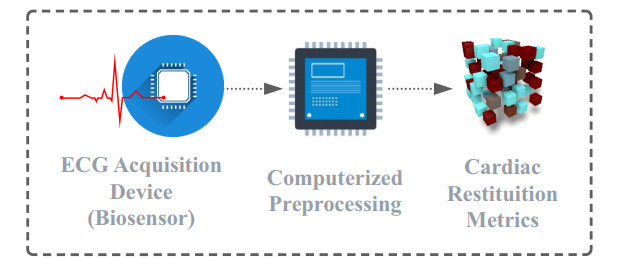
Citation: Miin-Shen Yang, Wajid Ali. Fuzzy Gaussian Lasso clustering with application to cancer data[J]. Mathematical Biosciences and Engineering, 2020, 17(1): 250-265. doi: 10.3934/mbe.2020014
[1] | Nicholas Pearce, Eun-jin Kim . Modelling the cardiac response to a mechanical stimulation using a low-order model of the heart. Mathematical Biosciences and Engineering, 2021, 18(4): 4871-4893. doi: 10.3934/mbe.2021248 |
[2] | Keyue Yan, Tengyue Li, João Alexandre Lobo Marques, Juntao Gao, Simon James Fong . A review on multimodal machine learning in medical diagnostics. Mathematical Biosciences and Engineering, 2023, 20(5): 8708-8726. doi: 10.3934/mbe.2023382 |
[3] | Chunkai Zhang, Yingyang Chen, Ao Yin, Xuan Wang . Anomaly detection in ECG based on trend symbolic aggregate approximation. Mathematical Biosciences and Engineering, 2019, 16(4): 2154-2167. doi: 10.3934/mbe.2019105 |
[4] | Danial Sharifrazi, Roohallah Alizadehsani, Javad Hassannataj Joloudari, Shahab S. Band, Sadiq Hussain, Zahra Alizadeh Sani, Fereshteh Hasanzadeh, Afshin Shoeibi, Abdollah Dehzangi, Mehdi Sookhak, Hamid Alinejad-Rokny . CNN-KCL: Automatic myocarditis diagnosis using convolutional neural network combined with k-means clustering. Mathematical Biosciences and Engineering, 2022, 19(3): 2381-2402. doi: 10.3934/mbe.2022110 |
[5] | Yajing Zeng, Siyu Yang, Xiongkai Yu, Wenting Lin, Wei Wang, Jijun Tong, Shudong Xia . A multimodal parallel method for left ventricular dysfunction identification based on phonocardiogram and electrocardiogram signals synchronous analysis. Mathematical Biosciences and Engineering, 2022, 19(9): 9612-9635. doi: 10.3934/mbe.2022447 |
[6] | Tetiana Biloborodova, Lukasz Scislo, Inna Skarga-Bandurova, Anatoliy Sachenko, Agnieszka Molga, Oksana Povoroznyuk, Yelyzaveta Yevsieieva . Fetal ECG signal processing and identification of hypoxic pregnancy conditions in-utero. Mathematical Biosciences and Engineering, 2021, 18(4): 4919-4942. doi: 10.3934/mbe.2021250 |
[7] | Lei Lu, Tingting Zhu, Ying Tan, Jiandong Zhou, Jenny Yang, Lei Clifton, Yuan-Ting Zhang, David A. Clifton . Refined matrix completion for spectrum estimation of heart rate variability. Mathematical Biosciences and Engineering, 2024, 21(8): 6758-6782. doi: 10.3934/mbe.2024296 |
[8] | Eun-jin Kim, Massimo Capoccia . Mechano-electric effect and a heart assist device in the synergistic model of cardiac function. Mathematical Biosciences and Engineering, 2020, 17(5): 5212-5233. doi: 10.3934/mbe.2020282 |
[9] | Rong Qiang, Wanbiao Ma, Ke Guo, Hongwu Du . The differential equation model of pathogenesis of Kawasaki disease with theoretical analysis. Mathematical Biosciences and Engineering, 2019, 16(5): 3488-3511. doi: 10.3934/mbe.2019175 |
[10] | Enes Efe, Emrehan Yavsan . AttBiLFNet: A novel hybrid network for accurate and efficient arrhythmia detection in imbalanced ECG signals. Mathematical Biosciences and Engineering, 2024, 21(4): 5863-5880. doi: 10.3934/mbe.2024259 |
Identifying patients at high risk for sudden cardiac death (SCD) is a major scientific challenge in cardiac diseases. SCD represents one of the leading causes of death around the world [1]. In particular, SCD corresponds to approximately 65% of the deaths of patients diagnosed with Chagas heart disease (ChHD) [2], a prevalent endemic disease in Brazil and 20 Latin American countries [3]. The rate of SCD in ChHD is 2.4 percent per year, substantially more significant than the SCD rate for the general population [4]. World Health Organization (WHO) estimates that 6–7 million people are infected with Chagas disease (CD). Caused by Trypanosoma cruzi, it is a life-threatening and persistent illness, and up to 1.5 million (30%) of them suffer from ChHD in the world [5]. Due to increasing migratory, it has trespassed frontiers, and is rising in non-endemic countries (North America, Europe, Japan, and Australia).
The unpredictability of SCD is widely recognized, making this identification a crucial open problem [1]. In ChHD, although the patients at high risk for SCD can theoretically be identified by their risk factors, in practice, the most significant number of SCD cases occurs in patients not previously determined to be at high risk [6]. Notably, this unpredictability hinders the widespread implementation of effective preventive measures on a large scale against SCD in ChHD patients [7,8,9]. A significant number of these patients die in this context, which could be empirically avoided if SCD risk was accurately identified and treated with implantable cardioverter defibrillators (ICDs) [10]. Idealistically, accurate and early identification of patients at high risk for SCD could enable the adoption of more advanced but costly treatments.
Several studies of Machine Learning (ML) algorithms applied to cardiology can be found in databases such as PubMed and MEDLINE. Almost all cardiology subfields have applied ML to automatic ECG interpretation, result analysis, monitoring, or diagnostic support systems [11,12]. Concerning the specific context related to SCD, some studies have shown that ML can classify SCD patients with the same or greater accuracy than clinicians [13]. Despite the relevance of the SCD issue, analyzed as a global phenomenon [1], its occurrence in the specific context of ChHD has been poorly exploited, especially considering the use of machine learning computing tools. In parallel, technologies for real-time cardiac activity monitoring has grown significantly in recent decades, being a valuable instrument for use in medicine, considering currently miniaturized devices, wearable or even implantable systems, which are based on highly efficient biosensors, to provide data related to the heart electrical activity, the heart's rate variability and other physiological signals such as blood pressure or body temperature [14,15]. The parameters can be transferred via a wireless or wired link to a microcontroller board, and diagnostic support systems may analyze and share information with specialists [16,17].
Numerous kinds of research have been used to enhance the accuracy of death risk stratification. They have used information from clinical notes or exams from many different sources, such as the electrocardiogram (ECG), 24-hour Holter, Exercise Test, Echocardiography, Cardiac Computed Tomography (CT), Cardiovascular Magnetic Resonance, and myocardial scintigraphy with single photon emission computed tomography (SPECT) or positron emission tomography (PET) [18,19,20]. However, most of them performed only classical linear analyses and did not fully evaluate the potential of combining different indices for the prognostication of patients with ChHD. This diversity of data and its origins is a favorable scenario for using feature selection (FS) techniques as a pre-processing step in ML to identify the most relevant attributes for the desired classification task. The main goal is to select the smallest possible subset of features appropriate for the problem, eliminating noise and redundant characteristics, preventing overfitting, and simplifying the development of intelligent systems [21,22].
This study offers the first evidence to support the use of ML techniques within Patients' Clinical Data and Cardiac Restitution Metrics (CRM) features extracted from ECG records as an adjuvant in the SCD risk assessment in ChHD. CRM features are critical and extensively researched in the context of heart disease [23,24,25]. These characteristics make the present study innovative since it uses information from non-invasive tests. Also, to the best of our knowledge, at this moment, only two previous studies have added information to these literature gaps*. One study has a limited approach, which only performed classical linear analyses and did not fully assess the potential of combining different parameters [8]. In another research, the authors extracted eight variables by applying heart rate variability (HRV) and heart rate turbulence (HRT) techniques over Holter-ECG records to investigate SCD in ChHD. The set of features was reduced with the forward and backward-stepwise approaches. The left ventricular ejection fraction (LVEF) was also analyzed with these variables. The study used ECG records of patients divided into SCD deaths and alive patients. The work used the k-nearest neighbors classifier, and a leave-one-out cross-validation [26]. However, this approach uses a limited sample of 82 individuals (20 SCD positives), compromising the generalization of the results, and the features are focused only on the metrics extracted from the ECG signal processing. Finally, because of the lack of sensitivity, specificity, and methodologies for leading with unbalanced data, it is impractical to replicate those works in different datasets.
