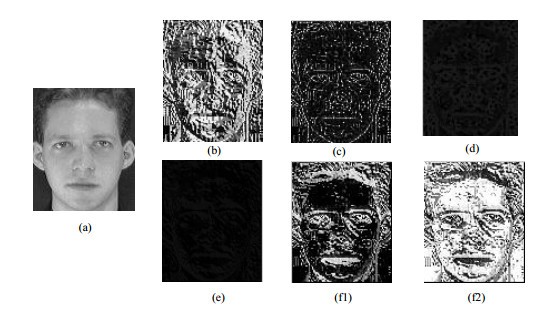
Citation: Qian Zhang, Haigang Li, Ming Li, Lei Ding. Feature extraction of face image based on LBP and 2-D Gabor wavelet transform[J]. Mathematical Biosciences and Engineering, 2020, 17(2): 1578-1592. doi: 10.3934/mbe.2020082
[1] | Zilong Liu, Jingbing Li, Jing Liu . Encrypted face recognition algorithm based on Ridgelet-DCT transform and THM chaos. Mathematical Biosciences and Engineering, 2022, 19(2): 1373-1387. doi: 10.3934/mbe.2022063 |
[2] | Xiaoguang Liu, Mingjin Zhang, Jiawei Wang, Xiaodong Wang, Tie Liang, Jun Li, Peng Xiong, Xiuling Liu . Gesture recognition of continuous wavelet transform and deep convolution attention network. Mathematical Biosciences and Engineering, 2023, 20(6): 11139-11154. doi: 10.3934/mbe.2023493 |
[3] | Song Yang, Huibin Wang, Hongmin Gao, Lili Zhang . Few-shot remote sensing scene classification based on multi subband deep feature fusion. Mathematical Biosciences and Engineering, 2023, 20(7): 12889-12907. doi: 10.3934/mbe.2023575 |
[4] | Guanghua Fu, Qingjuan Wei, Yongsheng Yang . Bearing fault diagnosis with parallel CNN and LSTM. Mathematical Biosciences and Engineering, 2024, 21(2): 2385-2406. doi: 10.3934/mbe.2024105 |
[5] | Li Wang, Changyuan Wang, Yu Zhang, Lina Gao . An integrated neural network model for eye-tracking during human-computer interaction. Mathematical Biosciences and Engineering, 2023, 20(8): 13974-13988. doi: 10.3934/mbe.2023622 |
[6] | Yong Tian, Tian Zhang, Qingchao Zhang, Yong Li, Zhaodong Wang . Feature fusion–based preprocessing for steel plate surface defect recognition. Mathematical Biosciences and Engineering, 2020, 17(5): 5672-5685. doi: 10.3934/mbe.2020305 |
[7] | Hao Chen, Shengjie Li, Xi Lu, Qiong Zhang, Jixining Zhu, Jiaxin Lu . Research on bearing fault diagnosis based on a multimodal method. Mathematical Biosciences and Engineering, 2024, 21(12): 7688-7706. doi: 10.3934/mbe.2024338 |
[8] | Ansheng Ye, Xiangbing Zhou, Kai Weng, Yu Gong, Fang Miao, Huimin Zhao . Image classification of hyperspectral remote sensing using semi-supervised learning algorithm. Mathematical Biosciences and Engineering, 2023, 20(6): 11502-11527. doi: 10.3934/mbe.2023510 |
[9] | Hong-an Li, Min Zhang, Zhenhua Yu, Zhanli Li, Na Li . An improved pix2pix model based on Gabor filter for robust color image rendering. Mathematical Biosciences and Engineering, 2022, 19(1): 86-101. doi: 10.3934/mbe.2022004 |
[10] | Viliam Ďuriš, Vladimir I. Semenov, Sergey G. Chumarov . Wavelets and digital filters designed and synthesized in the time and frequency domains. Mathematical Biosciences and Engineering, 2022, 19(3): 3056-3068. doi: 10.3934/mbe.2022141 |
As a specific application in biometric identification technologies, there have emerged a lot of algorithms for face recognition technology after nearly half a century of research. Chan and Bledsoe presented a research paper on automatic face recognition for the first time [1]. They hold that face recognition could be realized based on geometric structural features. In the 1990s, the research on automatic face recognition ushered in a new leaping development. Among numerous methods for face recognition, the face recognition based on facial features was undoubtedly the most representative [2]. The feature extraction from face image data was carried out by using discrete K-L transformation, also known as principal component analysis (PCA).
Since the beginning of this century, more in-depth studies on face recognition have been carried out. In order to improve the performance of face recognition algorithms, many scholars have done a fruitful work, which is summed up in the following areas:
a. Original face recognition algorithms have been improved. For example, in order to reduce the calculated amount of feature analysis required by PCA, Professor Yang et al. in Nanjing University of Science and Technology proposed 2-D PCA method, followed by (2D) 2PCA, MatPCA, G2D-PCA and UPCA later on; There have also been various improved versions for LDA method, such as 2D-LDA, NLDA, RKDA and NKDA [3].
b. New description of face image data has been searched. Image texture description has many methods such as local binary pattern(LBP)[4], gray level co-occurrence matrix, histogram of oriented gradients [5]; New descriptions can also be produced when some integral transformation is conducted on images. These transformations include Fourier transformation, Gabor wavelet transform, Harr wavelet transform, discrete cosine transform.
c. More innovative face recognition methods have been proposed; Such innovative face recognition methods include: Face recognition based on support vector machine [6], face detection and recognition based on Adaboost algorithm [7,8], face recognition based on manifold learning [9], face recognition based on compressed sensing [10].
d. Face recognition algorithms have been integrated and optimized [11]. Currently, it is very difficult to achieve better recognition performance only relying on a certain kind of approach for face recognition. The optimization and combination of different descriptions, different feature extractions, and different classifiers on face images will achieve face recognition algorithms with higher recognition rate and better robustness.
