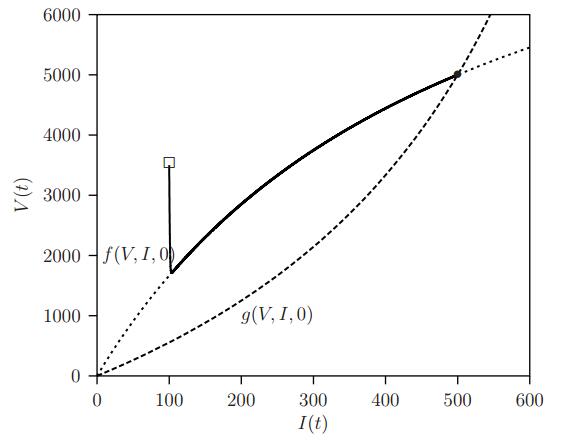
Citation: Peter Rashkov, Ezio Venturino, Maira Aguiar, Nico Stollenwerk, Bob W. Kooi. On the role of vector modeling in a minimalistic epidemic model[J]. Mathematical Biosciences and Engineering, 2019, 16(5): 4314-4338. doi: 10.3934/mbe.2019215
[1] | Yan-Xia Dang, Zhi-Peng Qiu, Xue-Zhi Li, Maia Martcheva . Global dynamics of a vector-host epidemic model with age of infection. Mathematical Biosciences and Engineering, 2017, 14(5&6): 1159-1186. doi: 10.3934/mbe.2017060 |
[2] | Pengyan Liu, Hong-Xu Li . Global behavior of a multi-group SEIR epidemic model with age structure and spatial diffusion. Mathematical Biosciences and Engineering, 2020, 17(6): 7248-7273. doi: 10.3934/mbe.2020372 |
[3] | Yilei Tang, Dongmei Xiao, Weinian Zhang, Di Zhu . Dynamics of epidemic models with asymptomatic infection and seasonal succession. Mathematical Biosciences and Engineering, 2017, 14(5&6): 1407-1424. doi: 10.3934/mbe.2017073 |
[4] | Mostafa Adimy, Abdennasser Chekroun, Claudia Pio Ferreira . Global dynamics of a differential-difference system: a case of Kermack-McKendrick SIR model with age-structured protection phase. Mathematical Biosciences and Engineering, 2020, 17(2): 1329-1354. doi: 10.3934/mbe.2020067 |
[5] | Mahmudul Bari Hridoy . An exploration of modeling approaches for capturing seasonal transmission in stochastic epidemic models. Mathematical Biosciences and Engineering, 2025, 22(2): 324-354. doi: 10.3934/mbe.2025013 |
[6] | Pengfei Liu, Yantao Luo, Zhidong Teng . Role of media coverage in a SVEIR-I epidemic model with nonlinear incidence and spatial heterogeneous environment. Mathematical Biosciences and Engineering, 2023, 20(9): 15641-15671. doi: 10.3934/mbe.2023698 |
[7] | Hongyong Zhao, Yangyang Shi, Xuebing Zhang . Dynamic analysis of a malaria reaction-diffusion model with periodic delays and vector bias. Mathematical Biosciences and Engineering, 2022, 19(3): 2538-2574. doi: 10.3934/mbe.2022117 |
[8] | Andrea Franceschetti, Andrea Pugliese, Dimitri Breda . Multiple endemic states in age-structured SIR epidemic models. Mathematical Biosciences and Engineering, 2012, 9(3): 577-599. doi: 10.3934/mbe.2012.9.577 |
[9] | Ling Xue, Caterina Scoglio . Network-level reproduction number and extinction threshold for vector-borne diseases. Mathematical Biosciences and Engineering, 2015, 12(3): 565-584. doi: 10.3934/mbe.2015.12.565 |
[10] | Jun Hyung Bae, Sang-Mook Lee . Investigation of SIS epidemics on dynamic network models with temporary link deactivation control schemes. Mathematical Biosciences and Engineering, 2022, 19(6): 6317-6330. doi: 10.3934/mbe.2022295 |
The spread of the vector-borne dengue fever in a human population has been studied in many papers: we mention a family of three nested multi-strain compartmental SIR type models analyzed in [1,2,3,4]. In [3] a realistic description of recorded disease incidents was produced. However, in these models the vector dynamics was taken into account by assuming that the size of the infected vector population is proportional to the infected human-host population.
On the other hand, the analysis of [5] shows that an increased degree of personal protection and vector control are effective control measures in dengue-endemic areas. This leads to the conclusion that in order to study the effects of vector-mosquito control an accurate vector population model together with a human-host population model describing the multiple-strain virus outbreaks and spread is required.
In [6,7,8] the epidemics of the vectors is modeled explicitly by adding to a model similar to those in [1,2,3] extra compartments for the vector epidemics without taking ecological processes into account. The dynamics of the epidemics of the vector is directly related to ecological processes during the very complex life-cycle with four stages: egg (embryo), larva, pupa (embryo) (in the water phase) and imago (adult). Furthermore the numbers of healthy and infected vector individuals will change due to epidemiological processes such as the spread of the disease in the host. The combination of the vector and the host model results then in an eco-epidemiology model where two scientific fields are combined to study ecological and epidemiological issues simultaneously.
The formulation and analyses of such a complicated model and its analysis based almost completely on numerical simulations is beyond the scope of this paper. In the vector-host epidemic model analyzed in this paper we couple a simplified dengue-fever model for the host population based on [3] with a simplified model for the mosquito vector population [9]. This is done in order to gain insight into the effects of the vector dynamics on the host dynamics, especially when the time-scale of the vector population is much faster than that of the host. In [9] it was investigated how far the fast time scale of the mosquito epidemiology can be slaved by the slower human epidemiology, so that for the understanding of human disease data mainly the dynamics of the human time scale is essential because it will be only slightly perturbed by the mosquito dynamics. Useful and realistic parameter values for the dengue fever case from [3] were used. It was concluded that owing to the faster vector dynamics it is slaved by the slower dynamics of the hosts.
Mathematically the central manifold approximation in was used [9] to improve the quasi-steady-state assumption (QSSA) results, see also [10]. Here we use the geometric singular perturbation method where asymptotic expansions give a better approximation when the time-scale difference is moderate, see also [10].
The change from the disease-free into the endemic state of the population under variation of the force of infection parameter is studied in the context of bifurcation theory. Instead of the threshold value for the force of infection where the basic reproduction number equals one, R0=1 we calculate this value by performing a bifurcation analysis. In this case an eigenvalue of the Jacobian matrix evaluated at an equilibrium of the autonomous system is zero.
Besides time-scale separation, another important issue is the effect of seasonality. In [11,12] seasonality in a model similar to the one in [9] is studied. In [13] it is concluded that assuming parameter stationarity in systems with approximate stationarity in disease incidence is unjustified and may result in missed opportunities to understand the drivers of disease variability. The effect of seasonality is studied where the force of infection changes sinusoidally in time, modeling the annual fluctuations of the presence of the vector. The resulting non-autonomous system is studied in the same way as the autonomous system whereby equilibria are replaced by limit cycles and eigenvalues by Floquet multipliers.