*The search strategy for reviewing the literature used the PubMed/MEDLINE, Web of Science, and SciELO databases, and Periodic CAPES portal. The descriptors used were: "Chagas disease"; "Chagas heart disease"; "Electrocardiography"; "Sudden Cardiac Death"; "Machine Learning".
The main goal of this paper is to create an intelligent diagnostic support system for predicting SCD in ChHD patients. We will use an ML process to detect a high predisposition to SCD based on an optimized and reduced set of non-invasive test attributes. Other contributions of this work include: 1) using CRM features extracted from the ECG waveform by an automatic subsystem in conjunction with patient clinical data and different feature selection flows based on exhaustive testing; and 2) the searching for the best combination considering performance rates as high as possible and number of reduced features as low as possible.
SCD was defined as an abrupt collapse with documented loss of vital signs that might result in attempts to restore circulation (cardiopulmonary resuscitation). The etiology was only considered cardiac after excluding of SCDs due to vascular non-cardiac disease, acute non-cardiac illnesses, drug overdose, metabolic causes, or terminal disease [27].
Concerning the vital status of all participants, a probabilistic linkage was conducted with the Brazilian National Mortality System (SIM-Sistema de Informação sobre Mortalidade, in Portuguese) [28]. The linkage algorithm has been previously validated with a sensitivity and specificity of 94% and 91%, respectively [29]. SIM covers the entire population nationwide. Mortality data are considered reliable from a qualitative point of view, as accurate as those of other countries with a long tradition in these statistics [30]. When contact was possible, SCD data were obtained by directly interviewing participants' relatives. In addition, information about SCD was also obtained annually from University Hospital Clementino Fraga Filho of Federal University of Rio de Janeiro (HUCFF-UFRJ), Digital Registry and mobile Emergency Medical Service (SAMU), Brazil. SAMU follows the French pre-hospital care model, which provides on-scene care for individuals and not just transport to the hospital. The Brazilian government supports this, being available 24 hours a day, and has teams of health professionals, including doctors. The doctors have the responsibility to complete the death certificates. Individuals who were not identified in the SIM were sanctioned in February 2016 (the date of the link).
In the used dataset, the diagnosis of ChHD required at least two positive serology tests for T. cruzi antibodies (indirect hemagglutination, indirect immunofluorescence, or enzyme-linked immunosorbent assay) and electrocardiographic changes typical of Chagas disease [18].
The New York Heart Association (NYHA) classifies the extent of heart failure. It divides patients into four groups (I, II, III, and IV) based on physical activity limitation (dyspnea) [31]. The Rassi risk score estimates general death risk from ChHD patients in the next 5–10 years. Patients are classified into low, intermediate, and high risk of death based on the sum of the regression coefficient points for six risk factors: NYHA class III or IV (5 points), cardiomegaly on chest radiography (5 points), left ventricular systolic dysfunction (3 points), non-sustained ventricular tachycardia (NSVT) on 24-hour Holter monitoring (3 points), low QRS voltage on ECG (2 points), and male sex (2 points) [4]. Stages based on the severity of cardiac (Guideline 2005) involvement were determined for all participants before the SCD event. The stages are defined by: the presence of abnormalities within ECG (stage A); abnormal ECG and Echo, left ventricular ejection fraction (LVEF) > 45% without symptoms of heart failure (stage B1); abnormal ECG and Echo, LVEF < 45% without symptoms of heart failure (stage B2); abnormal ECG, Echo, LVEF < 45%, and symptoms of heart failure (stage C) [32].
Cardiac restitution is a natural myocardial property that translates the heart's ability to dynamically recover the time interval QT (action potential duration) from one beat to the next. It is an essential mechanical point where the cardiac cycle is shortened at faster heart rates to allow more effective contraction and relaxation for the efficient function of the cardiac pump. The restitution function is nonlinear, highly dynamic, occurs independently from underlying heart rate variability, and varies with normal and abnormal physiological conditions, including the autonomic state. On the electrocardiogram (ECG), this can be estimated by comparing the current QT interval to the previous TQ interval (diastolic interval) [23].
The QT interval is long at slow heart rates (long diastolic interval and TQ), but it shortens at high heart rates or ectopic beats (short diastolic interval and TQ). The magnitude of QT interval shortening with a reduction in the diastolic interval, characterizing the dynamics of electrical restitution, can be described by a plot of QT interval versus diastolic interval (action potential duration restitution), usually assuming a mono-exponential curve. If the action potential duration restitution (APDR) slope is <1, changes in TQ lead to relatively little change in QT with a balanced QT-TQ relationship. If the APDR slope is ≥1, a slight change in TQ would lead to more extensive changes in QT interval, reducing the action potential duration (APD) and leading to unstable re-entry and cardiac arrhythmias. APDR dynamic is recognized as one of the techniques for measuring the electrical restitution heterogeneity as a result of sympathetic activation [23]. For this work, some CRM was used and defined below. The acquisition process from ECG signals is shown in Figure 1 and explained in the next section.
● Tpeak-Tend: beat-to-beat temporal distance between the peak and the end of each T-wave in ECG or Holter signal. It is associated with the polarization and depolarization time variations between the heart's myocardial, endocardial, and epicardial layers.
● TendQ 5th Percentile (s) and TpeakQ 5th Percentile (s): statistical measures related, respectively, to the beat-to-beat temporal distance between the end (and the peak) of each T-wave and the beginning of the subsequent QRS complex, which are related to the relative refractory period. It has been proposed that arrhythmia vulnerability may increase due to the likelihood of re-entry as the relative refractory period approaches zero. The TQ 5th quantile is hypothesized to quantify the lower boundary where arrhythmia vulnerability is the greatest;
● Percentage (%) of beats with QTend/TendQ > 1 and Percentage (%) of beats with QTpeak/TpeakQ > 1: relative probability of occurrences where a measure of ventricular activity duration (QTend or QTpeak) is higher than a measure of the relative refractory period (TendQ or TpeakQ). This relationship has been proposed to be associated with increased arrhythmia vulnerability by the steepness of the restitution relationship. Therefore, the percentage of beats with a QT/TQ ratio greater than 1 reflects the relative time spent on the restitution curve where there may be increased instability;
● Upper 98% quantile of the Qtend/TendQ ratio and Upper 98% quantile of the QTpeak/TpeakQ ratio: statistical measures related to the most extreme beats for which the percentages QTend/TendQ and QTpeak/TpeakQ present the highest values. This measure reflects the magnitude of the steepness of the restitution relationship. The 98% quantile takes the most extreme beats with the highest likelihood of leading to arrhythmia into account.
The cardiac restitution metrics (CRM) acquisition process from ECG signals is shown in Figure 1 and explained in the next section.
Feature selection (FS) is choosing a better subset of attributes with more meaningful information for a given context. FS can reduce the set of attributes, remove noisy variables, detect the most relevant variables, or decrease the computational cost of training and testing by improving or without hurting the efficiency of the classification model [33]. This technique aims to obtain the best subset (s) of attributes within a more extensive set (X) of attributes, where s⊆ X. In a dataset with X features that are intended to obtain ranks for a given feature (Y), the FS problem can be defined as a search for a subspace of attributes (Rs) in an X-dimensional space (RX) that allows finding the best equivalent Y. The total number of subspaces is 2X, and the number of subspaces with dimensions equal to or less than s is ∑si=1(1X).
