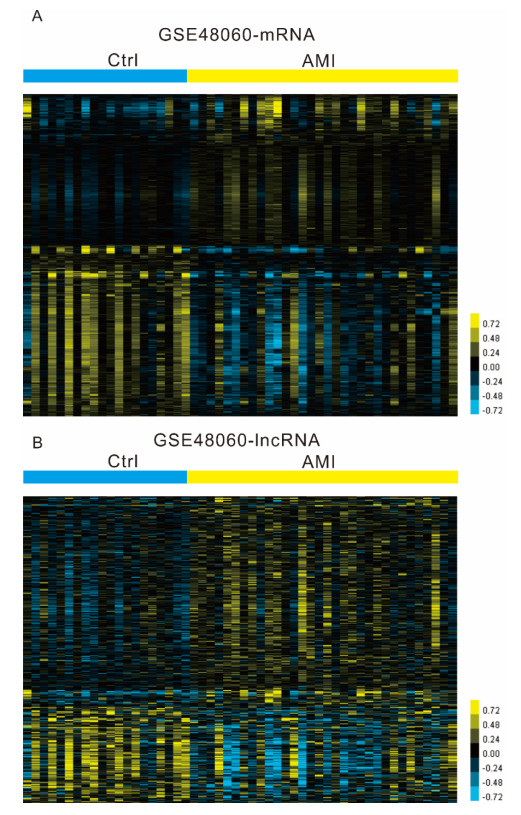
Citation: Lishui Shen, Xiaofeng Hu, Ting Chen, Guilin Shen, Dong Cheng. Integrated network analysis to explore the key mRNAs and lncRNAs in acute myocardial infarction[J]. Mathematical Biosciences and Engineering, 2019, 16(6): 6426-6437. doi: 10.3934/mbe.2019321
[1] | Jiaxin Luo, Lin Wu, Dinghui Liu, Zhaojun Xiong, Linli Wang, Xiaoxian Qian, Xiaoqiang Sun . Gene regulatory network analysis identifies key genes and regulatory mechanisms involved in acute myocardial infarction using bulk and single cell RNA-seq data. Mathematical Biosciences and Engineering, 2021, 18(6): 7774-7789. doi: 10.3934/mbe.2021386 |
[2] | Shuai Miao, Lijun Wang, Siyu Guan, Tianshu Gu, Hualing Wang, Wenfeng Shangguan, Weiding Wang, Yu Liu, Xue Liang . Integrated whole transcriptome analysis for the crucial regulators and functional pathways related to cardiac fibrosis in rats. Mathematical Biosciences and Engineering, 2023, 20(3): 5413-5429. doi: 10.3934/mbe.2023250 |
[3] | Yonghua Xue, Yiqin Ge . Construction of lncRNA regulatory networks reveal the key lncRNAs associated with Pituitary adenomas progression. Mathematical Biosciences and Engineering, 2020, 17(3): 2138-2149. doi: 10.3934/mbe.2020113 |
[4] | Sisi Qi, Youyu Sheng, Ruiming Hu, Feng Xu, Ying Miao, Jun Zhao, Qinping Yang . Genome-wide expression profiling of long non-coding RNAs and competing endogenous RNA networks in alopecia areata. Mathematical Biosciences and Engineering, 2021, 18(1): 696-711. doi: 10.3934/mbe.2021037 |
[5] | Yong Ding, Jian-Hong Liu . The signature lncRNAs associated with the lung adenocarcinoma patients prognosis. Mathematical Biosciences and Engineering, 2020, 17(2): 1593-1603. doi: 10.3934/mbe.2020083 |
[6] | Jinmiao Song, Shengwei Tian, Long Yu, Qimeng Yang, Qiguo Dai, Yuanxu Wang, Weidong Wu, Xiaodong Duan . RLF-LPI: An ensemble learning framework using sequence information for predicting lncRNA-protein interaction based on AE-ResLSTM and fuzzy decision. Mathematical Biosciences and Engineering, 2022, 19(5): 4749-4764. doi: 10.3934/mbe.2022222 |
[7] | Ming-Xi Zhu, Tian-Yang Zhao, Yan Li . Insight into the mechanism of DNA methylation and miRNA-mRNA regulatory network in ischemic stroke. Mathematical Biosciences and Engineering, 2023, 20(6): 10264-10283. doi: 10.3934/mbe.2023450 |
[8] | Li Li, Xueqing Wang, Xiaoting Liu, Rui Guo, Ruidong Zhang . Integrative analysis reveals key mRNAs and lncRNAs in monocytes of osteoporotic patients. Mathematical Biosciences and Engineering, 2019, 16(5): 5947-5971. doi: 10.3934/mbe.2019298 |
[9] | Beibei Zhu, Yue Mao, Mei Li . Identification of functional lncRNAs through constructing a lncRNA-associated ceRNA network in myocardial infarction. Mathematical Biosciences and Engineering, 2021, 18(4): 4293-4310. doi: 10.3934/mbe.2021215 |
