifies the conclusion of Figure 8.
Data | 2019.12.31 | 2020.1.1 | 2020.1.2 | 2020.1.3 | 2020.1.4 | 2020.1.5 |
ℜ0 | 1.7769 | 1.7526 | 1.7042 | 1.6171 | 1.4849 | 1.3279 |
Data | 2020.1.6 | 2020.1.7 | 2020.1.8 | 2020.1.9 | 2020.1.10 | 2020.1.11 |
ℜ0 | 1.1878 | 1.0905 | 1.0340 | 1.0044 | 0.9895 | 0.9821 |
Data | 2020.1.12 | 2020.1.13 | 2020.1.14 | 2020.1.15 | 2020.1.16 | 2020.1.17 |
ℜ0 | 0.9785 | 0.9766 | 0.9758 | 0.9753 | 0.9750 | 0.9749 |
Data | 2020.1.18 | 2020.1.19 | 2020.1.20 | 2020.1.21 | 2020.1.22 | 2020.1.23 |
ℜ0 | 0.9748 | 0.9748 | 0.9747 | 0.9747 | 0.9748 | 0.9747 |
Data | 2020.1.24 | 2020.1.25 | 2020.1.26 | 2020.1.27 | 2020.1.28 | 2020.1.29 |
ℜ0 | 0.9747 | 0.9747 | 0.9747 | 0.9747 | 0.9747 | 0.9747 |
Data | 2020.1.30 | 2020.1.31 | 2020.2.1 | 2020.2.2 | 2020.2.3 | 2020.2.4 |
ℜ0 | 0.9747 | 0.9747 | 0.9747 | 0.9747 | 0.9747 | 0.9747 |
Data | 2020.2.5 | 2020.2.6 | 2020.2.7 | 2020.2.8 | 2020.2.9 | 2020.2.10 |
ℜ0 | 0.9748 | 0.9748 | 0.9748 | 0.9747 | 0.9747 | 0.9746 |
Data | 2020.2.11 | 2020.2.12 | 2020.2.13 | 2020.2.14 | 2020.2.15 | 2020.2.16 |
ℜ0 | 0.9746 | 0.9747 | 0.9747 | 0.9748 | 0.9748 | 0.9748 |
Data | 2020.2.17 | 2020.2.18 | 2020.2.19 | 2020.2.20 | 2020.2.21 | 2020.2.22 |
ℜ0 | 0.9748 | 0.9748 | 0.9747 | 0.9747 | 0.9747 | 0.9747 |
Data | 2020.2.23 | 2020.2.24 | 2020.2.25 | 2020.2.26 | 2020.2.27 | |
ℜ0 | 0.9747 | 0.9747 | 0.9747 | 0.9747 | 0.9747 |
In this paper, we proposed a multiple-information susceptible-discussing-immune (M-SDI) model based on the quantities of public discussions in Chinese Sina-microblog. Our model considers the particular feature that users are likely to re-enter the susceptible (to a news item) state of related information after discussing a certain piece of information. Using this model, we analyzed the public opinion data on the COVID-19 in Chinese Sina-microblog and stratified events development into different stages according to the disease outbreak development. In each stage, we used a small amount of data for parameter estimation and then used the parameterized model for trend prediction which agreed with the real data well until the next event occurred. We attempted to give our prediction on trend in the near future using all the data available until February 27, 2020, so the usefulness of this predictive model can be tested in the coming days.
To our best knowledge, there is no appropriate model framework that can be used to analyze multiple-information propagation during a major public health emergency. Our work fills the gap and we have shown that our proposed multiple-information susceptible-discussing-immune (M-SDI) model, equipped with early data about news item users can faithfully describe the propagation mechanism of major news items in Chinese social networks during a public health emergency. We hope this model framework provides an important technical tool to predict evolution of public opinions, and thus may provide insights how to communicate public health strategies effectively during a public health crisis including the on-going COVID-19 outbreak.
The work was supported by the National Natural Science Foundation of China (Grant numbers: 61801440), the Natural Science and Engineering Research Council of Canada, the Canada Research Chair Program (JWu), the Fundamental Research Funds for the Central Universities and the High-quality and Cutting-edge Disciplines Construction Project for Universities in Beijing (Internet Information, Communication University of China).
The authors declare no conflict of interest.