Due to many possibilities for finding the best subset, several FS techniques have been developed to decrease the complexity and computational cost. Filters, embedded, wrapper, hybrid, and ensemble are methods of FS techniques present in the literature. This research employed the following FS techniques: variance (VAR) and ANOVA as statistical filtering methods, select from model (SFM) as an embedded method, and recursive feature elimination (RFE) as a wrapper method [34,35]. VAR filter eliminates features with similar values and reduces the initial number of features. ANOVA enables the search for feature similarity. A slight variation in the means of two variables suggests that there is likely little difference in the data of the two variables, making them nearly identical and implying that one of them should be removed [36]. SFM technique uses the punctuation approaches capable of generating appropriate coefficients for each attribute that some machine learning (ML) classifiers employ. This argument ranks and selects a set number (N) of relevant features within a context. RFE uses the rank features based on the coefficients or feature importance attributes of the ML model. It removes a small number of features per loop, removing any existing dependencies and collinearities in the model. It finds a subset of features by starting with all of the features in the training dataset and successfully removing features until the desired number is reached [37].
The research's sample space was a dataset (clinical and cardiac examinations) from 315 patients followed in the protocolized clinical follow-up program of patients of the ChHD outpatient clinic of HUCFF-UFRJ between 1992 and 2016. Only patients who presented hospital episode statistics, ECG, echocardiogram, and Holter with a previous date closest to the SCD event were considered. Exclusion criteria were: patients with hypertensive heart disease, with ICD, cardiac resynchronization therapy or pacemaker implantation, and those with low-quality ECG-Holter signals. After the exclusion criteria, the sample space contains 218 patients with ECG-Holter. The coronary disease was excluded by symptom-limited stress test or invasive coronary angiography.
For the acquisition and use of the information in this research, the authorization process was approved by the local ethics committee, which waived the need for written informed consent under number 45360915.1.1001.5262 conforming to standards currently applied by the Brazilian National Committee for Research Ethics and the principles outlined in the Declaration of Helsinki. All patients received treatment following norms and protocols previously established by the National Health Surveillance Secretariat/Ministry of Health for ChHD, Brazil [18].
The experiments were performed with 218 patients, 96 (44%) men, and 122 (56%) women. As for the type of death, 77 (35%) had SCD in ChHD, and 141 (65%) did not have SCD in ChHD. The dataset contains 57 attributes constituting the sample space, from which 51 attributes are Clinical Data of Patients and 7 attributes are CRM extracted from ECG Data Processing, described in the next section. The data acquisition is displayed in the top left and bottom left frames of Figure 2. For a better understanding, the applied 57 attributes were grouped into five categories (Table 1), namely: clinical, heart tests, Cardiology Guidelines Classification (CGC), treatments, and cardiac restitution metrics (CRM). Six features from Patients' Clinical Data were discarded to ensure the patients' anonymity.
Group | Qnt | Attributes/Features | |
Clinical Data | 20 | Age at Holter, Gender, Body Mass Index (BMI), Cancer, Systemic Hypertension, Type 2 Diabetes Mellitus, Pacemaker (non-dependent), Syncope, Atrial Fibrillation/Flutter, Chronic Renal Insufficiency, Dyslipidemia, Coronary heart disease, Heart failure, Cerebrovascular accident, Peripheral vascular disease, thyroid-stimulating hormone (TSH), Smoking, Alcoholism, Sedentary lifestyle, and Sudden Cardiac Death (SDC). | |
Heart Tests | 23 | ECG | Primary Alteration, Interventricular Conduction Disorder (IVCDs), Atrioventricular Conduction Disorder, ≥ 3 second pause, Supraventricular Extrasystole (SVES), Ventricular Extrasystole (VE), Nonsustained Ventricular Tachycardia (nonsustained VT), and Electrical Inactive Area. |
ECHO | Left Atrial Diameter (LAD), Left Ventricular Diastolic Diameter (LVAD), Left Ventricular Systolic Diameter (LVSD), Ejection Fraction calculated by the method of Teichholz (EF-Teichholz), Classification, Diastolic Dysfunction, and Segmental dysfunction. | ||
Holter | Atrioventricular Conduction Disorder, Sinus Node Dysfunction, Atrial Fibrillation/Flutter, Average Heart Rate (AHR), Sustained Ventricular Tachycardia (SVT), Non-Sustained Ventricular Tachycardia (NSVT), Ventricular Extrasystole (VE), and Total Ventricular Extrasystole (TVE). | ||
Treatments | 3 | ICD, Ablations and Amiodarone. | |
Cardiology Guidelines Classification (CGC) | 4 | New York Heart Association (NYHA), Rassi Point, Rassi Escore, and Stage based on severity of cardiac involvement (2005 Guideline Classification). | |
Cardiac Restitution Metrics (CRM) | 7 | TendQ 5th Percentile (s), TpeakQ 5th Percentile (s), Percentage (%) of beats with Qtend/TendQ > 1, Percentage (%) of beats with QTpeak/TpeakQ > 1, Upper 98% quantil of the Qtend/TendQ ratio, Upper 98% quantil of the QTpeak/TpeakQ ratio, and Tpeak-Tend 5th-Percentile (ms). |
The 24-hour ECG-Holter signal recordings started at 8 a.m. According to a study by Fossa [24], cardiac restitution metrics were used. They were extracted from 04 h (dawn) to 08 h (morning) at a frequency of 128 Hz. In this period, it is acceptable that a more extensive adrenergic discharge occurs which causes heterogeneity in cardiac excitability with a high probability of SCD [38]. The software developed by Madeiro [39] was used to extract the seven CRM features by detecting beat-to-beat QT and TQ intervals related to ventricular activity and refractory period (Figure 1). The inter- and intra-operator variability of the software was evaluated in previous work [25].
Classification experiments with machine learning were divided into two scenarios. The first scenario (S1) used patients' clinical data and cardiac restitution metrics (CRM) features extracted from ECG-Holter. The second scenario (S2) used only patients' clinical data, excluding the CRM features. Booth scenarios ran the seven Feature Select (FS) flows (variance, ANOVA, SFM, RFE, Pipeline 1, Pipeline 2, and Pipeline 3) described in Section 2.3.2.
The implementation strategy for the classification process consisted of four steps: standardization process, feature selection (FS) flows, machine learning models (training and testing), and the choice of the ML model with the best performance for predicting SCD in ChHD, as shown in Figure 2. The coding was done in the Python programming language using the scikit-learn library [40].
As for the type, 37 of the attributes were binary, 15 scalar, and 5 categorical (2005 Guideline Classification, Rassi Score, NYHA, Classification, and Diastolic Dysfunction). The One Hot Encoding (OHE) technique transformed the values of the categorical features into binaries. This transformation was necessary because some ML algorithms work only with numerical values [41]. The data were normalized with the Min-Max and Z-score strategies to guarantee that all features were on the same scale [42].
In this study, seven different FS flows were employed. Four applied the FS techniques (VAR, ANOVA, SFM, and RFE) individually, and three flows combined them sequentially in proposals for hierarchical pipelines (Pipeline 1, Pipeline 2, and Pipeline 3). The sequence of FS techniques in the pipeline considered each technique's computational cost and application. The objective was to reduce the number of features between the techniques. Pipeline 1 has two steps, combining the variance (VAR) filter and select from model (SFM). Pipeline 2 has three steps, the first uses the VAR, the second uses the ANOVA filter, and the third one uses the SFM. Pipeline 3 combined VAR, ANOVA, and the RFE.
Each FS Flow generated a filtered dataset with a reduced number of features used as input for the classification process with machine learning algorithms described in the next section. All seven FS flows and their filtered datasets are displayed in the center frames of Figure 2.
A grid of combinations was run on each FS flow to find the best output. The exhaustive tests were carried out with various configurations of each FS technique. VAR was tested with ten different threshold values (0.01, 0.02, ..., 0.09, 1). ANOVA was executed with 15 different sets of best attributes (k = 10, 13... 46, 49) with step = 3. The SFM and RFE were tested in Logistic Regression (LR), Balanced Random Forest (BRF), and Support Vector Machine (SVM). SFM was tested with eight different values (threshold = 0.2, 0.3, ..., 0.8, 0.9) and RFE with 16 subsets, varying the number of features from 5 to 16, with a step of 1 feature.
The classification model for SCD and non-SCD in ChHD was constructed using eight ML algorithms: 1) K-Nearest Neighbors (KNN), 2) Gradient Boosting (GB), 3) Logistic Regression (LR), 4) Naive Bayes (NB), 5) Support Vector Machine (SVM), 6) Balanced Random Forest (BRF), 7) Multilayer Perceptron (MLP), and 8) Catboost. Different types were used, such as probabilistic (LR and NB), neural networks (MLP), and tree algorithms (BRF and CatBoost).
