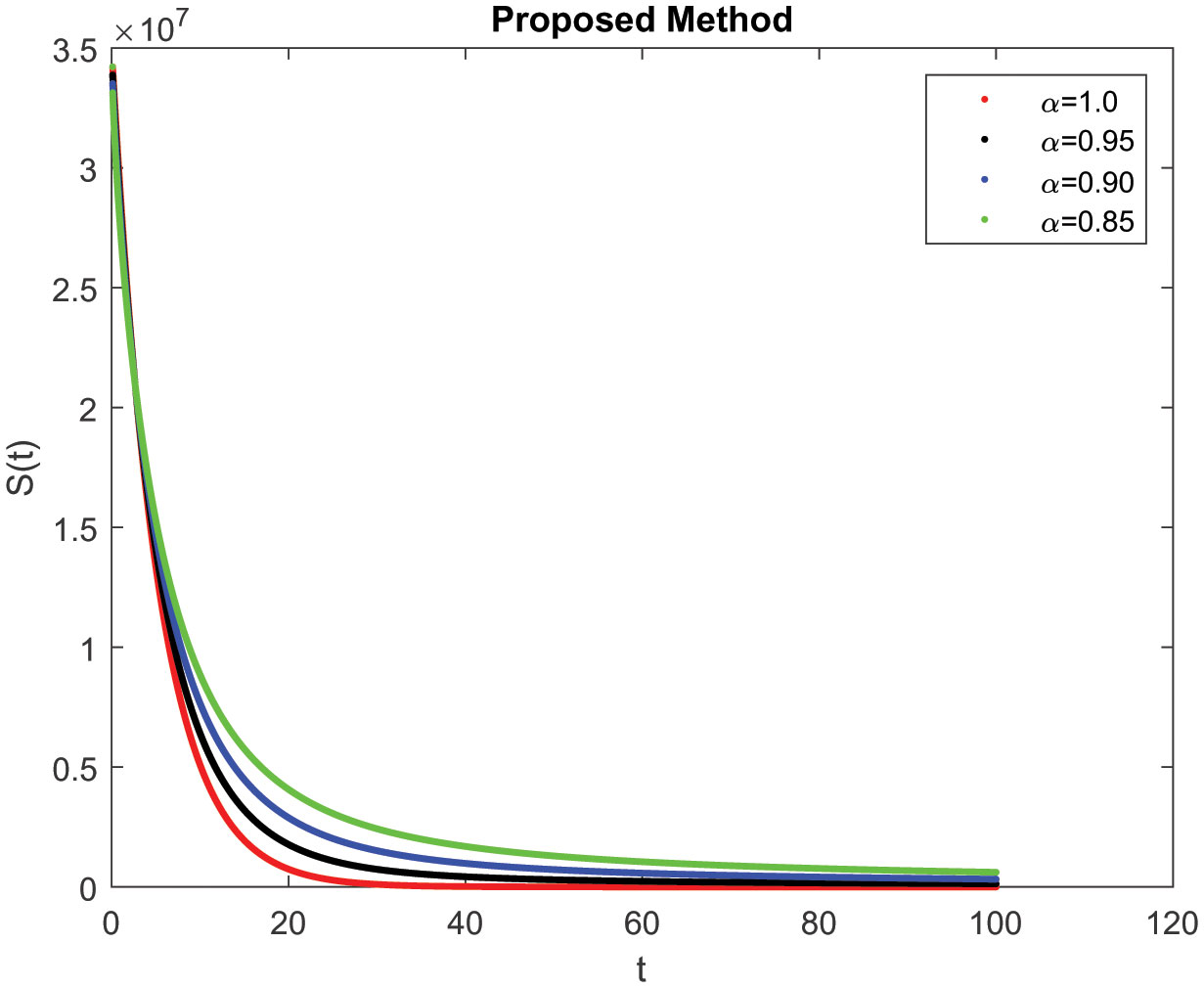
Citation: Francesco Salvarani, Gabriel Turinici. Optimal individual strategies for influenza vaccines with imperfect efficacy and durability of protection[J]. Mathematical Biosciences and Engineering, 2018, 15(3): 629-652. doi: 10.3934/mbe.2018028
[1] | Ali Moussaoui, El Hadi Zerga . Transmission dynamics of COVID-19 in Algeria: The impact of physical distancing and face masks. AIMS Public Health, 2020, 7(4): 816-827. doi: 10.3934/publichealth.2020063 |
[2] | Saina Abolmaali, Samira Shirzaei . A comparative study of SIR Model, Linear Regression, Logistic Function and ARIMA Model for forecasting COVID-19 cases. AIMS Public Health, 2021, 8(4): 598-613. doi: 10.3934/publichealth.2021048 |
[3] | Musyoka Kinyili, Justin B Munyakazi, Abdulaziz YA Mukhtar . Mathematical modeling and impact analysis of the use of COVID Alert SA app. AIMS Public Health, 2022, 9(1): 106-128. doi: 10.3934/publichealth.2022009 |
[4] | Pooja Yadav, Shah Jahan, Kottakkaran Sooppy Nisar . Analysis of fractal-fractional Alzheimer's disease mathematical model in sense of Caputo derivative. AIMS Public Health, 2024, 11(2): 399-419. doi: 10.3934/publichealth.2024020 |
[5] | Ahmed A Mohsen, Hassan Fadhil AL-Husseiny, Xueyong Zhou, Khalid Hattaf . Global stability of COVID-19 model involving the quarantine strategy and media coverage effects. AIMS Public Health, 2020, 7(3): 587-605. doi: 10.3934/publichealth.2020047 |
[6] | Mehreen Tariq, Margaret Haworth-Brockman, Seyed M Moghadas . Ten years of Pan-InfORM: modelling research for public health in Canada. AIMS Public Health, 2021, 8(2): 265-274. doi: 10.3934/publichealth.2021020 |
[7] | Kottakkaran Sooppy Nisar, Muhammad Wajahat Anjum, Muhammad Asif Zahoor Raja, Muhammad Shoaib . Recurrent neural network for the dynamics of Zika virus spreading. AIMS Public Health, 2024, 11(2): 432-458. doi: 10.3934/publichealth.2024022 |
[8] | Shahid Ahmed, Shah Jahan, Kamal Shah, Thabet Abdeljawad . On mathematical modelling of measles disease via collocation approach. AIMS Public Health, 2024, 11(2): 628-653. doi: 10.3934/publichealth.2024032 |
