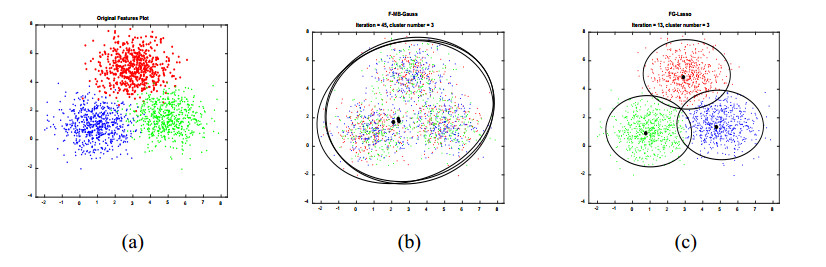
Citation: Miin-Shen Yang, Wajid Ali. Fuzzy Gaussian Lasso clustering with application to cancer data[J]. Mathematical Biosciences and Engineering, 2020, 17(1): 250-265. doi: 10.3934/mbe.2020014
[1] | Chen Zheng, Zhaobang Tan . A novel identified pyroptosis-related prognostic signature of colorectal cancer. Mathematical Biosciences and Engineering, 2021, 18(6): 8783-8796. doi: 10.3934/mbe.2021433 |
[2] | Muhammad Akram, Saba Siddique, Majed G. Alharbi . Clustering algorithm with strength of connectedness for $ m $-polar fuzzy network models. Mathematical Biosciences and Engineering, 2022, 19(1): 420-455. doi: 10.3934/mbe.2022021 |
[3] | He Ma . Achieving deep clustering through the use of variational autoencoders and similarity-based loss. Mathematical Biosciences and Engineering, 2022, 19(10): 10344-10360. doi: 10.3934/mbe.2022484 |
[4] | Muhammad Akram, Ahmad N. Al-Kenani, Anam Luqman . Degree based models of granular computing under fuzzy indiscernibility relations. Mathematical Biosciences and Engineering, 2021, 18(6): 8415-8443. doi: 10.3934/mbe.2021417 |
[5] | Xueyan Wang . A fuzzy neural network-based automatic fault diagnosis method for permanent magnet synchronous generators. Mathematical Biosciences and Engineering, 2023, 20(5): 8933-8953. doi: 10.3934/mbe.2023392 |
[6] | Meijiao Wang, Yu chen, Yunyun Wu, Libo He . Spatial co-location pattern mining based on the improved density peak clustering and the fuzzy neighbor relationship. Mathematical Biosciences and Engineering, 2021, 18(6): 8223-8244. doi: 10.3934/mbe.2021408 |
[7] | Shuo Sun, Xiaoni Cai, Jinhai Shao, Guimei Zhang, Shan Liu, Hongsheng Wang . Machine learning-based approach for efficient prediction of diagnosis, prognosis and lymph node metastasis of papillary thyroid carcinoma using adhesion signature selection. Mathematical Biosciences and Engineering, 2023, 20(12): 20599-20623. doi: 10.3934/mbe.2023911 |
[8] | Yingjian Yang, Wei Li, Yingwei Guo, Nanrong Zeng, Shicong Wang, Ziran Chen, Yang Liu, Huai Chen, Wenxin Duan, Xian Li, Wei Zhao, Rongchang Chen, Yan Kang . Lung radiomics features for characterizing and classifying COPD stage based on feature combination strategy and multi-layer perceptron classifier. Mathematical Biosciences and Engineering, 2022, 19(8): 7826-7855. doi: 10.3934/mbe.2022366 |
[9] | Jiaxi Lu, Yingwei Guo, Mingming Wang, Yu Luo, Xueqiang Zeng, Xiaoqiang Miao, Asim Zaman, Huihui Yang, Anbo Cao, Yan Kang . Determining acute ischemic stroke onset time using machine learning and radiomics features of infarct lesions and whole brain. Mathematical Biosciences and Engineering, 2024, 21(1): 34-48. doi: 10.3934/mbe.2024002 |
[10] | Chengkang Li, Ran Wei, Yishen Mao, Yi Guo, Ji Li, Yuanyuan Wang . Computer-aided differentiates benign from malignant IPMN and MCN with a novel feature selection algorithm. Mathematical Biosciences and Engineering, 2021, 18(4): 4743-4760. doi: 10.3934/mbe.2021241 |
Clustering is an unsupervised learning technique to divide data into similar groups/clusters. It has real applications in different areas such as biology, agriculture, economics, intelligent system, medical data and imaging [1,2,3,4]. It is a branch of multivariate analysis and briefly divided into two categories: non-parametric approaches and (probability) model-based clustering [5]. In non-parametric approaches, prototype-based clustering algorithms, such as k-mean [6], fuzzy c-means [7,8] and possibilistic c-means [9,10] are most used methods. In 1977, Dempster et al. [11] first proposed a probability mixture-model likelihood approach to clustering via the expectation and maximization (EM) algorithm. To consider variable selection, Pan and Shen [12] combined EM [11] with the idea of least absolute shrinkage and selection operator (Lasso) [13].
In 1993, Banfield and Raftery [14] first proposed a so-called model-based Gaussian clustering to overcome the drawbacks of existing classification maximum likelihood approaches [15,16]. Banfield and Raftery [14] utilized eigenvalue decomposition for covariance matrix so that they can assign which feature to be common to all clusters, and which feature to be different between clusters for the model-based Gaussian clustering. It was widely applied in various areas, such as image segmentation [17], gene expression data [18], and background subtraction [19]. Recently, Yang et al. [20] proposed a fuzzy model-based Gaussian (F-MB-Gauss) clustering that combines the model-based Gaussian [14] with fuzzy membership functions [21,22] for clustering. However, F-MB-Gauss [20] treats data points with feature (variable) components under equal importance, and so it cannot distinguish these irrelevant feature components. In general, there exist some irrelevant features in a data set that may cause bad performance for clustering algorithms. In this paper, we further consider the F-MB-Gauss clustering with a Lasso penalty term. We then propose a fuzzy Gaussian Lasso (FG-Lasso) clustering algorithm. The proposed FG-Lasso algorithm becomes a clustering algorithm fitted for feature selection.