For each filtered data set, a holdout of 80% was used for the training base and 20% for the test base. Tables 2 and 3 indicate the results of the test bases. ML algorithms were hyper-parameterized, 10-fold cross-validation techniques were used, and each was run 30 times to calculate the averages of six evaluation metrics: accuracy (ACC), the area under the ROC curve (AUC), precision, recall, F1-score, and their respective standard deviations. Due to the specificity of the data, this work uses only real data without using synthetic data generation techniques like SMOTE [43]. In all experiments, the algorithms were run with the following hyperparameters [40]:
FS Flow | Algorithm | Features | ACC | AUC | Recall | Precision | F1 |
ANOVA | NB | 24 | 76.36 ± 6.55 | 78.53 ± 6.5 | 86.46 ± 8.2 | 63.11 ± 7.09 | 72.79 ± 6.9 |
Pipeline 1 | NB | 16 | 77.12 ± 5.94 | 79.66 ± 5.8 | 88.96 ± 9.2 | 63.70 ± 6.64 | 73.93 ± 6.3 |
Pipeline 2 | NB | 6 | 77.80 ± 6.47 | 78.99 ± 6.9 | 83.33 ± 14.5 | 66.67 ± 9.29 | 72.88 ± 8.6 |
Pipeline 3 | NB | 13 | 77.80 ± 6.08 | 80.55 ± 5.6 | 90.63 ± 7.3 | 64.32 ± 7.42 | 74.98 ± 6.2 |
RFE | NB | 13 | 77.73 ± 6.44 | 79.69 ± 5.7 | 86.88 ± 10.2 | 65.59 ± 8.39 | 74.08 ± 6.2 |
SFM | NB | 18 | 74.62 ± 11.33 | 77.83 ± 8.7 | 89.58 ± 7.0 | 62.34 ± 10.25 | 72.82 ± 7.7 |
VAR | BRF | 56 | 79.17 ± 5.47 | 80.28 ± 5.1 | 84.38 ± 8.0 | 67.78 ± 7.77 | 74.78 ± 5.8 |
FS Flow | Algorithm | Features | ACC | AUC | Recall | Precision | F1 |
ANOVA | NB | 29 | 71.64 ± 5.83 | 75.57 ± 4.6 | 83.54 ± 9.6 | 47.66 ± 6.79 | 60.18 ± 5.4 |
Pipeline 1 | NB | 10 | 73.28 ± 10.7 | 77.97 ± 7.1 | 87.50 ± 10.0 | 51.12 ± 11.0 | 63.49 ± 8.4 |
Pipeline 2 | NB | 4 | 71.96 ± 10.54 | 77.70 ± 6.8 | 89.38 ± 9.0 | 49.34 ± 9.60 | 62.75 ± 7.6 |
Pipeline 3 | BRF | 5 | 78.34 ± 5.86 | 80.63 ± 5.7 | 89.58 ± 8.3 | 64.93 ± 7.26 | 75.02 ± 6.3 |
RFE | NB | 11 | 74.87 ± 6.17 | 78.14 ± 5.7 | 84.79 ± 12.7 | 51.41 ± 7.78 | 63.32 ± 7.0 |
SFM | NB | 7 | 74.23 ± 9.58 | 79.78 ± 7.0 | 91.04 ± 8.2 | 51.53 ± 10.09 | 65.14 ± 8.4 |
VAR | BRF | 56 | 79.37 ± 5.85 | 80.40 ± 5.6 | 82.50 ± 8.6 | 57.34 ± 7.86 | 67.30 ± 7.0 |
● KNN: numeral of neighbors (3, 5, 7, 9, 11);
● GB: learning_rate (0.01, 0.025, 0.05, 0.075, 0.1, 0.15, 0.2), min_samples_split (12 samples spaced evenly from the range starts from 0.1, ends at 0.5), min_samples_leaf (12 samples spaced evenly from the range starts from 0.1, ends at 0.5), max_depth (3, 5, 8), max_features (log2, sqrt), criterion_quality_split (friedman_mse, mean_squared _error), subsample (0.5, 0.618, 0.8, 0.85, 0.9, 0.95, 1.0), and n_estimators (10, 30, 70, 100);
● LR: C - regularization parameter (0, 0.01, 0.1, 1.0, 10, 100);
● NB: 100 samples spaced evenly on a log scale (starts from 0, ends at 9.);
● SVM: kernel (rbf, linear), C - regularization parameter (2i−5, for i from 0 to 21, step 2), and gamma (only RBF Kernel: 2i−15, for i from 0 to 19, step 2);
● BRF: criterion_quality_split (entropy, gini); max_depth (10 samples spaced evenly from the range starts from 10, ends at 1200), max_features (square root, binary logarithm, and all features), min_samples_leaf (4, 6, 8, and 12), min_samples_split (5, 7, 10, and 14), and n_estimators (10 samples spaced evenly from the range starts from 15, ends at 1200);
● MLP: hidden_layer_sizes (tests with 3, 2, and 1 hidden layers, varying the number of neurons in each, e.g., (200, 50, 30), (100, 50, 10), (100, 50), (200, 100), (500, 250), (20, ), (50, ), (100, ), (10, ), (200, )), activation_function (tangent, rectified linear unit), solver_weight_optimization (stochastic gradient descent, Adam), alpha (0.0001, 0.005, 0.05) and learning_rate (constant, adaptive)
● Catboost: verbose: (0), depth (3, 4, 5, 8, 12), learning_rate (0.01, 0.025, 0.05, 0.075, 0.1, 0.15, 0.2), l2_leaf_reg (1, 4, 9), and iterations (70, 100, 150, 200, 300).
For each of the datasets filtered by the seven FS flows (variance, ANOVA, SFM, RFE, Pipeline 1, Pipeline 2, and Pipeline 3) used in this study, all 8 ML algorithms were executed, and the results of their metrics (ACC, AUC, recall, precision, and F1) were recorded. Altogether, the results of the 56 classification models (7 FS flows × 8 ML algorithms) were obtained and compared. Figure 2 shows the ML models in the second-to-rightmost frame.
Only the best-performing algorithms in each FS flow (variance, ANOVA, SFM, RFE, Pipeline 1, Pipeline 2, and Pipeline 3) were selected and compared. Table 2 shows the results obtained in scenario 1, and Table 3 shows the results obtained in scenario 2. The recall (sensitivity) was the principal metric for the choice because it identifies the highest number of hits for patients prone to SCD in ChHD. Accuracy and the smallest number of used attributes were also considered. Accuracy keeps patients from getting unnecessary treatments, and having the fewest possible attributes makes the proposed model easy to use.
The test base (20% dataset) results of the machine learning models to predict SCD in patients with ChHD are presented. Table 2 shows the mean and standard deviation values of the metrics of the best-performing algorithms for each of the FS methods in scenario 1 (S1), including 7 CRM features extracted from ECG-Holter (57 features in total). Noting that VAR removes a single feature, its performance is comparable to that of the full-feature case, i.e., no-use feature selection. With a combination of feature selection techniques within flows, the correlated features were removed, leaving only those with little or no correlation. Except for using only the variance filter (VAR) with BRF, Naive Bayes (NB) performed the best in almost all flows. The NB algorithm classification model found the best results, which was most suited to scenario 1[44].
Table 2 displays that the proposed hierarchical technique Pipeline 3 with NB using 13 attributes achieved the best results for recall, AUC, and F1-Score, with particular attention to the 90.63% recall value. Other proposed hierarchical techniques, Pipeline 1, and SFM, both with NB, had very similar results for recall but with a large number of features. The VAR method with the BRF algorithm obtained 67.78% precision, but the other methods have similar results in this metric.
One can observe that the Pipeline 3 hierarchical technique with NB had the second-best result for precision using only six attributes. The proposed Pipeline 3, which combines the filter variance, ANOVA, and RFE techniques, improved recall by more than 4% and decreased its standard deviation by more than 28% compared to the result of using RFE alone.