[10] | Xudan Ma, Qijun Zhang, Haihong Zhu, Kefeng Huang, Weina Pang, Qin Zhang . Establishment and analysis of the lncRNA-miRNA-mRNA network based on competitive endogenous RNA identifies functional genes in heart failure. Mathematical Biosciences and Engineering, 2021, 18(4): 4011-4026. doi: 10.3934/mbe.2021201 |
Acute myocardial infarction (AMI) is one of the most severe cardiovascular events and causes more than a third of deaths in developed nations annually [1]. Of note, the global burden of acute myocardial infarction has shifted to low- and middle-income countries, including China [2]. AMI was divided into two kinds of histological categories, ST-elevation myocardial infarction (STEMI) and non-STEMI (NSTEMI) [3]. Cardiac troponin (cTn) isoforms I and T had emerged as the "gold standard" for the early diagnosis of AMI, which were detectable within 2-3 h and highly sensitive and specific for myocardial injury [4,5,6]. Of note, the mechanism underlying AMI progression remained unclear. Classic cardiovascular risk factors, including hypertension, diabetes, and smoking, had been showed to play a crucial role in AMI progression [7,8]. Recently, a few studies reported some mRNAs such as BNP and CRP were dysregulated in AMI progression [9,10]. Therefore, there was still an active demand to find new regulators to unveil the molecular mechanisms of AMI and provide new biomarkers for AMI.
More than 80%- of the human genome encodes non-coding transcripts [11]. In AMI, increasing studies reported showed non-coding RNAs, including miRNAs and lncRNAs, played important roles in AMI [12,13]. For example, inhibitions of microRNA-16 protect against AMI by reversing beta2-adrenergic receptor downregulation [14]. Lately, lncRNAs, a novel kind of ncRNAs containing longer than 200 nts, had gained increased attention [15]. Devaux et al. and Yang et al. reported a cluster of lncRNAs are dysregulated in peripheral blood cells of AMI patients [13,16]. The circulating lncRNA urothelial carcinoma-associated 1 (UCA1) was down-regulated within 3 days after the onset of AMI [17]. These reports suggested the potential prognostic value of lncRNAs in AMI. However, the knowledge of the role of lncRNAs in AMI is still limited.
In this work, we confirmed differentially expressed lncRNAs and mRNAs in the AMI by analyzing GSE48060. PPI network and lncRNA co-expression networks were built to determine hub mRNAs and lncRNAs. After that, GO and KEGG pathway analysis was utilized to study the potential functions of DEGs. This work would give important messages to determine the potential candidate biomarkers for diagnosis, prognosis, and drug targets in AMI.
According to Suresh et al.'s reports [18], the dataset of GSE48060 has downloaded the Gene Expression Omnibus database (GEO) (Dataset link: https://www.ncbi.nlm.nih.gov/geo/query/acc.cgi?acc=GSE48060). In this dataset, it contains 31 whole blood samples acquired from AMI patients and 21 controls samples acquired from normal echocardiogram AMI patients.