Medical data with gene expression in bioinformatics is an emerging systematic biological study. It is a discipline by combining biology, computer science, information engineering, mathematics and statistics to have better interpretation of data [23,24]. Bioinformatics is closely related to computational molecular biology, In a broad sense, computational biology covers all scientific operations related with biology that involve mathematics, computation, statistics, and algorithmic methods [25,26]. Genes/features selection is a significant task in bioinformatics due to having many irrelevant genes/features, and so discarding theses irrelevant genes/features may largely enhance clustering results and is suitable for further statistical/mathematical or any other treatment to get better results. Cancer is a disease in which WHO reported it is the first or second main leading cause of death. Cancer data are important medical data. Thus, to retain only relevant features is one of significant tasks and issues for researchers, especially in cancer data.
Since the proposed FG-Lasso algorithm is good for feature selection, we apply the FG-Lasso for cancer data, especially for feature selection. It is seen that the proposed FG-Lasso can perform both feature selection and regularization to increase accuracy and interpretability of clustering. It is also a good choice for high dimensional data set. The rest of the paper is organized as follows. In Section 2, we briefly review the F-MB-Gauss clustering and then propose the FG-Lasso algorithm. In Section 3, we present numerical results of the FG-Lasso clustering algorithm. In Section 4, we apply FG-Lasso for cancer data with feature selection. Conclusions are stated in Section 5.
Model-based clustering is an essential technique to pertain data into similar and dissimilar groups/clusters by using mixtures of probability distributions. The model-based Gaussian clustering was initially proposed by Banfield and Raftery [14] to extend the classification maximum likelihood of Scott and Symons [15] and Symons [16]. Let a data set X={x1,...,xn} be a random sample from a d-variate Gaussian mixture with Gaussian distributions N(x;μk,Σk)=(2π)−d/2|Σk|−1/2exp(−(1/2)(x−μk)TΣ−1k(x−μk)). Let P={P1,,…,Pc} be a hard c-partition on X, where {P1,...,PC} is equivalent to indicator functions {z1,...,zC} with zk(x)=1 as x∈Pk, and zk(x)=0 otherwise. The objective function of the model-based Gaussian is given by J(z,θ)=n∑i=1c∑k=1zkilnfk(xi;θk), where fk(xi;θk)=N(xi;μk,Σk), and zki=zk(xi) is the membership function with zki∈{0,1} . The model-based Gaussian clustering algorithm is iterated by using the necessary conditions for maximizing the objective function J(z,θ) with ˆμk=n∑i=1zkixi/n∑i=1zkixin∑i=1zkin∑i=1zki and ˆΣk=n∑i=1zki(xi−ˆμk)(xi−ˆμk)T/n∑i=1zki(xi−ˆμk)(xi−ˆμk)Tn∑i=1zkin∑i=1zki.
Zadeh [21] proposed fuzzy sets in 1965. Afterwards, Ruspini [27] extended the indicator functions {z1,...,zC} to allow the membership zk(x) to be in the interval [0, 1] with ∑ck=1zk(x)=1 for all x∈X . These extended membership functions {z1,...,zC} are called fuzzy c-partition. Recently, Yang et al. [20] proposed a fuzzy model-based Gaussian (F-MB-Gauss) clustering by combining the model-based Gaussian with fuzzy membership functions. The F-MB-Gauss objective function is as follows [20]:
J(z,θ)=n∑i=1c∑k=1zmkilnf(x;θ)=n∑i=1c∑k=1zmkilnN(xi;μk,Σk) |
where zki is a fuzzy c-partition with the condition ∑ck=1zki=1,∀i and m is a fuzziness index with m>1 that determines the fuzziness level of clusters. However, the fuzziness index m may influence clustering results. To avoid m, the entropy term −wn∑i=1c∑k=1zkilnzki of membership functions is added. Thus, the objective function becomes as
J(z,μ,∑)=n∑i=1c∑k=1zkilnN(xi;μk,Σk)−wn∑i=1c∑k=1zkilnzki |
where w⩾0 is a parameter whose value is determined by a suitable decreasing learning rate, such as 0.999t, e−t/100, e−t/10, or e−t . In Yang et al. [20], they considered the decreasing learning rate for w with w(t)=0.999t . We adopt it in this paper.
Although F-MB-Gauss [20] presents good clustering results for data sets, it always treats feature components of data points with equal importance. There exist some irrelevant features in most data sets that always affect performance of clustering algorithms with bad clustering results. However, the F-MB-Gauss cannot distinguish these irrelevant feature components. In this paper, we further study the F-MB-Gauss to have the algorithm be able to find out these irrelevant feature components. We use the idea of Lasso (least absolute shrinkage and selection operator) that was first proposed by Tibshirani [13] as variable selection in regression models. Note that Witten and Tibshirani [28] first proposed a feature selection framework using sparse clustering, where they use Lasso constraints of feature weights to shrink features toward 0 as feature selection. Witten and Tibshirani [28] defined the sparse clustering as the optimization of maxw,Θ∑dj=1wTΘ subjectto ‖w‖1⩽s,‖w‖2⩽1,wj⩾0,∀j, where w=(w1,w2,⋯,wd)∈Rd are feature weights, and s is L1 bound of w . They proposed the sparse k-means clustering by replacing the optimization of the k-means objective function. Castro and Pu [29] further proposed a simple approach to sparse k-means clustering based on the framework of Witten and Tibshirani [28]. Qiu et al. [30] extended the sparse k-means clustering to a sparse fuzzy c-means algorithm, and more recently, Chang et al. [31] proposed another sparse fuzzy c-means algorithm by extending the framework of Witten and Tibshirani [28] to Lq(0<q⩽)−norm regularization for shrinking irrelevant feature weights to 0. However, all of these clustering algorithms for feature selection are based on Lasso constraints of feature weights. For the F-MB-Gauss clustering with Gaussian mixture distributions, it is no way in considering feature weights. However, we can use mean components μkp with λ∑ck=1∑dp=1|μkp| . Thus, we consider the F-MB-Gauss with a Lasso penalty term, and then propose a fuzzy Gaussian Lasso (FG-Lasso) clustering algorithm. The FG-Lasso objective function is as follows:
JFG−Lasso(z,μ,∑)=n∑i=1c∑k=1zkilnN(xi;μk,Σk)−wn∑i=1c∑k=1zkilnzki−λc∑k=1d∑p=1|μkp| | (1) |
where λ⩾0 is the regularization parameter that manages the amount of shrinkage and μTk=(μk1,⋯,μkd), N(xi;μk,Σk)=(2π)−d/2|Σk|−1/2exp(−(1/2)(xi−μk)TΣ−1k(xi−μk)). The parameter λ can be used as a feature selection threshold. When the values of λ are increasing, more irrelevant features will be discarded. Of course, as λ=0, the FG-Lasso becomes the F-MB-Gauss.