Under the influence of light, gesture and facial expression, the performance of face recognition algorithms are generally affected to a certain extent. For how to effectively reduce the impact of various factors on the performance of face recognition algorithms, combined with LBP and 2-D Gabor wavelet transform, this paper described the features of face image, and carried on tests on the performance of face recognition described by LBP and 2-D Gabor wavelet in different scales and directions. Through synthesizing and analyzing the experimental results, this paper proposed a method of extracting local and global features from face image.
LBP was first proposed by Ojala et al.. It is a nonparametric operator which describes local image structure. The simplest LBP made a binary encoding on the pixel gray difference between center pixel and the pixel within its 8-neighborhood, then converted it into a decimal number which was used for the expression of texture feature of center pixel. Such description was invariable to the overall change of gray level in images. In order to eliminate the pattern change caused by image rotation, Ojala et al. further presented a new definition for rotation invariant local binary pattern. In addition to this, Marko et al. put forward center-symmetric local binary pattern [12], Xu et al. proposed dual local binary pattern, Tan et al. proposed local triple pattern. In 2004, Ahonen et al. first described face images by using LBP description operator and used the techniques such as histogram statistic and chi-square statistical measurement for face recognition. Experiments showed that this method could effectively eliminate the impact of factors such as illumination on the performance of face recognition [13,14].
Image texture feature generally means the characteristics of each pixel reflecting within its neighborhood, so the pixel neighborhood must first be defined generally when describing image texture features. The neighborhood used by LBP to describe an image is an annular area which can be written as (P,R), wherein, R is the radius of annular neighborhood, P is the pixels which are uniformly selected in annular neighborhood [15].
The feature information of any pixel in an image is determined by a combination of pixel itself and its neighborhood. The feature information of each pixel can be measured with joint probability distribution T(gc). T(gc) is defined as shown in formula (1):
T(gc)=t(gc,g0,...,gp−1) | (1) |
Where, gc is the gray value of central pixel, {gi|i=0,…,P−1} is the gray value of pixel on annular neighborhood. A new feature measure can be achieved when subtracting the gray value of center pixel from the gray value of each pixel within the neighborhood. Suppose any {(gi−gc)|i=0,1,…,P−1} is independent of gc, it is defined as follows:
ˉT=ˉt(gc)ˉt(g0−gc,g1−gc,…,gP−1−gc) | (2) |
Where, ˉt(gc) represents the grey information of image, ˉt(g0−gc,g1−gc,⋯,gP−1−gc) represents the texture information of image. Under the influence of illumination variation, ˉt(gc) generally changes greatly, while {gi−gc|i=0,1,⋯,P−1} is also affected by illumination variation to some extent. If ˉt(gc) is abandoned, the measure of gray difference {gi−gc|i=0,1,⋯,P−1} between the pixel within annular neighborhood and center pixel is transformed into a new measure {s(gi−gc)|i=0,1,⋯,P−1} which is not sensitive to light, the texture feature information which is not sensitive to illumination variation at any one pixel in the image can be further defined as formula (3):
ˆT(gc)=ˉt(s(g0−gc),s(g1−gc),…,s(gp−1−gc)) | (3) |
The values of {s(gi−gc)|i=0,1,…,P−1} generally do not change in the case of uniform illumination variation. LBP pattern is defined as follows:
LBPP,R=P−1∑i=0s(gi−gc)⋅2i | (4) |
The facial texture feature information can be effectively extracted by using local binary pattern operator to describe face image. Figure 1 shows the face description images by means of the operators such as traditional local binary pattern (LBP8,1(b)), rotation invariant local binary pattern (LBPri8,1(c)), consistent local binary pattern (LBPriu224,3(d)), center-symmetric local binary pattern (CS_LBP16,2(e)) and dual local binary pattern (DLBP8,1(f1, f2)).
In 1946, Dennis Gabor discovered the uncertainty principle of one-dimensional signal and provided the original form of Gabor function [16]. He pointed out that any complex sinusoidal signal modulated by Gaussian function could reach the lower limit of combined uncertainty relations in time domain and frequency domain. In 1980, Daugman proposed a two-dimensional Gabor (2-D Gabor) filter function and used it for image characterization [17]. In recent years, researchers have used 2-D Gabor filters for face image description and also for face recognition resulting in a lot of new research achievements.
The new image description obtained from image filtering by using 2-D Gabor filters in different scales and directions can effectively reduce the impact of the changes of illumination, facial expression and gesture on face recognition accuracy. 2-D Gabor filter is defined as follows:
ψu,v(x)=||ku,v||2σ2e−||ku,v||2||x||2σ2[ej(ku,v⋅x)−e−σ22] | (5) |
Where, σ reflects the size of Gaussian window, x=(x,y) is the coordinate location of a given image pixel, ku,v=kv(cos(ϕu),sin(ϕu)) is the center frequency of filter. Here, kv=kmax/fv, kmax is the maximum frequency, v determines the wavelength of 2-D Gabor wavelet; ϕu=πu/K is the direction of 2-D Gabor wavelet function. Figure 2a, b shows the real part and imaginary part of a set of 2-D Gabor filter functions. Figure 2c shows the amplitude values of a set of 2-D Gabor filter functions, wherein the preferences are: kmax=π/2,f=√2,σ=√2π,u=0,1,…,7,v=0,1,…,4.