Table 4 presents the classification models and the number of features per group. The model with the Pipeline 3 that obtained the best performance for recall selected 13 attributes:
FS Flow | Algorithm | Clinica Data | Classification | Treatments | CRM | Heart Tests | Total |
ANOVA | NB | 7 | 4 | 2 | 1 | 10 | 24 |
Pipeline 1 | NB | 3 | 3 | 2 | 2 | 6 | 16 |
Pipeline 2 | NB | 1 | 2 | 0 | 0 | 3 | 6 |
Pipeline 3 | NB | 0 | 4 | 1 | 1 | 7 | 13 |
RFE | NB | 3 | 1 | 1 | 3 | 5 | 13 |
SFM | NB | 3 | 4 | 2 | 3 | 6 | 18 |
VAR | BRF | 19 | 4 | 3 | 7 | 22 | 56 |
● 4 Cardiology Guidelines Classification (CGC): NYHA, Rassi points, Rassi score, 2005 Guideline Classification;
● 1 Treatment: Amiodarone;
● 1 CRM: Tpeak-Tend 5th-Percentile (ms);
● 7 Heart Tests: NSVT, TVE, LVAD, F-Teichholz, Classification, Diastolic Dysfunction, and Segmental dysfunction
The Pipeline 2 model with the NB selected 6 attributes:
● 1 Clinical: Sincope;
● 2 Cardiology Guidelines Classification (CGC): Rassi score, 2005 Guideline;
● 3 Heart Tests: NSVT, TVE, Classification.
One can see that CRM feature are present in the subset of best features of almost all of the SCD classification models. In the RFE and SFM methods, 3 of the seven cardiac restitution metrics were selected among the features of the highest relevance: TendQ 5th Percentile (s), Percentage (%) of beats with Qtend/TendQ > 1, and Tpeak-Tend 5th-Percentile (ms).
The results indicate an optimized set of attributes for using a possible classification model of SCD in patients with ChHD to support the therapeutic decision-making process. The "Tpeak-Tend 5th-Percentile (ms)" was selected among the most relevant features, showing the relevance of CRM calculation from ECG signals. Two reasons can probably explain this. First, it is well established that the electrophysiological heterogeneity resulting from the cardiac remodeling induced by autonomic dysfunction quantitatively favors the presence of fast ventricular arrhythmias and SCD in ChHD. Furthermore, these individuals do not have the parasympathetic protection [6], and the increase in norepinephrine levels [45] might be responsible for fast ventricular arrhythmia. The second possible explanation is that the vulnerability associated with the CRMs is not solely a function of a static set of electrophysiological properties but also depends on changes that occur over multiple time scales ranging from seconds to hours [46].
Table 5 displays studies relating to SCD in the context of ChHD and general cardiology (non-ChHD). Due to the scarcity of studies on SCD in ChHD, works with ML related to SCD in non-ChHD were included. All the studies satisfy the criteria of predicting SCD in patients in a way that goes back more than a day and with time spent watching the patients [8,13,26]. Due to a lack of access to a universal health system and underreporting of the disease, the majority of non-ChHD studies have a larger number of participants (N) than ChHD research, demonstrating the importance of N in this search. In the non-ChHD context, Random Forest (RF) is one of the most commonly used ML models. A variation of this model (Balanced RF) also appears in VAR Fs Flow, shown in Table 4. In relation to the number of features used to predict SCD, in the context of ChHD, the model by Souza et al. [8] uses only 4, do not use ML, and has several limitations. Alberto [26] used eight features but had only 82 participants. The no-ChHD research has a variance in the number of features (4, 7, 8, 9, 10, 12, 15, 26, 61, 72). Pipeline 2, our second-best result, used six features and is the third method with a smaller number of features, including ChHD and non-ChHD contexts. A possible hypothesis for some works using fewer features is the amount of data available, which does not happen in the context of SCD in ChHD.
Study | Participants | ChHD Context | Machine Learning Model | Features | |
Souza et al. [8] | 373 | Yes | Classical Linear Regression (Not using ML) | 4 | |
Tse et al. [47] | 376 | No | Non-negative matrix factorization | 4 | |
Lyon et al. [48] | 123 | No | Density-based clustering | 7 | |
Nakajima et al. [49] | 526 | No | Random Forest, KNN, Gradient Boosted | 8 | |
Alberto et al. [26], | 82 | Yes | KNN | 8 | |
Shakibfar et al. [50] | 19, 935 | No | Random Forest | 9 | |
Atallah et al. [51] | 288 | No | Random Forest | 10 | |
Rodriguez et al.[52] | 140 | No | SVM | 12 | |
This Work (Pipeline 3) | 218 | Yes | Naive Bayes | 13 | |
Vergara et al. [53] | 502 | No | Random Forest | 15 | |
Lee et al. [54] | 516 | No | SVM | 26 | |
Zoni-Berisso et al. [55] | 404 | No | Mandansky artificial neural network | 61 | |
Goldstein et al. [56] | 1628 | No | Random Forest and KNN | 72 |
The works shown in Table 5 have their results presented by several metrics (accuracy, AUC, sensitivity, specificity, or F1-score) [13]. For comparative purposes, Table 6 shows only the perfomance of these works with the same parameter (sensitivity) as the current study. It is observed that the results obtained in this present research are similar to those obtained in non-ChHD context studies, presenting the second highest sensitivity. Despite the differences in terms of number of features, number of participants, and ML method applied, our results are relevant to the scenario of SCD in ChHD.
Study | ChHD Context | Recall (Sensitivity) |
Tse et al. [47] | No | 70.00% |
Zoni-Berisso et al. [55] | No | 96.00% |
Lee et al. [54] | No | 89.00% |
Atallah et al. [51] | No | 88.40% |
This Work (Pipeline 3) | Yes | 90.63% |
In scenario 2, excluded 7 CRM features extracted from ECG-Holter (remaining 51 features in total), the experiments were repeated, running the eight algorithms on each FS technique. Table 3 shows the results for the test base (20% dataset). The NB algorithm, similar to scenario 1, performed better than the other ML algorithms in most FS flows. BRF performed better in the models using only the variance and the Pipeline 3 hierarchical techniques. Both algorithms ran with the same hyperparameters as in scenario 1. The results in S1 and S2 scenarios of all algorithms in each FS technique are available in this online document.
It can be seen from Table 3 that pipelines Pipeline 2 with NB, Pipeline 3 with BRF, and SFM with BRF had similar recall results in this second scenario (S2). The model with Pipeline 3 had the best accuracy result, with a value of 64.93%, improving the second-best result by 7%. It still had the best F1-score (75.02%), the best AUC (78.34%), and selected five features (1 Clinical, 2 Heart Tests, and 2 Classification), being the second smallest subset of attributes. Compared to S1, there was a significant decrease in precision and F1-score in all approaches run in S2, except Pipeline 3 with BRF. One hypothesis may be the absence of the use of CRM features.
The ROC curves were plotted in scenario 1 (Figure 3a) and scenario 2 (Figure 3b). The graphs show the ROC curve of one of the 30 repetitions of the best-performed ML algorithm in each FS technique, illustrated in Tables 2 and 3. The values obtained are within the statistical range of the metric.
The strengths of our study include the vital status of each participant determined by SIM and the use of artificial intelligence. Our work is perhaps best understood in the context of its limitations. Lacks external validity. Further studies are desired to evaluate its incorporation in the clinical. Another possible drawback is the question of how far our findings could be applied to the general CD population. Although our patients constituted an urban cohort from a CD reference center, their baseline characteristics were similar to those reported from rural [57] and urban [8] cohorts of Chagas' endemic areas. Thus, we believe that our cohort represents the factual scenario in a population with CD, where risk scores and prognostic evaluations are often studied.
This research presents an SCD Multiparametric Classification System for Chagas Heart Disease'sre Patients based on clinical data and 24-hour ECG Monitoring, which achieved 90.63% recall (sensitivity) and 80.55% AUC with the proposed approach called Pipeline 3 using Naive Bayes (NB) machine learning algorithm. This proposed approach combines three different feature selection techniques (VAR, ANOVA, and RFE) to find an optimized relevant subset of 13 features (among a total of 57 attributes) as input to a classification algorithm. This result is a significant achievement in addressing a requirement from the team of medical specialists, who considered the number of original attributes too large and hard to interpret. The Tpeak-Tend 5th-Percentile (ms) feature was selected among the most relevant, showing the relevance of the CRM feature from ECG signals.