To obtain the necessary conditions for minimizing the FG-Lasso objective function JFG−Lasso(z,μ,∑), we use the Lagrangian as follows:
˜JFG−Lasso(z,μ,∑)=n∑i=1c∑k=1zkilnN(xi;μk,Σk)−wn∑i=1c∑k=1zkilnzki−λc∑k=1d∑p=1|μkp|−τ1(c∑k=1zki−1) |
Differentiating ˜JFG−Lasso(z,μ,∑) with respect to the fuzzy membership function zki and setting it to be zero, we get the updating equation for zki as follows:
ˆzki=[N(xi;μki,∑k)]1/wc∑s=1[N(xi;μki,∑k)]1/w | (2) |
To obtain the updating equation of μkp by differentiating ˜JFG−Lasso(z,μ,∑) with respect to μkp, we only consider the case of Σk=Σ=diag(σ2p),p=1,⋯,d. Thus, we can obtain that ∂˜JFG−Lasso(z,μ,∑)∂μkp=∑ni=1zki(xi−μkp)σ2p−λsign(μkp) . By using direct inspection of the FG-Lasso objective function JFG−Lasso(z,μ,∑), we can get the following solution for ˆμkp :
ˆμkp={˜μkp+λˆσ2p∑ni=1ˆzki,if ˜μkp<−λˆσ2p∑ni=1ˆzki0,if |˜μkp|⩽λˆσ2p∑ni=1ˆzki˜μkp−λˆσ2p∑ni=1ˆzki,if ˜μkp>λˆσ2p∑ni=1ˆzki | (3) |
with
˜μkp=∑ni=1ˆzkixip∑ni=1ˆzki | (4) |
where ˜μkp=∑ni=1ˆzkixip/∑ni=1ˆzkixip∑ni=1ˆzki∑ni=1ˆzki is the maximum likelihood estimator (MLE) of the Gaussian means. As we increase the value of λ in Eq (3), it should have some ˆμkp = 0, otherwise it has the amount λˆσ2p/λˆσ2p∑ni=1ˆzki∑ni=1ˆzki of shrinkage. Thus, during clustering processes, if |˜μkp|⩽λˆσ2p/λˆσ2p∑ni=1ˆzki∑ni=1ˆzki, then ˆμkp = 0. Otherwise ˆμkp = ˜μkp−λˆσ2p/λˆσ2p∑ni=1ˆzki∑ni=1ˆzki . Note that ˜μkp=∑ni=1ˆzkixip/∑ni=1ˆzkixip∑ni=1ˆzki∑ni=1ˆzki is the MLE of the normal mean μkp. However, the updating Eq (3) for ˆμkp represents the contribution of the pth feature to the cluster k through the regularization parameter λ . If ˆμkp=0 for all k, then the pth feature has no contribution to clustering that is non-informative and then discarded. This is why we consider the Lasso penalty λ∑ck=1∑dp=1|μkp| to the F-MB-Gauss objective function such that it becomes the FG-Lasso objective function (1). Thus, the FG-Lasso algorithm can have a behavior of feature selection.
To drive the updating Eq (3) of ˆμkp, we use the FG-Lasso objective function JFG−Lasso(z,μ,∑). Taking the derivative of JFG−Lasso(z,μ,∑) with respect to μkp, we get ∂JFG−Lasso(z,μ,∑)∂μkp=∑ni=1zki(xi−μkp)σ2p−λsign(μkp) . Set it to be 0, and after simplification, we get n∑i=1ˆzkiσ−2p(xip−μkp)−λsign(μkp)=0, and have ∑ni=1ˆzkixip=λσ2psign(μkp)+∑ni=1ˆzkiμkp . Dividing both side by ∑nj=1ˆzkj, we obtain the Eq of ˆμkp with ˆμkp=∑ni=1ˆzkixip∑ni=1ˆzki−λσ2psign(ˆμkp)∑ni=1ˆzki . Thus, we have ˆμkp=˜μkp−λσ2psign(ˆμkp)∑ni=1ˆzki . As we know that |ˆμkp| is not differentiable at ˆμkp = 0, and so we need to cope up this problem by using subderivative or subetaadient of a convex function. In case of the absolute value function f(x)=λ|x|, the subetaadient or subderivative is defined by ∂f(x)={{−λ}ifx<0[−λ,λ]ifx=0{+λ}ifx>0 . To see further explanation about the soft threshold operator λ for regression models, Hastie et al. [32] is a good reference. Thus, we can obtain the updating Eq (3) for ˆμkp in our case.