In [9, Sec. 3] an elementary susceptible-infected-removed SIR model for the host where host individuals start off susceptible, at some stage catch the disease, and after a short infectious period become permanently immune, is coupled with also a minimal susceptible-infected SI model for the vector, where mosquito-vectors start off susceptible, at some stage catch the disease and remain infected for the duration of their lifetime. The resulting model is called the SIRUV model.
This simple model is formulated to study the effects of the difference in the time scales of the epidemics in the host population and in the vector population. The total human population is denoted N=S+I+R, assumed to be constant. The compartments of the model are formed by susceptible S, infected I and recovered R, all non-negative. The total vector population is denoted by M=U+V where U and V are the susceptible and infected disease vectors.
dSdt=−βMSV+μ(N−S),dIdt=βMSV−(γ+μ)I,dRdt=γI−μRdUdt=−ϑNUI+ν(M−U),dVdt=ϑNUI−νV. |
The law of mass action is assumed to model random encounters between vector and host. This model can be reduced to an equivalent three-dimensional model:
dSdt=−βMSV+μ(N−S), | (2.1a) |
dIdt=βMSV−(γ+μ)I, | (2.1b) |
dVdt=ϑN(M−V)I−νV. | (2.1c) |
In order to analyze this model in more detail a simplified model was formulated with a two-dimensional equivalent model [9, Sec. 2]. In this model the SIR model for the host is replaced by a SIS model where host individuals start off susceptible, at some stage catch the disease, and after a short infectious period become susceptible again (no protective immunity), called SISUV model, where γ=0: that is, it assumes no recovery compartment and allows the infected hosts after successfully defeating the disease to re-enter immediately into the compartment of the susceptible:
dSdt=−βMSV+μI,dIdt=βM(N−I)V−μI,dUdt=−ϑN(M−V)I+νV,dVdt=ϑN(M−V)I−νV. | (2.2) |
Note that assuming γ=0 turns the model (2.1) into (2.2), which is an SI model with no recovery, although formally it appears as if it were indeed an SIS model with the birth/death rate μ playing the role of the recovery rate.
Alternatively, using the constant host and vector populations, we obtain:
dIdt=βM(N−I)V−μI, | (2.3a) |
dVdt=ϑN(M−V)I−νV. | (2.3b) |
In this work the geometric singular perturbation technique is used to analyze the SIRUV model (2.1) and the SISUV model (2.3). We will also analyze the quasi-steady state solutions. The reader is referred to [14,15] for introductions into perturbation analysis and [16] for an illustrative application to the Rosenzweig-MacArthur predator-prey model [17,18].
We start with a short overview of singular perturbation technique. Singular perturbation theory deals with systems whose solutions evolve on different time scales whose ratio is characterized by a small parameter ε>0. When ε≪1 the system is a fast-slow system. For instance, in our epidemic models (2.1-2.3) the mosquito-vector dynamics occurs at a much faster time scale than the human-host dynamics. To set (2.3) as an illustration to this approach, the original equations can be rewritten in the form
dIdt=εg(V,I,ε)=ε(βM(N−I)V−μI), | (3.1a) |
dVdt=f(V,I,ε)=ϑN(M−V)I−νV. | (3.1b) |
With ε=0 we have the fast system also called the layer system:
dVdt=f(V,I(0),0)=ϑN(M−V)I(0)−νV,dIdt=0. | (3.2a) |
The infected host population remains constant, so that the trajectories are the vertical lines in Figure 1. With a change of time-scale τ=εt for system (2.3), where the resulting system with ε≪1 is named the slow system:
Par. | Description | Units | A | B |
Host | ||||
N | Host population size | # | 1000 | 1000 |
β | Infection rate | # y−1 | 730/7 | 0.2 |
μ | Susceptible birth rate | # y−1 | 1/65 | 0.1 |
γ | Recovery rate | # y−1 | 365/7 | n/a |
Vector | ||||
M | Vector population size | # | 10000 | 10000 |
ϑ | Infection rate | # y−1 | 0.2×365 | 0.2×365 |
ν | Susceptible birth rate | # y−1 | 0.1×365 | 0.1×365 |
ρ | Magnitude of sinusoidal fluctuation vectors | – | 0.9 | n/a |
T0 | Period of system | y | 1 | n/a |
εdIdτ=εg(V,I,ε)=ε(βM(N−I)V−μI),εdVdτ=f(V,I,ε)=ϑN(M−V)I−νV. |
After substitution of ε=0 we get:
0=f(V,I,0),V=IMI+Nνϑ, | (3.3a) |
dIdτ=g(V,I,0)=βM(N−I)V−μI. | (3.3b) |
This differential algebraic equation (DAE) is called the reduced system. The trajectory is a part of the f-nullcline of the original SISUV-system (2.3) shown in Figure 1. However, in the fast-slow context this is a set of equilibria of the fast layer-system (3.2), called critical manifold, in which V acts as a parameter.
These heuristic results suggest the following approach for dealing with the two different time scales. The first step consists in setting ε=0, which gives the set of fast equilibria of the fast system (3.2) yielding the algebraic equation (3.3a). This is the critical manifold, namely the set of equilibria on the hyperbola f(V,I,0)=0.
With a good hypothesis (see below for the details) the hyperbola f(V,I,0)=0 is equivalent to I=q(V) and we can substitute I by q(V) in equation (3.3b). The result is the slow or reduced-system:
I=q(V)=νNVϑ(M−V). | (3.4) |
Using (3.3b) and the chain rule
dIdτ=g(V,q(V))=dqdVdVdτ, |
from (3.4) we get formally:
dVdτ=βM(N−q(V))V−μq(V)dq/dV,dqdV=νNMϑ(M−V)2. | (3.5) |
For the case 0<ε≪1, we follow the geometric singular perturbation technique. Let us consider system (2.3) again. For ε=0 the f-nullcline, see Figure 1, the set
{(V,I)|f(V,I,0)=0,V≥0,I≥0} | (3.6) |
consists of the critical manifold
M={(V,I)|I=νVNϑ(M−V),0≤V≤M,0≤I≤N}. | (3.7) |
M forms a set of equilibria of the fast system (3.2).
We assume that the critical manifold M is normally hyperbolic. Then Fenichel's theorem [15,19,20] states that there exists ε0 such that for 0<ε<ε0, there are locally invariant manifolds Mε. Using its invariance, the perturbed manifold Mε can be approximated by asymptotic expansions in ε. With good hypothesis it can be described as a graph
{(V,I)|I=q(V,ε),0≤V≤M,0≤I≤N}. |
This manifold is invariant when the following equality holds
dIdτ=∂I∂VdVdτ=∂q(V,ε)∂VdVdτ, |
which yields with Eq (3.1) and I=q(V,ε) the invariance equation for the SISUV model:
∂q(V,ε)∂V(ϑN(M−V)q(V,ε)−νV)=ε(βM(N−q(V,ε))V−μq(V,ε)). | (3.8) |
Later we will furthermore assume that also the inverse of function q(V)=I exists, denoted by p(I)=q−1(I)=V(I). That is, besides the implicit function theorem also the inverse function theorem holds in region of the (V,I) plane we are interested in.