Our findings suggest that using the ML technique in ChHD may be an additional helpful tool to identify individuals with increased risk for SCD who may benefit from ICD implantation, possibly improving the selection of individuals for ICD. Our results have potential socio-economic implications when limited resources have to be allocated to the appropriate patients and may help guide ICD implantation in the Brazilian Unified Health System (SUS/Brazil) and among doctors in other countries highly affected by Chagas disease. Future research may conduct experiments with the entire 24-hour ECG-holter signal. Additionally, we may use alternative methods to address the problem, such as extracting other features from ECG signals (e.g., heart rate variability metrics) and various types of deep learning, such as convolutional neural networks (CNN), recurrent neural networks (RNN), or long short-term memory (LSTM).
The authors thank the support of the Federal Institute of Education and Technology of Ceará, Ceará Foundation for Scientific and Technological Development Support - Funcap (PS1-0186-00439.01.00/21, BP4-0172-00075.01.00/20), and the Brazilian National Council for Scientific and Technological Development (CNPQ).
The authors declare no conflict of interest.
[1] | L. Kaufman, P.J. Rousseeuw, Finding Groups in Data: An Introduction to Cluster Analysis, Wiley-Interscience, New York, 2009. |
[2] | J. C. Bezdek, Pattern Recognition with fuzzy objective function algorithms, Plenum Press, New York, 1981. |
[3] | D. Jiang, C. Tang and A. Zhang, Cluster analysis for gene expression data: A survey, IEEE Trans. Knowl. Data Eng.,16 (2004), 1370-1386. |
[4] | J. M. T. Wu, C. W. Lin, P. Fournier-Viger, et al., The density-based clustering method for privacy-preserving data mining, Math. Biosci. Eng., 16 (2019), 1718-1728. |
[5] | M. S. Yang, C. Y. Lai and C. Y. Lin, A robust EM clustering algorithm for Gaussian mixture models, Pattern Recognit., 45 (2012), 3950-3961. |
[6] | A. K. Jain, Data clustering: 50 years beyond k-means, Pattern Recognition Lett., 31 (2010), 651-666. |
[7] | A. Baraldi and P. Blonda, A survey of fuzzy clustering algorithms for pattern recognition-part I and part II, IEEE Trans. Syst. Man Cybern. B, 29 (1999), 778-785. |
[8] | M. S. Yang and Y. Nataliani, Robust-learning fuzzy c-means clustering algorithm with unknown number of clusters, Pattern Recogni t., 71 (2017), 45-59. |
[9] | R. Krishnapuram and J. M. Keller, A possibilistic approach to clustering, IEEE Trans. Fuzzy Syst., 1 (1993), 98-110. |
[10] | M. S. Yang, S. J. Chang-Chien and Y. Nataliani, A fully-unsupervised possibilistic c-means clustering method, IEEE Access, 6 (2018), 78308-78320. |
[11] | A. P. Dempster, N. M. Laird and D. B. Rubin, Maximum likelihood from incomplete data via the EM algorithm (with discussion), J. R. Stat. Soc. Ser. B, 39 (1977), 1-38. |
[12] | W. Pan and X. Shen, Penalized model-based clustering with application to variable selection, J. Mach. Learn. Res., 8 (2007), 1145-1164. |
[13] | R. Tibshirani, Regression shrinkage and selection via the Lasso, J. R. Stat. Soc. Ser. B, 58 (1996), 267-288. |
[14] | J. D. Banfield and A. E. Raftery, Model-based Gaussian and non-Gaussian Clustering, Biometrics, 49 (1993), 803-821. |
[15] | A. J. Scott and M. J. Symons, Clustering methods based on likelihood ratio criteria, Biometrics, 27 (1971), 387-397. |
[16] | M. J. Symons, Clustering criteria and multivariate normal mixtures, Biometrics,37 (1981), 35-43. |
[17] | R. Wehrens, L. M. C. Buydens, C. Fraley, et al., Model-based clustering for image segmentation and large datasets via sampling, J. Classif., 21 (2004), 231-253. |
[18] | W. C. Young, A. E. Raftery and K. Y. Yeung, Model-based clustering with data correction for removing artifacts in geneexpression data, Ann. Appl. Stat., 11 (2017), 1998-2026. |
[19] | T. Akilan, Q. M. J. Wu and Y. Yang, Fusion-based foreground enhancement for background subtraction using multivariate multi-model Gaussian distribution, Inf. Sci., 430-431 (2018), 414-431. |
[20] | M. S. Yang, S. J. Chang-Chien and Y. Nataliani, Unsupervised fuzzy model-based Gaussian clustering,Inf. Sci., 481 (2019), 1-23. |
[21] | L. A. Zadeh, Fuzzy sets, Inf. Control, 8 (1965), 338-353. |
[22] | M. S. Yang and Y. Nataliani, A feature-reduction fuzzy clustering algorithm based on feature-weighted entropy,IEEE Trans. Fuzzy Syst., 26(2018), 817-835. |
[23] | K. Voevodski, M. F. Balcan, H. Röglin, et al., Active clustering of biological sequences, J. Mach. Learn. Res., 13 (2012), 203-225. |
[24] | D. Gaweł and K. Fujarewicz, On the sensitivity of feature ranked lists for large-scale biological data, Math. Biosci. Eng., 10 (2013), 667-690. |
[25] | J. Xiong, Essential Bioinformatics, Cambridge University Press, New York, 2006. |
[26] | R. Jiang, X. Zhang, M. Q. Zhang, Basics of Bioinformatics, Springer-Verlag Berlin An, 2013. |
[27] | E. H. Ruspini, A new approach to clustering,Inf. Control, 15 (1969), 22-32. |
[28] | D. M. Witten and R. Tibshirani, A framework for feature selection in clustering, J. Am. Stat. Assoc.,105 (2010), 713-726. |
[29] | E. A. Castro and X. Pu, A simple approach to sparse clustering, Comput. Stat. Data Anal.,105 (2017), 217-228. |
[30] | X. Qiu, Y. Qiu, G. Feng, et al., A sparse fuzzy c-means algorithm base on sparse clustering framework, Neurocomputing,157 (2015), 290-295. |
[31] | X. Chang, Q. Wang, Y. Liu, et al., Sparse regularization in fuzzy c-means for high-dimensional data clustering,IEEE Trans. Cybern., 47 (2017), 2616-2627. |
[32] | T. Hastie, R. Tibshirani and M. Wainwright, Statistical Learning with Sparsity: The lasso and Generalization, Chapman and Hall/CRC press, New York, (2015). |
[33] | C. L. Blake and C. J. Merz, UCI repository of machine learning database, a huge collection of artificial and real-world data sets, (1988). |
[34] | N. K. Phan, Biological therapy: A new age of cancer treatment, Biomed. Res. Ther., 1 (2014), 32-34. |
[35] | Global Health Observatory (GHO) data, World Health Organization, Geneva, 2018. Available from: https://www.who.int/gho/en/. |