Similarly, for the common diagonal covariance matrix Σk=Σ=diag(σp),p=1,⋯,d, differentiating ˜JFG−Lasso(z,μ,∑) with respect to σ2p,p=1,⋯,d, we can get the following updating Eq:
ˆσ2p=∑ck=1∑ni=1zki(xip−˜μkp)2∑ck=1∑ni=1ˆzki | (5) |
Due to the expected singularity of matrix when the cluster number is large in the FG-Lasso algorithm, we use the following condition to overcome this problem:
˜σ2p=(1−γ)σ2p+γω | (6) |
where γ is a small positive number and ω is a diagonal matrix with a small positive number. Here we use γ = 0.0001, ω=d2min, Id=min{d2ij=‖xi−xj‖2>0,1⩽i,j⩽n}. For w, we use the same decreasing learning rate as Yang et al. [20] with
w(t)=0.999t | (7) |
Thus, the proposed FG-Lasso clustering algorithm can be summarized as follows.
FG-Lasso Algorithm
Step 1: Fix ε>0 . Give initials for μ and σ2,(0)p . Set λ = 1 and t=1 .
Step 2: Compute ˆz(0)kj by using Eq (2).
Step 3: Compute ˜μ(t)kp by using Eq (4).
Step 4: Compute w(t) by using Eq (7).
Step 5: Update ˆσ2,(t)p with ˜μ(t)kp and ˆz(t−1)kj by Eqs (5) and (6).
Step 6: Update ˆz(t)kj with ˜μ(t)kp, w(t) and ˆσ2,(t)p by using Eq (2).
Step 7: Update ˜μ(t+1)kp with ˆz(t)kj by using Eq (4).
If max‖˜μkp(t+1)−˜μkp(t)‖<ε stop.
Else t=t+1 and return to Step 3.
Step 8: Update ˆσ2,(t+1)p with ˜μ(t+1)kp and ˆz(t)kj by using Eqs (5) and (6).
Step 9: Update ˆμ(t)kp with ˆz(t)kj, ˜μ(t+1)kp and ˆσ2,(t+1)kp by using Eq (3), that is,
If |˜μ(t+1)kp|⩽λˆσ2,(t+1)p∑nj=1ˆz(t)kj, then let ˆμ(t)kp = 0.
Else ˆμ(t)kp=˜μ(t+1)kp−λˆσ2,(t+1)p∑nj=1ˆz(t)kj.
Step 10: Increase λ and return to Step 3, or output results.
In this section, we demonstrate the performance of the proposed FG-Lasso clustering algorithm. Several synthetic and real data sets are used to have more insights to the feature selection behaviors of the FG-Lasso algorithm. We also give the comparisons of the proposed FG-Lasso with F-MB-Gauss [20]. The accuracy rate (AR) is used as a criterion for evaluating the performance of a clustering algorithm. AR is the percentage of data points that are correctly identified by the clustering algorithm in which AR is defined as AR=∑ki=1ri/n, where ri is the number of points in Ci′ that are also in Ci in which C={C1,C2,⋯,Cc} is the set of c clusters for the given data set and C′={C′1,C′2,⋯,C′c} is the set of c clusters generated by the clustering algorithm.
Example 1. In this example, a simulation data set is used to demonstrate the significance of the FG-Lasso algorithm, where the usefulness of λ for feature selection, especially to remove irrelevant features, is demonstrated. A data set, called 3-cluser Gaussian data, with having 1800 points are generated from a Gaussian mixture with α1=α2=α3=1/3 where 600 points are from the normal distribution with μ1=(11) and ∑1=(10;01), 600 points are from the normal distribution with μ2=(35) and ∑2=(10;01) while the same size in the cluster three with μ3=(51.5) and ∑1=(10;01) . We consider the two features, named as feature1 and feature2, as informative. We then add other two irrelevant features generated from the uniform distributions over the intervals [﹣5, 5] and [﹣10, 10], respectively, named as feature3 and feature4, that are considered as non-informative features. The original data set with two informative features feature1 and feature2 is shown in Figure 1(a). Figure 1(b) represents the clustering results of the F-MB-Gauss algorithm with the 3 cluster centers after 45 iterations. The final clustering results of the FG-Lasso algorithm with the 3 clusters after 13 iterations are shown in Figure 1(c). Results of ARs from the FG-Lasso and F-MB-Gauss algorithms are shown in Table 1. It is seen that the proposed FG-Lasso is feasible for feature selection with the final feature number of d* = 2. However, the F-MB-Gauss algorithm cannot have feature selection and so it give the final feature number of d = 4. It is clearly that the irrelevant features of feature3 and feature4 actually distort the final clustering results for the F-MB-Gauss algorithm with d = 4 and average AR = 0.3501. However, the proposed FG-Lasso can discard these non-informative features of feature3 and feature4 with d* = 2 and a high average AR = 0.961. The details of discarded features as increasing the values of λ using FG-Lasso are shown in Table 2. When the value of λ is increasing as 10, we obtain ˆμ13=ˆμ23=ˆμ33=0 and so the feature feature3 is discarded. Similarly, when we increase the value of λ as 15, we obtain ˆμ14=ˆμ24=ˆμ34=0 and, so the feature feature4 is discarded. Thus, it successfully discards all irrelevant features of feature3 and feature4 when the value of λ is 15. From Table 2, we find that both features of feature1 and feature2 are informative, but features feature3 and feature4 are non-informative, and then discarded.