The two-dimensional model is given in (3.1). There is a trivial, disease-free equilibrium I0=0,V0=0 and S0=N and an interior, endemic equilibrium given by:
I∗=Nβϑ−μν(μ+β)ϑ,V∗=Mβϑ−μνβ(ν+ϑ). | (4.1) |
The biologically relevant domain for (2.3) is Ω={(I,V)|0≤I≤N,0≤V≤M}. Following [21, Lemma 2.1], one can demonstrate that Ω is positively invariant under (2.3). The details are not shown here.
Since the system is two-dimensional we calculate the determinant and trace of the Jacobian evaluated at the equilibria, [22]. The trace and the determinant of the 2×2 Jacobian matrix J read
TrJ=ϵ(−βV/M−μ)−ϑI/N−ν, | (4.2a) |
detJ=ϵ((μν−βϑ)N+Iϑ(β+μ))M+NVβ(ν+ϑ)MN | (4.2b) |
At the trivial equilibrium we have the following stability properties. Evaluated at this equilibrium the trace and the determinant read:
TrJ=−(ϵμ+ν),detJ=ϵ(μν−βϑ). | (4.3) |
Note that the N and M do not appear in these expressions, but only the force of infections β, ϑ and the birth and death rates μ,ν of host and vector respectively are present. The trace TrJ<0 is always negative. For the reference values given in Table 1 we have β=2μ and ϑ=2ν. Then the determinant is negative:
detJ=ϵ(μν−4μν)=−3ϵμν<0. | (4.4) |
Hence, the Routh-Hurwitz criterion shows the instability of the trivial equilibrium.
At the interior point given by Eq (4.1) we have
TrJ=ϵ(νμ−βϑν+ϑ−μ)+νμ−βϑμ+β−ν,detJ=−ϵ(μν−βϑ). | (4.5) |
The determinant has just the opposite value of the trivial equilibrium. It changes sign when μν=βϑ. At this point both equilibria coincide and the trace TrJ<0 is again negative. This means that we have detJ=0 at the transcritical bifurcation TC given by
β=μνϑ | (4.6) |
For values of the parameter β above this point we have detJ>0 and the interior equilibrium Eq (4.1) is asymptotically stable.
In the epidemiological literature the basic reproduction number R0 represents the number of secondary cases one case generates on average over the course of its infectious period in an otherwise uninfected population [23,24]. R0 equals one at the TC-point.
The QSSA equilibrium is calculated, assuming ε=0, from Eq (2.3b)
V∗QSSA=MIνN/ϑ+I. | (4.7) |
This means that the fast variable V changes instantaneously to this value and we substitute this equilibrium value V∗QSSA in Eq (2.3a) yielding:
dIdt=β(N−I)IνN/ϑ+I−μI | (4.8) |
After I(t) is calculated by solving the single ODE Eq (2.3) and thereafter Eq (4.7) gives V(t).
Hence, the time-scale argument gives a further reduction to an equivalent one-dimensional model. When we use the zero-order approximation we got the classical QSSA assumption. Then we finally get using Eq (3.3) the single ODE for the SISUV-system with large time-scale differences between the host and the vector for the infected host population. Observe that in this one-dimensional version the parameters ν, ϑ from the vector modeling are present in the hyperbolic expression but not the size of the vector population M. This hyperbolic expression models a saturated incidence rate. It resembles the Holling type Ⅱ functional response well known in predator-prey models. In ecology the quantity Nν/ϑ is called the half-saturation constant. A mechanistic derivation is obtained when besides searching time also handling time of the predator to digest a prey individual is introduced. In biochemistry, Michaelis-Menten kinetics is one of the best-known models of enzyme kinetics. Then the reaction rate is an hyperbolic expression in the substrate concentration. The Michaelis constant is again the substrate concentration at which the reaction rate is half of the maximum rate at abundant substrate. In the epidemiological literature it has also been considered to describe the infection mechanism with a saturated incidence rate [25,26,27,28].
Motivated by Holling's derivation it is tempting to follow a similar approach in to obtain the hyperbolic expression in epidemics. In [24, p.155 and p.277–280] it is argued why this is impossible. Namely, it is due to the fact that in the ecological predator-prey setting the two populations are treated independently, whereas in the epidemiology there is one population in which the individuals can reside in two states, namely susceptible and infective.
Interestingly for a vector-borne disease we have here a simple mechanistic formulation where, as in the derivation of the Holling type Ⅱ, a time-scale argument plays a crucial role.
The following asymptotic expansion in 0<ε≪1 is introduced:
I(V)=q(V,ε)=q0(V)+εq1(V)+ε2q2(V)+…, | (4.9) |
hence
∂q∂V=dq0dV+εdq1dV+ε2dq2dV+…. | (4.10) |
Substitution into the invariance equation (3.8) gives
(dq0dV+εdq1dV+ε2dq2dV+…)(ϑN(M−V)(q0(V)+εq1(V)+ε2q2(V)+…)−νV)=ε(βM(N−(q0(V)+εq1(V)+ε2q2(V)+…))V−μ(q0(V)+εq1(V)+ε2q2(V)+…)). | (4.11) |
Gathering zero orders of ε and assuming V>0 results in the following formula, accurate of order O(1):
dq0dV(ϑN(M−V)q0(V)−νV)=0, |
and this gives
q0(V)=νNVϑ(M−V),dq0dV=νNMϑ(M−V)2, | (4.12) |
which are Eq (3.4) and Eq (3.5), respectively.
Gathering the first orders of ε and assuming V>0 results in the following formula, accurate of order O(1):
dq1dV(ϑN(M−V)q0(V)−νV)+dq0dVϑN(M−V)q1(V)=βM(N−q0(V))V−μq0(V). |
or using Eq (4.12)
dq1dV(ϑN(M−V)νNVϑ(M−V)−νV)+νNMϑ(M−V)2(ϑN(M−V)q1(V))=βM(N−νNVϑ(M−V))V−μνNVϑ(M−V), |
or, further
dq1dV(νV−νV)+νMM−Vq1(V)=βM(N−νNVϑ(M−V))V−μνNVϑ(M−V), |
or, finally:
q1(V)=(βνM(M−V−νϑV)−μϑ)NVM. | (4.13) |
In order to get the slow dynamics along this curve we can solve the ODE for the reduced one-dimensional system
dIdt=β(N−I)p(I)−μI | (4.14) |
where p(I)=q−1(I)=V.
Alternatively the same asymptotic expansion procedure as for I=q(V,ε) yielding Eq (4.9) can be used for p(I,ε). The results are not shown here.
We recall the three-dimensional SIRUV model (2.1)
dSdt=εg1(S,I,V)=ε(−βMSV+μ(N−S)), | (5.1a) |
dIdt=εg2(S,I,V)=ε(βMSV−(γ+μ)I), | (5.1b) |
dVdt=f(S,I,V)=ϑN(M−V)I−νV. | (5.1c) |
There is a trivial equilibrium S0=N,I0=0,V0=0 and an interior equilibrium (whenever ν(μ+γ)<ϑβ) given by:
S∗=Nν(γ+μ)+μϑϑ(β+μ),I∗=μNβϑ−ν(γ+μ)ϑ(β+μ)(γ+μ),V∗=μMβϑ−ν(γ+μ)β(ν(γ+μ)+μϑ), | (5.2) |
and furthermore R∗=N−(S∗+I∗) and U∗=M−V∗.