[9] | Rafat Zreiq, Souad Kamel, Sahbi Boubaker, Asma A Al-Shammary, Fahad D Algahtani, Fares Alshammari . Generalized Richards model for predicting COVID-19 dynamics in Saudi Arabia based on particle swarm optimization Algorithm. AIMS Public Health, 2020, 7(4): 828-843. doi: 10.3934/publichealth.2020064 |
[10] | Mario Coccia . Sources, diffusion and prediction in COVID-19 pandemic: lessons learned to face next health emergency. AIMS Public Health, 2023, 10(1): 145-168. doi: 10.3934/publichealth.2023012 |
A mathematical model is a helpful tool to recognize the conduct of an infection when it starts to affect the community and it is useful to analyze under what conditions it can be screened out or to be continued [1]. A virus is known as infectious when any disease is transferred from one person to another via different ways of transmission like droplets generated when an infected person coughs, sneezes, or exhales, or direct contact with another human, water, or any physical product. To analyze this type of transmission we need some authentic mathematical tools in which few of them are difference equations, initial conditions, working parameters, and statistical estimation. In this new era, new mathematical techniques give us more updated and reliable tools to understand many diseases or infections in epidemiology and even give us updated strategies to control disease or infection in different and suitable conditions [2].
From all of the viruses, the COVID-19 is gradually becoming a watershed pandemic in the antiquity of the planet. COVID-19 is an abbreviation of Coronavirus disease which started in 2019. In December of 2019, the first case of COVID-19 was observed in Wuhan, the city of China [3]. The common symptoms of COVID-19 are loss of smell and taste, fever, dry cough, shortening of breath, fatigue, muscle, and joint pain, phlegm production, sore throat, headache, and chills, these symptoms vary from person to person. The most common incubation period ranges from 1 to 12 days. COVID-19 spreads by physical interaction between individuals. Use of masks, sanitizer, and having a distance of 2 m between individuals results in minimizing the spread of the virus up to much extent [4]. These vaccines played a bold role in minimizing the spread of COVID-19. The main focused area of this spread is the working area, schools, offices, markets, and other open circles [5],[6].