[1] | Nanshan Zhong confirmed that the COVID-19 could be transmitted from human to human, Sinamicroblog, January 20, 2020. Available from: https://m.weibo.cn/2656274875/4462928671836931. |
[2] | Surgical masks was instantly in urgent need and in short supply, Sina-microblog, January 21, 2020. Available from: https://m.weibo.cn/2803301701/4463206620092777. |
[3] | Q. Gao, F. Abel, G. J. Houben, Y. Yu, A comparative study of users' microblogging behavior on Sina Weibo and Twitter, International Conference on User Modeling, Adaptation, and Personalization Springer, Berlin, Heidelberg, (2012), 88-101. |
[4] | F. Chen, A susceptible-infected epidemic model with voluntary vaccinations, J. Math. Biol., 53 (2006), 253-272. |
[5] | Z. Lu, S. Gao, L. Chen, Analysis of an SI epidemic model with nonlinear transmission and stage structure, Acta Math. Sci., 23 (2003), 440-446. |
[6] | W. O. Kermack, A. G. McKendrick, Contributions to the mathematical theory of epidemics-I. 1927, Bull. Math. Biol., 53 (1991). |
[7] | L. Stone, B. Shulgin, Z. Agur, Theoretical examination of the pulse vaccination policy in the SIR epidemic model, Math. Comput. Model., 31 (1991), 207-215. |
[8] | D. Xiao, S. Ruan, Global analysis of an epidemic model with nonmonotone incidence rate, Math. Biosci., 208 (2007), 419-429. |
[9] | M. Li, J. S. Muldowney, Global stability for the SEIR model in epidemiology, Math. Biosci., 125 (1995), 155-164. |
[10] | C. Xia, S. Sun, F. Rao, J. Sun, J. Wang, H. Chen, SIS model of epidemic spreading on dynamical networks with community, Front. Comput. Sci. in China, 3 (2009), 361-365. |
[11] | L. Zhao, J. Wang, Y. Chen, Q. Wang, J. Cheng, H. Cui, SIHR rumor spreading model in social networks, Physica A, 391 (2012), 2444-2453. |
[12] | L. Zhao, X. Wang, J. Wang, X. Qiu, W. Xie, Rumor-propagation model with consideration of refutation mechanism in homogeneous social networks, Discrete Dyn. Nat. Soc., 2014 (2014), 1-11. |
[13] | Y. Zhang, J. Xu, A rumor spreading model considering the cumulative effects of memory, Discrete Dyn. Nat. Soc., 2015 (2015), 1-11. |
[14] | P. Chen, N. Gao, The simulation of rumor's spreading and controlling in micro-blog users' network, J. Softw. Eng. Appl., 6 (2013), 102-105. |
[15] | N. Zhang, H. Huang, B. Su, J. Zhao, B. Zhang, Dynamic 8-state ICSAR rumor propagation model considering official rumor refutation, Physica A, 415 (2014), 333-346. |
[16] | J. Huang, Q. Su, A rumor spreading model based on user browsing behavior analysis in microblog, 2013 10th International Conference on Service Systems and Service Management. IEEE, (2013), 170-173. |
[17] | D. Trpevski, W. K. S. Tang, L. Kocarev, Model for rumor spreading over networks, Phys. Rev. E, 81 (2010), 056102. |
[18] | Z. Qian, S. Tang, X. Zhang, Z. Zheng, The independent spreaders involved SIR rumor model in complex networks, Physica A, 429 (2015), 95-102. |
[19] | J. Wang, L. Zhao, R. Huang, SIRaRu rumor spreading model in complex networks, Physica A, 398 (2014), 43-55. |
[20] | Y. Wang, X. Yang, Y. Han, X. Wang, Rumor spreading model with trust mechanism in complex social networks, Commun. Theor. Phys., 59 (2013), 510-516. |
[21] | J. Cheng, Y. Liu, B. Shen, W. Yuan, An epidemic model of rumor diffusion in online social networks, Eur. Phys. J. B, 86 (2013), 1-7. |
[22] | J. Liu, K. Niu, Z. He, J. Lin, Analysis of rumor spreading in communities based on modified SIR model in microblog, International Conference on Artificial Intelligence: Methodology, Systems, and Applications. Springer, Cham, (2014), 69-79. |
[23] | F. Xiong, Y. Liu, Z. Zhang, J. Zhu, Y. Zhang, An information diffusion model based on retweeting mechanism for online social media, Phys. Lett. A, 376 (2012), 2103-2108. |
[24] | X. Rui, F. Meng, Z. Wang, G. Yuan, C. Du, SPIR: The potential spreaders involved SIR model for information diffusion in social networks, Physica A, 506 (2018), 254-269. |
[25] | F. Yin, X. Shao, J. Wu, Nearcasting forwarding behaviors and information propagation in Chinese Sina-Microblog, Math. Biosci. Eng., 16 (2019), 5380-5394. |
[26] | Y. Zan, DSIR double-rumors spreading model in complex networks, Chaos Soliton Fract., 110 (2018), 191-202. |
