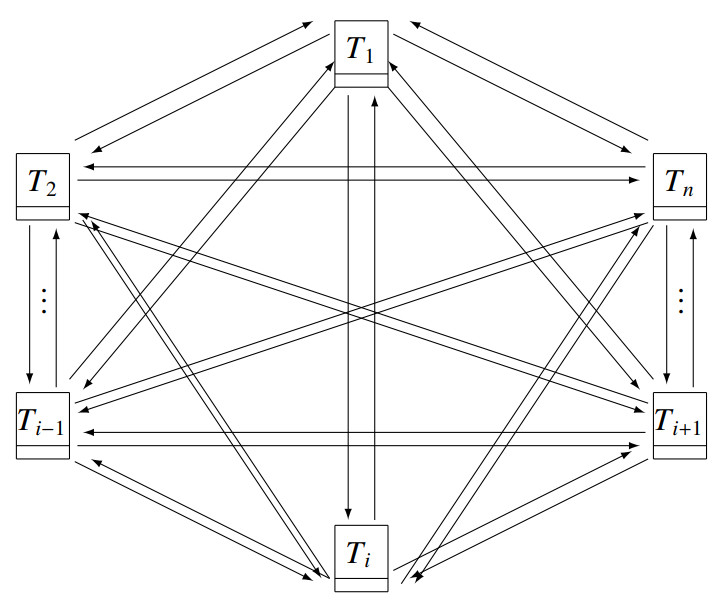
In recent years, the peer-to-peer (P2P) educational information sharing system was modeled by a system of fuzzy relation inequalities (FRIs) with addition-min or max-min composition. The max-min FRIs system was applicable to the P2P network considering the highest download traffic among the terminals. Moreover, every solution to such a max-min FRIs system corresponds exactly to one feasible flow control scheme. To embody the stability of a given feasible scheme, we introduce the concept of the widest symmetrical interval solution (WSIS), regarding the corresponding solution in the max-min FRIs system. Some effective procedures are proposed to find the WSIS regarding a provided solution. In addition, aiming to find the most stable feasible scheme, we further define the concept of a centralized solution. Some effective procedures are also proposed to find the centralized solution regarding the max-min FRIs system. Some numerical examples are provided, respectively, to demonstrate our proposed resolution procedures. Our obtained centralized solution will provide decision support for system administrators considering the stability of the feasible scheme.
Citation: Miaoxia Chen, Guocheng Zhu, Shayla Islam, Xiaopeng Yang. Centralized solution in max-min fuzzy relation inequalities[J]. AIMS Mathematics, 2025, 10(4): 7864-7890. doi: 10.3934/math.2025361
[1] | Miaoxia Chen, Abdul Samad Shibghatullah, Kasthuri Subramaniam, Xiaopeng Yang . Weighted minimax programming subject to the max-min fuzzy relation inequalities. AIMS Mathematics, 2024, 9(6): 13624-13641. doi: 10.3934/math.2024665 |
[2] | Guocheng Zhu, Zhining Wang, Xiaopeng Yang . On the minimal solution for max-product fuzzy relation inequalities. AIMS Mathematics, 2024, 9(11): 30667-30685. doi: 10.3934/math.20241481 |
[3] | Helena Myšková, Ján Plavka . Optimizing the max-min function with a constraint on a two-sided linear system. AIMS Mathematics, 2024, 9(4): 7791-7809. doi: 10.3934/math.2024378 |
[4] | Ting Xie, Dapeng Li . On the stability of projected dynamical system for generalized variational inequality with hesitant fuzzy relation. AIMS Mathematics, 2020, 5(6): 7107-7121. doi: 10.3934/math.2020455 |
[5] | Taixiang Sun, Guangwang Su, Bin Qin, Caihong Han . Global behavior of a max-type system of difference equations of the second order with four variables and period-two parameters. AIMS Mathematics, 2023, 8(10): 23941-23952. doi: 10.3934/math.20231220 |
[6] | Muhammad Bilal Khan, Muhammad Aslam Noor, Thabet Abdeljawad, Bahaaeldin Abdalla, Ali Althobaiti . Some fuzzy-interval integral inequalities for harmonically convex fuzzy-interval-valued functions. AIMS Mathematics, 2022, 7(1): 349-370. doi: 10.3934/math.2022024 |
[7] | Muhammad Bilal Khan, Pshtiwan Othman Mohammed, Muhammad Aslam Noor, Abdullah M. Alsharif, Khalida Inayat Noor . New fuzzy-interval inequalities in fuzzy-interval fractional calculus by means of fuzzy order relation. AIMS Mathematics, 2021, 6(10): 10964-10988. doi: 10.3934/math.2021637 |
[8] | Hadeel Zaki Mohammed Azumi, Wafa Mohammed Ahmed Shammakh, Abdeljabbar Ghanmi . Min-max method for some classes of Kirchhoff problems involving the ψ-Hilfer fractional derivative. AIMS Mathematics, 2023, 8(7): 16308-16319. doi: 10.3934/math.2023835 |
[9] | Ebrahim. A. Youness, Abd El-Monem. A. Megahed, Elsayed. E. Eladdad, Hanem. F. A. Madkour . Min-max differential game with partial differential equation. AIMS Mathematics, 2022, 7(8): 13777-13789. doi: 10.3934/math.2022759 |
[10] | Sana Shahab, Mohd Anjum, Ashit Kumar Dutta, Shabir Ahmad . Gamified approach towards optimizing supplier selection through Pythagorean Fuzzy soft-max aggregation operators for healthcare applications. AIMS Mathematics, 2024, 9(3): 6738-6771. doi: 10.3934/math.2024329 |
In recent years, the peer-to-peer (P2P) educational information sharing system was modeled by a system of fuzzy relation inequalities (FRIs) with addition-min or max-min composition. The max-min FRIs system was applicable to the P2P network considering the highest download traffic among the terminals. Moreover, every solution to such a max-min FRIs system corresponds exactly to one feasible flow control scheme. To embody the stability of a given feasible scheme, we introduce the concept of the widest symmetrical interval solution (WSIS), regarding the corresponding solution in the max-min FRIs system. Some effective procedures are proposed to find the WSIS regarding a provided solution. In addition, aiming to find the most stable feasible scheme, we further define the concept of a centralized solution. Some effective procedures are also proposed to find the centralized solution regarding the max-min FRIs system. Some numerical examples are provided, respectively, to demonstrate our proposed resolution procedures. Our obtained centralized solution will provide decision support for system administrators considering the stability of the feasible scheme.