Fractional derivative was originated in 1695. If we describe the list of fractional derivatives then it is divided into two types. Caputo, Riemann-Liouville, and Katugampola [7] are fractional derivatives with the singular kernel. Caputo-Fabrizio(exponential) [8] and ABC(Mittag-Leffler) [9] are fractional derivatives without singular kernels. Fractional calculus has very vast application properties in our daily life. It is being used in chemical, biological, physical, finance, pharmaceutical, engineering [10],[11], and many other fields [12]–[14]. FFD is mostly used because it gives a realistic way of representation of our model and hence we have used this same for representing our COVID-19 epidemics [15]–[18]. A time-fractional compartmental model for the COVID-19 pandemic [21] and classical SIR model for COVID-19 in United States is study in [22]. The COVID-19 pandemic (caused by SARS-CoV-2) has introduced significant challenges for accurate prediction of population morbidity and mortality by traditional variable-based methods of estimation. Challenges to modeling include inadequate viral physiology comprehension and fluctuating definitions of positivity between national-to-international data. This paper proposes that accurate forecasting of COVID-19 caseload may be best preformed non-perimetrically, by vector autoregressive (VAR) of verifiable data regionally [23]. Fundamental properties of the new generalized fractional derivatives in the sense of Caputo and RiemannLiouville are rigorously studied and its related work [24]–[26]. COVID-19 Decision-Making System (CDMS) was developed to study disease transmission in [27]. The change in atmospheric pollution from a public lockdown in Greece introduced to curb the spread of the COVID-19 is examined based on ground-based and satellite observations and some related issues in [28]–[30].
The fractional-order derivative of AB in Reimann Liouville-Caputo sense (ABC) [19] is given by
where Eγ is the Mittag-Leffler function and AB(γ) is a normalization function and AB(0) = AB(1) = 1. The Laplace transform of above is given by
with the aid of sumudu transformation, we get
The ABC fractional integral of order γ of a function f(t) is given by
In this section, consider the improved SEIR model given in [20] having compartments SEIQRPD, where S represents the number of uninfected individuals, E represents infected individuals at the time t but still in incubation period (without clinical symptoms and low infectivity), I represents the number of infected individuals at the time t (with obvious clinical symptoms), Q represents the number of individuals who have been diagnosed and isolated at the time t, R represents the number of recovered individuals at the time t, P represents the number of susceptible individuals who are not exposed to the external environment at the time t and D represents the number of death cases at time t.
here
applying ST operator on both sides, we get
where
taking inverse Sumudu Transform on both sides, we get
Therefore, the following is obtained
Let's consider Eq 10, and then we get
Theorem 3.1: Let
for all
Let us consider Eq 10, and we obtain
the above equation is associated with the fractional Lagrange multiplier.
Proof
Define K be a self-map is given by
Applying the properties of the norm and triangular inequality, we get
K fulfills the conditions associated with theorem 3.1 when
and we add that K is Picard K-stable.
In this section, consider the numerical simulations of the proposed scheme using the ABC technique for the fractional-order COVID-19 model. Figure 1 shows the simulation S(t) represents the number of uninfected individuals. Shows a deep decreasing curve till point (20, 0.25) and then becomes constant and reduced to zero at (100, 0). Figure 2 shows the simulation E(t) of infected individuals but still is in the incubation period (without clinical symptoms and low infectivity). Figure 3 I(t) which represents the number of infected individuals. Here the graph shows a rapid increase (10, 9) and then decrease rapidly with the same rate and then it becomes constant at (100, 0). Figure 4 represents the number of individuals who have been diagnosed and isolated. Figures 5 and 6 shows the simulation of recovered individuals and those not exposed to the external environment respectively. Figure 7 shows the simulation D(t), which represents the death due to increasing or decreasing the infection rate of COVID-19 in society. It can be easily observed from all figures the solution will converge to steady-state and lie in the bounded domain by decreasing the fractional value. Moreover, it has been demonstrated that physical processes are better well described using the derivatives of fractional order which are more accurate and reliable in comparison with the classical-order derivatives. Moreover, it can be seen from all figures that tell that all infected individual comes zero after a few days due to the quarantine effect. The behavior of the dynamics obtained for different instances of fractional-order was shown in the form of numerical results has been reported.