Different image characterizations can be obtained from image filtering by using 2-D Gabor filters in different scales and directions. Each description can be calculated by formula (6):
Ou,v(x)=I(x)∗ψu,v(x) | (6) |
Where, * represents convolution operation.
Figure 3 shows the filter output and amplitude output at real and imaginary parts of 2-D Gabor filter for face image. It is thus clear that the new images obtained from image filtering by using 2-D Gabor filter can not only carry out effective global description but also effectively extract the detail features of images. Relative to single original grayscale image, the features at different levels of image can be extracted through 2-D Gabor filter. These levels of features can be separated for face classification [15].
Suppose that face image can be represented by I, the steps for face recognition by using simplest LBP pattern LBP8,1 are as follows:
a. Use LBP8,1 to describe face image I for a texture description I_LBP8,1 on face image. Figure 4b shows LBP description I_LBP8,1 in Figure 3a.
b. Divide I_LBP8,1 into several pieces (I_LBP8,1 in Figure 4b is divided into 3 × 3, nine pieces). Use histograms to count all pieces to obtain a histogram description I_LBP_histi of each small piece, and connect the histograms to form a new histogram description of face image I_LBP_Hist=[I_LBP_hist1,I_LBP_hist2,...,I_LBP_histn]. Figure 5 shows the histograms of each small piece and the intuitive connection of histograms of each small piece.
c. I_LBP_Hist is a vector expression for original face image I. However, the dimension of I_LBP_Hist is generally higher. For the partition mode as shown above. The dimension of I_LBP_Hist reaches up to 256×9=2304. Such high-dimensional data are not conducive to classification and recognition, especially in small samples. Direct at solving the problem, I_LBP_Hist is generally treated with dimension reduction, then classified and identified. The commonly used methods of dimension reduction include PCA, independent component analysis (ICA) and so on. Figure 6b shows the performance for the face database of ORL was treated with dimension reduction by using PCA method, and the face recognition was conducted by using NN (Nearest Neighbor) of Euclidean distance metric; Figure 6a shows the performance for the face images in ORL face database were first treated with LBP8,1 description, then described with histograms, done with dimension reduction by PCA method, and the face recognition was conducted by using NN of Euclidean distance metric. Each person had four face images involving in the training, and the remaining six images were used for testing. Compare Figure 6a with Figure 6b, it shows that the method of LBP + PCA has achieved better recognition effect.
Similarly, we compare the number of recognition samples and the average time consumed by different methods, and the results are shown in Table 1.
Methods | Number of training samples | Number of test samples | Number of identified samples(rank1) | Number of identified samples(rank10) | Average time consumed(s) |
LBP | 40 | 40 | 28 | 34 | 0.76 |
PCA | 40 | 40 | 32 | 37 | 0.89 |
LBP+PCA | 40 | 40 | 33 | 39 | 0.61 |
Table 1 shows the first hit recognition rate value (rank1) of three different algorithms and the tenth hit recognition rate value (rank10) when the final recognition rate tends to be stable after many training, as well as the time-consuming. The experimental data of the table show that with the increase of training times, the recognition rates of the three algorithms are improved. The recognition rates of LBP algorithm are low, while PCA algorithm takes a long time because of the high dimension of feature vectors. In this paper, the algorithm first uses LBP to process the histogram of the encoding image and then uses PCA to reduce the dimension of the extracted features, which greatly improves the recognition rate while reducing the computational complexity and improving the recognition speed.
Gabor transform not only has an astonishing similarity to the human retinal cell receiving field model, but also obtains the minimum uncertainty of frequency and time, and has strong robustness to external disturbances such as illumination, posture, expression and so on. The traditional face feature extraction method based on Gabor transform firstly uses convoluted face images and Gabor multi-direction and multi-scale kernels, and then cascades and down sampling to obtain Gabor face features. However, this method can get a higher dimension, which takes a lot of time and has no rotation invariance. When the face image rotates in the plane, the recognition performance decreases. LBP is an operator for texture analysis. It has many advantages such as simple algorithm, not too complicated calculation process, strong discrimination ability and so on. It has been widely studied and used in recent years. LBP operator has strong gray scale invariance and rotation invariance characteristics, which can reduce the influence of rotation shift and illumination unevenness.
Therefore, this paper uses 2-D Gabor wavelet to extract the original image features, including multi-angle feature extraction and multi-scale feature extraction, in order to effectively reduce the interference of illumination and rotation on image extraction. The obtained image is encoded and histogram is constructed by LBP algorithm, so that the image details are adequately information. Then PCA method is used to reduce the image dimension. It's named GBLBP here.
From the feature selection method, dimension reduction method and classifier introduced above, the corresponding algorithm is obtained.
Step 1: According to (6), 40 subetaaphs of face image I are obtained by Gabor wavelet transform, in which μ∈{0, 1, 2, 3, 4, 5, 6, 7} and V∈{0, 1, 2, 3, 4};
Step 2: All subetaaphs are divided into M blocks R0, R1, …, Rm − 1;
Step 3: For the local points (a, b) in each specific region Rk, according to (4), take the GLLBP feature with the scale ν0 and the direction µc as the center, and get the corresponding histogram I_LBP_histi;
Step 4: The final feature representation vector I_LBP_Hist of I is obtained by integrating each feature;
Step 5: Dimension reduction according to PCA;
Step 6: Perform steps 1–5 for all training samples;
Step 7: For each test sample I′, get the eigenvector I′_LBP_Hist of the test sample according to steps 1–4;
Step 8: The dimension of I′_LBP_Hist is reduced according to the dimension reduction matrix of PCA;
Step 9: Get test sample label by NN classifier.