[36] | F. Bray, J. Ferlay, I. Soerjomataram, et al., A. Jemal, Global cancer statistics 2018: GLOBOCAN estimates of incidence and mortality worldwide for 36 cancers in 185 countries, CA A Cancer J. Clin., 68 (2018), 394-424. |
[37] | D. N. K. Boulos and R. R. Ghali, Awareness of breast cancer among female students at Ain Shams University, Egypt, Glob. J. Health Sci., 6 (2014), 154-161. |
[38] | K. McPherson, C. M. Steel and J. M. Dixon, Breast cancer-epidemiology, risk factors, and genetics, BMJ, 321 (2000), 624-628. |
[39] | R. R. Janghel, A. Shukla, R. Tiwari, et al., Intelligent decision support system for breast cancer, International Conference in Swarm Intelligence, Beijing, China, 2010, 351-358. Available from: https://link_springer.gg363.site/chapter/10.1007/978-3-642-13498-2_46#citeas. |
[40] | W. N. Street, W. H. Wolberg and O. L. Mangasarian, Nuclear feature extraction for breast tumor diagnosis, Biomedical image processing and biomedical visualization, 1905 (1993), 861-870. Available from: https://doi.org/10.1117/12.148698. |
[41] | A. R. Marley and H. Nan, Epidemiology of colorectal cancer, Int. J. Mol. Epidemiol. Genet., 7 (2016), 105-114. |
[42] | M. Arnold, M. S. Sierra, M. Laversanne, et al., Global patterns and trends in colorectal cancer incidence and mortality, Gut, 66 (2017), 683-691. |
[43] | Cancer Stat Facts: Leukemia, National Cancer Institute, Surveillance Epidemiology and End Results Program, 2006-2010. Available from: http://seer.cancer.gov/statfacts/html/leuks.html. |
[44] | A. S. Davis, A. J. Viera and M. D. Mead, Leukemia: An overview for primary care, Am. Fam. Physician, 89 (2014), 731-738. |
[45] | T. R. Golub, D. K. Slonim, P. Tamayo, et al., Molecular classification of cancer: Class discovery and class prediction by gene expression monitoring, Science,286 (1999), 531-537. |
1. | Keyue Yan, Simon Fong, Tengyue Li, Qun Song, Multimodal Machine Learning for Prognosis and Survival Prediction in Renal Cell Carcinoma Patients: A Two-Stage Framework with Model Fusion and Interpretability Analysis, 2024, 14, 2076-3417, 5686, 10.3390/app14135686 | |
2. | Roberto Coury Pedrosa, João Paulo do Vale Madeiro, Alex C. Alberto, Gabriel A. Limeira, Basílio de Bragança Pereira, Emília Matos do Nascimento, Fernando Soares Schlindwein, Ghulam Andre Ng, Risk stratifier for sudden cardiac death beyond the left ventricular ejection fraction in Chagas cardiomyopathy, 2024, 47, 0147-8389, 312, 10.1111/pace.14908 | |
3. | Isabelly P. da Costa, Bruno M. P. Takazono, Carlos H. L. Cavalcante, João P. V. Madeiro, Roberto C. Pedrosa, 2025, Chapter 16, 978-3-031-79031-7, 224, 10.1007/978-3-031-79032-4_16 |
Group | Qnt | Attributes/Features | |
Clinical Data | 20 | Age at Holter, Gender, Body Mass Index (BMI), Cancer, Systemic Hypertension, Type 2 Diabetes Mellitus, Pacemaker (non-dependent), Syncope, Atrial Fibrillation/Flutter, Chronic Renal Insufficiency, Dyslipidemia, Coronary heart disease, Heart failure, Cerebrovascular accident, Peripheral vascular disease, thyroid-stimulating hormone (TSH), Smoking, Alcoholism, Sedentary lifestyle, and Sudden Cardiac Death (SDC). | |
Heart Tests | 23 | ECG | Primary Alteration, Interventricular Conduction Disorder (IVCDs), Atrioventricular Conduction Disorder, ≥ 3 second pause, Supraventricular Extrasystole (SVES), Ventricular Extrasystole (VE), Nonsustained Ventricular Tachycardia (nonsustained VT), and Electrical Inactive Area. |
ECHO | Left Atrial Diameter (LAD), Left Ventricular Diastolic Diameter (LVAD), Left Ventricular Systolic Diameter (LVSD), Ejection Fraction calculated by the method of Teichholz (EF-Teichholz), Classification, Diastolic Dysfunction, and Segmental dysfunction. | ||
Holter | Atrioventricular Conduction Disorder, Sinus Node Dysfunction, Atrial Fibrillation/Flutter, Average Heart Rate (AHR), Sustained Ventricular Tachycardia (SVT), Non-Sustained Ventricular Tachycardia (NSVT), Ventricular Extrasystole (VE), and Total Ventricular Extrasystole (TVE). | ||
Treatments | 3 | ICD, Ablations and Amiodarone. | |
Cardiology Guidelines Classification (CGC) | 4 | New York Heart Association (NYHA), Rassi Point, Rassi Escore, and Stage based on severity of cardiac involvement (2005 Guideline Classification). | |
Cardiac Restitution Metrics (CRM) | 7 | TendQ 5th Percentile (s), TpeakQ 5th Percentile (s), Percentage (%) of beats with Qtend/TendQ > 1, Percentage (%) of beats with QTpeak/TpeakQ > 1, Upper 98% quantil of the Qtend/TendQ ratio, Upper 98% quantil of the QTpeak/TpeakQ ratio, and Tpeak-Tend 5th-Percentile (ms). |
FS Flow | Algorithm | Features | ACC | AUC | Recall | Precision | F1 |
ANOVA | NB | 24 | 76.36 ± 6.55 | 78.53 ± 6.5 | 86.46 ± 8.2 | 63.11 ± 7.09 | 72.79 ± 6.9 |
Pipeline 1 | NB | 16 | 77.12 ± 5.94 | 79.66 ± 5.8 | 88.96 ± 9.2 | 63.70 ± 6.64 | 73.93 ± 6.3 |
Pipeline 2 | NB | 6 | 77.80 ± 6.47 | 78.99 ± 6.9 | 83.33 ± 14.5 | 66.67 ± 9.29 | 72.88 ± 8.6 |
Pipeline 3 | NB | 13 | 77.80 ± 6.08 | 80.55 ± 5.6 | 90.63 ± 7.3 | 64.32 ± 7.42 | 74.98 ± 6.2 |
RFE | NB | 13 | 77.73 ± 6.44 | 79.69 ± 5.7 | 86.88 ± 10.2 | 65.59 ± 8.39 | 74.08 ± 6.2 |
SFM | NB | 18 | 74.62 ± 11.33 | 77.83 ± 8.7 | 89.58 ± 7.0 | 62.34 ± 10.25 | 72.82 ± 7.7 |
VAR | BRF | 56 | 79.17 ± 5.47 | 80.28 ± 5.1 | 84.38 ± 8.0 | 67.78 ± 7.77 | 74.78 ± 5.8 |
FS Flow | Algorithm | Features | ACC | AUC | Recall | Precision | F1 |
ANOVA | NB | 29 | 71.64 ± 5.83 | 75.57 ± 4.6 | 83.54 ± 9.6 | 47.66 ± 6.79 | 60.18 ± 5.4 |
Pipeline 1 | NB | 10 | 73.28 ± 10.7 | 77.97 ± 7.1 | 87.50 ± 10.0 | 51.12 ± 11.0 | 63.49 ± 8.4 |
Pipeline 2 | NB | 4 | 71.96 ± 10.54 | 77.70 ± 6.8 | 89.38 ± 9.0 | 49.34 ± 9.60 | 62.75 ± 7.6 |
Pipeline 3 | BRF | 5 | 78.34 ± 5.86 | 80.63 ± 5.7 | 89.58 ± 8.3 | 64.93 ± 7.26 | 75.02 ± 6.3 |
RFE | NB | 11 | 74.87 ± 6.17 | 78.14 ± 5.7 | 84.79 ± 12.7 | 51.41 ± 7.78 | 63.32 ± 7.0 |
SFM | NB | 7 | 74.23 ± 9.58 | 79.78 ± 7.0 | 91.04 ± 8.2 | 51.53 ± 10.09 | 65.14 ± 8.4 |
VAR | BRF | 56 | 79.37 ± 5.85 | 80.40 ± 5.6 | 82.50 ± 8.6 | 57.34 ± 7.86 | 67.30 ± 7.0 |
FS Flow | Algorithm | Clinica Data | Classification | Treatments | CRM | Heart Tests | Total |
ANOVA | NB | 7 | 4 | 2 | 1 | 10 | 24 |
Pipeline 1 | NB | 3 | 3 | 2 | 2 | 6 | 16 |