In the past few decades, the research on fuzzy relation systems, including equation systems and inequality systems, has developed rapidly and comprehensively. The concept of fuzzy relation equation (FRE) was first proposed by E. Sanchez [1]. In [1], the composition operation was max-min. A sufficient and necessary condition for the solvable (having at least one solution) FRE system composed of max-min was displayed in [1]. Moreover, for the solvable system, the full solution set contains a finite set of lower solutions and a greatest solution. It was widely acknowledged that numerous issues related to body knowledge can be addressed as FRE problems [2]. In an FRE system, the initial composition max-min was soon later generalized to max-T, where T represents a triangular norm [3,4,5,6]. The structure of the solution set in a solvable max-T FRE system is the same as that in a solvable max-min one. The identification of solvability and the determination of the solution set are the central and foundational aspects of the research on FRE. Many scholars devoted their time to searching all the solutions to an FRE system, with the max-T composition [7,8,9,10,11].
J. Drewniak mentioned the fuzzy relation inequality (FRI) with max-min for the first time [12]. The max-min FRIs were completely solved in [13], using the so-called conservative path approach. In [13], the authors also studied a kind of optimization problem, in which the objective function was expressed in a latticized linear form and the constraint was the max-min FRIs. F. Guo et al. also proposed the FRI path approach for solving the minimal solutions in a max-min FRIs system [14].
In 2012, J.-X. Li et al. [15] employed a new composition operator, i.e., addition-min, in a system of FRIs. The addition-min of FRIs
∑1≤j≤naij∧xj≥bi,i=1,2,⋯,m, | (1.1) |
were introduced for describing the peer-to-peer (P2P) educational information sharing system. In such a P2P network system, n terminals are represented by the notations T1,⋯,Tn as shown in Figure 1.
In system (1.1), the variable xj represents the quality level on which the terminal Tj sends out (shares) its local data. The parameter aij represents the bandwidth. The download traffic requirement of Ti is requested to be no less than bi. Obviously, the total download traffic of the ith terminal Ti is satisfied if and only if it holds that
∑1≤j≤n(aij∧xj)≥bi. | (1.2) |
Satisfying the download traffic requirements of all the terminals, the above system (1.1) is naturally generated and established.
Some detailed properties, particularly the number of minimal solutions and the convexity of the solution set, were extensively discussed in [16]. It was highlighted in [16] that the addition-min FRIs system often has infinitely many minimal solutions. Thus, it is difficult to compute its complete solution set. Of course, when the scale of the problem is small enough, it is still possible to find out all the minimal solutions, as well as the complete solution set [17]. The min-max programming and the leximax programming were formulated and studied subject to the addition-min FRIs system [18,19,20]. Considering the random line fault [21] or the distinguishing quality levels on which the terminals send out their local files [22,23], some variants of the addition-min FRIs were also investigated.
As pointed out above, characterizing the P2P network system by the addition-min of the FRIs system (1.1), the authors only considered the total download traffic of the ith terminal. However, when considering the highest download traffic, the above system (1.1) is ineffective. In fact, the highest download traffic of the ith terminal Ti should be
⋁1≤j≤n(aij∧xj)≥bi. | (1.3) |
As a consequence, adopting the highest download traffic, the P2P educational information sharing system was characterized by the max-min FRIs below [24,25,26,27],
⋁1≤j≤n(aij∧xj)≥bi,i=1,2,⋯,m. | (1.4) |
G. Xiao et al. [25] discussed the classification of the solution set of (1.4), while X. Yang [26] attempted to find the approximate solution for the unsolvable system (1.4). Considering the rigid requirement, exactly equal to bi, system (1.4) turns out to be
⋁1≤j≤n(aij∧xj)=bi,i=1,2,⋯,m. | (1.5) |
Different resolution methods were proposed for solving the approximate solutions of the above system (1.5) [28,29] when it was unsolvable. In the solvable case, three types of geometric programming problems subject to system (1.5) were solved [30,31,32]. Considering the flexible requirement, [33,34,35] assumed the highest download traffic of Ti to be no less than ci and no more than di, and established the following max-min FRIs with bidirectional constraints.
ci≤(bi1∧y1)∨(bi2∧y2)∨⋯∨(bin∧yn)≤di,i=1,2,⋯,m. | (1.6) |
After standardization, it is assumed that ci,bij,yj,di∈[0,1], ∀i∈I,j∈J, where
I={1,…,m},J={1,…,n}. |
Similar to system (1.1) for describing the P2P educational information sharing system, any solution of system (1.6) stands for a feasible flow control scheme for the P2P network system. A given solution is indeed a feasible scheme prepared in advance. However, in the actual implementation process, the values of components in the given solution might encounter some temporary adjustment or uncontrollable variation. Thus, we consider the tolerable variation for a given solution in this work. The widest symmetrical interval solution will be defined and investigated. It embodies the tolerable variation for a given solution. Moreover, we will further define the centralized solution for system (1.6). The centralized solution is indeed the solution with the biggest tolerable variation. As is well known, a feasible scheme is considered to be more stable if it is able to bear a bigger tolerable variation. As a result, the centralized solution would be considered to be the most stable feasible scheme in the P2P network system.
The remaining sections are arranged as follows. Section 2 presents the basic foundations on the max-min FRIs system (1.6). In Section 3, we propose an effective approach for solving the widest symmetrical interval solution regarding a provided solution. In Section 4, we further provide a resolution approach for searching the centralized solution for system (1.6). Some numerical examples are enumerated for illustrating our presented resolution approaches. Section 5 concludes the work.
For convenience, we abbreviate
(bi1∧y1)∨(bi2∧y2)∨⋯∨(bin∧yn)=(bi1,⋯,bin)∘(y1,⋯,yn), |
for any i∈I. Furthermore, the above max-min FRIs system (1.6) could be rewritten in its abbreviation form as
c≤B∘y≤d, | (2.1) |
where c=(c1,⋯,cm), B=(bij)m×n, y=(y1,⋯,yn) and d=(d1,⋯,dm). Accordingly, the set of all solutions to system (1.6) or system (2.1) is exactly
S(B,c,d)={y∈V∣c≤B∘y≤d}. | (2.2) |
where V=[0,1]n.
Definition 1. (Consistent)[33,34,35] System (1.6) is called consistent if it has a solution, i.e., S(B,c,d)≠∅. Conversely, S(B,c,d)=∅; we call it inconsistent.
Definition 2. [33,34,35] ˆv∈V is a maximum solution if ˆv∈S(B,c,d) and ˆv≥v hold for any v∈S(B,c,d). vm∈V is a minimal solution if vm∈S(B,c,d) and v≤vm⇒v=vm holds for any v∈S(B,c,d).
Define the operator "@" as follows:
bij@di={1,ifbij≤di,di,ifbij>di. | (2.3) |
Let ˆv=(ˆv1,ˆv2,⋯,ˆvn), where
ˆvj=⋀i∈Ibij@di. | (2.4) |
Theorem 1. [33,34,35] S(B,c,d)≠∅ if and only if ˆv∈S(B,c,d).