Since face description in each scale and direction contains some facial feature information, this paper carried on the face recognition performance test on each description separately, and investigated the face recognition performance of 2-D Gabor wavelet description in different scales and directions. This paper carried on test on ORL face database by using PCA + NN respectively. Figure 7a shows the output result of the input face image at real part of small-scale 2-D Gabor wavelet filter; Figure 7b shows the output of the input face images at real part of large-scale 2-D Gabor wavelet filter.
The experiment showed that the recognition performance was poor when the wavelet scale of 2-D Gabor wavelet filter was smaller; when the wavelet scale was larger, the recognition performance of new description became better. This paper carried on the test on the face description in both scale and direction by using PCA + NN respectively. The variation diagrams in which face recognition rate increased with the number of features are shown in Figure 8a, b.
As can be seen from the two pictures in Figure 8, large-scale 2-D Gabor wavelet filter could be better used for the classification and identification of face image. Actually, the description of face image by using small-scale 2-D Gabor wavelet filter reflected the high frequency in the image, corresponding to the local feature information, including contour information of each organ on human face, but also the noise information; while the description of face image by using large-scale 2-D Gabor wavelet filter reflected the low frequency in the image, corresponding to the global feature information.
Another experiment was conducted in the paper. The face descriptions in the same scale but different directions were set as a group, and the face descriptions of the same group in different directions were superimposed each other to form a face description of 2-D Gabor wavelet in a certain scale. Figure 9 shows a number of face description of 2-D Gabor wavelet in different scales about the same face, wherein, Figure 9a corresponded to the smallest wavelet scale, Figure 9e corresponded to the maximum wavelet scale. The classification and recognition performance test on face descriptions corresponding to scales in Figure 9a, e by using PCA + NN were conducted respectively. The test results are shown in Figure 10a, b; The classification and recognition performance test on face descriptions corresponding to scales in Figure 9a, e by using LBP + PCA + NN were conducted respectively. The test results are shown in Figure 10c, d.
As can be seen from the comparison of Figure 10a, c, for the face description of 2-D Gabor wavelet in small scale, the texture description was first carried on by using LBP, then it was described with blocks, histograms, PCA feature extraction, which could get better recognition performance compared to PCA feature extraction directly from the data via 2-D Gabor wavelet transform. This is due to the use of PCA for feature extraction, wherein the corresponding features were the low frequency information of the data; while the face description of 2-D Gabor wavelet in small scale corresponded to the contour information of face organ, i.e. high frequency; In the case of face description of 2-D Gabor wavelet in small scale, we hoped to take advantage of our face contour information, namely high-frequency to recognize faces. So the performance of feature extraction directly from the data via 2-D Gabor wavelet transform by using PCA as well as classification and identification was poor; First, the expression of texture pattern about the converted data through LBP could extract the contour information of face image data, and secondly a new vector expression of face image data was formed through the description of blocks and histograms. The vector expression still retained sufficient contour information, so it was reasonable to obtain higher recognition performance for feature extraction and recognition through PCA.
As can be seen from Figure 10b, d, for the face description of 2-D Gabor wavelet in large scale, the performance of face classification and recognition by direct use of "PCA + NN" and use of "LBP + PCA + NN " were all good. This is because that the face description of 2-D Gabor wavelet in large scale corresponded to the information of low frequency in the original face image. The corresponding extraction features using PCA were the information of low frequency about the data. However, the face description of large-scale 2-D Gabor wavelet did not lose too much low-frequency information in face images, so the direct use of "PCA + NN" could achieve better recognition performance for the face description of large-scale 2-D Gabor wavelet; while the use of "LBP + PCA + NN " could also achieve better recognition performance, for the contour information of large-scale 2-D Gabor wavelet description was extracted for face recognition.
Thus an method for extracting global and local features can be drawn in this paper: (1) Global feature extraction–large-scale 2-D Gabor wavelet description on face images, data dimension reduction with principal component analytical method, thereby obtaining effective global features; (2) Local feature extraction—different-scale 2-D Gabor wavelet description on face images, then use LBP for texture description, then image block, histogram description, PCA data dimension reduction, thereby obtaining effective local features of face images.
In order to better reflect the recognition rate comparison between LBP and 2-D Gabor expression recognition algorithm and 2D-DCT, CBP, PCA algorithm to be compared. Ten-fold cross validation method was used to test the accuracy of the algorithm. The data set was divided into ten parts, nine of which were used as training data and one as test data in turn. The corresponding accuracy will be obtained in each test. The average accuracy of the results of 10 times is used to estimate the accuracy of the algorithm. For the facial expression database, the recognition results are shown in Table 2.