The biologically relevant domain for (2.1) is Ω={(S,I,V)|0≤S+I≤N,0≤S≤N,0≤I≤N,0≤V≤M}. Following [21,Lemma 2.1], one can demonstrate that Ω is positively invariant under (2.1). The details are not shown here.
Theorem 1. When the interior equilibrium (5.2) exists, it is locally asymptotically stable and a spiral as long as μ is sufficiently small.
Proof. We provide the proof for the reference values β=2γ,ϑ=2ν in Table 1. The Jacobian computed at the interior equilibrium gives a characteristic polynomial
J(λ)=(2γ3+9γ2μ+10γμ2+3μ3)λ3+(2γ4+19γ3μ+2νγ3+35γ2μ2+12νγ2μ+23γμ3+18νγμ2+5μ4)λ2+μ(8γ4+24γ3μ+11νγ3+26γ2μ2+39νγ2μ+12γμ3+17νγμ2+2μ4−3νμ3)λ+μν(6γ4+25γ3μ+21γ2μ2−γμ3−3μ4) |
Since μ is the smallest parameter in the coefficients of J, all coefficients are {positive} and Descartes' rule of signs shows that J has no positive real roots. Similarly, because γ is the dominant parameter, the coefficient of λ2 is the largest with respect to those of λ3 and λ, hence, the local extrema of J are both negative. A result on the geometric interpretation of the complex conjugate roots of a cubic [29], shows that these have negative real parts. Therefore, the interior equilibrium (S∗,I∗,V∗) is a spiral.
For the reference parameter values, the slow variables S,I enslave the fast variables V (Figure 8). There is rapid approach to the locus (V∗(S),I∗(S)) shown by the dotted line and fast switching between values near the origin and the interior equilibria.
Applying the singular perturbation technique for the SIRUV model (5.1) is now more complicated than for the SISUV model (3.1). The slow manifold is in this case two-dimensional. Using the time-scale argument similar as for Eq (3.3) we get for ε=0 the two-dimensional f-nullspace, the set
{V(S,I)|g1(S,V,I)=0,g2(S,V,I)=0,0≤V≤M,0≤S,0≤I,S+I≤N} | (5.3) |
which is the critical manifold M of the system (2.1) with
dSdt=g1(V,I,0)=−βMSV+μ(N−S),dIdt=g2(V,I,0)=βMSV−(γ+μ)I. | (5.4) |
In case of the SIRUV model, using the QSSA approach with ε=0, the two-dimensional slow manifold is
M={0≤S,I=νNVϑ(M−V)|S+I≤N,0≤V≤M}, | (5.5) |
Note that apparently I grows unbounded when V→M−. But this is in fact prevented by the constraint I≤N, see (5.3). The system (5.4) with V=MIνN/ϑ+I from (5.5) is the reduced-system. This hyperbolic expression for V(S,I) with saturation with respect to V is the same as that for V(I) in the SISUV model (4.12).
It is interesting to compare model (2.1) with the simplest host-only model where the vector dynamics is taken into account implicitly, and where contact rates between the host individuals are described by the law of mass action. To this end we use the linearized version of the inverse of the expression I=νNVϑ(M−V), namely V=MIνN/ϑ+I at its origin, see also the QSSA assumption (4.7). This gives for the hidden infected vector population size V:
VQSSA=ϑMνNI. | (5.6) |
and the host-only model:
dSdt=−βϑνNSI+μ(N−S),dIdt=βϑνNSI−(γ+μ)I,dRdt=γI−μR, | (5.7) |
This version can be considered as a simplified version of the model formulated and analyzed in [3] when the size of the infected vector population is proportional to the infected host population.
The algebraic expression (5.5) for the two-dimensional slow manifold M for the infected host population size I(V) where ε=0 is the same as given in Eq (3.4) and there are no restrictions for the susceptible population size. Hence, unlike in the SISUV case we can at most express the fast variable V in terms of the slow variables. The statement of Fenichel's theorem [15,19,20] under the condition that the critical manifold M is normally hyperbolic is as follows. There exists ε0 such that for 0<ε<ε0, there are locally invariant manifolds Mε. Using its invariance, the perturbed manifold Mε can be approximated by asymptotic expansions in ε.
The invariance equation is similar to (3.8) as follows
dVdτ=∂V∂SdSdτ+∂V∂IdIdτ, | (5.8) |
where dSdτ and dIdτ are given in (5.4). With V(S,I)=p(S,I,ε) we get
dp(S,I,ε)dτ=∂p(S,I,ε)∂SdSdτ+∂p(S,I,ε)∂IdIdτ. | (5.9) |
The following asymptotic expansion in 0<ε≪1 is now introduced:
V(S,I)=p(S,I,ε)=p0(S,I)+εp1(S,I)+ε2p2(S,I)+…, | (5.10) |
hence
dp(S,I,ε)dτ=ϑN(M−V)I−νV=∂p0∂SdSdτ+∂p0∂IdIdτ+ε(∂p1∂SdSdτ+∂p1∂IdIdτ)+…. | (5.11) |
Substituting into the invariance equation (5.8) leads to
ϑN(M−p0(S,I)−εp1(S,I)−…)I−ν(p0(S,I)+εp1(S,I)+…)=ε∂p0∂S((−βMS(p0(S,I)+εp1(S,I)+…)+μ(N−S))+ε∂p0∂I(βMS(p0(S,I)+εp1(S,I)+…)−(γ+μ)I)+ε2(∂p1∂S(−βMSV+μ(N−S))+∂p1∂I(βMSV−(γ+μ)I))+…. | (5.12) |
Gathering the zero orders of ε and assuming V>0 gives the following result with O(1) accuracy:
ϑN(M−p0)I−νp0=0, |
which in turn yields
p0(S,I)=MII+νNϑ,∂p0∂S=0,∂p0∂I=MϑνN(Iϑ+Nν)2 | (5.13) |
and this is the same expression we found for the SISUV model in (4.12). This is also the same as the QSSA result in (5.5).
Gathering the first orders of ε results in the formula with accuracy O(1):
−ϑINp1(S,I)−νp1(S,I)=∂p0∂I(βMSp0(S,I)−(γ+μ)I). |
or, using (5.13),
p1(S,I)=−MνϑN2(ϑI+νN)3(βϑSIϑI+νN−(γ+μ)I). | (5.14) |
Taking only up to the first order terms into account, the reduced model is described by the two-dimensional system (5.4) with V(S,I) given by the following asymptotic expansion up to the first order:
V(S,I)=p(S,I,ε)=p0(S,I)+εp1(S,I), | (5.15) |
with p0(S,I) obtained by (5.13) and p1(S,I) by (5.14). The three-dimensional plot of p0,εp1 for the reference parameter values with ε=1/365 is shown in Figure 3. The zero-order expression, the tilted surface in the SIV-space in the left panel, gives the reduced-system (5.4) where V(S,I) is described by the zero-order asymptotic expansion (5.15). The size of the first-order term in the right panel shows that the the contribution of the term is marginal. Numerical experiments show that the use of the first-order term can be counterproductive already for ε>1/365 and that spurious equilibria can occur when the trajectory starts not close the the equilibrium.