Proposition 1. [33,34,35] If S(B,c,d)≠∅ and v∈S(B,c,d) is an arbitrary solution, then we have v≤ˆv.
According to Theorem 1 and Proposition 1, when (1.6) is a consistent system, ˆv is always its maximum solution.
Proposition 2. [33,34,35] Let u,v∈S(B,c,d) be two solutions of system (1.6), with u≤v. Then for any x∈[u,v], it holds x∈S(B,c,d), i.e., x is also a solution of (1.6).
Theorem 2. [33,34,35] Let system (1.6) be consistent. Then the solution set is
S(B,c,d)=⋃vm∈Sm(B,c,d)[vm,ˆv], | (2.5) |
where Sm(B,c,d) is the collection of all minimal solutions, while ˆv is the maximum one.
In this section, we posit the hypothesis that
v=(v1,v2,⋯,vn)∈V |
is one of the given solutions in the max-min FRIs system (1.6), i.e., v∈S(B,c,d). We will define the concept of the widest symmetrical interval solution regarding the provided solution v. Moreover, some effective procedures will be proposed for obtaining the widest symmetrical interval solution.
Definition 3. (Intervalsolution&width) Let u,v be two vectors in V, with u≤v. If
[u,v]⊆S(B,c,d), |
then we say [u,v] an interval solution of (1.6). Moreover, the following non-negative number, denoted by w[u,v], i.e.,
w[u,v]=(v1−u1)∧(v2−u2)∧⋯∧(vn−un) | (3.1) |
is said to be the width of the interval solution [u,v].
Remark 1. Let [u,v],[x,y]⊆S(B,c,d) be two interval solutions of (1.6). If [u,v]⊆[x,y], then it holds w[u,v]≤w[x,y]. However, this conclusion does not hold in reverse. For example, suppose [u,v]=([0.2,0.8],[0.3,0.4],[0.4,0.9]),[x,y]=([0.3,0.5],[0.4,0.6],[0.4,0.7]). It is clear that w[u,v]=0.1≤0.2=w[x,y]. However, the inclusion relation [u,v]⊆[x,y] doesn't hold.
Definition 4. (Symmetricalintervalsolution(SIS)regardingv) Let ε=(ε1,ε2,⋯,εn)∈V be an arbitrary vector. Denote the following vectors v+ε and v−ε, according to ε and v,
{v+ε=(v1+ε1,⋯,vn+εn),v−ε=(v1−ε1,⋯,vn−εn). | (3.2) |
If [v−ε,v+ε] is an interval solution of system (1.6), we say [v−ε,v+ε] a symmetricalintervalsolution regarding the provided solution v.
Obviously, if [v−ε,v+ε], represented by (3.2), is a symmetrical interval solution (SIS) regarding v, then by (3.1), its width is indeed
w[v−ε,v+ε]=2(ε1∧ε2∧⋯∧εn). | (3.3) |
Definition 5. (Widest symmetrical interval solution (WSIS) regarding v) Let [v−εv,v+εv] be an SIS regarding the provided solution v, where εv∈V. [v−εv,v+εv] is said to be a widest symmetrical interval solution regarding v, if
w[v−εv,v+εv]≥w[v−ε,v+ε] | (3.4) |
holds for any SIS [v−ε,v+ε] regarding v, where ε∈V. Moreover, the width of [v−εv,v+εv], i.e., w[v−εv,v+εv], is said to be the (biggest) symmetrical width regarding v.
According to our provided solution v, define m indicator sets as
Jvi={j∈J|bij∧vj≥ci}, | (3.5) |
where i∈I. Furthermore, define m indices as
jvi=argmax{vj|j∈Jvi}, | (3.6) |
where i∈I. Now we find the indices jv1,jv2,⋯,jvm. These indices enable us to further define
Ivj={i∈I|jvi=j}, | (3.7) |
where j∈J. Next, we could construct a vector, denoted by ˘v=(˘v1,˘v2,⋯,˘vn), in which
˘vj={⋁i∈Ivjci,ifIvj≠∅,0,ifIvj=∅,j∈J. | (3.8) |
Proposition 3. Assume that v∈S(B,c,d). Then there is Jvi≠∅ for any i∈I.
Proof. Since v∈S(B,c,d), according to system (1.6), we have
ci≤(bi1∧v1)∨(bi2∧v2)∨⋯∨(bin∧vn)≤di,∀i∈I. |
Therefore, for any i∈I, there is ji∈J satisfying
biji∧yji≥ci. |
Observing Eq (3.5), we have ji∈Jvi, i.e., Jvi≠∅.
Proposition 4. For the solution v∈S(B,c,d) of (1.6) and the vector ˘v defined by (3.8), we have ˘v≤v.
Proof. Let j∈J be an arbitrary indicator.
Case 1. If Ivj=∅, it follows from (3.8) that ˘vj=0≤vj.
Case 2. If Ivj≠∅, it follows from (3.8) that ˘vj=⋁i∈Ivjci. Take arbitrarily i∈Ivj. By (3.7), we have
jvi=j. |
According to (3.6), it is clear j=jvi∈Jvi. It follows from (3.5) that
vj≥bij∧vj≥ci. |
In view of the arbitrariness of i in Ivj, there is vj≥⋁i∈Ivjci=˘vj.
Cases 1 and 2 indicate ˘vj≤vj, ∀j∈J. Thus, we obtain ˘v≤v
Theorem 3. For the solution v∈S(B,c,d) of (1.6) and the vector ˘v defined by (3.8), there is ˘v∈S(B,c,d). That is to say, ˘v serves as a solution of (1.6).
Proof. Take arbitrarily i∈I. It is clear jvi∈Jvi by (3.6). According to (3.5), we have
bijvi≥bijvi∧vjvi≥ci. | (3.9) |
Denote j†=jvi. Then we have i∈Ivj†≠∅ by (3.7). According to (3.8), we have
˘vj†=⋁k∈Ivj†ck≥ci. | (3.10) |
Considering j†=jvi, by (3.9) and (3.10) we further have
(bi1∧˘v1)∨(bi2∧˘v2)∨⋯∨(bin∧˘vn)≥bij†∧˘vj†≥ci,∀i∈I. | (3.11) |
Since v∈S(B,c,d), according to system (1.6), we have
ci≤(bi1∧v1)∨(bi2∧v2)∨⋯∨(bin∧vn)≤di,∀i∈I. |
Thus,
bij∧vj≤di,∀i∈I,∀j∈J. |
Following Proposition 4, it holds ˘vj≤vj, j∈J. Thus we get
bij∧˘vj≤bij∧vj≤di,∀i∈I,∀j∈J, |
i.e.,
(bi1∧˘v1)∨(bi2∧˘v2)∨⋯∨(bin∧˘vn)≤di,∀i∈I. | (3.12) |
Combining Inequalities (3.11) and (3.12), it is evident to have ˘v∈S(B,c,d).