c | F-MB-Gauss | FG-Lasso | ||
d | AR | d* | AR | |
3 | 4 | 0.3501 | 2 | 0.961 |
Features | λ | |
10 | 15 | |
feature1 | - | - |
feature2 | - | - |
feature3 | ˆμ13=ˆμ23=ˆμ33=0 | × |
feature4 | - | ˆμ14=ˆμ24=ˆμ34=0 |
Example 2. We also use a simulation data set for the proposed FG-Lasso algorithm to demonstrate the significance of λ for feature selection, especially to remove irrelevant features. A data set, called 5-cluser Gaussian data, with 800 points are generated from a Gaussian mixture with α1=α2=α3=α4=α5=1/5, μ1=(810), μ2=(820), μ3=(1510), μ4=(1520), and μ5=(1116) with ∑1=∑2=∑3=∑4=∑5=(10;01) . We consider the two features, named as feature1 and feature2, as informative. We then add one irrelevant feature generated from the uniform distributions over the intervals [0, 65], named as feature3, that is considered as a non-informative feature. The original data set with two informative features feature1 and feature2 is shown in Figure 2(a). Figure 2(b) represents the final clustering results of the F-MB-Gauss algorithm with 5 cluster centers after 1270 iterations. The final clustering results of the proposed FG-Lasso algorithm with 5 clusters after 15 iterations are shown in Figure 2(c). Results of average ARs from the FG-Lasso and F-MB-Gauss algorithms with 50 different initializations are shown in Table 3. It is seen that the proposed FG-Lasso is feasible for feature selection with the final feature number of d* = 2. It is clearly that irrelevant features actually distort the final clustering results for the F-MB-Gauss algorithm with average AR = 0.320 that cannot give a feature selection behavior with the final feature number of d = 3. However, the proposed FG-Lasso can discard the non-informative feature feature3 with a high average AR = 0.810. The details of discarded feature as increasing the values of λ using FG-Lasso are also shown in Table 4. When the values of λ is increasing as 80, we obtain ˆμ13=ˆμ43=ˆμ53=0 and when we increase the value of λ is 105, we obtain ˆμ23=ˆμ33=0 . It is clearly the feature feature3 is discarded. Thus, it successfully discards irrelevant feature feature3 as the value of λ is 80 or 105. From Table 4, we find that both features feature1 and feature2 are informative, but feature feature3 is non-informative, and then discarded.
c | F-MB- Gauss | FG-Lasso | ||
d | AR | d* | AR | |
5 | 3 | 0.320 | 2 | 0.810 |
Features | λ | |
80 | 105 | |
feature1 | - | - |
feature2 | - | - |
feature3 | ˆμ13=ˆμ43=ˆμ53=0 | ˆμ23=ˆμ33=0 |
Except the above two synthetic data sets, we also use a real data set, Pima indian, from UCI repository data [33].
Example 3 (Pima indian [33]). In this example, we consider the real data set of Pima indian [33]. This data set consists of 8 features, named as the number of times of Pregnant, Plasma glucose concentration a 2 hours in an oral glucose tolerance test, Diastolic blood pressure (mm Hg), Triceps skin fold thickness (mm), 2-Hour serum insulin (mu U/ml), Body mass index (weight in kg/(height in m)^2), Diabetes pedigree function, and Age (years), while class variable (Outcomes). The data set has two classes. By using F-MB-Gauss, we obtain the average AR = 0.45 when 30 different initializations are considered. As we increase the values of λ to λ = 50, the proposed FG-Lasso algorithm discards the features, Plasma glucose concentration a 2 hours in an oral glucose tolerance test, Diastolic blood pressure, and 2-Hour serum insulin, with a higher average AR = 0.67. This shows the good aspect of the proposed FG-Lasso clustering algorithm for the Pima indian data set.
Cancer is uncontrolled growth of abnormal cells in a body found in a group of diseases [34]. According to the estimate from WHO (World Health Organization), it is the first or second main leading cause of death before 70 years in 91 out of 172 countries [35]. It spreads and affects the other parts of body if there is not properly diagnosed. This severe disease has many symptoms such as tumor, abnormal bleeding, long-term cough, more weight loss, etc. According to the Global Cancer Incidence, Mortality and Prevalence (GLOBOCAN), cancer has extended 36 types in which lung cancer is the most common diseases (11.6%) of the total cases in male and female [36]. Other alarming leading cancer diseases are breast cancer (11.6%), prostate cancer (7.1%), colorectal cancer (6.1%), stomach cancer (8.2%) and liver cancer (8.2%) [35,36]. Breast cancer is the most leading and commonly diagnosed cancer disease among females [36,37,38] and leading breast cancer issue in 154 out of 185 countries [38]. According to the WHO findings, there were 2.1 million newly women diagnosed breast cases in 2018. The highest statistics found countries are Australia/New-Zealand, United Kingdom, Sweden Finland, Denmark Belgium (Highest rate), the Netherlands and France. There are many risk factors of breast cancer like family history, physical activity, breast feeding, hormones intake, alcohol intake, greater weight and body fat [36,39]. There are three important techniques to diagnose breast cancer, namely as mammography, Fine Needle Aspirate (FNA) biopsy and surgical biopsy. To demonstrate the applicability of the proposed FG-Lasso clustering algorithm, we use the following three real cancer data sets, Breast cancer Wisconsin, Colon tissues, and Leukemia data sets. We implement the FG-Lasso and F-MB-Gauss algorithms to the three cancer data sets and compare their results.
Example 4 (Breast Cancer Wisconsin). Breast cancer Wisconsin data was created in Street et al. [40]. This data consists of 569 FNA with 212 malignant (patients sample) and 357 benign (healthy samples) [36,40]. The 30 attributes are computed from a digitized image of a FNA of breast mass. They describe characteristics of the cell nuclei presented in the image. Real values are computed from each cell nucleus, namely as radius (from center to points on the perimeter), texture (gray-scale values), perimeter, area, smoothness (local variation in radius lengths), compactness (perimeter^2 /area-1.0), concavity (severity of concave portions of the contour), concave points (number of concave portions of the contour), symmetry and fractal dimension ("coastline approximation"-1), where each one in the 10 features has three components as mean, standard error and worst. That has 30 features. The FG-Lasso and F-MB-Gauss algorithms are implemented on Breast cancer Wisconsin where clustering results are shown in Table 5. From Table 5, it is seen that the F-MB-Gauss algorithm obtains a good accuracy rate with d = 30 and AR = 0.905 when 30 different initializations are considered. Using the same 30 initializations, we also implement the proposed FG-Lasso algorithm on the data set. There are 27 from 30 features to be selected with d* = 27 and a little better accuracy rate of AR = 0.925 when the λ value is 12, where the features of area mean, area standard error and area worst are considered as unimportant features and then discarded.
c | F-MB-Gauss | FG-Lasso | ||
d | AR | d* | AR | |
2 | 30 | 0.905 | 27 | 0.925 |
Next, we implement the FG-Lasso and F-MB-Gauss algorithms to the colon tissue data set [41].