Algorithm | Set 1 | Set 2 | Set 3 | Set 4 | Set 5 | Set 6 | Set 7 | Set 8 | Set 9 | Set 10 | Average |
2D-DCT | 65.27 | 58.43 | 68.20 | 72.14 | 64.04 | 63.16 | 72.08 | 74.31 | 68.12 | 66.35 | 67.21 |
CBP | 48.16 | 68.13 | 62.01 | 61.13 | 59.38 | 62.36 | 66.27 | 72.01 | 70.27 | 81.82 | 65.15 |
PCA | 57.93 | 70.84 | 69.48 | 64.36 | 67.38 | 64.53 | 71.57 | 74.68 | 77.56 | 83.68 | 70.21 |
GBLBP | 76.29 | 68.45 | 65.76 | 68.86 | 72.47 | 64.35 | 68.96 | 71.97 | 70.45 | 78.53 | 70.60 |
Experimental results show that the GBLBP algorithm has a higher recognition rate in facial expression recognition than other commonly used algorithms.
In order to have objective and scientific comparison results, hypothesis testing is used on the experimental results. Let the variable X1,X2,X3,X4 denote the classification error rate of algorithms proposed in this paper, Gabor, LBP and PCA, respectively. Since the value of X1,X2,X3,X4 is subject to many random factors, we assume that they submit to normal distribution, Xi∼N(μi,σ2i), i=1,2,3,4. Now, we compare the random variable mean of these algorithms, μi (i=1,2,3,4). The smaller the μi is, the lower the expected classification error rate is, and the higher the efficiency is. Because the sample variance is the unbiased estimation of the overall variance, the sample variance value is used as an estimate of the generality variance. In this experiment the significance level α sets as 0.01.
Table 3 shows the comparison process on μi and other parameters. We can see from Table 1 that, the expectations of classification error rate in this paper is far below than other algorithms.
Hypothesis | H0:μ1⩾μ2H1:μ1<μ2 | H0:μ1⩾μ3H1:μ1<μ3 | H0:μ1⩾μ4H1:μ1<μ4 |
Statistics | U1=ˉX1−ˉX2√σ21n1+σ22n2 | U2=ˉX1−ˉX3√σ21n1+σ23n3 | U3=ˉX1−ˉX4√σ21n1+σ24n4 |
Rejection region | U1⩽−Zα=−2.325 | U2⩽−Zα=−2.325 | U3⩽−Zα=−2.325 |
Value of the statistic | U1=−34.75 | U2=−42.28 | U3=−53.26 |
Conclusion | H1:μ1<μ2 | H1:μ1<μ3 | H1:μ1<μ4 |
In order to verify the feasibility of this algorithm, this section compares the algorithm with other common algorithms under the same hardware and software conditions, in which LBP, Gabor and GBLBP use PCA to reduce dimension. In the experiment, Gabor selected 5 scales, 8 directions and 35 × 35 window size. Face image block mode is 5 × 3 and dimension is reduced to 75. ORL face database contains 400 samples, a total of 40 groups, each group of 10 images, and each group is the same person under different postures, expressions of images, size 112*92. In ORL face database, the training set sample images are randomly extracted for experiment. When the sample data are 80 to 320, 30 experiments are carried out and their mean values are calculated. If the number of samples is 360, the recognition rate can be obtained by ranking method, and the CPU running time of different algorithms under different number of training samples can be calculated by ranking method. The experimental results are shown in Tables 4 and 5.
Differentmethods | Recognition rate (%) | ||||||||
80 | 120 | 160 | 200 | 240 | 280 | 320 | 360 | Average value | |
Gabor | 81.95 | 85.54 | 90.14 | 92.42 | 94.18 | 95.35 | 96.15 | 96.49 | 91.53 |
LBP | 79.38 | 82.02 | 87.53 | 90.13 | 91.26 | 92.46 | 93.64 | 94.17 | 88.82 |
PCA | 77.63 | 79.86 | 83.56 | 87.69 | 88.78 | 92.18 | 92.09 | 92.68 | 86.81 |
GBLBP | 85.72 | 90.31 | 92.34 | 95.24 | 97.36 | 97.67 | 97.86 | 98.74 | 94.41 |
Differentmethods | Recognition time(s) | |||||||
80 | 120 | 160 | 200 | 240 | 280 | 320 | 360 | |
Gabor | 0.812 | 0.956 | 1.058 | 1.127 | 1.312 | 1.451 | 1.693 | 1.902 |
LBP | 0.564 | 0.646 | 0.759 | 0.877 | 0.991 | 1.128 | 1.320 | 1.659 |
PCA | 0.407 | 0.498 | 0.596 | 0.693 | 0.829 | 0.945 | 1.192 | 1.438 |
GBLBP | 1.051 | 1.192 | 1.316 | 1.474 | 1.637 | 1.781 | 1.879 | 2.120 |
Compared with ORL face database, Table 4 shows that the proposed algorithm has higher recognition rate than Gabor and LBP only, and the average recognition rate of PCA is the lowest. Because the proposed algorithm is compatible with the advantages of two algorithms: 2-D Gabor wavelet can extract multi-directional and multi-scale spatial frequency features of local images; LBP algorithm can represent the details of adjacent image regions, and extract more comprehensive and effective feature information. Compared with 2-D Gabor transform and LBP method, the algorithm used in this paper is better. The PCA-based feature extraction method is to extract the overall features of face images from the overall contour of face images. It cannot effectively describe the local details of face images, and cannot represent the relationship between regions. In the process of dimension reduction, it is easy to ignore some effective feature information. The recognition rate is the lowest.
From Table 5, it can be seen that the algorithm in this paper has high computational complexity and takes longer time to recognize a single image than the other three methods. If the size of a face image is 112 × 92, 40 images of the same size will be generated after Gabor transformation, and then after LBP, the dimension will increase many times. Therefore, the recognition of a face image will be done. It takes longer than the other three feature extraction methods, but in terms of recognition rate, it is more effective than the other three feature extraction algorithms. For face recognition, correct recognition is the key, so the algorithm in this paper is more feasible.