In the previous section, we have obtained the vector ˘v based on the provided solution v. Moreover, ˘v is found to be a solution of system (1.6), no more than v, i.e., ˘v≤v. In this section, we further construct a symmetrical interval solution regarding v, based on the solutions ˘v,v,ˆv.
Remind that ˆv is the maximum solution, while v is a provided solution of (1.6). Besides, ˘v is as obtained following Eqs (3.5)–(3.8). Now we denote the vector εv=(εv1,εv2,⋯,εvn) related to v as
εvj=(vj−˘vj)∧(ˆvj−vj),j∈J. | (3.13) |
Note that ˘vj≤v≤ˆv≤(1,⋯,1). It could be evidently found that 0≤εvj≤1 for all j∈J. Hence, we have εv∈V.
Next, we provide some properties on the above-obtained vector εv.
Proposition 5. Let εv∈V be the vector defined by (3.13), related to the given solution v. Then there is v+εv∈S(B,c,d), i.e., v+εv satisfies system (1.6).
Proof. Since 0≤εvj≤1 and
εvj=(vj−˘vj)∧(ˆvj−vj)≤ˆvj−vj,∀j∈J. |
we have
vj≤vj+εvj≤ˆvj,∀j∈J. |
That is v≤v+εv≤ˆv. Since v,ˆv∈S(B,c,d), following Proposition 2 we have v+εv∈S(B,c,d).
Proposition 6. Let εv∈V be the vector defined by (3.13), related to the given solution v. Then there is v−εv∈S(B,c,d).
Proof. Since 0≤εvj≤1 and
εvj=(vj−˘vj)∧(ˆvj−vj)≤vj−˘vj,∀j∈J, |
we have
˘vj≤vj−εvj≤vj,∀j∈J. |
That is ˘v≤v−εv≤v. According to Theorem 3 and the given condition, both v and ˘v are solutions to system (1.6). It follows from Proposition 2 that v−εv∈S(B,c,d).
According to the above Propositions 5 and 6, one easily discovers the Corollary 1 below.
Corollary 1. Let εv∈V be the vector defined by (3.13), related to the given solution v. Then there is [v−εv,v+εv]⊆S(B,c,d), i.e., [v−εv,v+εv] is a symmetrical interval solution regarding v.
It is shown in Corollary 1 that [v−εv,v+εv] is a symmetrical interval solution regarding v. Next, it will be further verified to be the widest one.
Proposition 7. Let u∈S(B,c,d) be a solution of (1.6) with u≤v. Then it holds w[u,v]≤w[˘v,v].
Proof. Select arbitrarily j∈J. Now let us examine the inequality w[u,v]≤vj−˘vj.
Case 1. If Ivj=∅, then ˘vj=0≤uj by (3.8). Hence
w[u,v]=⋀k∈J(vk−uk)≤vj−uj≤vj−˘vj. | (3.14) |
Case 2. If Ivj≠∅, then ˘vj=⋁i∈Ivjci by (3.8). Accordingly, there is i†∈Ivj, satisfying
˘vj=ci†. | (3.15) |
At the same time, by (3.7) we have
jvi†=j, | (3.16) |
since i†∈Ivj. Note that u∈S(B,c,d). u satisfies the i†th inequality from (1.6), i.e.,
ci†≤(bi†1∧u1)∨(bi†2∧u2)∨⋯∨(bi†n∧un)≤di†. |
As a result, there is j†∈J satisfying
bi†j†∧uj†≥ci†. | (3.17) |
Considering u≤v, we have bi†j†∧vj†≥bi†j†∧uj†≥ci†. According to (3.5), it holds that j†∈Jvi†. Moreover, Inequality (3.17) implies that
uj†≥bi†j†∧uj†≥ci†. | (3.18) |
Observing (3.6) and (3.16), we have j=jvi†=argmax{vl|l∈Jvi†}. Thus,
vj≥vl,∀l∈Jvi†. |
Thereby, j†∈Jvi† indicates
vj≥vj†. | (3.19) |
Combining (3.15), (3.18), and (3.19), we have
vj−˘vj=vj−ci†≥vj−uj†≥vj†−uj†≥⋀k∈J(vk−uk)=w[u,v]. | (3.20) |
According to Inequalities (3.14) and (3.20), for any j in J, there is vj−˘vj≥w[u,v]. So we have
w[˘v,v]=⋀j∈J(vj−˘vj)≥w[u,v]. |
The proof is complete.
Theorem 4. Let εv∈V be the vector defined by (3.13), related to the given solution v. Then [v−εv,v+εv] is the widest symmetrical interval solution regarding v.
Proof. It has been verified in Corollary 1 that [v−εv,v+εv] is an SIS regarding v.
Let [v−ε,v+ε] be an arbitrary SIS regarding the solution v, where ε∈V=[0,1]n. According to the definition of interval solution, it holds
[v−ε,v+ε]⊆S(B,c,d), |
That is to say, both v+ε and v−ε are solutions. Since ˆv is maximum in S(B,c,d), it holds that v+ε≤ˆv. Thus, for any j∈J,
vj+εj≤ˆvj, |
or written as
ˆvj−vj≥εj. |
As a result,
⋀j∈Jεj≤⋀j∈J(ˆvj−vj). | (3.21) |
In addition, since v−ε∈S(B,c,d) and v−ε≤v, it follows from Proposition 7 that
w[˘v,v]≥w[v−ε,v]. | (3.22) |
According to (3.1) and (3.2), w[v−ε,v]=ε1∧ε2∧⋯∧εn=⋀j∈Jεj, and w[˘v,v]=⋀j∈J(vj−˘vj). The above inequality (3.22) turns out to be
⋀j∈J(vj−˘vj)≥⋀j∈Jεj. | (3.23) |
Combining Inequalities (3.21), (3.23), and (3.13), it holds
⋀j∈Jεvj=⋀j∈J[(ˆvj−vj)∧(vj−˘vj)]=[⋀j∈J(ˆvj−vj)]∧[⋀j∈J(vj−˘vj)]≥⋀j∈Jεj. | (3.24) |
Consequently, it follows from (3.3) and (3.24)
w[v−εv,v+εv]=⋀j∈Jεvj≥⋀j∈Jεj=w[v−ε,v+ε]. |
Following Definition 5, [v−εv,v+εv] is the widest symmetrical interval solution regarding v.
This subsection provides an algorithm to find the widest symmetrical interval solution regarding a given solution. Moreover, some numerical examples are given for verifying our proposed algorithm.
Algorithm Ⅰ: solving the widest symmetrical interval solution regarding v
Step 1. Following (3.5), calculate the indicator set Jvi for any i∈I.
Step 2. Following (3.6), select the indicator jvi from the indicator set Jvi for any i∈I.
Step 3. Following (3.7), construct the indicator set Ivj for any j∈J.