1. | Yun-Ming Wang, Tian-Yi Guo, Wei-Dong Li, Bo Chen, Direct immune-SCIR public-opinion propagation model based on real-time online users, 2020, 29, 1674-1056, 100204, 10.1088/1674-1056/aba9c0 | |
2. | Mohammed Nasser Al-Suqri, Jamal Mattar Al Salmi, Ayida Mohamed Al Shabibi, THE ROLE OF INFORMATION IN INFLUENCING PUBLIC ATTITUDES AND BEHAVIORS IN A GLOBAL PANDEMIC, 2021, 9, 2395-6518, 46, 10.18510/hssr.2021.915 | |
3. | Fulian Yin, Xueying Shao, Meiqi Ji, Jianhong Wu, Quantifying the Influence of Delay in Opinion Transmission of COVID-19 Information Propagation: Modeling Study, 2021, 23, 1438-8871, e25734, 10.2196/25734 | |
4. | Ibrahim Sabuncu, Mehmet Emin Aydin, 2021, chapter 5, 9781799867364, 78, 10.4018/978-1-7998-6736-4.ch005 | |
5. | Yingying Cheng, Liang'an Huo, Laijun Zhao, Dynamical behaviors and control measures of rumor-spreading model in consideration of the infected media and time delay, 2021, 564, 00200255, 237, 10.1016/j.ins.2021.02.047 | |
6. | Rajanish Kumar Rai, Subhas Khajanchi, Pankaj Kumar Tiwari, Ezio Venturino, Arvind Kumar Misra, Impact of social media advertisements on the transmission dynamics of COVID-19 pandemic in India, 2021, 1598-5865, 10.1007/s12190-021-01507-y | |
7. | Qing Xu, Ziyi Shen, Neal Shah, Raphael Cuomo, Mingxiang Cai, Matthew Brown, Jiawei Li, Tim Mackey, Characterizing Weibo Social Media Posts From Wuhan, China During the Early Stages of the COVID-19 Pandemic: Qualitative Content Analysis, 2020, 6, 2369-2960, e24125, 10.2196/24125 | |
8. | Dongsong Zhang, Lina Zhou, Jaewan Lim, From Networking to Mitigation: The Role of Social Media and Analytics in Combating the COVID-19 Pandemic, 2020, 37, 1058-0530, 318, 10.1080/10580530.2020.1820635 | |
9. | Prasantha Bharathi Dhandapani, Dumitru Baleanu, Jayakumar Thippan, Vinoth Sivakumar, On stiff, fuzzy IRD-14 day average transmission model of COVID-19 pandemic disease, 2020, 7, 2375-1495, 208, 10.3934/bioeng.2020018 | |
10. | Shu-Feng Tsao, Helen Chen, Therese Tisseverasinghe, Yang Yang, Lianghua Li, Zahid A Butt, What social media told us in the time of COVID-19: a scoping review, 2021, 3, 25897500, e175, 10.1016/S2589-7500(20)30315-0 | |
11. | Yuqi Gao, Hang Hua, Jiebo Luo, Analyzing public opinion on COVID-19 through different perspectives and stages, 2021, 10, 2048-7703, 10.1017/ATSIP.2021.5 | |
12. | Xinwei Chai, Xian Guo, Jihua Xiao, Jie Jiang, Analysis of spatiotemporal mobility of shared‐bike usage during COVID‐19 pandemic in Beijing, 2021, 25, 1361-1682, 2866, 10.1111/tgis.12784 | |
13. | Fulian Yin, Xinyu Xia, Yanyan Pan, Yuwei She, Xiaoli Feng, Jianhong Wu, Sentiment mutation and negative emotion contagion dynamics in social media: A case study on the Chinese Sina Microblog, 2022, 594, 00200255, 118, 10.1016/j.ins.2022.02.029 | |
14. | Liang’an Huo, Yuqing Zhang, Effect of Global and Local Refutation Mechanism on Rumor Propagation in Heterogeneous Network, 2022, 10, 2227-7390, 586, 10.3390/math10040586 | |
15. | Mohammad (Behdad) Jamshidi, Sobhan Roshani, Fatemeh Daneshfar, Ali Lalbakhsh, Saeed Roshani, Fariborz Parandin, Zahra Malek, Jakub Talla, Zdeněk Peroutka, Alireza Jamshidi, Farimah Hadjilooei, Pedram Lalbakhsh, Hybrid Deep Learning Techniques for Predicting Complex Phenomena: A Review on COVID-19, 2022, 3, 2673-2688, 416, 10.3390/ai3020025 | |
16. | Yi-Cheng Zhang, Zhi Li, Guo-Bing Zhou, Nai-Ru Xu, Jia-Bao Liu, Wei Wang, The Evolution Model of Public Risk Perception Based on Pandemic Spreading Theory under Perspective of COVID-19, 2021, 2021, 1099-0526, 1, 10.1155/2021/1015049 | |
17. | Xing Zhang, Yan Zhou, Fuli Zhou, Saurabh Pratap, Internet public opinion dissemination mechanism of COVID-19: evidence from the Shuanghuanglian event, 2022, 56, 2514-9288, 283, 10.1108/DTA-11-2020-0275 | |
18. | Omaji Samuel, Akogwu Blessing Omojo, Abdulkarim Musa Onuja, Yunisa Sunday, Prayag Tiwari, Deepak Gupta, Ghulam Hafeez, Adamu Sani Yahaya, Oluwaseun Jumoke Fatoba, Shahab Shamshirband, IoMT: A COVID-19 Healthcare System Driven by Federated Learning and Blockchain, 2023, 27, 2168-2194, 823, 10.1109/JBHI.2022.3143576 | |