This paper described face images in different scales and directions by using 2-D Gabor wavelet, and extracted features from various descriptions respectively combined with PCA method. The recognition performance tests on face description in various scales and directions were separately conducted by using NN classifier. The experiment results showed that higher classification accuracy could be obtained for large-scale face image description was used for separately pattern classification; then 2-D Gabor wavelet descriptions of face images in various scales and directions were treated with LBP description, and classified with blocks, histograms, PCA data dimension reduction and NN. The experiment results showed that this algorithm could obtain a relatively good recognition performance of 2-D Gabor wavelet description in a variety of scales and describe. Finally, this paper proposed a method of extracting global and local features from face images based on LBP and Gabor wavelet transform through the analysis of experimental data.
This work was jointly supported by National Natural Science Fund (NO. 61876185) and the Fundamental Research Funds for Central Universities (NO. 2015QNB21).
We declare that we do not have any commercial or associative interest that represents a conflict of interest in connection with the work submitted.
[1] | R. Alessandro, B. Marco, B. Alessio, F. Marcellonia, Comparing ensemble strategies for deep learning: An application to facial expression recognition, Expert. Syst. Appl., 136 (2019), 1-11. |
[2] | M. Q. Mei, J. Z. Huang, W. W. Xiong, A discriminant subspace learning based face recognition method, IEEE Access, 6 (2017), 13050-13056. |
[3] | R. Senthilkumar, R. K. Gnanamurthy, A comparative study of 2D PCA face recognition method with other statistically based face recognition methods, J. Inst. Eng., 97 (2016), 425-430. |
[4] | S. F. Liang, Y. H. Liu, L. C. Li, Face recognition under unconstrained based on LBP and deep learning, J. Commun., 35 (2014), 154-160. |
[5] | J. Bobulski, Multimodal face recognition method with two-dimensional hidden Markov model, Bull. Pol. Acad. Sci. Tech. Sci., 65 (2017), 121-128. |
[6] | A. Abaza, M. A. Harrison, T. Bourlai, A. Ross, Design and evaluation of photometric image quality measures for effective face recognition, IET Biom., 3 (2014), 314-324. |
[7] | W. T. Fang, P. Ma, Z. B. Cheng, 2-Dimensional projective non-negative matrix factorization and its application to face recognition, Acta Autom. Sin., 38 (2012), 1503-1512. |
[8] | Q. Zhang, M. Li, X. S. Wang, Y. H. Cheng, M. Q. Zhu, Instance-based transfer learning for multi-source domains, Acta Autom. Sin., 40 (2014), 1176-1183. |
[9] | Z. Chen, W. Shen, Y. M. Zeng, Sparse representation for pose invariant face recognition, Int. J. Eng. Modell., 30 (2017), 37-47. |
[10] | A. Adler, M. E. Schuckers, Comparing human and automatic face recognition performance, IEEE Trans. Syst. Man Cybern. Part B, 37 (2007), 1248-1255. |
[11] | M. M. Liao, X. D. Gu, Face recognition based on dictionary learning and subspace learning, Digital Signal Process., 90 (2019) 110-124. |
[12] | W. K. Xu, E. J. Lee, A hybrid method based on dynamic compensatory fuzzy neural network algorithm for face recognition, Int. J. Control Autom., 12 (2014), 688-696. |
[13] | K. K. Sang, J. P. Young, K. A. Toh, S. Y. Lee, SVM-based feature extraction for face recognition, Pattern Recognit., 43 (2010), 2871-2881. |
[14] | H. G. Li, Q. Zhang, C. D. Li, An effective hand vein feature extraction method, Technol. Health Care, 23 (2015), 343-353. |
[15] | D. Sadhya, S. K. Singh, A comprehensive survey of unimodal facial databases in 2D and 3D domains, Neurocomputing, 358 (2019), 188-210. |
[16] | J. W. Lu, V. E. Liong, X. Z. Zhou, Learning compact binary face descriptor for face recognition, IEEE Trans. Pattern Anal. Mach. Intell., 37 (2015), 2041-2056. |