Step 4. Following (3.8), generate the vector ˘v=(˘v1,˘v2,⋯,˘vn), where the ˘vj is defined by (3.8).
Step 5. Following (3.13), calculate the vector εv=(εv1,εv2,⋯,εvn), where the εvj is defined by (3.13).
Step 6. Construct the widest symmetrical interval solution as [v−εv,v+εv], according to (3.2).
Computational complexity of Algorithm Ⅰ: In Algorithm Ⅰ, Step 1 requires 2mn operations for calculating the indicator sets. Moreover, Steps 2–4 have an identical calculation amount as mn. Besides, Steps 5 and 6 require 3n and 2n operations, respectively. As a result, applying Algorithm Ⅰ to calculate the widest symmetrical interval solution regarding v, it requires totally
2mn+mn+mn+mn+3n+2n=5mn+5n |
operations. The computational complexity of Algorithm Ⅰ is O(mn).
Here, we have to mention that the reference [36] also proposes an algorithm to calculate the widest symmetrical interval solution regarding a given solution. However, the algorithm presented in [36] requires totally
12m2n+172mn+6n−1 |
operations. The computational complexity is O(m2n). Obviously, our proposed algorithm Ⅰ is superior to the algorithm presented in [36], considering the computational complexity.
Example 1. Consider the max-min FRIs system as follows:
{0.4≤(0.1∧y1)∨(0.7∧y2)∨(0.5∧y3)∨(0.3∧y4)∨(0.3∧y5)≤0.7,0.2≤(0.3∧y1)∨(0.5∧y2)∨(0.8∧y3)∨(0.2∧y4)∨(0.1∧y5)≤0.6,0.2≤(0.6∧y1)∨(0.1∧y2)∨(0.3∧y3)∨(0.5∧y4)∨(0.5∧y5)≤0.8,0.5≤(0.3∧y1)∨(0.2∧y2)∨(0.6∧y3)∨(0.8∧y4)∨(0.2∧y5)≤0.7. | (3.25) |
It is easy to check that both v1=(0.8,0.6,0.6,0.6,0.3), v2=(0.6,0.45,0.3,0.65,0.8) and v3=(0.4,0.6,0.52,0.2,0.4) are solutions of system (3.25). Next, try to obtain the widest symmetrical interval solution regarding vt(t=1,2,3) and calculate the (biggest) symmetrical width regarding vt(t=1,2,3).
Solution: According to (2.3) and (2.4), we find
ˆv=(1,1,0.6,0.7,1). |
as the maximum solution for (3.25) after calculation.
(ⅰ) Find the WSIS regarding v1=(0.8,0.6,0.6,0.6,0.3).
Steps 1 and 2. Following (3.5) and (3.6), we have
Jv11={j∈J|b1j∧v1j≥c1}={j∈J|b1j∧v1j≥0.4}={2,3},Jv12={j∈J|b2j∧v1j≥c2}={j∈J|b2j∧v1j≥0.2}={1,2,3,4},Jv13={j∈J|b3j∧v1j≥c3}={j∈J|b3j∧v1j≥0.2}={1,3,4,5},Jv14={j∈J|b4j∧v1j≥c4}={j∈J|b4j∧v1j≥0.5}={3,4}, |
and
jv11=argmax{v1j|j∈Jv11}=argmax{v12,v13}=2 or 3,jv12=argmax{v1j|j∈Jv12}=argmax{v11,v12,v13,v14}=1,jv13=argmax{v1j|j∈Jv13}=argmax{v11,v13,v14,v15}=1,jv14=argmax{v1j|j∈Jv14}=argmax{v13,v14}=3 or 4. |
Step 3. If we take jv11=2, jv12=1, jv13=1, jv14=4 and combine (3.7), we can obtain that
Iv11={i∈I|jv1i=1}={2,3},Iv12={i∈I|jv1i=2}={1},Iv13={i∈I|jv1i=3}=∅,Iv14={i∈I|jv1i=4}={4},Iv15={i∈I|jv1i=5}=∅. |
Step 4. Following (3.8), we find
˘v11=⋁i∈Iv11ci=c2∨c3=0.2,˘v12=⋁i∈Iv12ci=c1=0.4,˘v14=⋁i∈Iv14ci=c4=0.5,˘v13=˘v15=0. |
Step 5. As a result, we get the vector ˘v1=(0.2,0.4,0,0.5,0). Moreover,
εv11=(ˆv1−v11)∧(v11−˘v11)=(1−0.8)∧(0.8−0.2)=0.2∧0.6=0.2,εv12=(ˆv2−v12)∧(v12−˘v12)=(1−0.6)∧(0.6−0.4)=0.4∧0.2=0.2,εv13=(ˆv3−v13)∧(v13−˘v13)=(0.6−0.6)∧(0.6−0)=0∧0.6=0,εv14=(ˆv4−v14)∧(v14−˘v14)=(0.7−0.6)∧(0.6−0.5)=0.1∧0.1=0.1,εv15=(ˆv5−v15)∧(v15−˘v15)=(1−0.3)∧(0.3−0)=0.7∧0.3=0.3. |
Thus,
εv1=(0.2,0.2,0,0.1,0.3). |
Step 6. Based on the above-obtained εv1, we further obtain the widest symmetrical interval solution regarding v1 as
[v1−εv1,v1+εv1]=([0.6,1],[0.4,0.8],0.6,[0.5,0.7],[0,0.6]). |
The (biggest) symmetrical width regarding v1 is
w[v1−εv1,v1+εv1]=2(εv11∧εv12∧εv13∧εv14∧εv15)=2(0.2∧0.2∧0∧0.1∧0.3)=0. |
In fact, since \(v_{3}^{1} = \hat{v}_{3}^{1} = 0.6\), it can be concluded from equations (3.3) and (3.13) that there must be \(w[ v^1-\varepsilon^{v^1}, v^1+\varepsilon^{v^1} ] = 0\).
(ⅱ) Find the WSIS regarding v2=(0.6,0.45,0.3,0.65,0.8).