Example 5 (Colon Tissues). The Colon tissue data set consists of colon cancer which contains 62 samples from the microarray experiments of colon tissues samples with 2000 genes and two classes (40 tumor tissues and 22 normal tissues) [41]. Colorectal cancer is the kind of cancer that starts tissue or tumor growth on the inner lining of the colon. It is the third incidence of death and second in term of mortality in the world [41]. Most suffered in colon cancer countries are Hungry, Slovenia, Netherlands, Norway, Australia New Zealand, North America, Japan, Republic of Korea, and Singapore (in Females). Among these countries, Hungry is ranked first in males and Norway is ranked first in females, while highest colon incidence rates are found in Republic of Korea among males and Macedonia among females [36,42]. We first standardize the data set, and then apply the FG-Lasso and F-MB-Gauss algorithms to the Colon tissue data set where the clustering results are shown in Table 6. From Table 6, it is seen that the F-MB-Gauss algorithm obtains the average accuracy rate AR = 0.601 with 30 different initializations. When the proposed FG-Lasso algorithm is implemented on the data set with the same 30 different initializations, there are 1384 from 2000 features to be selected with the final feature number of d* = 1384 when the λ value is increasing to be 90 in which it obtains a better average accuracy rate AR = 0.653, as shown in Table 6. This demonstrates that the proposed FG-Lasso algorithm is significant for feature selection on the Colon tissue data set.
c | F-MB-Gauss | FG-Lasso | ||
d | AR | d* | AR | |
2 | 2000 | 0.601 | 1384 | 0.653 |
Finally, we implement the FG-Lasso and F-MB-Gauss algorithms to the Leukemia cancer data set. Leukemia is the type of cancer as the uncontrolled growth of hematopoietic stem cell in the bone marrow occurs. Leukemia is a well-known data that the type of cancer occurs as the uncontrolled growth of hematopoietic stem cell in the bone marrow. It is the most common in white male people and increase according to ages [43,44]. Broadly there are four subtypes of leukemia, acute lymphoblastic, acute myelogenous, chronic lymphocytic, and chronic myelogenous. Acute lymphoblastic leukemia (ALL) is most commonly found in children while the other three types occur in adults. ALL causes fever, lethargy, bleeding, musculoskeletal pain or dysfunction. On the other hand, fever, fatigue, weight loss, bleeding or bruising are the most commonly symptoms of acute myelogenous leukemia (AML) [44]. According to the global cancer statistics [36], leukemia has 2.4% rate of new cases, and 3.2% of deaths rate worldwide in 2018.
Example 6 (Leukemia Data). Leukemia data had originally considered by Golub et al. [45]. The data consists of 38 patients considered as observations each from leukemia patients with their biological sample array while 7129 genes are considered as features. Among these samples, 27 are acute lymphoblastic leukemia (ALL) and 11 are acute myelogenous leukemia (AML). Golub et al. [45] distinguished two types of patients due to their isolation clinical treatments. We only sort 2000 genes according to their variances and we also standardize data so each attribute has mean 0 and variance 1. We implement the FG-Lasso and F-MB-Gauss algorithms to the Leukemia data set where the clustering results are shown in Table 7. From Table 7, it is seen that the F-MB-Gauss algorithm obtains the low average accuracy rate AR = 0.393 with 30 different initializations. This shows that these irrelevant features in the Leukemia data set actually affects clustering results. When the proposed FG-Lasso algorithm is applied to the data set, there are 654 from 2000 features to be selected with the final feature number of d* = 654 when the λ value is increasing to be 350 in which it promotes the accuracy rate to AR = 0.615. That is, the proposed FG-Lasso algorithm is quite significant for feature selection on the Leukemia data set by selecting 654 from 2000 features.
c | F-MB-Gauss | FG-Lasso | ||
d | AR | d* | AR | |
2 | 2000 | 0.393 | 654 | 0.615 |
The F-MB-Gauss clustering proposed by Yang et al. [20] always treats feature components in data points with equal importance, and so it does not have a feature selection behavior. However, there generally exist irrelevant features in data that may badly affect the performance of clustering algorithms. In this paper, we extended the F-MB-Gauss clustering to the fuzzy Gaussian Lasso (FG-Lasso) using a Lasso penalty term of Gaussian means components. The FG-Lasso algorithm is then proposed for clustering data sets with feature selection. The proposed FG-Lasso has good behaviors with better choice for feature selection. Several experimental results and comparisons have actually demonstrated the feature selection aspect of the proposed FG-Lasso algorithm. According to the estimate from WHO, cancer is the first or second main leading cause of death. This severe disease has many symptoms such as tumor, abnormal bleeding, long-term cough, and more weight loss. Cancer data are important medical data where they are high dimensional and exist many irrelevant features. In this paper, we also apply the proposed FG-Lasso algorithm to the three cancer data, Breast cancer Wisconsin, Colon tissues, and Leukemia. According to clustering results, it is seen that the proposed FG-Lasso can select these important features with a higher accuracy as increasing of the threshold λ . However, our question is what value of the threshold λ should be to have an optimal number of features in the FG-Lasso clustering algorithm. That is, to find a good estimate for the threshold parameter λ should be important and will be our further research topic. On the other hand, to consider a whole covariance matrix, not only a diagonal matrix, is also another problem in FG-Lasso, and it would be also our future work.
The authors would like to thank the anonymous referees for their helpful comments in improving the presentation of this paper.
The authors declare there is no conflict of interest.