In this work, a pipeline was utilized to identify the lncRNA expression pattern in AMI, which was described by Zhang et al [19]. Briefly, the Affymetrix HG-U133 Plus 2.0 probe set ID was used to map to the NetAffx Annotation Files (HG-U133 Plus 2.0 Annotations, CSV format, release 31, 08/23/10). Second, the probe sets that were assigned with a RefSeq transcript ID in the NetAffx annotations were extracted. For the probe sets with RefSeq IDs, we only retained those labeled as "NR_" (NR indicates non-coding RNA in the RefSeq database). Third, we filtered the probe sets obtained in step 2 by filtering out pseudogenes, rRNAs, microRNAs and other short RNAs including tRNAs, snRNAs and snoRNAs. Finally, 2448 annotated lncRNA transcripts with corresponding Affymetrix probe IDs were generated.
The raw datasets of AMI were collected from GEO. The raw data was analyzed by utilizing the R software limma package [20]. The differential expression genes were satisfied with fold changes ≥2 and P values < 0.05.
The Database for Annotation Visualization and Integrated Discovery (DAVID; http://david.ncifcrf.gov) (version 6.8) integrating the information of biological functions of genes and analysis tools was used to unravel the GO and KEGG pathway of targets. [21].
In this study, the Pearson correlation coefficient between lncRNA and mRNA was calculated according to their expression value. The co-expressed DEG-lncRNA pairs with the absolute value of the Pearson correlation coefficient ≥ 0.75 were selected and the co-expression network was established using Cytoscape software. We applied the analysis of the interactions among mRNAs by utilizing STRING online software was utilized to analyze [22] and the combined score > 0.4 was utilized as the cut-off criterion [23]. The PPI network was built by utilizing Cytoscape software [24].
Because the distribution of expression data is normal, statistical comparisons between groups of normalized data were performed using the T-test according to the test condition. p < 0.05 was selected statistical significance with a 95% confidence level.
In our work, a public AMI mRNA expression profiling (GSE48060), which included 31 AMI patients and 21 controls blood samples, was analyzed to identify differentially expressed genes (DEGs) in AMI. DEGs analysis showed totally 4835 DEGs were determined through AMI patients samples, containing 2497 up-regulated genes and 2348 down-regulated genes. The heat map of the DEGs in AMI and control samples is shown in Figure 1A.
We further compared the lncRNA expression patterns between FL and DLBCL. We found 300 lncRNAs were up-regulated and 142 lncRNAs were down-regulated in AMI paralleled with normal samples (Figure 1B).
Based on the STRING database [25], we constructed PPI networks for up- and down-regulated mRNAs in AMI. The cut-off criterion of this analysis was that the score > 0.9. As shown in Figure 2, the up-regulated PPI network included 598 nodes and 1735 edges. 8 significant modules (score > 10) were identified in the up-regulated PPI network using the MCODE plug-in of Cytoscape. RHOA (degree = 43), GNB1 (degree = 41), GNG7 (degree = 41), and RAC1 (degree = 26) were identified as hub nodes in PPI network (Figure 2).
We found down-regulated PPI network included 662 nodes and 3114 edges. Meanwhile, nine significant modules with score > 10 were identified in down-regulated PPI (Figure 3). The nodes that had higher degrees including CDC23 (degree = 40), FBXO32 (degree = 37), DET1 (degree = 36), MEX3C (degree = 35), HECTD1 (degree = 34), HERC4 (degree = 33), POLR2K (degree = 33), CUL5 (degree = 31), UNKL (degree = 30) (Figure 3).
It is worth noting that the roles of dys-regulated lncRNAs in AMI are still dimness. Therefore, the co-expression network of lncRNAs was built to unveil their potential functions in AMI. In this research, only the co-expressed DEG-lncRNA pairs (Pearson correlation coefficient ≥ 0.75) were selected to construct the co-expression network. The up-regulated lncRNA associated network contained 49 lncRNAs and 502 differentially expressed genes (Figure 4). Meanwhile, 71 lncRNAs and 1156 DEGs were included in the down-regulated lncRNA linked network totally (Figure 5).