[17] | Y. F. Li, Z. Y. Lu, J. Li, Y. Deng, Improving deep learning feature with facial texture feature for face recognition, Wireless Pers. Commun., 103 (2018), 1195-1206. |
1. | Yaolin Zhu, Jiayi Huang, Tong Wu, Xueqin Ren, Identification method of cashmere and wool based on texture features of GLCM and Gabor, 2021, 16, 1558-9250, 155892502198917, 10.1177/1558925021989179 | |
2. | M. Magdin, J. Kapusta, S. Koprda, Investigation of Behavioral Characteristic Parameters Intended for Emotional State Classification, 2022, 10, 2169-3536, 129029, 10.1109/ACCESS.2022.3227627 | |
3. | Hangzhou Qu, Yinwei Wang, Application of Optimized Local Binary Pattern Algorithm in Small Pose Face Recognition under Machine Vision, 2022, 81, 1380-7501, 29367, 10.1007/s11042-021-11809-9 | |
4. | Xiliang Xiao, Jingbing Li, Dan Yi, Yangxiu Fang, Wenfeng Cui, Uzair Aslam Bhatti, Baoru Han, 2021, Chapter 7, 978-981-16-3012-5, 75, 10.1007/978-981-16-3013-2_7 | |
5. | Jing Xue, Balakrishnan Nagaraj, EEG Analysis with Wavelet Transform under Music Perception Stimulation, 2021, 2021, 2040-2309, 1, 10.1155/2021/9725762 | |
6. | Dongmei Shi, Hongyu Tang, Face recognition algorithm based on self-adaptive blocking local binary pattern, 2021, 80, 1380-7501, 23899, 10.1007/s11042-021-10825-z | |
7. | Sujuan Zhou, Huajian Lin, Jiang Meng, Discrimination and chemical composition quantitative model of Raw Moutan Cortex and Moutan Cortex Carbon based on electronic nose and machine learning, 2022, 19, 1551-0018, 9079, 10.3934/mbe.2022422 | |
8. | Hao Wang, Guangmin Sun, Kun Zheng, Hui Li, Jie Liu, Yu Bai, Privacy protection generalization with adversarial fusion, 2022, 19, 1551-0018, 7314, 10.3934/mbe.2022345 | |
9. | Yanjing Lu, Mudassir Khan, Mohd Dilshad Ansari, Face recognition algorithm based on stack denoising and self-encoding LBP, 2022, 31, 2191-026X, 501, 10.1515/jisys-2022-0011 | |
10. | Huan Ding, Qirui Huang, Dragan Rodriguez, Modified Locust Swarm optimizer for oral cancer diagnosis, 2023, 83, 17468094, 104645, 10.1016/j.bspc.2023.104645 | |
11. | Xingli Liu, Jian Guo, Hengyu Mu, Shuxuan Wang, Yiwen Tai, Chong Han, 2022, Multimodal recognition method based on fingerprint and finger vein, 978-988-75815-3-6, 6829, 10.23919/CCC55666.2022.9902490 | |
12. | Yongsheng Ding, Yunbo Wei, Shuisheng Zhang, Shihang Yu, Yuvaraja Teekaraman, Reversible Information of Encrypted Image Based on Feature Difference Detection and Wavelet Transform, 2021, 2021, 1555-4317, 1, 10.1155/2021/5483001 | |
13. | Khadidja Sadeddine, Fatma Zohra Chelali, Rachida Djeradi, Amar Djeradi, Sidahmed Benabderrahmane, Recognition of user-dependent and independent static hand gestures: Application to sign language, 2021, 79, 10473203, 103193, 10.1016/j.jvcir.2021.103193 | |
14. | Yaolin Zhu, Ran Liu, Gang Hu, Xin Chen, Wenya Li, Accurate identification of cashmere and wool fibers based on enhanced ShuffleNetV2 and transfer learning, 2023, 10, 2196-1115, 10.1186/s40537-023-00830-4 | |
15. | Zhiqiang Li, Gabor wavelet transform combined with area CNN in appearance intelligent detection of stayed cables, 2023, 25, 1392-8716, 1465, 10.21595/jve.2023.23231 | |
16. | Donghui Li, Wei Yuan, Xu Chen, Xuehui Guan, Dayu Liu, Kannimuthu Subramaniam, Pavel Loskot, 2023, Research and implementation of image edge detection based on Db4 wavelet transform, 9781510668522, 129, 10.1117/12.3006021 | |
17. | Tao Shen, Man Zhang, Ting Wu, Emotion-driven action interaction design in small quadruped robots: leveraging NXEIK multilevel network, 2024, 35, 0954-4828, 159, 10.1080/09544828.2023.2301231 | |
18. | Zhengping Gao, Fansheng Kong, Jiagui Tao, Min Chen, FACE RECOGNITION ALGORITHM BASED ON OPTIMAL WEIGHTED MULTI-DIRECTIONAL LOG-GABOR FEATURES , 2023, 30, 1065-3090, 97, 10.1615/JFlowVisImageProc.2023046834 | |