Here we present a heuristic analysis of the trajectory of system (2.1) in the two-dimensional slow manifold. The S,I variables for the host population are slow while the variable of the vector population V is fast. However, because the variable I does not appear in the differential equation for S, it would be convenient to analyze the behavior of the fast V flow in the VI-system, assuming a fixed value of S. For a value of the slow variable S=ˉS, the VI-system reads as
dIdt=βMˉSV−(μ+γ)I, | (5.16a) |
dVdt=ϑN(M−V)I−νV. | (5.16b) |
We consider the dynamics of V,I driven by system (5.16) with ˉS acting as a parameter. Depending on the value of ˉS, this system has either one or two equilibria: a trivial one {(0,0)} or a pair {(0,0);(V∗(ˉS),I∗(ˉS))} with the interior equilibrium dependent on ˉS given by
I∗(ˉS)=βˉS(μ+γ)(1−ν(μ+γ)NϑβˉS),V∗(ˉS)=M(1−ν(μ+γ)NϑβˉS). | (5.17) |
Let Sc=ν(μ+γ)ϑβN. It is important to note that for biological relevance we have the constrains V(t)>0 and I(t)>0 and that for S<Sc both are negative in equilibrium. Furthermore, the transcritical bifurcation is degenerate at S=Sc because V=0 and I=0 at the same time.
Theorem 2. The biologically relevant domain of (5.16) is Ω={(V,I)|0≤V<M,0<I<(Nβ)/(μ+γ)}. Given initial data in Ω, the trivial equilibrium (0,0) is the single local and global asymptotically stable equilibrium of (5.16) when ˉS≤Sc, and otherwise the interior equilibrium V∗(ˉS),I∗(ˉS) is locally and globally asymptotically stable.
Proof. Denote by F the flow of the system (ddtV=f(I,V),ddtI=g2(I,V)) defined by (5.16). It is straightforward to verify that the boundary of Ω is impenetrable from the interior under F so the biologically relevant domain Ω is positively invariant under F.
Consider first a fixed 0<ˉS≤Sc. To show local asymptotic stability of (0,0), examine the eigenvalues of its respective Jacobian
(−νϑMNβˉSM−(μ+γ)). |
Its characteristic polynomial is
H(λ)=λ2+((μ+γ)+ν)λ+(ν(μ+γ)−ϑβˉSN), |
which cannot have roots with positive real part.
Fix ˉS>Sc. To show local asymptotic stability of the interior equilibrium (V∗(ˉS),I∗(ˉS)), examine the eigenvalues of its respective Jacobian
(−ϑβˉS(μ+γ)N(μ+γ)νMβˉS,βˉSM−(μ+γ)). | (5.18) |
Its characteristic polynomial is
H(λ)=λ2+((μ+γ)+ϑβˉS(μ+γ)N)λ+(ϑβˉSN−ν(μ+γ)), | (5.19) |
which cannot have roots with positive real part.
To show the global behavior it is enough to exclude the possibility of a closed orbit in Ω. It is straightforward to verify that
divF=∂fdV+∂g2dI=−μ−γ−ν−ϑNI<0, | (5.20) |
in Ω, so Bendixson's negative criterion [30,p. 329] shows that there is no limit cycle in Ω, and all trajectories starting inside Ω converge to the asymptotically stable equilibrium.
The phase portrait of the system (5.16) is shown in the Figure 4 for changing values of ˉS. Observe that the I-nullcline changes with ˉS, but the V-nullcline is independent of ˉS.
When ˉS is increasing, the I-nullcline rotates counterclockwise and system (5.16) converges to the interior equilibrium (Figure 4, left panel). When ˉS drops beyond the threshold value Sc, the I-nullcline rotates clockwise, leading to disappearance of the interior equilibrium, and system (5.16) converges to the origin (Figure 4, right panel).
To summarize, system (5.16) undergoes a bifurcation at ˉS=Sc. From the trivial branch B1={(ˉS,0,0)|0≤ˉS≤N} a second branch of interior equilibria B2={(ˉS,V∗(ˉS),I∗(ˉS))|ˉS>Sc} emanates. Note that B1 consists of 2 parts with different stability properties: when S<Sc, B1 is locally asymptotically stable, and when S>Sc, B1 is a saddle.
Next we characterize the dynamics of the slow variable S in terms of the fast variable V. As the dependence of S is explicit on V in (2.1), it is sufficient to consider the dynamics in the SV-plane. Consider the locus of points {(S,V):μ(N−S)−βMVS=0} representing the S nullcline in the system (2.1) (the dotted curve in Figure 5). First, for (S,V) below this curve, dS/dt is positive and the slow flow is oriented to the right, and for (S,V) above this curve, dS/dt is negative and the slow flow is oriented to the left.
However, when S crosses the critical value Sc the dynamics in the VI plane will rapidly change because of the reappearance or the disappearance of the interior equilibrium. The V(t) value will make a large jump toward the globally asymptotically stable equilibrium of (5.16) (shown as continuous solid line in Figure 5). This would be either an equilibrium in {(S,0,0):S<Sc} or in B2 (fast V-flow shown as long arrows).
Hence, after an initial fast convergence of (V,I) to the locus of equilibria (V∗,I∗), the slow variable S will evolve monotonically (slow flow shown as short arrows) until the VI-system is destabilized and switches between the trivial and interior equilibrium. This switching between the respective trivial and interior equilibria in the VI-plane causes the direction of the slow flow to change sign, leading to S(t) spiraling slowly into the equilibrium (S∗,V∗,I∗) which we showed to be locally asymptotically stable.
Seasonality is introduced in the non-seasonal, autonomous SIRUV-system by assuming that the size of the vector population changes in a perfect sinusoidal way, this assumption being motivated by dengue fever epidemiology [3]. The main motivation lies in being able to reproduce the observed yearly or semiyearly, see [12], cycle in dengue incidences. Note that the cause of this yearly periodic changes is outside our modeling approach but includes environmental conditions such as temperature and rainfall.
The expression for the size of the vector population M(t), which is now time-dependent, reads
M(t)=M0(1+ρcos(2πt)). | (6.1) |
In [3], where no vector dynamics is modeled, the seasonality was introduced as a sinusoidal fluctuation in the host infection rate (based on mass action contact between susceptible hosts S and infected hosts I instead of susceptible hosts S and infected vectors V). The expression (6.1) for M, with the expression for the infected host based infection rate given in (5.6), is consistent with the expression for the infection rate used in [3].
In [12], it is shown that the amplitude of the forcing function does not have any substantial influence on the system outcome if a steady state is attained, while simulations indicate that if an oscillatory dynamics arises, the amplitude of the cycles is directly proportional to the one of the forcing function.
In this section we give numerical results of the analysis of the two models: the SISUV model of Eq (2.3) and SIRUV model given by Eq (2.1). To be able to draw conclusions from the obtained results realistic empirical parameter values for the studied eco-epidemiological problem are needed. The reference parameter values used in our study are given in Table 1, with M0=10000, and taken from [9], which are realistic for the dengue fever case. There is a yearly periodic solution with fixed period equal to 1 year.
In order to apply a time-scale separation technique we have to decouple the three dimensional SIRUV-system (2.1) or (5.1) with state variables S,I,V into one system for the host with state variables S,I and one for the vector with state variable V where the rate of change for the vector is much faster than the one for the host. The ratio of these two rates is defined as ε.