A part of lncRNAs were well-known and reported to play important roles in many types of human diseases, such as GAS5, TUG1 and MALAT1. However, most of these lncRNAs were never reported in human diseases. Several lncRNAs were identified as key regulators in AMI by regulating more than 50 DEGs, such as up-regulated CA5BP1 and LOC101927608, and down-regulated BZRAP1-AS1, EBLN3, FGD5-AS1, HNRNPU-AS1, LINC00342, and LOC101927204.
Furthermore, for obtaining differentially expressed mRNAs, we utilized GO and KEGG analysis. GO analysis demonstrated that the dys-regulated lncRNAs were associated with regulating transcription, protein amino acid phosphorylation, development, interspecies interaction between organisms, cell cycle, signal transduction, cell adhesion, intracellular signaling cascade, protein transport, apoptosis, ubiquitin-dependent protein catabolism, negative regulation of cell proliferation, modification-dependent protein catabolism, RNA splicing, cell division, nuclear mRNA splicing, DNA repair, response to DNA damage stimulus (Figure 6A-6B).
The results of the KEGG pathway indicated that upregulated genes were associated with pathways associated with Ubiquitin-mediated proteolysis, Insulin signaling pathway, Tight junction, Axon guidance, Basal transcription factors, Regulation of actin cytoskeleton, MAPK signaling pathway, and ErbB signaling pathway. Downregulated genes were mainly associated with T cell receptor signaling pathway, MAPK signaling pathway, Chronic myeloid leukemia, Cell cycle, ErbB signaling pathway, Axon guidance, Ubiquitin mediated proteolysis, Insulin signaling pathway, p53 signaling pathway (Figure 6C-6D).
Bioinformatics analysis, such as PPI network analysis, co-expression analysis, and KEGG analysis, had been suggested as useful tools to reveal disease-specific mechanisms and widely applied to identify novel diagnostic and prognostic biomarkers for human diseases [26]. Most importantly, there was still an active demand to determine new regulators to find the molecular mechanisms and provide new biomarkers for AMI. In our work, we reanalyzed a public AMI mRNA expression profiling GSE48060. A total of 2497 up-regulated mRNAs and 2348 down-regulated mRNAs were identified in AMI patients. PPI networks were constructed and the network analysis revealed several key regulators in AMI, such as RHOA, GNB1, GNG7, RAC1, CDC23, FBXO32, DET1, and MEX3C.
Recently, the important roles of lncRNAs were revealed in human disease progression [27]. LncRNAs could regulate expression and activity of protein through transcriptional, post-transcriptional, posttranslational and/or epigenetic regulation [28,29]. In AMI, a few groups had showed dysregulated lncRNAs could serve as biomarkers [30]. MIAT was the first reported lncRNA which confers risk of myocardial infarction [31]. Zhang et al. also found that Zinc finger antisense 1 (ZFAS1) and Cdr1 antisense (CDR1AS) showed significant differential expression between AMI patients and control subjects [32]. The Comprehensive whole-genome screen of the transcriptome was also performed to reveal differently expressed genes and lncRNAs in AMI. For example, Devaux et al. and Yang et al. reported a cluster of lncRNAs are dysregulated in peripheral blood cells of AMI patients [13,16]. Hence, the functions of most lncRNAs in AMI was unknown.
In this research, we found 300 lncRNAs were up-regulated and 142 lncRNAs were down-regulated in AMI compared to normal samples. Among them, some lncRNAs had been reported in human diseases, including GAS5, TUG1 and MALAT1. Most dysregulated lncRNAs remained unclear. Therefore, the co-expression network of lncRNAs was built to show their potential roles in AMI. We found 49 lncRNAs and 502 differentially expressed genes were included in the up-regulated lncRNA associated network. Meanwhile, 71 lncRNAs and 1156 DEGs were included in the down-regulated lncRNA associated network. Several lncRNAs were identified as key regulators in AMI by regulating more than 50 DEGs, such as up-regulated CA5BP1 and LOC101927608, and down-regulated BZRAP1-AS1, EBLN3, FGD5-AS1, HNRNPU-AS1, LINC00342, and LOC101927204. KEGG and GO analysis were also performed. GO analysis demonstrated that the dysregulated lncRNAs were mainly involved in regulating transcription, signal transduction, development, interspecies interaction between organisms, cell cycle, cell adhesion, intracellular signaling cascade, protein transport, apoptosis, ubiquitin-dependent protein catabolism, RNA splicing. KEGG pathways showed the dysregulated lncRNAs were associated with Regulation of the actin cytoskeleton, T cell receptor signaling pathway, MAPK signaling pathway, Cell cycle, ErbB signaling pathway, Ubiquitin mediated proteolysis, Chronic myeloid leukemia, p53 signaling pathway, Insulin signaling pathway, Tight junction, Basal transcription factors.