19. | Jing He, Yuanbo Qi, Jie Feng, Anling Xiang, Zhihan Liu, Towards Public Opinion Digital Twin: A Conceptual Prototype, 2022, 2022, 1875-919X, 1, 10.1155/2022/3917853 | |
20. | Joanna Sooknanan, Terence A. R. Seemungal, FOMO (fate of online media only) in infectious disease modeling: a review of compartmental models, 2022, 2195-268X, 10.1007/s40435-022-00994-6 | |
21. | Yongxi Jang, Jinling Hua, Bismark Adu Gyamfi, Rajib Shaw, 2022, Chapter 4, 978-981-16-6958-3, 45, 10.1007/978-981-16-6959-0_4 | |
22. | Yang Liu, Yi Chen, Kefan Xie, Jia Liu, Effectiveness and policies analysis of pool testing method for COVID-19, 2023, 52, 0368-492X, 64, 10.1108/K-01-2021-0052 | |
23. | Liyi Liu, Yan Tu, Xiaoyang Zhou, How local outbreak of COVID-19 affect the risk of internet public opinion: A Chinese social media case study, 2022, 71, 0160791X, 102113, 10.1016/j.techsoc.2022.102113 | |
24. | Connie Cai Ru Gan, Shuo Feng, Huiyun Feng, King-wa Fu, Sara E Davies, Karen A Grépin, Rosemary Morgan, Julia Smith, Clare Wenham, #WuhanDiary and #WuhanLockdown: gendered posting patterns and behaviours on Weibo during the COVID-19 pandemic, 2022, 7, 2059-7908, e008149, 10.1136/bmjgh-2021-008149 | |
25. | Ali AlArjani, Md Taufiq Nasseef, Sanaa M. Kamal, B. V. Subba Rao, Mufti Mahmud, Md Sharif Uddin, Application of Mathematical Modeling in Prediction of COVID-19 Transmission Dynamics, 2022, 47, 2193-567X, 10163, 10.1007/s13369-021-06419-4 | |
26. | Gang Deng, Yuting Peng, Yang Tian, Xuzhen Zhu, Analysis of Influence of Behavioral Adoption Threshold Diversity on Multi-Layer Network, 2023, 25, 1099-4300, 458, 10.3390/e25030458 | |
27. | Jiali Yan, The evolution and governance of online rumors during the public health emergency: taking COVID-19 pandemic related rumors as an example, 2022, 17, 1750-9653, 1, 10.1080/17509653.2021.1972353 | |
28. | Quan Xiao, Weiling Huang, Xing Zhang, Shanshan Wan, Xia Li, Internet Rumors During the COVID-19 Pandemic: Dynamics of Topics and Public Psychologies, 2021, 9, 2296-2565, 10.3389/fpubh.2021.788848 | |
29. | Egberto Selerio, June Anne Caladcad, Mary Rose Catamco, Esehl May Capinpin, Lanndon Ocampo, Emergency preparedness during the COVID-19 pandemic: Modelling the roles of social media with fuzzy DEMATEL and analytic network process, 2022, 82, 00380121, 101217, 10.1016/j.seps.2021.101217 | |
30. | Ling Zhang, De Li, Robert J. Boncella, Research on influencing factors of information diffusion in online social networks under different themes, 2021, 39, 0264-0473, 732, 10.1108/EL-12-2020-0329 | |
31. | Xue Gong, Mengchi Hou, Yangyang Han, Hailun Liang, Rui Guo, Application of the Internet Platform in Monitoring Chinese Public Attention to the Outbreak of COVID-19, 2022, 9, 2296-2565, 10.3389/fpubh.2021.755530 | |
32. | Olaide Nathaniel Oyelade, Irunokhai Eric Aghiomesi, Owamoyo Najeem, Ahamed Aminu Sambo, A semantic web rule and ontologies based architecture for diagnosing breast cancer using select and test algorithm, 2021, 1, 26669900, 100034, 10.1016/j.cmpbup.2021.100034 | |
33. | Bin Wang, Enhui Wang, Zikun Zhu, Yangyang Sun, Yaodong Tao, Wei Wang, An explainable sentiment prediction model based on the portraits of users sharing representative opinions in social sensors, 2021, 17, 1550-1477, 155014772110337, 10.1177/15501477211033765 | |
34. | Qingyuan Zhao, Liang Nie, Xiaying Xu, 2023, Chapter 15, 978-981-19-9992-5, 309, 10.1007/978-981-19-9993-2_15 | |
35. | Yue Yu, Yuanshun Tan, Sanyi Tang, Stability analysis of the COVID-19 model with age structure under media effect, 2023, 42, 2238-3603, 10.1007/s40314-023-02330-w | |
36. | Yanyuan Su, Yaming Zhang, Li Weigang, Multi-Stage Information Spreading Model in Simplicial Complexes Driven by Spatiotemporal Evolution of Public Health Emergency, 2023, 11, 2169-3536, 128316, 10.1109/ACCESS.2023.3332656 | |
37. | Majid Jangi, Maryam Jahanbakhsh, Nahid Tavakoli, Hossein Bagherian, Asghar Ehteshami, Sakineh Saghaeian Nejad Isfahani, Mohammad Sattari, Extracting Hidden Patterns of Iranian User Trust in Social Networks Regarding Coronavirus Disease 2019 Using Data Mining Techniques, 2024, 13, 2277-9183, 10.4103/ijehe.ijehe_39_23 | |