Steps 1 and 2. Following (3.5) and (3.6), we find
Jv21={j∈J|b1j∧v2j≥c1}={j∈J|b1j∧v2j≥0.4}={2},Jv22={j∈J|b2j∧v2j≥c2}={j∈J|b2j∧v2j≥0.2}={1,2,3,4},Jv23={j∈J|b3j∧v2j≥c3}={j∈J|b3j∧v2j≥0.2}={1,3,4,5},Jv24={j∈J|b4j∧v2j≥c4}={j∈J|b4j∧v2j≥0.5}={4}, |
and
jv21=argmax{v2j|j∈Jv21}=argmax{v22}=2,jv22=argmax{v2j|j∈Jv22}=argmax{v21,v22,v23,v24}=4,jv23=argmax{v2j|j∈Jv23}=argmax{v21,v23,v24,v25}=5,jv24=argmax{v2j|j∈Jv24}=argmax{v24}=4. |
Step 3. Following (3.7), we can obtain that
Iv21={i∈I|jv2i=1}=∅,Iv22={i∈I|jv2i=2}={1},Iv23={i∈I|jv2i=3}=∅,Iv24={i∈I|jv2i=4}={2,4},Iv25={i∈I|jv2i=5}={3}. |
Step 4. According to (3.8), we further obtain
˘v21=˘v23=0,˘v22=⋁i∈Iv22ci=c1=0.4,˘v24=⋁i∈Iv24ci=c2∨c4=0.5,˘v25=⋁i∈Iv25ci=c3=0.2. |
Step 5. As a result, we obtain the vector ˘v2=(0,0.4,0,0.5,0.2). Moreover,
εv21=(ˆv1−v21)∧(v21−˘v21)=(1−0.6)∧(0.6−0)=0.4∧0.6=0.4,εv22=(ˆv2−v22)∧(v22−˘v22)=(1−0.45)∧(0.45−0.4)=0.55∧0.05=0.05,εv23=(ˆv3−v23)∧(v23−˘v23)=(0.6−0.3)∧(0.3−0)=0.3∧0.3=0.3,εv24=(ˆv4−v24)∧(v24−˘v24)=(0.7−0.65)∧(0.65−0.5)=0.05∧0.15=0.05,εv25=(ˆv5−v25)∧(v25−˘v25)=(1−0.8)∧(0.8−0.2)=0.2∧0.6=0.2. |
Thus,
εv2=(0.4,0.05,0.3,0.05,0.2). |
Step 6. Based on the above-obtained εv2, we further obtain the widest symmetrical interval solution regarding v2 as
[v2−εv2,v2+εv2]=([0.2,1],[0.4,0.5],[0,0.6],[0.6,0.7],[0.6,1]). |
The (biggest) symmetrical width regarding v2 is
w[v2−εv2,v2+εv2]=2(εv21∧εv22∧εv23∧εv24∧εv25)=2(0.4∧0.05∧0.3∧0.05∧0.2)=0.1. |
(ⅲ) Find the WSIS regarding v3=(0.4,0.6,0.52,0.2,0.4).
Steps 1 and 2. Following (3.5) and (3.6), we find
Jv31={j∈J|b1j∧v3j≥c1}={j∈J|b1j∧v3j≥0.4}={2,3},Jv32={j∈J|b2j∧v3j≥c2}={j∈J|b2j∧v3j≥0.2}={1,2,3,4},Jv33={j∈J|b3j∧v3j≥c3}={j∈J|b3j∧v3j≥0.2}={1,3,4,5},Jv34={j∈J|b4j∧v3j≥c4}={j∈J|b4j∧v3j≥0.5}={3}, |
and
jv31=argmax{v3j|j∈Jv31}=argmax{v32,v33}=2,jv32=argmax{v3j|j∈Jv32}=argmax{v31,v32,v33,v34}=2,jv33=argmax{v3j|j∈Jv33}=argmax{v31,v33,v34,v35}=3,jv34=argmax{v3j|j∈Jv34}=argmax{v33}=3. |
Step 3. Following (3.7), we can obtain that
Iv31={i∈I|jv3i=1}=∅,Iv32={i∈I|jv3i=2}={1,2},Iv33={i∈I|jv3i=3}={3,4},Iv34={i∈I|jv3i=4}=∅,Iv35={i∈I|jv3i=5}=∅, |
Step 4. According to (3.8), we further obtain
˘v31=˘v34=˘v35=0,˘v32=⋁i∈Iv32ci=c1∨c2=0.4,˘v33=⋁i∈Iv33ci=c3∨c4=0.5. |
Step 5. As a result, we obtain the vector ˘v3=(0,0.4,0.5,0,0). Moreover,
εv31=(ˆv1−v31)∧(v31−˘v31)=(1−0.4)∧(0.4−0)=0.6∧0.4=0.4,εv32=(ˆv2−v32)∧(v32−˘v32)=(1−0.6)∧(0.6−0.4)=0.4∧0.2=0.2,εv33=(ˆv3−v33)∧(v33−˘v33)=(0.6−0.52)∧(0.52−0.5)=0.08∧0.02=0.02,εv34=(ˆv4−v34)∧(v34−˘v34)=(0.7−0.2)∧(0.2−0)=0.5∧0.2=0.2,εv35=(ˆv5−v35)∧(v35−˘v35)=(1−0.4)∧(0.4−0)=0.6∧0.4=0.4. |
Thus,
εv3=(0.4,0.2,0.02,0.2,0.4). |
Step 6. Based on the above-obtained εv3, we further obtain the widest symmetrical interval solution regarding v3 as
[v3−εv3,v3+εv3]=([0,0.8],[0.4,0.8],[0.5,0.54],[0,0.4],[0,0.8]). |
The (biggest) symmetrical width regarding v3 is
w[v3−εv3,v3+εv3]=2(εv31∧εv32∧εv33∧εv34∧εv35)=2(0.4∧0.2∧0.02∧0.2∧0.4)=0.04. |
Then, the problem has already been solved.
Remark 2. In fact, the widest symmetrical interval solution regarding an identical solution might be not unique. For example, considering v1=(0.8,0.6,0.6,0.6,0.3) in system (3.25), we find jv11=2 or 3, jv12=1, jv13=1, and jv14=3 or 4 in Example 1. Taking jv11=2, jv12=1, jv13=1, and jv14=4, we find the widest symmetrical interval solution regarding v1 as
[v1−εv1,v1+εv1]=([0.6,1],[0.4,0.8],0.6,[0.5,0.7],[0,0.6]), |
with the (biggest) symmetrical width w[v1−εv1,v1+εv1]=0. However, when taking jv11=3, jv12=1, jv13=1, jv14=4, the corresponding widest symmetrical interval solution is
([0.6,1],[0.2,1],0.6,[0.5,0.7],[0,0.6]). |
The corresponding (biggest) symmetrical width is also w([0.6,1],[0.2,1],0.6,[0.5,0.7],[0,0.6])=0. That is to say, we find two different widest symmetrical interval solutions regarding the solution v1=(0.8,0.6,0.6,0.6,0.3). But they have the same (biggest) symmetrical width.