Several limitations in present study should be noted. First, the present study analyzed a public dataset GSE48060 to identify AMI related mRNAs and lncRNAs. A much larger number of public datasets are necessary to support in silico analysis. Moreover, these results were mainly based on bioinformatics analysis. Despite this study provide novel and useful information for us to understand the potential functions of lncRNAs in AMI, further validation of our analysis using clinical samples is still needed.
In conclusion, a public dataset GSE48060 was utilized to confirm differentially expressed lncRNAs and mRNAs in the AMI. PPI networks were built to unveil key mRNAs in AMI. We also conducted lncRNA co-expression networks to shoe the functions of these novel lncRNAs. Several lncRNAs, including CA5BP1, LOC101927608, BZRAP1-AS1, EBLN3, FGD5-AS1, HNRNPU-AS1, LINC00342, and LOC101927204 were identified as key lncRNAs. KEGG and GO analysis were revealed these dysregulated lncRNAs widely participated in regulating a series of biological processes, such as transcription, signal transduction and MAPK pathway. We thought this work would provide information to study new molecular therapy for AMI.
The authors declare no financial conflicts of interest.
[1] | G. W. Reed, J. E. Rossi and C. P. Cannon, Acute myocardial infarction, The Lancet, 389 (2017), 197–210. |
[2] | J. L. Anderson and D. A. Morrow, Acute myocardial infarction, N. Engl. J. Med., 376 (2017), 2053–2064. |
[3] | C. Basso, S. Rizzo and G. Thiene, The metamorphosis of myocardial infarction following coronary recanalization, Cardiovasc. Pathol., 19 (2010), 22–28. |
[4] | D. W. Kehl, N. Iqbal, A. Fard, et al., Biomarkers in acute myocardial injury, Transl. Res., 159 (2012), 252–264. |
[5] | S. Korff, H. A. Katus and E. Giannitsis, Differential diagnosis of elevated troponins, Heart, 92 (2006), 987–993. |
[6] | P. O'Brien, D. Smith, T. Knechtel, et al., Cardiac troponin I is a sensitive, specific biomarker of cardiac injury in laboratory animals, Lab. Anim-UK, 40 (2006), 153–171. |
[7] | M. Saito, T. Ishimitsu, J. Minami, et al., Relations of plasma high-sensitivity C-reactive protein to traditional cardiovascular risk factors, Atherosclerosis, 167 (2003), 73–79. |
[8] | V. A. Triant, H. Lee, C. Hadigan, et al., Increased acute myocardial infarction rates and cardiovascular risk factors among patients with human immunodeficiency virus disease, J. Clin. Endocr. Metab., 92 (2007), 2506–2512. |
[9] | R. B. Ramos, C. M. Strunz, S. D. Avakian, et al., B-type natriuretic peptide as a predictor of anterior wall location in patients with non-ST-elevation myocardial infarction, Clinics, 66 (2011), 437–441. |
[10] | O. M. Martins, V. F. Fonseca, I. Borges, et al., C-reactive protein predicts acute myocardial infarction in high-risk noncardiac and vascular surgery, Am. Heart Assoc., 2009. |
[11] | P. D. Zamore and B. Haley, Ribo-gnome: The big world of small RNAs, Science, 309 (2005), 1519–1524. |
[12] | T. Adachi, M. Nakanishi, Y. Otsuka, et al., Plasma microRNA 499 as a biomarker of acute myocardial infarction, Clin. Chem., 56 (2010), 1183–1185. |