This is most easily done for the SISUV-system (3.1) when we assume β=2μ and ϑ=2ν and the ratio of the population rates is β/ϑ. For the SIRUV-S,I,V system (5.1) the situation is more complex. Here we need to decouple the host S,I-system (5.1a) and (5.1b) from the vector V-system (5.1c) because V occurs on the right-hand side of system (5.1a) and (5.1b). To that end we can use the QSSA expression (4.7) (effectively the ε=0 case). The dominant (real part of the) eigenvalues of the Jacobian matrix evaluated at the equilibrium given by (5.2) are the two dominant system rates, given by the return rates to the equilibrium.
With the reference parameter values from Table 1 the calculated time-scale ratio for the host/vector system becomes ε=−0.04228/−36.51614=0.00116. In [9] and in this paper the ratio of the life expectancies μ/ν of the host and vector is used for the SISUV model giving ε=1/365=0.00274. In the case of the SIRUV model we get even μ/ν=0.000421. Note that the three values differ by a factor of approximately three and are therefore of the same order. For consistency we used the reference ratio ε=1/365=0.00274 for both models as a natural reference ratio for the host/vector return to equilibrium time-scales.
The small values justify that with the parameters values for Table 1 we can apply a time-scale argument to reduce the full three dimensional S,I,V-system (5.1) to the two dimensional S,I-system (5.1a) and (5.1b).
The trajectory with the reference parameter setting and initial conditions I=100,V=3500 in shown in Figure 1. From the initial values there is a fast phase where the variable I is almost constant. This fast transient episode ends close to the nullcline f(V,I,ε) and the trajectory follows this slow manifold and rests at the equilibrium point. Considered as an initial value problem for points far from the slow manifold the first episode is simple, there is an instantaneous reset at the quasi-steady state equilibrium, but this holds only for small ε.
For moderate ε values we can still do the reduction, but only when the initial condition is close but not at the slow manifold. To that end we use the parameter expansion in ε explained in the previous section. For larger ε no reduction is obtained, one has to solve the two-dimensional original system either analytically or numerically.
We study the case with parameter values in Table 1 except for the ratio between the rates of the host and vector which is now ε=60/365 instead of default value ε=1/365. The results are shown in Figure 6. The initial point is the same as in Figure 1. The difference in time scale is reduced and ε is moderately small but not very small as can be seen from the initial part of the trajectory, which differs from a vertical orbit a lot. Nevertheless, when the trajectory approaches the stable equilibrium it is for a large region along the calculated invariant manifold Mε with first order approximation in the asymptotic expansion in ε derived here, which is itself an approximation of the stable manifold belonging to the dominant negative eigenvalue of the Jacobian of the stable equilibrium. This approximation is better than that obtained for the zero-order approximation with ε=0.
Figure 7 gives a trajectory of SIRUV-system (2.1) in the three-dimensional phase-space. With parameter values in Table 1 there is convergence to the spiral stable interior equilibrium (5.2). The projection of the trajectory on the I,V-plane is shown in Figure 2. This shows that the asymptotic dynamics occurs close the the plane V=q(I) given in (4.7) with the saturated incidence rate. Figure 8 gives the results where this relationship is replaced by the linear relationship (5.6) without incidence saturation.
The numerical bifurcation analysis was performed using AUTO [31] with the reference parameters values from [9] given in Table 1 for the SIRUV model. The one-parameter diagram for parameter β in Figure 9 shows equilibrium values of the susceptible hosts S, infected hosts I and the infected vectors V. The solid lines represent the non-seasonal system and the dashed lines denote the maximum and minimum values in the annually fluctuating system.
In both the non-seasonal and seasonal case there is a transcritical bifurcation TC that separates the parameter space into two regimes where the system is disease-free and endemic. In the non-seasonal case the TC occurs where one eigenvalue of the Jacobian matrix evaluated at the disease-free equilibrium is zero and in the seasonal case where one Floquet multiplier of the limit cycle equals one.
The numerical results suggest that both TC's occur at the same parameter threshold value. For the non-seasonal case, below the TC the system is disease-free, that is S=N and U=M, and both infected I=0 and recovered R=0 host populations and infected vector V=0 are extinct. For the seasonal case, below the TC the susceptible vector population U(t) oscillates with the same phase and amplitude of the forcing given by Eq (6.1) U(t)=M(t), while the infected population is extinct V(t)=0. For the host population the susceptible population is constant at S(t)=N=10000 while the infected I(t)=0 and the recovered R(t)=N−S(t)+I(t)=0 are extinct.
Above the TC the disease is endemic and all populations oscillate between minimum and maximum values shown in Figure 9 with period of 1 year, equal to that of the forcing function.
In Figure 10 the resulting limit cycles by seasonal forcing are shown for various parameter ρ-values where β=104.
The two-parameter bifurcation diagram for the two infection rates β of the host and ϑ for the vector is shown in Figure 11. The transcritical bifurcation TC-curve for the non-seasonal system is described by Eq (4.6). The TC-curve for the seasonal case is almost the same; the curves in both cases are indistinguishable in the figure.
In many dengue epidemic models neither the epidemics nor the ecology of the vector population is modeled explicitly. In the case of dengue fever one vector is the mosquito Aedes aegypti which has a very complex life-cycle with four stages: egg (embryo), larva, pupa (embryo) (in the water phase) and imago (adult). When effects of vector-mosquito control are investigated, an accurate vector population model describing the multiple dengue-strain virus outbreak and spread coupled with a human-host population model is required. This paper is a first step toward an eco-epidemiological approach where we use simplified models for both the host and the vector dynamics.
In [9] it was investigated how far the fast time scale of the mosquito epidemiology can be slaved by the slower human epidemiology, so that for the understanding of human disease data, mainly the dynamics of the human time scale is essential and only slightly perturbed by the mosquito dynamics. It was concluded that in a very simple mono-strain SIS host model including vector dynamics, this vector dynamics is slaved by the host dynamics, owing to its slower evolution in time.
In this paper we used the parameter values from [9] which are realistic for the dengue fever case. This means that the results from that paper can be directly compared to those obtained here, which in some cases are supplementary. In [9] a center-manifold approach is used via eigenvectors of zero eigenvalues at the equilibrium point while here an invariant manifold together with an asymptotic expansion in the perturbation parameter is used. This facilitates the approximation when the differences between time scales of host and vector are moderate and not very large. The results obtained show that in those cases modeling of the vector becomes important. Furthermore, as demonstrated by experimental host-pathogen models [32,33,34], pathogenic virus aligns its behavior differently in the target cells of the vector and the host in order to maximize its chance of further transmission. Additional effects such as pest control or personal protection also influence the vectors, factors that speak in favor for an explicit inclusion of the vector dynamics into the epidemic model.
The zero-order assumption, equivalent to the quasi-steady-state, gives a mechanistic derivation of the hyperbolic relationship where the number of contacts saturate with increasing population densities. This resembles the derivation of the Holling type Ⅱ functional response in the case of a predator-prey system.
Another result is that the transcritical bifurcation of the non-seasonal and seasonal forced system by annual abundance cyclic change of the number of mosquitoes that separates the parameter space into two regimes where the system is disease-free and endemic occur at the same parameter value.
In the near future we will use this approach and the obtained results with the analysis of a much more complex eco-epidemiological model for dengue fever by taking explicitly the vector dynamics into account. Examples are the evaluation of vaccines, vector control by application of insecticides, releasing of male mosquitoes infected by a special strain of Wolbachia [35] and potential release of genetically modified mosquitoes [36].