[1] | L. Kaufman, P.J. Rousseeuw, Finding Groups in Data: An Introduction to Cluster Analysis, Wiley-Interscience, New York, 2009. |
[2] | J. C. Bezdek, Pattern Recognition with fuzzy objective function algorithms, Plenum Press, New York, 1981. |
[3] | D. Jiang, C. Tang and A. Zhang, Cluster analysis for gene expression data: A survey, IEEE Trans. Knowl. Data Eng.,16 (2004), 1370-1386. |
[4] | J. M. T. Wu, C. W. Lin, P. Fournier-Viger, et al., The density-based clustering method for privacy-preserving data mining, Math. Biosci. Eng., 16 (2019), 1718-1728. |
[5] | M. S. Yang, C. Y. Lai and C. Y. Lin, A robust EM clustering algorithm for Gaussian mixture models, Pattern Recognit., 45 (2012), 3950-3961. |
[6] | A. K. Jain, Data clustering: 50 years beyond k-means, Pattern Recognition Lett., 31 (2010), 651-666. |
[7] | A. Baraldi and P. Blonda, A survey of fuzzy clustering algorithms for pattern recognition-part I and part II, IEEE Trans. Syst. Man Cybern. B, 29 (1999), 778-785. |
[8] | M. S. Yang and Y. Nataliani, Robust-learning fuzzy c-means clustering algorithm with unknown number of clusters, Pattern Recogni t., 71 (2017), 45-59. |
[9] | R. Krishnapuram and J. M. Keller, A possibilistic approach to clustering, IEEE Trans. Fuzzy Syst., 1 (1993), 98-110. |
[10] | M. S. Yang, S. J. Chang-Chien and Y. Nataliani, A fully-unsupervised possibilistic c-means clustering method, IEEE Access, 6 (2018), 78308-78320. |
[11] | A. P. Dempster, N. M. Laird and D. B. Rubin, Maximum likelihood from incomplete data via the EM algorithm (with discussion), J. R. Stat. Soc. Ser. B, 39 (1977), 1-38. |
[12] | W. Pan and X. Shen, Penalized model-based clustering with application to variable selection, J. Mach. Learn. Res., 8 (2007), 1145-1164. |
[13] | R. Tibshirani, Regression shrinkage and selection via the Lasso, J. R. Stat. Soc. Ser. B, 58 (1996), 267-288. |
[14] | J. D. Banfield and A. E. Raftery, Model-based Gaussian and non-Gaussian Clustering, Biometrics, 49 (1993), 803-821. |
[15] | A. J. Scott and M. J. Symons, Clustering methods based on likelihood ratio criteria, Biometrics, 27 (1971), 387-397. |
[16] | M. J. Symons, Clustering criteria and multivariate normal mixtures, Biometrics,37 (1981), 35-43. |
[17] | R. Wehrens, L. M. C. Buydens, C. Fraley, et al., Model-based clustering for image segmentation and large datasets via sampling, J. Classif., 21 (2004), 231-253. |
[18] | W. C. Young, A. E. Raftery and K. Y. Yeung, Model-based clustering with data correction for removing artifacts in geneexpression data, Ann. Appl. Stat., 11 (2017), 1998-2026. |
[19] | T. Akilan, Q. M. J. Wu and Y. Yang, Fusion-based foreground enhancement for background subtraction using multivariate multi-model Gaussian distribution, Inf. Sci., 430-431 (2018), 414-431. |
[20] | M. S. Yang, S. J. Chang-Chien and Y. Nataliani, Unsupervised fuzzy model-based Gaussian clustering,Inf. Sci., 481 (2019), 1-23. |
[21] | L. A. Zadeh, Fuzzy sets, Inf. Control, 8 (1965), 338-353. |
[22] | M. S. Yang and Y. Nataliani, A feature-reduction fuzzy clustering algorithm based on feature-weighted entropy,IEEE Trans. Fuzzy Syst., 26(2018), 817-835. |
[23] | K. Voevodski, M. F. Balcan, H. Röglin, et al., Active clustering of biological sequences, J. Mach. Learn. Res., 13 (2012), 203-225. |
[24] | D. Gaweł and K. Fujarewicz, On the sensitivity of feature ranked lists for large-scale biological data, Math. Biosci. Eng., 10 (2013), 667-690. |
[25] | J. Xiong, Essential Bioinformatics, Cambridge University Press, New York, 2006. |
[26] | R. Jiang, X. Zhang, M. Q. Zhang, Basics of Bioinformatics, Springer-Verlag Berlin An, 2013. |
[27] | E. H. Ruspini, A new approach to clustering,Inf. Control, 15 (1969), 22-32. |
[28] | D. M. Witten and R. Tibshirani, A framework for feature selection in clustering, J. Am. Stat. Assoc.,105 (2010), 713-726. |
[29] | E. A. Castro and X. Pu, A simple approach to sparse clustering, Comput. Stat. Data Anal.,105 (2017), 217-228. |
[30] | X. Qiu, Y. Qiu, G. Feng, et al., A sparse fuzzy c-means algorithm base on sparse clustering framework, Neurocomputing,157 (2015), 290-295. |
[31] | X. Chang, Q. Wang, Y. Liu, et al., Sparse regularization in fuzzy c-means for high-dimensional data clustering,IEEE Trans. Cybern., 47 (2017), 2616-2627. |
[32] | T. Hastie, R. Tibshirani and M. Wainwright, Statistical Learning with Sparsity: The lasso and Generalization, Chapman and Hall/CRC press, New York, (2015). |
[33] | C. L. Blake and C. J. Merz, UCI repository of machine learning database, a huge collection of artificial and real-world data sets, (1988). |
[34] | N. K. Phan, Biological therapy: A new age of cancer treatment, Biomed. Res. Ther., 1 (2014), 32-34. |