[13] | M. Vausort, D. R. Wagner and Y. Devaux, Long noncoding RNAs in patients with acute myocardial infarction novelty and significance, Circ. Res., 115 (2014), 668–677. |
[14] | J. Liu, F. Sun, Y. Wang, et al., Suppression of microRNA-16 protects against acute myocardial infarction by reversing beta2-adrenergic receptor down-regulation in rats, Oncotarget, 8 (2017), 20122. |
[15] | A. E. Kornienko, P. M. Guenzl, D. P. Barlow, et al., Gene regulation by the act of long non-coding RNA transcription, BMC biol., 11 (2013), 59. |
[16] | K. C. Yang and J. M. Nerbonne, Mechanisms contributing to myocardial potassium channel diversity, regulation and remodeling, Trends Cardiovas. Med., 26 (2016), 209–218. |
[17] | Y. Guo, F. Luo, Q. Liu, et al., Regulatory non-coding RNAs in acute myocardial infarction, J. Cell Mol. Med., (2016). |
[18] | R. Suresh, X. Li, A. Chiriac, et al., Transcriptome from circulating cells suggests dysregulated pathways associated with long-term recurrent events following first-time myocardial infarction, J. Mol. Cell Cardiol., 74 (2014), 13–21. |
[19] | X. Zhang, S. Sun, J. K. Pu, et al., Long non-coding RNA expression profiles predict clinical phenotypes in glioma, Neurobiol. Dis., 48 (2012), 1–8. |
[20] | J. M. Wettenhalland G. K. Smyth, limmaGUI: A graphical user interface for linear modeling of microarray data, Bioinformatics, 20 (2004), 3705–3706. |
[21] | D. W. Huang, B. T. Sherman and R. A. Lempicki, Systematic and integrative analysis of large gene lists using DAVID bioinformatics resources, Nat. Protoc., 4 (2009), 44–57. |
[22] | D. Zhang, W. Ma, Y. He, et al., Data of the interacting protein networks and nucleotide metabolism pathways related to NDK and NT5, Data Brief, 9 (2016), 1063–1066. |
[23] | H. Yan, Z. Li, Q. Shen, et al., Aberrant expression of cell cycle and material metabolism-related genes contributes to hepatocellular carcinoma occurrence, Pathol. Res. Pract., 213 (2017), 316–321. |
[24] | M. Kohl, S. Wiese and B. Warscheid, Cytoscape: Software for visualization and analysis of biological networks, Methods Mol. Biol., 696 (2011), 291–303. |
[25] | D. Szklarczyk, A. Franceschini, S. Wyder, et al., STRING v10: protein-protein interaction networks, integrated over the tree of life, Nucleic. Acids Res., 43 (2014), D447–D452. |
[26] | X. Wangand L. Liotta, Clinical bioinformatics: a new emerging science, J. clin. Bioinform., 1 (2011), 1. |
[27] | J. R. Prensner and A. M. Chinnaiyan, The emergence of lncRNAs in cancer biology, Cancer Discov., 1 (2011), 391–407. |
[28] | M. Huarte, The emerging role of lncRNAs in cancer, Nat. Med., 21 (2015), 1253. |
[29] | A. Fatica and I. Bozzoni, Long non-coding RNAs: New players in cell differentiation and development, Nat. Rev. Genet., 15 (2014), 7. |
[30] | Q. Shi and X. Yang, Circulating microRNA and long noncoding RNA as biomarkers of cardiovascular diseases, J. Cell Physiol., 231 (2016), 751–755. |