38. | Muhammad Adil, Miftachul Huda, Understanding Responses to Worship Regulations in the Pandemic Era: Text Data Mining Analysis in the Indonesian Context, 2023, 14, 2077-1444, 549, 10.3390/rel14040549 | |
39. | Xiaoying Pan, Longkun Tang, A new model for COVID-19 in the post-pandemic era, 2024, 9, 2473-6988, 21255, 10.3934/math.20241032 | |
40. | Guangyu Mu, Zehan Liao, Jiaxue Li, Nini Qin, Ziye Yang, Nebojsa Bacanin, IPSO-LSTM hybrid model for predicting online public opinion trends in emergencies, 2023, 18, 1932-6203, e0292677, 10.1371/journal.pone.0292677 | |
41. | Qingqing Zhou, Fine-grained detection on the public's multi-dimensional communication preferences in emergency events, 2023, 9, 24058440, e16312, 10.1016/j.heliyon.2023.e16312 | |
42. | Longkun Tang, Rong Shen, Xiaoying Pan, A node-based SIRS epidemic model on two-layer interconnected networks: Dynamical analysis of interplay between layers, 2024, 361, 00160032, 106784, 10.1016/j.jfranklin.2024.106784 | |
43. | Alfonso Méndiz-Noguero, Laia Wennberg-Capellades, Elisa Regadera-González, Blanca Goni-Fuste, Public health communication and the Covid-19: A review of the literature during the first wave, 2023, 16992407, 10.3145/epi.2023.may.13 | |
44. | Yu Guo, Hongzhe Xiang, Yongkang Hou, Coping with Unknown Health Crisis via Social Media: A Content Analysis of Online Mutual Aid Group in the Beginning of the COVID-19 Pandemic, 2024, 18, 1935-7893, 10.1017/dmp.2024.278 | |
45. | Manoj Kumar Dash, Gaurvendra Singh, Chetanya Singh, Analyzing the role of social media in addressing public health development in india through a multi-criteria decision-making approach, 2024, 58, 0399-0559, 3621, 10.1051/ro/2024009 | |
46. | Bowen Li, Hua Li, Qiubai Sun, Rongjian Lv, Huining Yan, Dynamics analysis and optimal control study of uncertain information dissemination model triggered after major emergencies, 2024, 12, 2296-424X, 10.3389/fphy.2024.1349284 | |
47. | S. Sankaranarayanan, An approach to statistical analysis-using the average transmission model of Covid 19, 2020, 8, 23193786, 2158, 10.26637/MJM0804/0141 | |
48. | Delin Yuan, Yang Li, Discovering and early predicting popularity evolution patterns of social media emergency information, 2024, 2050-3806, 10.1108/AJIM-10-2023-0450 | |
49. | Zhenhua Yu, Haiyan Zi, Yun Zhang, Shixing Wu, Xuya Cong, Almetwally M. Mostafa, Dynamic modeling and simulation of double-rumor spreaders in online social networks with IS2TR model, 2024, 0924-090X, 10.1007/s11071-024-09538-3 | |
50. | Xuelong Chen, Yiping Chen, Guojie Yin, Hanyue He, Dynamic evolution of information diffusion networks of news agencies in emergencies: a case study of microblogs of urban fire disasters on Sina Weibo, 2023, 1380-7501, 10.1007/s11042-023-16498-0 | |
51. | Yuwei She, Xinyi Jiang, Chang Wu, Fulian Yin, 2022, Modeling and Analyzing the Multi-Information Network Propagation Dynamics on Hot Events, 9781450397056, 609, 10.1145/3581807.3581897 | |
52. | Yuewei Wu, Zhiqiang Zhang, Jianhong Wu, Jinxia Wang, Yuanye Zhou, Fulian Yin, An advanced deep learning framework for simulating information propagation dynamics, 2025, 13, 2296-424X, 10.3389/fphy.2025.1524104 |
Date | Typical hot topics | Discussion(*105) |
2019.12.31 | #Pneumonia of unknown cause was found in Wuhan# | 1.01 |
2020.1.20 | #Nanshan Zhong confirms the human-to-human transmission of the new coronavirus pneumonia# | 3.89 |
2020.1.21 | #291 cases of novel coronavirus pneumonia have been confirmed in China# | 1.48 |
2020.1.22 | #Nationally confirmed cases of novel coronavirus pneumonia# | 29.03 |
#The COVID-19 is not resistant to alcohol and high temperatures# | 4.97 | |
2020.1.23 | #Cheer up! Wuhan# | 355.26 |
#Wuhan Sealed off# | 6.07 | |
2020.1.25 | #Latest outbreak map# | 25.21 |
2020.1.26 | #5 million people left Wuhan# | 4.31 |
#Wuhan# | 5.41 | |
#Wuhan diary# | 43.69 | |
2020.1.28 | #Real-time information on joint epidemic prevention and control# | 3.71 |
2020.1.29 | #The outbreak is still spreading# | 2.49 |
2020.1.30 | #The World Health Organization# | 2.23 |
2020.1.31 | #Shuanghuanglian can inhibit the new coronavirus# | 5.41 |
#The charity worker Han Hong# | 3.11 | |
2020.2.1 | #Doctor Wenliang Li# | 2.00 |
2020.2.2 | #Huoshenshan hospital completed# | 3.01 |