We consider the COVID-19 model with fractional operator for this work to check the dynamical behavior of infection of disease in society. In this regard, ABC derivative gave a realistic approach to analyze the effect of disseise during Quarantine which will be helpful for such type of epidemic. The existence and unique solution of the fractional-order model was made with the help of fixed point theory and iterative method. Numerical simulation has been made to check the actual behavior of the COVID-19 effect during quarantine which shows that infected individuals start decreasing after a few days. Such kind of results are very helpful for planning, decision-making, and developing control strategies to overcome the effect of COVID-19 in society.
[1] | [ A. Abakuks, Optimal immunisation policies for epidemics, Advances in Appl. Probability, 6 (1974): 494-511. |
[2] | [ R. M. Anderson and R. M. May, Infectious Diseases of Humans Dynamics and Control, Oxford University Press, 1992. |
[3] | [ J. Appleby, Getting a flu shot? it may be better to wait, CNN, September 15, http://edition.cnn.com/2016/09/26/health/wait-for-flu-shot/index.html, 2016. |
[4] | [ N. Bacaër, A Short History of Mathematical Population Dynamics, Springer-Verlag London, Ltd., London, 2011. |
[5] | [ Y. Bai,N. Shi,Q. Lu,L. Yang,Z. Wang,L. Li,H. Han,D. Zheng,F. Luo,Z. Zhang,X. Ai, Immunological persistence of a seasonal influenza vaccine in people more than 3 years old, Human Vaccines & Immunotherapeutics, 11 (2015): 1648-1653. |
[6] | [ C. T. Bauch,D. J. D. Earn, Vaccination and the theory of games, Proc. Natl. Acad. Sci. USA, 101 (2004): 13391-13394 (electronic). |
[7] | [ C. T. Bauch,A. P. Galvani,D. J. D. Earn, Group interest versus self-interest in smallpox vaccination policy, Proceedings of the National Academy of Sciences, 100 (2003): 10564-10567. |
[8] | [ C. T. Bauch, Imitation dynamics predict vaccinating behaviour, Proc Biol Sci, 272 (2005): 1669-1675. |
[9] | [ E. A. Belongia,M. E. Sundaram,D. L. McClure,J. K. Meece,J. Ferdinands,J. J. VanWormer, Waning vaccine protection against influenza a (h3n2) illness in children and older adults during a single season, Vaccine, 33 (2015): 246-251. |
[10] | [ Adrien Blanchet and Guillaume Carlier, From Nash to Cournot-Nash equilibria via the Monge-Kantorovich problem Philos. Trans. R. Soc. Lond. Ser. A Math. Phys. Eng. Sci., 372 (2014), 20130398, 11pp. |
[11] | [ R. Breban, R. Vardavas and S. Blower, Mean-field analysis of an inductive reasoning game: Application to influenza vaccination Phys. Rev. E, 76 (2007), 031127. |
[12] | [ D. L. Brito,E. Sheshinski,M. D. Intriligator, Externalities and compulsary vaccinations, Journal of Public Economics, 45 (1991): 69-90. |
[13] | [ B. Buonomo,A. d'Onofrio,D. Lacitignola, Global stability of an {SIR} epidemic model with information dependent vaccination, Mathematical Biosciences, 216 (2008): 9-16. |
[14] | [ P. Cardaliaguet,S. Hadikhanloo, Learning in mean field games: The fictitious play, ESAIM Control Optim. Calc. Var., 23 (2017): 569-591. |
[15] | [ F. Carrat,A. Flahault, Influenza vaccine: The challenge of antigenic drift, Vaccine, 25 (2007): 6852-6862. |
[16] | [ F. H. Chen, A susceptible-infected epidemic model with voluntary vaccinations, Journal of Mathematical Biology, 53 (2006): 253-272. |
[17] | [ M. L. Clements,B. R. Murphy, Development and persistence of local and systemic antibody responses in adults given live attenuated or inactivated influenza a virus vaccine, Journal of Clinical Microbiology, 23 (1986): 66-72. |