[31] | K. Wang, C. Y. Liu, L. Y. Zhou, et al., APF lncRNA regulates autophagy and myocardial infarction by targeting miR-188-3p, Nat. Commun., 6 (2015), 6779. |
[32] | Y. Zhang, L. Sun, L. Xuan, et al., Reciprocal changes of circulating long non-coding RNAs ZFAS1 and CDR1AS predict acute myocardial infarction, Sci. Rep-UK, 6 (2016). |
1. | Zsófia Gál, András Gézsi, Ágnes F. Semsei, Adrienne Nagy, Monika Sultész, Zsuzsanna Csoma, Lilla Tamási, Gabriella Gálffy, Csaba Szalai, Investigation of circulating lncRNAs as potential biomarkers in chronic respiratory diseases, 2020, 18, 1479-5876, 10.1186/s12967-020-02581-9 | |
2. | Beibei Zhu, Yue Mao, Mei Li, Identification of functional lncRNAs through constructing a lncRNA-associated ceRNA network in myocardial infarction, 2021, 18, 1551-0018, 4293, 10.3934/mbe.2021215 | |
3. | Li Zhang, Yao Zhao, Hao Guan, Di Zhang, HnRNPU-AS1 inhibits the proliferation, migration and invasion of HCC cells and induces autophagy through miR-556-3p/ miR-580-3p/SOCS6 axis, 2022, 34, 18758592, 443, 10.3233/CBM-210261 | |
4. | Wei-Tao Zhang, Ji-Jun Zhang, Quan Shao, Ying-Kai Wang, Jie-Peng Jia, Bo Qian, Xiao-Wen Tian, Wen-Ji Yan, FGD5‑AS1 is an oncogenic lncRNA in pancreatic cancer and regulates the Wnt/β‑catenin signaling pathway via miR‑577, 2021, 47, 1021-335X, 10.3892/or.2021.8232 | |
5. | Ting-Wei Kao, Chin-Chou Huang, Inflammatory Burden and Immunomodulative Therapeutics of Cardiovascular Diseases, 2022, 23, 1422-0067, 804, 10.3390/ijms23020804 | |
6. | Yongdi Wang, Jian Wang, Diagnostic significance of serum FGD5-AS1 and its predictive value for the development of cardiovascular diseases in patients with type 2 diabetes, 2022, 14, 1758-5996, 10.1186/s13098-022-00789-x | |
7. | Zhaoyuan Niu, Fengling Wang, Shihui Lv, Yingpin Lv, Ming Liu, Lei Fu, Yushuang Yao, Lingzhi Wang, Wei Lin, Fang Yuan, HNRNPU-AS1 Regulates Cell Proliferation and Apoptosis via the MicroRNA 205-5p/AXIN2 Axis and Wnt/β-Catenin Signaling Pathway in Cervical Cancer, 2021, 41, 1098-5549, 1, 10.1128/MCB.00115-21 | |
8. | O. V. Kochetova, D. Sh. Avzaletdinova, G. F. Korytina, The expression of long non-coding RNAs TP53TG1, LINC00342, MALAT1, H19 and MEG3 in type 2 Diabetes mellitus, 2024, 58, 0026-8984, 260, 10.31857/S0026898424020075 | |
9. | Jun Wei, XueShuang Zhu, AYu Sun, XiaoTian Yan, Xing Meng, Shenglin Ge, Long non-coding RNA FGD5 antisense RNA 1 targets Baculovirus inhibitor 5 via microRNA-497-5p to alleviate calcific aortic valve disease, 2024, 86, 13860291, 285, 10.3233/CH-221692 | |
10. | O. V. Kochetova, D. Sh. Avzaletdinova, G. F. Korytina, The Expression of TP53TG1, LINC00342, MALAT1, H19, and MEG3 Long Noncoding RNAs in Type 2 diabetes mellitus, 2024, 58, 0026-8933, 246, 10.1134/S0026893324020080 | |
11. | Ya‐Nan Yang, Yong‐Bai Luo, Gang Xu, Kang Li, Ru‐Lan Ma, Wei Yuan, CircHECTD1 promoted MIRI‐associated inflammation via inhibiting miR‐138‐5p and upregulating ROCK2, 2023, 39, 1607-551X, 675, 10.1002/kjm2.12686 | |
12. | Yan Shen, Jiahui Li, Zehao Zhao, Xiaomin Chen, Progress on long non-coding RNAs in calcific aortic valve disease, 2025, 12, 2297-055X, 10.3389/fcvm.2025.1522544 |