This publication is based upon work from COST Action CA16227 Investigation & Mathematical Analysis of Avant-garde Disease Control via Mosquito Nano-Tech-Repellents, supported by COST (European Cooperation in Science and Technology). Weblink www.cost.eu. Peter Rashkov acknowledges financial support from the Bulgarian National Fund for Scientific Research (FNI) under contract DKOST01/29 and would like to thank the Mathematical Biosciences Institute (funded from the National Science Foundation Division of Mathematical Sciences and supported by The Ohio State University) for the helpful discussions during the Emphasis Semester on Infectious Diseases: Data, Modeling, Decisions-Spring 2018. Maira Aguiar has received funding from the European Union's Horizon 2020 research and innovation programme under the Marie Skłodowska-Curie grant agreement No 792494. Nico Stollenwerk is supported by National Funding from FCT-Fundação para a Ciência e a Tecnologia, under the project: UID/MAT/04561/2019, and supported as an Investigador FCT.
The authors declare there is no conflict of interest.
[1] | N. Ferguson, R. Anderson and S. Gupta, The effect of antibody-dependent enhancement on the transmission dynamics and persistence of multiple-strain pathogens, Proc. Natl. Acad. Sci. USA, 96 (1999), 790–794. |
[2] | L. Billings, I. B. Schwartz, L. B. Shaw, et al., Instabilities in multiserotype disease models with antibody-dependent enhancement, J. Theor. Biol., 246 (2007), 18–27. |
[3] | M. Aguiar, S. Ballesteros, B. W. Kooi, et al., The role of seasonality and import in a minimalistic multi-strain dengue model capturing differences between primary and secondary infections: complex dynamics and its implications for data analysis, J. Theor. Biol., 289 (2011), 181–196. |
[4] | B. W. Kooi, M. Aguiar and N. Stollenwerk, Bifurcation analysis of a family of multi-strain epidemiology models, J. Comput. Appl. Math., 252 (2013), 148–158. |
[5] | T.-T. Zheng and L.-F. Nie, Modelling the transmission dynamics of two-strain Dengue in the presence awareness and vector control, J. Theor. Biol., 443 (2018), 82–91. |
[6] | Z. Feng and J. X. Velasco-Hernandez, Competitive exclusion in a vector-host model for the dengue fever, J. Math. Biol., 35 (1997), 523–544. |
[7] | M. Zhu and Y. Xu, A time-periodic dengue fever model in a heterogeneous environment, Math. Comput. Simulat., 155 (2019), 115–129. |
[8] | B. Althouse, J. Lessler, A. Sall, et al., Synchrony of sylvatic dengue isolations: A multi-host, multi-vector SIR model of dengue virus transmission in Senegal, PLoS Negl. Trop. Dis., 6 (2012), e1928. |
[9] | F. Rocha, M. Aguiar, M. Souza, et al., Time-scale separation and centre manifold analysis describing vector-borne disease dynamics, Int. J. Comput. Math., 90 (2013), 2105–2125. |
[10] | A. Segel and M. Slemrod, The Quasi-Steady-State Assumption: A case study in perturbation, SIAM Rev., 31 (1989), 446–477. |
[11] | F. Rocha, L. Mateus, U. Skwara, et al., Understanding dengue fever dynamics: a study of seasonality in vector-borne disease models, Int. J. Comput. Math., 93 (2016), 1405–1422. |
[12] | T. G¨otz, K. P. Wijaya and E. Venturino, Introducing seasonality in an SIR-UV epidemic model: an application to dengue, in 18th International Conference on Computational and Mathematical Methods in Science and Engineering, CMMSE 2018 (ed. J. V. Aguiar), Wiley Online Library, 2018, Online ISSN:2577-7408. |
[13] | Y. Nagao and K. Koelle, Decreases in dengue transmission may act to increase the incidence of dengue hemorrhagic fever, Proc. Natl. Acad. Sci. USA, 105 (2008), 2238–2243. |
[14] | C. K. R. T. Jones, Geometric singular perturbation theory, in Dynamical Systems: Lectures Given at the 2nd Session of the Centro Internazionale Matematico Estivo (C.I.M.E.) held in Montecatini Terme, Italy, June 13–22, 1994 (ed. R. Johnson), Springer, Berlin, Heidelberg, 1995, 44–118. |
[15] | G. Hek, Geometric singular perturbation theory in biological practice, J. Math. Biol., 60 (2010), 347–386. |
[16] | B. W. Kooi and J.-C. Poggiale, Modelling, singular perturbation and bifurcation analyses of bitrophic food chains, Math. Biosci., 301 (2018), 93–110. |
[17] | C. S. Holling, Some characteristics of simple types of predation and parasitism, Can. Entomol., 91 (1959), 385–398. |
[18] | M. L. Rosenzweig and R. H. MacArthur, Graphical representation and stability conditions of predator-prey interactions, Am. Nat., 97 (1963), 209–223. |
[19] | N. Fenichel, Persistence and smoothness of invariant manifolds for flows, Indiana U. Math. J., 21 (1971), 193–226. |
[20] | N. Fenichel, Geometric singular perturbation theory, J. Differ. Equations, 31 (1979), 53–98. |
[21] | A. Korobeinikov, Lyapunov functions and global properties for SEIR and SEIS epidemic models, Math. Med. Biol., 21 (2004), 75–83. |
[22] | M. Kot, Elements of Mathematical Ecology, Cambridge University Press, Cambridge, 2001. |
[23] | O. Diekmann, J. A. P. Heesterbeek and J. A. J. Metz, On the definition and the computation of the basic reproduction ratio R0 in models for infectious diseases in heterogeneous populations, J. Math. Biol., 28 (1990), 365–382. |
[24] | O. Diekmann and J. A. P. Heesterbeek, Mathematical epidemiology of infectious diseases, Wiley series in mathematical and computional biology, Wiley, Chichester, 2000. |
[25] | V. Capasso and G. Serio, A generalization of the Kermack-McKendrick deterministic epidemic model, Math. Biosci., 42 (1978), 43–61. |
[26] | K. Dietz, Overall population patterns in the transmission cycle of infectious disease agents, in Population biology of infectious diseases (eds. R. M. Anderson and R. M. May), Springer, Berlin, Heidelberg, 1982, 87–102. |
[27] | J. Mena-Lorca and H. W. Hethcote, Dynamic models of infectious diseases as regulators of population size, J. Math. Biol., 30 (1992), 693–716. |
[28] | B. W. Kooi, G. A. K. van Voorn and K. P. Das, Stabilization and complex dynamics in a predatorprey model with predator suffering from an infectious disease, Ecol. Complex., 8 (2011), 113–122. |