2020.2.4 | #Hand-written encouragement relay# | 135.28 |
#There is no spring that does not come# | 4.03 | |
2020.2.6 | #Vice governor of Hubei responds to citizens' asking for help online# | 3.92 |
2020.2.7 | #Doctor Wenliang Li is still under rescue# | 4.40 |
#Doctor Wenliang Li passed away# | 13.79 | |
#Wuhan central hospital# | 2.32 | |
2020.2.8 | #Record the anti-epidemic time# | 3.29 |
2020.2.9 | #CCTV Lantern Festival special program# | 2.71 |
2020.2.10 | #Fight against the epidemic clocking action# | 104.38 |
2020.2.12 | #Xinjiang doctors in Wuhan# | 1.68 |
2020.2.13 | #How to save masks scientifically# | 20.48 |
2020.2.14 | #Valentine's day under the epidemic# | 38.93 |
2020.2.15 | #The PK game of cooking food at home# | 20.75 |
2020.2.19 | #Easy epidemic prevention station# | 13.99 |
2020.2.21 | #Epidemic prevention and anti-epidemic east in action# | 6.24 |
2020.2.23 | #It depends on immunity# | 3.54 |
2020.2.25 | #The smile under a mask# | 18.26 |
Date | 2019.12.31 | 2020.1.1 | 2020.1.2 | 2020.1.3 | 2020.1.4 | 2020.1.5 | 2020.1.6 |
C (*105) | 0.87 | 0.96 | 1.09 | 1.18 | 1.23 | 1.35 | 1.43 |
Date | 2020.1.7 | 2020.1.8 | 2020.1.9 | 2020.1.10 | 2020.1.11 | 2020.1.12 | 2020.1.13 |
C (*105) | 1.44 | 1.49 | 1.68 | 1.72 | 1.77 | 1.78 | 1.80 |
Date | 2020.1.14 | 2020.1.15 | 2020.1.16 | 2020.1.17 | 2020.1.18 | 2020.1.19 | 2020.1.20 |
C (*105) | 1.84 | 2.04 | 2.15 | 2.26 | 3 | 4 | 14 |
Date | 2020.1.21 | 2020.1.22 | 2020.1.23 | 2020.1.24 | 2020.1.25 | 2020.1.26 | 2020.1.27 |
C (*105) | 34 | 76 | 238 | 319 | 378 | 434 | 470 |
Date | 2020.1.28 | 2020.1.29 | 2020.1.30 | 2020.1.31 | 2020.2.1 | 2020.2.2 | 2020.2.3 |
C (*105) | 503 | 543 | 578 | 624 | 696 | 756 | 787 |
Date | 2020.2.4 | 2020.2.5 | 2020.2.6 | 2020.2.7 | 2020.2.8 | 2020.2.9 | 2020.2.10 |
C (*105) | 847 | 902 | 993 | 1121 | 1173 | 1211 | 1253 |
Date | 2020.2.11 | 2020.2.12 | 2020.2.13 | 2020.2.14 | 2020.2.15 | 2020.2.16 | 2020.2.17 |
C (*105) | 1294 | 1336 | 1390 | 1438 | 1481 | 1523 | 1557 |
Date | 2020.2.18 | 2020.2.19 | 2020.2.20 | 2020.2.21 | 2020.2.22 | 2020.2.23 | 2020.2.24 |
C (*105) | 1590 | 1617 | 1639 | 1662 | 1688 | 1714 | 1742 |
Date | 2020.2.25 | 2020.2.26 | 2020.2.27 | ||||
C (*105) | 1775 | 1798 | 1821 |
Date | Urgent hot topics |
2019.12.31 | #Unexplained pneumonia found in Wuhan# |
2019.1.9 | #The pathogen of unexplained pneumonia in Wuhan is a novel coronavirus# |
2019.1.15 | #Wuhan pneumonia does not rule out the possibility of limited human-to-human transmission# |
2019.1.18 | #5 rumors of viral pneumonia in Wuhan# |
2019.1.23 | #Wuhan Sealed off# |
2020.2.1 | #Shuanghuanglian can inhibit the COVID-19# |
2020.2.7 | #Doctor Wenliang Li passed away# |
β | p | q | α | θ | S0 | |
Figure 6(a1) | 0.1118 | 0.0082 | 0.6604 | 1.3443×10−6 | 0.8016 | 125.9721×105 |
Figure 6(a2) | 0.3078 | 0.0268 | 0.4592 | 2.1700×10−9 | 0.2390 | 14.9323×105 |
Figure 6(b1) | 0.0257 | 0.4352 | 0.0068 | 1.2132 | 0.9997 | 16.0828×105 |
Figure 6(b2) | 0.9032 | 0.0481 | 0.6979 | 0.2236 | 0.9867 | 4.1934×105 |
Figure 6(c1) | 0.0045 | 0.1619 | 0.0113 | 0.6268 | 0.9972 | 181.2324×105 |
Figure 6(c2) | 0.0155 | 0.3977 | 0.9945 | 0.4330 | 0.0036 | 19.6208×105 |
Figure 6(d1) | 0.0346 | 0.0275 | 4.0345×10−4 | 1.0443×10−5 | 0.4732 | 648.2643×105 |
Figure 6(d2) | 0.0110 | 0.0042 | 4.3398×10−6 | 2.3233×10−5 | 0.9729 | 15567×105 |
Figure 6(e1) | 0.0432 | 0.1485 | 0.0956 | 0.4879 | 0.9808 | 307.3187×105 |
Figure 6(e2) | 0.4196 | 0.5003 | 0.1059 | 0.8724 | 0.9337 | 94.0182×105 |
Figure 6(f1) | 0.0020 | 0.2662 | 0.1001 | 0.6349 | 0.7655 | 198.4130×105 |
Figure 6(f2) | 0.1086 | 0.7769 | 1.2098×10−13 | 0.0897 | 1.0000 | 8.9430×105 |
Figure 6(g1) | 0.0047 | 0.1183 | 0.0441 | 1.5000 | 0.9937 | 10.0017×105 |
Figure 6(g2) | 0.2737 | 0.4832 | 0.4094 | 0.1000 | 0.9925 | 116.2541×105 |
Name | Estimated Value | Standard Error | CI Low Bound | CI High Bound | p-value | t-statistic | Min | Max |
S0(*105) | 168.8749 | 8.7633 | 151.1021 | 186.6476 | 1.4913×10−20 | 19.2707 | 1.0000 | 2.0000×104 |
α | 0.9792 | 0.5558 | -0.1481 | 2.1064 | 0.0866 | 1.7617 | 0.0000 | 1.50000 |
β | 0.0179 | 0.0049 | 0.0080 | 0.0278 | 7.8418×10−4 | 3.6681 | 0.0000 | 1.0000 |