19. | Eswar Sai Bokkasam, Blessy Manogna Danavulapadu, Dinesh Lakshmaiah, Jona Rufus Enrathi, Sudhakar Kothandapani, 2025, 3281, 0094-243X, 020019, 10.1063/5.0247025 |
Methods | Number of training samples | Number of test samples | Number of identified samples(rank1) | Number of identified samples(rank10) | Average time consumed(s) |
LBP | 40 | 40 | 28 | 34 | 0.76 |
PCA | 40 | 40 | 32 | 37 | 0.89 |
LBP+PCA | 40 | 40 | 33 | 39 | 0.61 |
Algorithm | Set 1 | Set 2 | Set 3 | Set 4 | Set 5 | Set 6 | Set 7 | Set 8 | Set 9 | Set 10 | Average |
2D-DCT | 65.27 | 58.43 | 68.20 | 72.14 | 64.04 | 63.16 | 72.08 | 74.31 | 68.12 | 66.35 | 67.21 |
CBP | 48.16 | 68.13 | 62.01 | 61.13 | 59.38 | 62.36 | 66.27 | 72.01 | 70.27 | 81.82 | 65.15 |
PCA | 57.93 | 70.84 | 69.48 | 64.36 | 67.38 | 64.53 | 71.57 | 74.68 | 77.56 | 83.68 | 70.21 |
GBLBP | 76.29 | 68.45 | 65.76 | 68.86 | 72.47 | 64.35 | 68.96 | 71.97 | 70.45 | 78.53 | 70.60 |
Hypothesis | H0:μ1⩾μ2H1:μ1<μ2 | H0:μ1⩾μ3H1:μ1<μ3 | H0:μ1⩾μ4H1:μ1<μ4 |
Statistics | U1=ˉX1−ˉX2√σ21n1+σ22n2 | U2=ˉX1−ˉX3√σ21n1+σ23n3 | U3=ˉX1−ˉX4√σ21n1+σ24n4 |
Rejection region | U1⩽−Zα=−2.325 | U2⩽−Zα=−2.325 | U3⩽−Zα=−2.325 |
Value of the statistic | U1=−34.75 | U2=−42.28 | U3=−53.26 |
Conclusion | H1:μ1<μ2 | H1:μ1<μ3 | H1:μ1<μ4 |
Differentmethods | Recognition rate (%) | ||||||||
80 | 120 | 160 | 200 | 240 | 280 | 320 | 360 | Average value | |
Gabor | 81.95 | 85.54 | 90.14 | 92.42 | 94.18 | 95.35 | 96.15 | 96.49 | 91.53 |
LBP | 79.38 | 82.02 | 87.53 | 90.13 | 91.26 | 92.46 | 93.64 | 94.17 | 88.82 |
PCA | 77.63 | 79.86 | 83.56 | 87.69 | 88.78 | 92.18 | 92.09 | 92.68 | 86.81 |
GBLBP | 85.72 | 90.31 | 92.34 | 95.24 | 97.36 | 97.67 | 97.86 | 98.74 | 94.41 |
Differentmethods | Recognition time(s) | |||||||
80 | 120 | 160 | 200 | 240 | 280 | 320 | 360 | |
Gabor | 0.812 | 0.956 | 1.058 | 1.127 | 1.312 | 1.451 | 1.693 | 1.902 |
LBP | 0.564 | 0.646 | 0.759 | 0.877 | 0.991 | 1.128 | 1.320 | 1.659 |
PCA | 0.407 | 0.498 | 0.596 | 0.693 | 0.829 | 0.945 | 1.192 | 1.438 |
GBLBP | 1.051 | 1.192 | 1.316 | 1.474 | 1.637 | 1.781 | 1.879 | 2.120 |
Methods | Number of training samples | Number of test samples | Number of identified samples(rank1) | Number of identified samples(rank10) | Average time consumed(s) |
LBP | 40 | 40 | 28 | 34 | 0.76 |
PCA | 40 | 40 | 32 | 37 | 0.89 |
LBP+PCA | 40 | 40 | 33 | 39 | 0.61 |
Algorithm | Set 1 | Set 2 | Set 3 | Set 4 | Set 5 | Set 6 | Set 7 | Set 8 | Set 9 | Set 10 | Average |
2D-DCT | 65.27 | 58.43 | 68.20 | 72.14 | 64.04 | 63.16 | 72.08 | 74.31 | 68.12 | 66.35 | 67.21 |
CBP | 48.16 | 68.13 | 62.01 | 61.13 | 59.38 | 62.36 | 66.27 | 72.01 | 70.27 | 81.82 | 65.15 |
PCA | 57.93 | 70.84 | 69.48 | 64.36 | 67.38 | 64.53 | 71.57 | 74.68 | 77.56 | 83.68 | 70.21 |
GBLBP | 76.29 | 68.45 | 65.76 | 68.86 | 72.47 | 64.35 | 68.96 | 71.97 | 70.45 | 78.53 | 70.60 |
Hypothesis | H0:μ1⩾μ2H1:μ1<μ2 | H0:μ1⩾μ3H1:μ1<μ3 | H0:μ1⩾μ4H1:μ1<μ4 |
Statistics | U1=ˉX1−ˉX2√σ21n1+σ22n2 | U2=ˉX1−ˉX3√σ21n1+σ23n3 | U3=ˉX1−ˉX4√σ21n1+σ24n4 |
Rejection region | U1⩽−Zα=−2.325 | U2⩽−Zα=−2.325 | U3⩽−Zα=−2.325 |
Value of the statistic | U1=−34.75 | U2=−42.28 | U3=−53.26 |
Conclusion | H1:μ1<μ2 | H1:μ1<μ3 | H1:μ1<μ4 |
Differentmethods | Recognition rate (%) | ||||||||
80 | 120 | 160 | 200 | 240 | 280 | 320 | 360 | Average value | |
Gabor | 81.95 | 85.54 | 90.14 | 92.42 | 94.18 | 95.35 | 96.15 | 96.49 | 91.53 |
LBP | 79.38 | 82.02 | 87.53 | 90.13 | 91.26 | 92.46 | 93.64 | 94.17 | 88.82 |
PCA | 77.63 | 79.86 | 83.56 | 87.69 | 88.78 | 92.18 | 92.09 | 92.68 | 86.81 |
GBLBP | 85.72 | 90.31 | 92.34 | 95.24 | 97.36 | 97.67 | 97.86 | 98.74 | 94.41 |
Differentmethods | Recognition time(s) | |||||||
80 | 120 | 160 | 200 | 240 | 280 | 320 | 360 | |
Gabor | 0.812 | 0.956 | 1.058 | 1.127 | 1.312 | 1.451 | 1.693 | 1.902 |
LBP | 0.564 | 0.646 | 0.759 | 0.877 | 0.991 | 1.128 | 1.320 | 1.659 |
PCA | 0.407 | 0.498 | 0.596 | 0.693 | 0.829 | 0.945 | 1.192 | 1.438 |
GBLBP | 1.051 | 1.192 | 1.316 | 1.474 | 1.637 | 1.781 | 1.879 | 2.120 |