Pipeline 2 | NB | 1 | 2 | 0 | 0 | 3 | 6 |
Pipeline 3 | NB | 0 | 4 | 1 | 1 | 7 | 13 |
RFE | NB | 3 | 1 | 1 | 3 | 5 | 13 |
SFM | NB | 3 | 4 | 2 | 3 | 6 | 18 |
VAR | BRF | 19 | 4 | 3 | 7 | 22 | 56 |
Study | Participants | ChHD Context | Machine Learning Model | Features | |
Souza et al. [8] | 373 | Yes | Classical Linear Regression (Not using ML) | 4 | |
Tse et al. [47] | 376 | No | Non-negative matrix factorization | 4 | |
Lyon et al. [48] | 123 | No | Density-based clustering | 7 | |
Nakajima et al. [49] | 526 | No | Random Forest, KNN, Gradient Boosted | 8 | |
Alberto et al. [26], | 82 | Yes | KNN | 8 | |
Shakibfar et al. [50] | 19, 935 | No | Random Forest | 9 | |
Atallah et al. [51] | 288 | No | Random Forest | 10 | |
Rodriguez et al.[52] | 140 | No | SVM | 12 | |
This Work (Pipeline 3) | 218 | Yes | Naive Bayes | 13 | |
Vergara et al. [53] | 502 | No | Random Forest | 15 | |
Lee et al. [54] | 516 | No | SVM | 26 | |
Zoni-Berisso et al. [55] | 404 | No | Mandansky artificial neural network | 61 | |
Goldstein et al. [56] | 1628 | No | Random Forest and KNN | 72 |
Study | ChHD Context | Recall (Sensitivity) |
Tse et al. [47] | No | 70.00% |
Zoni-Berisso et al. [55] | No | 96.00% |
Lee et al. [54] | No | 89.00% |
Atallah et al. [51] | No | 88.40% |
This Work (Pipeline 3) | Yes | 90.63% |
Group | Qnt | Attributes/Features | |
Clinical Data | 20 | Age at Holter, Gender, Body Mass Index (BMI), Cancer, Systemic Hypertension, Type 2 Diabetes Mellitus, Pacemaker (non-dependent), Syncope, Atrial Fibrillation/Flutter, Chronic Renal Insufficiency, Dyslipidemia, Coronary heart disease, Heart failure, Cerebrovascular accident, Peripheral vascular disease, thyroid-stimulating hormone (TSH), Smoking, Alcoholism, Sedentary lifestyle, and Sudden Cardiac Death (SDC). | |
Heart Tests | 23 | ECG | Primary Alteration, Interventricular Conduction Disorder (IVCDs), Atrioventricular Conduction Disorder, ≥ 3 second pause, Supraventricular Extrasystole (SVES), Ventricular Extrasystole (VE), Nonsustained Ventricular Tachycardia (nonsustained VT), and Electrical Inactive Area. |
ECHO | Left Atrial Diameter (LAD), Left Ventricular Diastolic Diameter (LVAD), Left Ventricular Systolic Diameter (LVSD), Ejection Fraction calculated by the method of Teichholz (EF-Teichholz), Classification, Diastolic Dysfunction, and Segmental dysfunction. | ||
Holter | Atrioventricular Conduction Disorder, Sinus Node Dysfunction, Atrial Fibrillation/Flutter, Average Heart Rate (AHR), Sustained Ventricular Tachycardia (SVT), Non-Sustained Ventricular Tachycardia (NSVT), Ventricular Extrasystole (VE), and Total Ventricular Extrasystole (TVE). | ||
Treatments | 3 | ICD, Ablations and Amiodarone. | |
Cardiology Guidelines Classification (CGC) | 4 | New York Heart Association (NYHA), Rassi Point, Rassi Escore, and Stage based on severity of cardiac involvement (2005 Guideline Classification). | |
Cardiac Restitution Metrics (CRM) | 7 | TendQ 5th Percentile (s), TpeakQ 5th Percentile (s), Percentage (%) of beats with Qtend/TendQ > 1, Percentage (%) of beats with QTpeak/TpeakQ > 1, Upper 98% quantil of the Qtend/TendQ ratio, Upper 98% quantil of the QTpeak/TpeakQ ratio, and Tpeak-Tend 5th-Percentile (ms). |
FS Flow | Algorithm | Features | ACC | AUC | Recall | Precision | F1 |
ANOVA | NB | 24 | 76.36 ± 6.55 | 78.53 ± 6.5 | 86.46 ± 8.2 | 63.11 ± 7.09 | 72.79 ± 6.9 |
Pipeline 1 | NB | 16 | 77.12 ± 5.94 | 79.66 ± 5.8 | 88.96 ± 9.2 | 63.70 ± 6.64 | 73.93 ± 6.3 |
Pipeline 2 | NB | 6 | 77.80 ± 6.47 | 78.99 ± 6.9 | 83.33 ± 14.5 | 66.67 ± 9.29 | 72.88 ± 8.6 |
Pipeline 3 | NB | 13 | 77.80 ± 6.08 | 80.55 ± 5.6 | 90.63 ± 7.3 | 64.32 ± 7.42 | 74.98 ± 6.2 |
RFE | NB | 13 | 77.73 ± 6.44 | 79.69 ± 5.7 | 86.88 ± 10.2 | 65.59 ± 8.39 | 74.08 ± 6.2 |
SFM | NB | 18 | 74.62 ± 11.33 | 77.83 ± 8.7 | 89.58 ± 7.0 | 62.34 ± 10.25 | 72.82 ± 7.7 |
VAR | BRF | 56 | 79.17 ± 5.47 | 80.28 ± 5.1 | 84.38 ± 8.0 | 67.78 ± 7.77 | 74.78 ± 5.8 |
FS Flow | Algorithm | Features | ACC | AUC | Recall | Precision | F1 |
ANOVA | NB | 29 | 71.64 ± 5.83 | 75.57 ± 4.6 | 83.54 ± 9.6 | 47.66 ± 6.79 | 60.18 ± 5.4 |
Pipeline 1 | NB | 10 | 73.28 ± 10.7 | 77.97 ± 7.1 | 87.50 ± 10.0 | 51.12 ± 11.0 | 63.49 ± 8.4 |
Pipeline 2 | NB | 4 | 71.96 ± 10.54 | 77.70 ± 6.8 | 89.38 ± 9.0 | 49.34 ± 9.60 | 62.75 ± 7.6 |
Pipeline 3 | BRF | 5 | 78.34 ± 5.86 | 80.63 ± 5.7 | 89.58 ± 8.3 | 64.93 ± 7.26 | 75.02 ± 6.3 |
RFE | NB | 11 | 74.87 ± 6.17 | 78.14 ± 5.7 | 84.79 ± 12.7 | 51.41 ± 7.78 | 63.32 ± 7.0 |
SFM | NB | 7 | 74.23 ± 9.58 | 79.78 ± 7.0 | 91.04 ± 8.2 | 51.53 ± 10.09 | 65.14 ± 8.4 |
VAR | BRF | 56 | 79.37 ± 5.85 | 80.40 ± 5.6 | 82.50 ± 8.6 | 57.34 ± 7.86 | 67.30 ± 7.0 |
FS Flow | Algorithm | Clinica Data | Classification | Treatments | CRM | Heart Tests | Total |
ANOVA | NB | 7 | 4 | 2 | 1 | 10 | 24 |
Pipeline 1 | NB | 3 | 3 | 2 | 2 | 6 | 16 |
Pipeline 2 | NB | 1 | 2 | 0 | 0 | 3 | 6 |
Pipeline 3 | NB | 0 | 4 | 1 | 1 | 7 | 13 |
RFE | NB | 3 | 1 | 1 | 3 | 5 | 13 |
SFM | NB | 3 | 4 | 2 | 3 | 6 | 18 |
VAR | BRF | 19 | 4 | 3 | 7 | 22 | 56 |
Study | Participants | ChHD Context | Machine Learning Model | Features | |
Souza et al. [8] | 373 | Yes | Classical Linear Regression (Not using ML) | 4 | |
Tse et al. [47] | 376 | No | Non-negative matrix factorization | 4 | |
Lyon et al. [48] | 123 | No | Density-based clustering | 7 | |
Nakajima et al. [49] | 526 | No | Random Forest, KNN, Gradient Boosted | 8 | |
Alberto et al. [26], | 82 | Yes | KNN | 8 | |
Shakibfar et al. [50] | 19, 935 | No | Random Forest | 9 | |
Atallah et al. [51] | 288 | No | Random Forest | 10 | |
Rodriguez et al.[52] | 140 | No | SVM | 12 | |
This Work (Pipeline 3) | 218 | Yes | Naive Bayes | 13 | |
Vergara et al. [53] | 502 | No | Random Forest | 15 | |
Lee et al. [54] | 516 | No | SVM | 26 | |
Zoni-Berisso et al. [55] | 404 | No | Mandansky artificial neural network | 61 | |
Goldstein et al. [56] | 1628 | No | Random Forest and KNN | 72 |
Study | ChHD Context | Recall (Sensitivity) |
Tse et al. [47] | No | 70.00% |
Zoni-Berisso et al. [55] | No | 96.00% |
Lee et al. [54] | No | 89.00% |
Atallah et al. [51] | No | 88.40% |
This Work (Pipeline 3) | Yes | 90.63% |