p | 0.5756 | 0.1799 | 0.2107 | 0.9405 | 0.0029 | 3.1990 | 0.0000 | 1.0000 |
q | 0.0017 | 0.0318 | -0.0628 | 0.0661 | 0.9588 | 0.0520 | 0.0000 | 1.0000 |
θ | 0.9776 | 0.0660 | 0.8439 | 1.1114 | 6.7306×10−17 | 14.8202 | 0.0000 | 1.0000 |
Data | 2019.12.31 | 2020.1.1 | 2020.1.2 | 2020.1.3 | 2020.1.4 | 2020.1.5 |
ℜ0 | 1.7769 | 1.7526 | 1.7042 | 1.6171 | 1.4849 | 1.3279 |
Data | 2020.1.6 | 2020.1.7 | 2020.1.8 | 2020.1.9 | 2020.1.10 | 2020.1.11 |
ℜ0 | 1.1878 | 1.0905 | 1.0340 | 1.0044 | 0.9895 | 0.9821 |
Data | 2020.1.12 | 2020.1.13 | 2020.1.14 | 2020.1.15 | 2020.1.16 | 2020.1.17 |
ℜ0 | 0.9785 | 0.9766 | 0.9758 | 0.9753 | 0.9750 | 0.9749 |
Data | 2020.1.18 | 2020.1.19 | 2020.1.20 | 2020.1.21 | 2020.1.22 | 2020.1.23 |
ℜ0 | 0.9748 | 0.9748 | 0.9747 | 0.9747 | 0.9748 | 0.9747 |
Data | 2020.1.24 | 2020.1.25 | 2020.1.26 | 2020.1.27 | 2020.1.28 | 2020.1.29 |
ℜ0 | 0.9747 | 0.9747 | 0.9747 | 0.9747 | 0.9747 | 0.9747 |
Data | 2020.1.30 | 2020.1.31 | 2020.2.1 | 2020.2.2 | 2020.2.3 | 2020.2.4 |
ℜ0 | 0.9747 | 0.9747 | 0.9747 | 0.9747 | 0.9747 | 0.9747 |
Data | 2020.2.5 | 2020.2.6 | 2020.2.7 | 2020.2.8 | 2020.2.9 | 2020.2.10 |
ℜ0 | 0.9748 | 0.9748 | 0.9748 | 0.9747 | 0.9747 | 0.9746 |
Data | 2020.2.11 | 2020.2.12 | 2020.2.13 | 2020.2.14 | 2020.2.15 | 2020.2.16 |
ℜ0 | 0.9746 | 0.9747 | 0.9747 | 0.9748 | 0.9748 | 0.9748 |
Data | 2020.2.17 | 2020.2.18 | 2020.2.19 | 2020.2.20 | 2020.2.21 | 2020.2.22 |
ℜ0 | 0.9748 | 0.9748 | 0.9747 | 0.9747 | 0.9747 | 0.9747 |
Data | 2020.2.23 | 2020.2.24 | 2020.2.25 | 2020.2.26 | 2020.2.27 | |
ℜ0 | 0.9747 | 0.9747 | 0.9747 | 0.9747 | 0.9747 |
Date | Typical hot topics | Discussion(*105) |
2019.12.31 | #Pneumonia of unknown cause was found in Wuhan# | 1.01 |
2020.1.20 | #Nanshan Zhong confirms the human-to-human transmission of the new coronavirus pneumonia# | 3.89 |
2020.1.21 | #291 cases of novel coronavirus pneumonia have been confirmed in China# | 1.48 |
2020.1.22 | #Nationally confirmed cases of novel coronavirus pneumonia# | 29.03 |
#The COVID-19 is not resistant to alcohol and high temperatures# | 4.97 | |
2020.1.23 | #Cheer up! Wuhan# | 355.26 |
#Wuhan Sealed off# | 6.07 | |
2020.1.25 | #Latest outbreak map# | 25.21 |
2020.1.26 | #5 million people left Wuhan# | 4.31 |
#Wuhan# | 5.41 | |
#Wuhan diary# | 43.69 | |
2020.1.28 | #Real-time information on joint epidemic prevention and control# | 3.71 |
2020.1.29 | #The outbreak is still spreading# | 2.49 |
2020.1.30 | #The World Health Organization# | 2.23 |
2020.1.31 | #Shuanghuanglian can inhibit the new coronavirus# | 5.41 |
#The charity worker Han Hong# | 3.11 | |
2020.2.1 | #Doctor Wenliang Li# | 2.00 |
2020.2.2 | #Huoshenshan hospital completed# | 3.01 |
2020.2.4 | #Hand-written encouragement relay# | 135.28 |
#There is no spring that does not come# | 4.03 | |
2020.2.6 | #Vice governor of Hubei responds to citizens' asking for help online# | 3.92 |
2020.2.7 | #Doctor Wenliang Li is still under rescue# | 4.40 |
#Doctor Wenliang Li passed away# | 13.79 | |
#Wuhan central hospital# | 2.32 | |
2020.2.8 | #Record the anti-epidemic time# | 3.29 |
2020.2.9 | #CCTV Lantern Festival special program# | 2.71 |
2020.2.10 | #Fight against the epidemic clocking action# | 104.38 |
2020.2.12 | #Xinjiang doctors in Wuhan# | 1.68 |
2020.2.13 | #How to save masks scientifically# | 20.48 |
2020.2.14 | #Valentine's day under the epidemic# | 38.93 |
2020.2.15 | #The PK game of cooking food at home# | 20.75 |
2020.2.19 | #Easy epidemic prevention station# | 13.99 |
2020.2.21 | #Epidemic prevention and anti-epidemic east in action# | 6.24 |
2020.2.23 | #It depends on immunity# | 3.54 |
2020.2.25 | #The smile under a mask# | 18.26 |
Date | 2019.12.31 | 2020.1.1 | 2020.1.2 | 2020.1.3 | 2020.1.4 | 2020.1.5 | 2020.1.6 |
C (*105) | 0.87 | 0.96 | 1.09 | 1.18 | 1.23 | 1.35 | 1.43 |
Date | 2020.1.7 | 2020.1.8 | 2020.1.9 | 2020.1.10 | 2020.1.11 | 2020.1.12 | 2020.1.13 |
C (*105) | 1.44 | 1.49 | 1.68 | 1.72 | 1.77 | 1.78 | 1.80 |
Date | 2020.1.14 | 2020.1.15 | 2020.1.16 | 2020.1.17 | 2020.1.18 | 2020.1.19 | 2020.1.20 |
C (*105) | 1.84 | 2.04 | 2.15 | 2.26 | 3 | 4 | 14 |
Date | 2020.1.21 | 2020.1.22 | 2020.1.23 | 2020.1.24 | 2020.1.25 | 2020.1.26 | 2020.1.27 |
C (*105) | 34 | 76 | 238 | 319 | 378 | 434 | 470 |