[18] | [ C. T. Codeço,P. M. Luz,F. Coelho,A. P Galvani,C. Struchiner, Vaccinating in disease-free regions: a vaccine model with application to yellow fever, Journal of The Royal Society Interface, 4 (2007): 1119-1125. |
[19] | [ F. Coelho and C. T. Codeço, Dynamic modeling of vaccinating behavior as a function of individual beliefs PLoS Comput Biol, 5 (2009), e1000425, 10pp. |
[20] | [ M.-G. Cojocaru, Dynamic equilibria of group vaccination strategies in a heterogeneous population, Journal of Global Optimization, 40 (2008): 51-63. |
[21] | [ R. B. Couch,J. A. Kasel, Immunity to influenza in man, Annual Reviews in Microbiology, 37 (1983): 529-549. |
[22] | [ N. Cox, Influenza seasonality: Timing and formulation of vaccines, Bulletin of the World Health Organization, 92 (2014): 311-311. |
[23] | [ O. Diekmann and J. A. P. Heesterbeek, Mathematical Epidemiology of Infectious Diseases. Model Building, Analysis and Interpretation, Wiley Series in Mathematical and Computational Biology. John Wiley & Sons, Ltd., Chichester, 2000. |
[24] | [ Josu Doncel, Nicolas Gast, and Bruno Gaujal, Mean-Field Games with Explicit Interactions, working paper or preprint, 2016. |
[25] | [ A. d'Onofrio,P. Manfredi,E. Salinelli, Vaccinating behaviour, information, and the dynamics of SIR vaccine preventable diseases, Theoretical Population Biology, 71 (2007): 301-317. |
[26] | [ A. d'Onofrio,P. Manfredi,E. Salinelli, Fatal SIR diseases and rational exemption to vaccination, Mathematical Medicine and Biology, 25 (2008): 337-357. |
[27] | [ P. Doutor,P. Rodrigues,M. do Céu Soares,F. A. C. C. Chalub, Optimal vaccination strategies and rational behaviour in seasonal epidemics, Journal of Mathematical Biology, 73 (2016): 1437-1465. |
[28] | [ J. Dushoff,J. B Plotkin,C. Viboud,D. J. D. Earn,L. Simonsen, Mortality due to influenza in the United States-an annualized regression approach using multiple-cause mortality data, American journal of epidemiology, 163 (2006): 181-187. |
[29] | [ J. M. Ferdinands,A. M. Fry,S. Reynolds,J. G. Petrie,B. Flannery,M. L. Jackson,E. A. Belongia, Intraseason waning of influenza vaccine protection: Evidence from the us influenza vaccine effectiveness network, 2011-2012 through 2014-2015, Clinical Infectious Diseases, 64 (2017): p544. |
[30] | [ P. E. M. Fine,J. A. Clarkson, Individual versus public priorities in the determination of optimal vaccination policies, American Journal of Epidemiology, 124 (1986): 1012-1020. |
[31] | [ P. J. Francis, Optimal tax/subsidy combinations for the flu season, Journal of Economic Dynamics and Control, 28 (2004): 2037-2054. |
[32] | [ D. Fudenberg and D. K. Levine, The Theory of Learning in Games volume 2 of MIT Press Series on Economic Learning and Social Evolution, MIT Press, Cambridge, MA, 1998. |
[33] | [ S. Funk,M. Salathé,V. A. A. Jansen, Modelling the influence of human behaviour on the spread of infectious diseases: A review, Journal of The Royal Society Interface, 7 (2010): 1247-1256. |
[34] | [ A. P. Galvani,T. C. Reluga,G. B. Chapman, Long-standing influenza vaccination policy is in accord with individual self-interest but not with the utilitarian optimum, Proceedings of the National Academy of Sciences, 104 (2007): 5692-5697. |
[35] | [ P.-Y. Geoffard,T. Philipson, Disease eradication: Private versus public vaccination, The American Economic Review, 87 (1997): 222-230. |
[36] | [ N. C. Grassly,C. Fraser, Seasonal infectious disease epidemiology, Proceedings of the Royal Society of London B: Biological Sciences, 273 (2006): 2541-2550. |
[37] | [ S. Greenland and R. R. Frerichs, On measures and models for the effectiveness of vaccines and vaccination programmes, International Journal of Epidemiology, 17 (1988), p456. |