Example 2. Consider the max-min FRIs system as follows:
{0.37≤(0.35∧y1)∨(0.48∧y2)∨(0.87∧y3)∨(0.63∧y4)∨(0.26∧y5)∨(0.74∧y6)≤0.82,0.34≤(0.28∧y1)∨(0.92∧y2)∨(0.76∧y3)∨(0.81∧y4)∨(0.53∧y5)∨(0.19∧y6)≤0.87,0.25≤(0.89∧y1)∨(0.76∧y2)∨(0.42∧y3)∨(0.35∧y4)∨(0.56∧y5)∨(0.75∧y6)≤0.79,0.36≤(0.33∧y1)∨(0.85∧y2)∨(0.27∧y3)∨(0.49∧y4)∨(0.93∧y5)∨(0.35∧y6)≤0.85,0.41≤(0.59∧y1)∨(0.28∧y2)∨(0.18∧y3)∨(0.66∧y4)∨(0.83∧y5)∨(0.91∧y6)≤0.89,0.32≤(0.29∧y1)∨(0.23∧y2)∨(0.31∧y3)∨(0.73∧y4)∨(0.56∧y5)∨(0.82∧y6)≤0.86. | (3.26) |
It is easy to check that system (3.26) is consistent and v=(0.65,0.48,0.51,0.43,0.39,0.45) is one of its solutions. Apply Algorithm Ⅰ to find the widest symmetrical interval solution regarding v and calculate the (biggest) symmetrical width regarding v.
Solution: According to (2.3) and (2.4), we find
ˆv=(0.79,0.87,0.82,1,0.85,0.89). |
as the maximum solution for (3.26) after calculation.
Step 1. Following (3.5), we have
Jv1={2,3,4,6},Jv2={2,3,4,5},Jv3={1,2,3,4,5,6},Jv4={2,4,5},Jv5={1,4,6},Jv6={4,5,6}. |
Step 2. Following (3.6), we have
jv1=argmax{vj|j∈Jv1}=3,jv2=argmax{vj|j∈Jv2}=3,jv3=argmax{vj|j∈Jv3}=1,jv4=argmax{vj|j∈Jv4}=2,jv5=argmax{vj|j∈Jv5}=1,jv6=argmax{vj|j∈Jv6}=6. |
Step 3. Following (3.7), we have
Iv1={i∈I|jvi=1}={3,5},Iv2={i∈I|jvi=2}={4},Iv3={i∈I|jvi=3}={1,2},Iv4={i∈I|jvi=4}=∅,Iv5={i∈I|jvi=5}=∅,Iv6={i∈I|jvi=6}={6}. |
Step 4. Following (3.8), we have
˘v1=⋁i∈Iv1ci=c3∨c5=0.41,˘v2=⋁i∈Iv2ci=c4=0.36,˘v3=⋁i∈Iv3ci=c1∨c2=0.37,˘v4=˘v5=0,˘v6=⋁i∈Iv6ci=c6=0.32. |
Hence, we obtain the vector ˘v=(0.41,0.36,0.37,0,0,0.32).
Step 5. Following (3.13), we have
εv1=(v1−˘v1)∧(ˆv1−v1)=(0.65−0.41)∧(0.79−0.65)=0.24∧0.14=0.14. |
In a similar way, we further have εv2=0.12, εv3=0.14, εv4=0.43, εv5=0.39, and εv6=0.13. Then we find the vector εv=(0.14,0.12,0.14,0.43,0.39,0.13).
Step 6. According to (3.2), we can obtain the widest symmetrical interval solution regarding v as
[v−εv,v+εv]=([0.51,0.79],[0.36,0.6],[0.37,0.65],[0,0.86],[0,0.78],[0.32,0.58]). |
Moreover, the (biggest) symmetrical width regarding v is
2(εv1∧⋯∧εv6)=0.14∧0.12∧0.14∧0.43∧0.39∧0.13=0.24. |
In the previous section, we have studied the symmetrical interval solution having the biggest width, which is named WSIS. The width of a WSIS reflects its stability, i.e., the ability to bear the tolerable variation of a provided feasible scheme. For characterizing the most stable feasible scheme, we further define the concept of a centralized solution regarding the max-min FRI system (1.6). We will proposed an effective method for solving the centralized solution.
Definition 6. (Centralized solution regarding system (1.6)) Let vC∈S(B,c,d) be a solution of (1.6). The WSIS regarding vC is [vC−εvC,vC+εvC]. Then vC is said to be a centralized solution regarding system (1.6), if
w[vC−εvC,vC+εvC]≥w[v−εv,v+εv] |
holds for any solution v∈S(B,c,d), where [v−εv,v+εv] is the WSIS regarding v.
Define the index set
Jˆvi={j∈J|bij∧ˆvj≥ci}, | (4.1) |
where i∈I, in accordance with the maximum solution ˆv. Moreover, the following m indices are induced by the above index sets:
jˆvi=argmax{ˆvj|j∈Jˆvi}, | (4.2) |
where i∈I. In addition, based on the indices jˆv1,jˆv2,⋯,jˆvm, we could define
Iˆvj={i∈I|jˆvi=j}, | (4.3) |
where j∈J. Accordingly, we construct the vector ˇv=(ˇv1,ˇv2,⋯,ˇvn) by
ˇvj={⋁i∈Iˆvjci,ifIˆvj≠∅,0,ifIˆvj=∅,j∈J. | (4.4) |
Proposition 8. Let ˇv be defined by (4.4). Then it holds ˇv∈S(B,c,d), serving as a solution of (1.6).
Proof. The given conditions indicate ci∈[0,1], ∀i∈I. According to (4.4), it is evident that ˇvj∈[0,1], ∀j∈J.
For arbitrary i†∈I, denote j†=jˆvi†. By (4.2), it is clear j†=jˆvi†∈Jˆvi†. According to (4.1), it holds
bi†j†≥bi†j†∧ˆvj†≥ci†. | (4.5) |
On the other hand, j†=jˆvi† indicates i†∈Iˆvj†, by (4.3). Hence, Iˆvj†≠∅ and by (4.4), we have ˇvj†=⋁i∈Iˆvj†ci. It follows from i†∈Iˆvj† that
ˇvj†=⋁i∈Iˆvj†ci≥ci†. | (4.6) |
Combining (4.5) and (4.6), we have
⋁j∈J(bi†j∧ˇvj)≥bi†j†∧ˇvj†≥ci†∧ci†=ci†. | (4.7) |
Note that Inequality (4.7) holds for all i†∈I. Therefore, ˇv is a solution in the inequalities (1.6).
Proposition 9. Let u∈S(B,c,d) be a solution of (1.6). Then it holds that w[ˇv,ˆv]≥w[u,ˆv].
Proof. Let j be an arbitrary index in J. Next, we first examine that w[u,ˆv]≤ˆvj−ˇvj in two cases.