Date | 2020.1.28 | 2020.1.29 | 2020.1.30 | 2020.1.31 | 2020.2.1 | 2020.2.2 | 2020.2.3 |
C (*105) | 503 | 543 | 578 | 624 | 696 | 756 | 787 |
Date | 2020.2.4 | 2020.2.5 | 2020.2.6 | 2020.2.7 | 2020.2.8 | 2020.2.9 | 2020.2.10 |
C (*105) | 847 | 902 | 993 | 1121 | 1173 | 1211 | 1253 |
Date | 2020.2.11 | 2020.2.12 | 2020.2.13 | 2020.2.14 | 2020.2.15 | 2020.2.16 | 2020.2.17 |
C (*105) | 1294 | 1336 | 1390 | 1438 | 1481 | 1523 | 1557 |
Date | 2020.2.18 | 2020.2.19 | 2020.2.20 | 2020.2.21 | 2020.2.22 | 2020.2.23 | 2020.2.24 |
C (*105) | 1590 | 1617 | 1639 | 1662 | 1688 | 1714 | 1742 |
Date | 2020.2.25 | 2020.2.26 | 2020.2.27 | ||||
C (*105) | 1775 | 1798 | 1821 |
Date | Urgent hot topics |
2019.12.31 | #Unexplained pneumonia found in Wuhan# |
2019.1.9 | #The pathogen of unexplained pneumonia in Wuhan is a novel coronavirus# |
2019.1.15 | #Wuhan pneumonia does not rule out the possibility of limited human-to-human transmission# |
2019.1.18 | #5 rumors of viral pneumonia in Wuhan# |
2019.1.23 | #Wuhan Sealed off# |
2020.2.1 | #Shuanghuanglian can inhibit the COVID-19# |
2020.2.7 | #Doctor Wenliang Li passed away# |
β | p | q | α | θ | S0 | |
Figure 6(a1) | 0.1118 | 0.0082 | 0.6604 | 1.3443×10−6 | 0.8016 | 125.9721×105 |
Figure 6(a2) | 0.3078 | 0.0268 | 0.4592 | 2.1700×10−9 | 0.2390 | 14.9323×105 |
Figure 6(b1) | 0.0257 | 0.4352 | 0.0068 | 1.2132 | 0.9997 | 16.0828×105 |
Figure 6(b2) | 0.9032 | 0.0481 | 0.6979 | 0.2236 | 0.9867 | 4.1934×105 |
Figure 6(c1) | 0.0045 | 0.1619 | 0.0113 | 0.6268 | 0.9972 | 181.2324×105 |
Figure 6(c2) | 0.0155 | 0.3977 | 0.9945 | 0.4330 | 0.0036 | 19.6208×105 |
Figure 6(d1) | 0.0346 | 0.0275 | 4.0345×10−4 | 1.0443×10−5 | 0.4732 | 648.2643×105 |
Figure 6(d2) | 0.0110 | 0.0042 | 4.3398×10−6 | 2.3233×10−5 | 0.9729 | 15567×105 |
Figure 6(e1) | 0.0432 | 0.1485 | 0.0956 | 0.4879 | 0.9808 | 307.3187×105 |
Figure 6(e2) | 0.4196 | 0.5003 | 0.1059 | 0.8724 | 0.9337 | 94.0182×105 |
Figure 6(f1) | 0.0020 | 0.2662 | 0.1001 | 0.6349 | 0.7655 | 198.4130×105 |
Figure 6(f2) | 0.1086 | 0.7769 | 1.2098×10−13 | 0.0897 | 1.0000 | 8.9430×105 |
Figure 6(g1) | 0.0047 | 0.1183 | 0.0441 | 1.5000 | 0.9937 | 10.0017×105 |
Figure 6(g2) | 0.2737 | 0.4832 | 0.4094 | 0.1000 | 0.9925 | 116.2541×105 |
Name | Estimated Value | Standard Error | CI Low Bound | CI High Bound | p-value | t-statistic | Min | Max |
S0(*105) | 168.8749 | 8.7633 | 151.1021 | 186.6476 | 1.4913×10−20 | 19.2707 | 1.0000 | 2.0000×104 |
α | 0.9792 | 0.5558 | -0.1481 | 2.1064 | 0.0866 | 1.7617 | 0.0000 | 1.50000 |
β | 0.0179 | 0.0049 | 0.0080 | 0.0278 | 7.8418×10−4 | 3.6681 | 0.0000 | 1.0000 |
p | 0.5756 | 0.1799 | 0.2107 | 0.9405 | 0.0029 | 3.1990 | 0.0000 | 1.0000 |
q | 0.0017 | 0.0318 | -0.0628 | 0.0661 | 0.9588 | 0.0520 | 0.0000 | 1.0000 |
θ | 0.9776 | 0.0660 | 0.8439 | 1.1114 | 6.7306×10−17 | 14.8202 | 0.0000 | 1.0000 |
Data | 2019.12.31 | 2020.1.1 | 2020.1.2 | 2020.1.3 | 2020.1.4 | 2020.1.5 |
ℜ0 | 1.7769 | 1.7526 | 1.7042 | 1.6171 | 1.4849 | 1.3279 |
Data | 2020.1.6 | 2020.1.7 | 2020.1.8 | 2020.1.9 | 2020.1.10 | 2020.1.11 |
ℜ0 | 1.1878 | 1.0905 | 1.0340 | 1.0044 | 0.9895 | 0.9821 |
Data | 2020.1.12 | 2020.1.13 | 2020.1.14 | 2020.1.15 | 2020.1.16 | 2020.1.17 |
ℜ0 | 0.9785 | 0.9766 | 0.9758 | 0.9753 | 0.9750 | 0.9749 |
Data | 2020.1.18 | 2020.1.19 | 2020.1.20 | 2020.1.21 | 2020.1.22 | 2020.1.23 |
ℜ0 | 0.9748 | 0.9748 | 0.9747 | 0.9747 | 0.9748 | 0.9747 |
Data | 2020.1.24 | 2020.1.25 | 2020.1.26 | 2020.1.27 | 2020.1.28 | 2020.1.29 |
ℜ0 | 0.9747 | 0.9747 | 0.9747 | 0.9747 | 0.9747 | 0.9747 |
Data | 2020.1.30 | 2020.1.31 | 2020.2.1 | 2020.2.2 | 2020.2.3 | 2020.2.4 |
ℜ0 | 0.9747 | 0.9747 | 0.9747 | 0.9747 | 0.9747 | 0.9747 |
Data | 2020.2.5 | 2020.2.6 | 2020.2.7 | 2020.2.8 | 2020.2.9 | 2020.2.10 |
ℜ0 | 0.9748 | 0.9748 | 0.9748 | 0.9747 | 0.9747 | 0.9746 |
Data | 2020.2.11 | 2020.2.12 | 2020.2.13 | 2020.2.14 | 2020.2.15 | 2020.2.16 |
ℜ0 | 0.9746 | 0.9747 | 0.9747 | 0.9748 | 0.9748 | 0.9748 |
Data | 2020.2.17 | 2020.2.18 | 2020.2.19 | 2020.2.20 | 2020.2.21 | 2020.2.22 |
ℜ0 | 0.9748 | 0.9748 | 0.9747 | 0.9747 | 0.9747 | 0.9747 |
Data | 2020.2.23 | 2020.2.24 | 2020.2.25 | 2020.2.26 | 2020.2.27 | |
ℜ0 | 0.9747 | 0.9747 | 0.9747 | 0.9747 | 0.9747 |