[38] | [ M. E. Halloran, I. M. Longini and C. J. Struchiner, Design and Analysis of Vaccine Studies, Statistics for Biology and Health. Springer New York, 2009. |
[39] | [ H. W. Hethcote,P. Waltman, Optimal vaccination schedules in a deterministic epidemic model, Mathematical Biosciences, 18 (1973): 365-381. |
[40] | [ M. Huang,R. P. Malhamé,P. E. Caines, Nash equilibria for large-population linear stochastic systems of weakly coupled agents, In Elkébir Boukas and Roland P. Malhamé, editors,, Analysis, Control and Optimization of Complex Dynamic Systems, Springer US,, 4 (2005): 215-252. |
[41] | [ M. Huang,R. P. Malhamé,P. E. Caines, Large population stochastic dynamic games: Closed-loop mckean-vlasov systems and the Nash certainty equivalence principle, Commun. Inf. Syst., 6 (2006): 221-252. |
[42] | [ R. Jordan,D. Kinderlehrer,F. Otto, The variational formulation of the Fokker-Planck equation, SIAM J. Math. Anal., 29 (1998): 1-17. |
[43] | [ S. Kakutani, A generalization of Brouwer's fixed point theorem, Duke Math. J., 8 (1941): 457-459. |
[44] | [ E. Kissling, B. Nunes, C. Robertson, M. Valenciano, A. Reuss, A. Larrauri, J. M. Cohen, B. Oroszi, C. Rizzo, A. Machado, D. Pitigoi, L. Domegan, I. Paradowska-Stankiewicz, U. Buchholz, A. Gherasim, I. Daviaud, J. K. Horvath, A. Bella, E. Lupulescu, J. O'Donnell, M. Korczynska, A. Moren and I.-MOVE case-control study team, I-move multicentre casecontrol study 2010/11 to 2014/15: Is there within-season waning of influenza type/subtype vaccine effectiveness with increasing time since vaccination?, Euro Surveill., 21 (2016), 30201. |
[45] | [ A. Lachapelle,J. Salomon,G. Turinici, Computation of mean field equilibria in economics, Math. Models Methods Appl. Sci., 20 (2010): 567-588. |
[46] | [ L. Laguzet,G. Turinici, Global optimal vaccination in the SIR model: Properties of the value function and application to cost-effectiveness analysis, Mathematical Biosciences, 263 (2015): 180-197. |
[47] | [ L. Laguzet,G. Turinici, Individual vaccination as Nash equilibrium in a SIR model with application to the 2009-2010 influenza A (H1N1) epidemic in France, Bulletin of Mathematical Biology, 77 (2015): 1955-1984. |
[48] | [ J.-M. Lasry,P.-L. Lions, Lions, Jeux à champ moyen. I: Le cas stationnaire,, C. R., Math., Acad. Sci. Paris, 343 (2006): 619-625. |
[49] | [ J.-M. Lasry,P.-L. Lions, Lions, Jeux à champ moyen. II: Horizon fini et contrôle optimal,, C. R., Math., Acad. Sci. Paris, 343 (2006): 679-684. |
[50] | [ J.-M. Lasry,P.-L. Lions, Mean field games, Japanese Journal of Mathematics, 2 (2007): 229-260. |
[51] | [ A. S. Monto,S. E. Ohmit,J. G. Petrie,E. Johnson,R. Truscon,E. Teich,J. Rotthoff,M. Boulton,J. C. Victor, Comparative efficacy of inactivated and live attenuated influenza vaccines, New England Journal of Medicine, 361 (2009): 1260-1267. |
[52] | [ R. Morton,K. H. Wickwire, On the optimal control of a deterministic epidemic, Advances in Appl. Probability, 6 (1974): 622-635. |
[53] | [ J. Müller, Optimal vaccination strategies-for whom?, Mathematical Biosciences, 139 (1997): 133-154. |
[54] | [ S. Ng,V. J. Fang,D. K. M. Ip,K.-H. Chan,G. M. Leung,J. S. Malik Peiris,B. J. Cowling, Estimation of the association between antibody titers and protection against confirmed influenza virus infection in children, Journal of Infectious Diseases, 208 (2013): 1320-1324. |
[55] | [ K. L. Nichol,A. Lind,K. L. Margolis,M. Murdoch,R. McFadden,M. Hauge,S. Magnan,M. Drake, The effectiveness of vaccination against influenza in healthy, working adults, New England Journal of Medicine, 333 (1995): 889-893. |