[35] | Global Health Observatory (GHO) data, World Health Organization, Geneva, 2018. Available from: https://www.who.int/gho/en/. |
[36] | F. Bray, J. Ferlay, I. Soerjomataram, et al., A. Jemal, Global cancer statistics 2018: GLOBOCAN estimates of incidence and mortality worldwide for 36 cancers in 185 countries, CA A Cancer J. Clin., 68 (2018), 394-424. |
[37] | D. N. K. Boulos and R. R. Ghali, Awareness of breast cancer among female students at Ain Shams University, Egypt, Glob. J. Health Sci., 6 (2014), 154-161. |
[38] | K. McPherson, C. M. Steel and J. M. Dixon, Breast cancer-epidemiology, risk factors, and genetics, BMJ, 321 (2000), 624-628. |
[39] | R. R. Janghel, A. Shukla, R. Tiwari, et al., Intelligent decision support system for breast cancer, International Conference in Swarm Intelligence, Beijing, China, 2010, 351-358. Available from: https://link_springer.gg363.site/chapter/10.1007/978-3-642-13498-2_46#citeas. |
[40] | W. N. Street, W. H. Wolberg and O. L. Mangasarian, Nuclear feature extraction for breast tumor diagnosis, Biomedical image processing and biomedical visualization, 1905 (1993), 861-870. Available from: https://doi.org/10.1117/12.148698. |
[41] | A. R. Marley and H. Nan, Epidemiology of colorectal cancer, Int. J. Mol. Epidemiol. Genet., 7 (2016), 105-114. |
[42] | M. Arnold, M. S. Sierra, M. Laversanne, et al., Global patterns and trends in colorectal cancer incidence and mortality, Gut, 66 (2017), 683-691. |
[43] | Cancer Stat Facts: Leukemia, National Cancer Institute, Surveillance Epidemiology and End Results Program, 2006-2010. Available from: http://seer.cancer.gov/statfacts/html/leuks.html. |
[44] | A. S. Davis, A. J. Viera and M. D. Mead, Leukemia: An overview for primary care, Am. Fam. Physician, 89 (2014), 731-738. |
[45] | T. R. Golub, D. K. Slonim, P. Tamayo, et al., Molecular classification of cancer: Class discovery and class prediction by gene expression monitoring, Science,286 (1999), 531-537. |
1. | Jianfei Song, Zhenyu Li, Guijin Yao, Songping Wei, Ling Li, Hui Wu, Vijayalakshmi Kakulapati, Framework for feature selection of predicting the diagnosis and prognosis of necrotizing enterocolitis, 2022, 17, 1932-6203, e0273383, 10.1371/journal.pone.0273383 | |
2. | Dan Wang, Yier Lin, Liang Hong, Ce Zhang, Yajie Bai, Zhen Zhen Bi, Naeem Jan, Detection of the Driver’s Mental Workload Level in Smart and Autonomous Systems Using Physiological Signals, 2022, 2022, 1563-5147, 1, 10.1155/2022/5233257 | |
3. | Wajid Ali, Ishtiaq Hussain, Miin-Shen Yang, 2022, Chapter 25, 978-981-16-7181-4, 307, 10.1007/978-981-16-7182-1_25 | |
4. | Wajid Ali, Miin-Shen Yang, Mehboob Ali, Saif Ud-Din, Fuzzy model-based sparse clustering with multivariate t-mixtures, 2023, 37, 0883-9514, 10.1080/08839514.2023.2169299 | |
5. | Maria Brigida Ferraro, Marco Forti, Paolo Giordani, 2024, FKML0: A Matlab Routine for Sparse Fuzzy Clustering, 979-8-3503-1954-5, 1, 10.1109/FUZZ-IEEE60900.2024.10611853 | |
6. | Young-Geun Choi, Soohyun Ahn, Jayoun Kim, Model-Based Clustering of Mixed Data With Sparse Dependence, 2023, 11, 2169-3536, 75945, 10.1109/ACCESS.2023.3296790 | |
7. | Maria Brigida Ferraro, Marco Forti, Paolo Giordani, 2025, Chapter 5, 978-3-031-64446-7, 28, 10.1007/978-3-031-64447-4_5 | |
8. | Maria Brigida Ferraro, Marco Forti, Paolo Giordani, Fuzzy clustering with $$\hbox {L}_0$$ regularization, 2025, 0254-5330, 10.1007/s10479-025-06502-1 |
c | F-MB-Gauss | FG-Lasso | ||
d | AR | d* | AR | |
3 | 4 | 0.3501 | 2 | 0.961 |
Features | λ | |
10 | 15 | |
feature1 | - | - |
feature2 | - | - |
feature3 | ˆμ13=ˆμ23=ˆμ33=0 | × |
feature4 | - | ˆμ14=ˆμ24=ˆμ34=0 |
c | F-MB- Gauss | FG-Lasso | ||
d | AR | d* | AR | |
5 | 3 | 0.320 | 2 | 0.810 |
Features | λ | |
80 | 105 | |
feature1 | - | - |
feature2 | - | - |
feature3 | ˆμ13=ˆμ43=ˆμ53=0 | ˆμ23=ˆμ33=0 |
c | F-MB-Gauss | FG-Lasso | ||
d | AR | d* | AR | |
2 | 30 | 0.905 | 27 | 0.925 |
c | F-MB-Gauss | FG-Lasso | ||
d | AR | d* | AR | |
2 | 2000 | 0.601 | 1384 | 0.653 |
c | F-MB-Gauss | FG-Lasso | ||
d | AR | d* | AR | |
2 | 2000 | 0.393 | 654 | 0.615 |
c | F-MB-Gauss | FG-Lasso | ||
d | AR | d* | AR | |
3 | 4 | 0.3501 | 2 | 0.961 |
Features | λ | |
10 | 15 | |
feature1 | - | - |
feature2 | - | - |
feature3 | ˆμ13=ˆμ23=ˆμ33=0 | × |
feature4 | - | ˆμ14=ˆμ24=ˆμ34=0 |
c | F-MB- Gauss | FG-Lasso | ||
d | AR | d* | AR | |
5 | 3 | 0.320 | 2 | 0.810 |
Features | λ | |
80 | 105 | |
feature1 | - | - |
feature2 | - | - |
feature3 | ˆμ13=ˆμ43=ˆμ53=0 | ˆμ23=ˆμ33=0 |
c | F-MB-Gauss | FG-Lasso | ||
d | AR | d* | AR | |
2 | 30 | 0.905 | 27 | 0.925 |
c | F-MB-Gauss | FG-Lasso | ||
d | AR | d* | AR | |
2 | 2000 | 0.601 | 1384 | 0.653 |
c | F-MB-Gauss | FG-Lasso | ||
d | AR | d* | AR | |
2 | 2000 | 0.393 | 654 | 0.615 |