[29] | F. Irwin and H. N. Wright, Some properties of polynomial curves, Ann. Math. Second Series, 19 (1917), 152–158. |
[30] | L. Edelstein-Keshet, Mathematical Models in Biology, SIAM, Philadelphia, 2005. |
[31] | E. J. Doedel and B. Oldeman, AUTO 07p: Continuation and Bifurcation software for ordinary differential equations, Technical report, Concordia University, Montreal, Canada, 2009. |
[32] | A.-M. Helt and E. Harris, S-phase-dependent enhancement of dengue virus 2 replication in mosquito cells, but not in human cells, J. Virology, 79 (2005), 13218–13230. |
[33] | J. Hily, A. García, A. Moreno, M. Plaza, M.Wilkinson, A. Fereres, A. Fraile and F. García-Arenal, The relationship between host lifespan and pathogen reservoir potential: An analysis in the system Arabidopsis thaliana-Cucumber mosaic virus, PLoS Pathog., 10 (2014), e1004492. |
[34] | N. M. Nguyen, D. Thi Hue Kien, T. V. Tuan, et al., Host and viral features of human dengue cases shape the population of infected and infectious Aedes aegypti mosquitoes, Proc. Natl. Acad. Sci. USA, 110 (2013), 9072–9077. |
[35] | J. Yu, Modeling mosquito population suppression based on delay differential equations, SIAM J. Appl. Math., 78 (2018), 3168–3187. |
[36] | M. R. W. de Valdez, D. Nimmo, J. Betz, et al., Genetic elimination of dengue vector mosquitoes, Proc. Natl. Acad. Sci. USA, 108 (2011), 4772–4775. |
1. | Peter Rashkov, Bob W. Kooi, Complexity of host-vector dynamics in a two-strain dengue model, 2021, 15, 1751-3758, 35, 10.1080/17513758.2020.1864038 | |
2. | KKWH Erandi, SSN Perera, AC Mahasinghe, Analysis and forecast of dengue incidence in urban Colombo, Sri Lanka, 2021, 18, 1742-4682, 10.1186/s12976-020-00134-7 | |
3. | Peter Rashkov, A model for a vector-borne disease with control based on mosquito repellents: A viability analysis, 2021, 498, 0022247X, 124958, 10.1016/j.jmaa.2021.124958 | |
4. | Peter Rashkov, Modeling repellent-based interventions for control of vector-borne diseases with constraints on extent and duration, 2022, 19, 1551-0018, 4038, 10.3934/mbe.2022185 | |
5. | Vanessa Steindorf, Sergio Oliva, Jianhong Wu, Maíra Aguiar, Chunrui Zhang, Effect of General Cross-Immunity Protection and Antibody-Dependent Enhancement in Dengue Dynamics, 2022, 2022, 2577-7408, 1, 10.1155/2022/2074325 | |
6. | K. K. W. H. Erandi, S. S. N. Perera, A. C. Mahasinghe, 2021, Chapter 20, 978-981-16-4771-0, 253, 10.1007/978-981-16-4772-7_20 | |
7. | Leon Diniz Alves, Raquel Martins Lana, Flávio Codeço Coelho, A Framework for Weather-Driven Dengue Virus Transmission Dynamics in Different Brazilian Regions, 2021, 18, 1660-4601, 9493, 10.3390/ijerph18189493 | |
8. | J. Banasiak, S.Y. Tchoumi, Multiscale malaria models and their uniform in-time asymptotic analysis, 2024, 221, 03784754, 1, 10.1016/j.matcom.2024.02.015 | |
9. | Piyumi Chathurangika, Lakmini S. Premadasa, S. S. N. Perera, Kushani De Silva, Determining dengue infection risk in the Colombo district of Sri Lanka by inferencing the genetic parameters of Aedes mosquitoes, 2024, 24, 1471-2334, 10.1186/s12879-024-09878-w | |
10. | Matthew D. Johnston, Bruce Pell, David A. Rubel, A two-strain model of infectious disease spread with asymmetric temporary immunity periods and partial cross-immunity, 2023, 20, 1551-0018, 16083, 10.3934/mbe.2023718 | |
11. | Vanessa Steindorf, Akhil Kumar Srivastav, Nico Stollenwerk, Bob W. Kooi, Maíra Aguiar, Beyond the biting - limited impact of explicit mosquito dynamics in dengue models, 2024, 24, 1471-2334, 10.1186/s12879-024-09995-6 | |
12. | Florin Avram, Rim Adenane, Lasko Basnarkov, Some Probabilistic Interpretations Related to the Next-Generation Matrix Theory: A Review with Examples, 2024, 12, 2227-7390, 2425, 10.3390/math12152425 | |
13. | Panagiotis Kaklamanos, Andrea Pugliese, Mattia Sensi, Sara Sottile, A Geometric Analysis of the SIRS Model with Secondary Infections, 2024, 84, 0036-1399, 661, 10.1137/23M1565632 | |
14. | Akhil Kumar Srivastav, Vanessa Steindorf, Nico Stollenwerk, Maíra Aguiar, The effects of public health measures on severe dengue cases: An optimal control approach, 2023, 172, 09600779, 113577, 10.1016/j.chaos.2023.113577 | |
15. | Urszula Skwara, Dorota Mozyrska, Maira Aguiar, Nico Stollenwerk, Dynamics of vector-borne diseases through the lens of systems incorporating fractional-order derivatives, 2024, 181, 09600779, 114643, 10.1016/j.chaos.2024.114643 | |
16. | Maíra Aguiar, Vizda Anam, Konstantin B. Blyuss, Carlo Delfin S. Estadilla, Bruno V. Guerrero, Damián Knopoff, Bob W. Kooi, Luís Mateus, Akhil Kumar Srivastav, Vanessa Steindorf, Nico Stollenwerk, Prescriptive, descriptive or predictive models: What approach should be taken when empirical data is limited? Reply to comments on “Mathematical models for Dengue fever epidemiology: A 10-year systematic review”, 2023, 46, 15710645, 56, 10.1016/j.plrev.2023.05.003 | |
17. | R. Prem Kumar, G.S. Mahapatra, Sanjoy Basu, P.K. Santra, Global stability and sensitivity analysis of dengue transmission using four host and three vector classes along with control strategies, 2024, 0020-7160, 1, 10.1080/00207160.2024.2431683 |
Par. | Description | Units | A | B |
Host | ||||
N | Host population size | # | 1000 | 1000 |
β | Infection rate | # y−1 | 730/7 | 0.2 |
μ | Susceptible birth rate | # y−1 | 1/65 | 0.1 |
γ | Recovery rate | # y−1 | 365/7 | n/a |
Vector | ||||
M | Vector population size | # | 10000 | 10000 |
ϑ | Infection rate | # y−1 | 0.2×365 | 0.2×365 |
ν | Susceptible birth rate | # y−1 | 0.1×365 | 0.1×365 |
ρ | Magnitude of sinusoidal fluctuation vectors | – | 0.9 | n/a |
T0 | Period of system | y | 1 | n/a |
Par. | Description | Units | A | B |
Host | ||||
N | Host population size | # | 1000 | 1000 |
β | Infection rate | # y−1 | 730/7 | 0.2 |
μ | Susceptible birth rate | # y−1 | 1/65 | 0.1 |
γ | Recovery rate | # y−1 | 365/7 | n/a |
Vector | ||||
M | Vector population size | # | 10000 | 10000 |
ϑ | Infection rate | # y−1 | 0.2×365 | 0.2×365 |
ν | Susceptible birth rate | # y−1 | 0.1×365 | 0.1×365 |
ρ | Magnitude of sinusoidal fluctuation vectors | – | 0.9 | n/a |
T0 | Period of system | y | 1 | n/a |