[56] | [ M. T Osterholm,N. S. Kelley,A. Sommer,E. A. Belongia, Efficacy and effectiveness of influenza vaccines: A systematic review and meta-analysis, The Lancet Infectious Diseases, 12 (2012): 36-44. |
[57] | [ T. C. Reluga,C. T. Bauch,A. P. Galvani, Evolving public perceptions and stability in vaccine uptake, Math. Biosci., 204 (2006): 185-198. |
[58] | [ T. C. Reluga,A. P. Galvani, A general approach for population games with application to vaccination, Mathematical Biosciences, 230 (2011): 67-78. |
[59] | [ S. P. Sethi,P. W. Staats, Optimal control of some simple deterministic epidemic models, J. Oper. Res. Soc., 29 (1978): 129-136. |
[60] | [ E. Shim,G. B. Chapman,J. P. Townsend,A. P. Galvani, The influence of altruism on influenza vaccination decisions, Journal of The Royal Society Interface, 9 (2012): 2234-2243. |
[61] | [ D. M. Skowronski,S. Aleina Tweed,S. Aleina Tweed,G. De Serres, Rapid decline of influenza vaccine-induced antibody in the elderly: Is it real, or is it relevant?, The Journal of Infectious Diseases, 197 (2008): 490-502. |
[62] | [ N. M. Smith, J. S. Bresee, D. K. Shay, T. M. Uyeki, N. J. Cox and R. A. Strikas, Prevention and control of influenza: Recommendations of the advisory committee on immunization practices (acip), MMWRRecomm Rep, 55 (2006), 1-42. https://www.cdc.gov/mmwr/preview/mmwrhtml/rr5510a1.htm. |
[63] | [ P. G. Smith, L. C. Rodrigues and P. E. M. Fine, Assessment of the protective efficacy of vaccines against common diseases using case-control and cohort studies, International Journal of Epidemiology, 13 (1984), 87-93. |
[64] | [ C. J. Struchiner, M. E. Halloran, J. M. Robins and A. Spielman, The behaviour of common measures of association used to assess a vaccination programme under complex disease transmission patterns-a computer simulation study of malaria vaccines, International Journal of Epidemiology, 19 (1990), 187-196. |
[65] | [ I. Swiecicki, T. Gobron and D. Ullmo, Schrödinger approach to mean field games, Phys. Rev. Lett., 116(2016), 128701. |
[66] | [ J. D Tamerius, J. Shaman, W. J. Alonso, K. Bloom-Feshbach, C. K. Uejio, An. Comrie and C. Viboud, Environmental predictors of seasonal influenza epidemics across temperate and tropical climates, PLoS Pathog, 9 (2013), e1003194. |
[67] | [ J. J. Treanor,H. K. Talbot,S. E. Ohmit,L. A. Coleman,M. G. Thompson,P.-Y. Cheng,J. G. Petrie,G. Lofthus,J. K. Meece,J. V. Williams,L. Berman,C. Breese Hall,A. S. Monto,M. R. Griffin,E. Belongia,D. K. Shay, Effectiveness of seasonal influenza vaccines in the United States during a season with circulation of all three vaccine strains, Clinical Infectious Diseases, 55 (2012): 951-959. |
[68] | [ G. Turinici, Metric gradient flows with state dependent functionals: the Nash-MFG equilibrium flows and their numerical schemes, Nonlinear Analysis 165 (2017) 163-181. |
[69] | [ R. Vardavas, R. Breban and S. Blower, Can influenza epidemics be prevented by voluntary vaccination?, PLoS Comput Biol, 3 (2007), e85. |
[70] | [ G. A. Weinberg and P. G. Szilagyi, Vaccine epidemiology: Efficacy, effectiveness, and the translational research roadmap, Journal of Infectious Diseases, 201 (2010), 1607-1610 |
[71] | [ X. Zhao,V. J. Fang,S. E. Ohmit,A. S. Monto,A. R. Cook,B. J. Cowling, Quantifying protection against influenza virus infection measured by hemagglutination-inhibition assays in vaccine trials,, Epidemiology, 27 (2016): 143-151. |
1. | Changjin Xu, Muhammad Farman, Ali Hasan, Ali Akgül, Mohammed Zakarya, Wedad Albalawi, Choonkil Park, Lyapunov stability and wave analysis of Covid-19 omicron variant of real data with fractional operator, 2022, 61, 11100168, 11787, 10.1016/j.aej.2022.05.025 |