Case 1. If Iˆvj=∅, then by (4.4), ˇvj=0. The given condition u∈S(B,c,d) indicates uj∈[0,1]. Hence
ˆvj−ˇvj=ˆvj−uj≤ˆvj−0≥⋀k∈J(ˆvk−uk)=w[u,ˆv]. | (4.8) |
Case 2. If Iˆvj≠∅, then by (4.4), ˇvj=⋁i∈Iˆvjci. There exists i∗∈Iˆvj such that
ci∗=⋁i∈Iˆvjci=ˇvj. | (4.9) |
Meanwhile, i∗∈Iˆvj indicates jˆvi∗=j by (4.3). The given condition u∈S(B,c,d) also indicates
(bi∗1∧u1)∨(bi∗2∧u2)∨⋯∨(bi∗n∧un)≥ci∗. | (4.10) |
There is j∗∈J such that
bi∗j∗∧uj∗≥ci∗. | (4.11) |
Inequality (4.11) implies that
uj∗≥bi∗j∗∧uj∗≥ci∗. | (4.12) |
Since ˆv is maximum and u∈S(B,c,d), we have ˆvj∗≥uj∗. Hence by (4.11) we further have
bi∗j∗∧ˆvj∗≥bi∗j∗∧uj∗≥ci∗. | (4.13) |
According to (4.1), it holds that j∗∈Jˆvi∗. Considering jˆvi∗=j and j∗∈Jˆvi∗, it follows from (4.2) that
ˆvj≥ˆvj∗. | (4.14) |
Using the inequalities (4.9), (4.12), and (4.14), we have
ˆvj−ˇvj=ˆvj−ci∗≥ˆvj−uj∗≥ˆvj∗−uj∗≥⋀k∈J(ˆvk−uk)=w[u,ˆv]. | (4.15) |
Considering the arbitrariness of the index j in J, by (4.8) and (4.15) we have ˆvj−ˇvj≥w[u,ˆv], ∀j∈J. Hence it holds w[ˇv,ˆv]=⋀j∈J(ˆvj−ˇvj)≥w[u,ˆv].
In accordance with the solutions ˆv and ˇv, we denote
vC=ˆv+ˇv2,εvC=ˆv−ˇv2. | (4.16) |
Some properties are investigated for the vectors vC and εvC as follows.
Theorem 5. Let vC and εvC be defined by (4.16). Then there is vC∈S(B,c,d). Moreover, [vC−εvC,vC+εvC] is the widest symmetrical interval solution regarding vC.
Proof. According to Proposition 8, it holds ˇv∈S(B,c,d). ˆv is the maximum solution. It is clear that ˆv≥ˇv. Then we have
vC=ˆv+ˇv2∈[ˇv,ˆv]. | (4.17) |
Hence, vC∈S(B,c,d) is a solution. Note that
{ˇv=ˆv+ˇv2−ˆv−ˇv2=vC−εvC∈S(B,c,d),ˆv=ˆv−ˇv2+ˆv+ˇv2=vC+εvC∈S(B,c,d). | (4.18) |
It follows from Definitions 3 and 4 that [vC−εvC,vC+εvC] is an SIS regarding the solution vC.
Let [vC−ε†,vC+ε†] be an arbitrary SIS regarding vC, where ε†∈V. Following Definitions 3 and 4, we obtain
vC−ε†,vC+ε†∈S(B,c,d). | (4.19) |
Thus, vC−ε†≤vC+ε†≤ˆv. This indicates
w[vC−ε†,ˆv]≥w[vC−ε†,vC+ε†]. | (4.20) |
On the other hand, since vC−ε†∈S(B,c,d), we have
w[vC−εvC,vC+εvC]=w[ˇv,ˆv]≥w[vC−ε†,ˆv], | (4.21) |
by Proposition 9. Inequalities (4.20) and (4.21) imply that w[vC−εvC,vC+εvC]≥w[vC−ε†,vC+ε†].
As a result, by Definition 5 we know that [vC−εvC,vC+εvC] is a widest symmetrical interval solution regarding vC.
Proposition 10. Let u∈S(B,c,d) be an arbitrary solution in (1.6). Then it holds w[u,ˆv]≤w[ˇv,ˆv].
Proof. Let j be an arbitrary index in J. Now we examine the inequality ˆvj−ˇvj≥w[u,ˆv].
Case 1. If Iˆvj=∅, then ˇvj=0 by (4.4). Hence
w[u,ˆv]=⋀k∈J(ˆvk−uk)≤ˆvj−uj≤ˆvj−0=ˆvj−ˇvj. | (4.22) |
Case 2. If Iˆvj≠∅, then ˇvj=⋁i∈Iˆvjci by (4.4). Obviously, there exists i†∈Iˆvj, such that
ˇvj=ci†. | (4.23) |
At the same time, by (4.3) and i†∈Iˆvj we have
jˆvi†=j. | (4.24) |
Note that u∈S(B,c,d). Obviously, u satisfies the i†th inequality in (1.6), i.e.,
ci†≤(bi†1∧u1)∨(bi†2∧u2)∨⋯∨(bi†n∧un)≤di†. |
As a result, there is j†∈J satisfying
bi†j†∧uj†≥ci†. | (4.25) |
Considering u≤ˆv since ˆv is the maximum solution, we obtain bi†j†∧ˆvj†≥bi†j†∧uj†≥ci†. According to (4.1), it holds j†∈Jˆvi†. Moreover, Inequality (4.25) implies that
uj†≥bi†j†∧uj†≥ci†. | (4.26) |
Observing (4.2) and (4.24), we have j=jˆvi†=argmax{ˆvl|l∈Jˆvi†}. Thus,
ˆvj=ˆvjˆvi†≥ˆvl,∀l∈Jˆvi†. |
Since j†∈Jvi†, it holds
ˆvj≥ˆvj†. | (4.27) |
Combining (4.23), (4.26), and (4.27), we have
ˆvj−ˇvj=ˆvj−ci†≥ˆvj−uj†≥ˆvj†−uj†≥⋀k∈J(ˆvk−uk)=w[u,ˆv]. | (4.28) |
Inequalities (4.22) and (4.28) contribute to ˆvj−ˇvj≥w[u,ˆv], ∀j∈J. So we have
w[ˇv,ˆv]=⋀j∈J(ˆvj−ˇvj)≥w[u,ˆv]. |
Theorem 6. vC and εvC are defined by (4.16). Then it holds that vC is a centralized solution regarding system (1.6).
Proof. Let v∈S(B,c,d) be an arbitrary solution in (1.6). [v−εv,v+εv] is the WSIS regarding v. Since [v−εv,v+εv] is an interval solution of (1.6); by Definition 3, it holds
[v−εv,v+εv]⊆S(B,c,d). | (4.29) |
That is v−εv,v+εv∈S(B,c,d). According to Proposition 10,
w[ˇv,ˆv]≥w[v−εv,ˆv]. | (4.30) |
Since ˆv is the maximum solution, it is clear ˆv≥v+εv. Thus
w[v−εv,ˆv]≥w[v−εv,v+εv]. | (4.31) |
Inequalities (4.30) and (4.31) imply that
w[ˇv,ˆv]≥w[v−εv,v+εv]. | (4.32) |
By (4.16), it is clear ˇv=vC−εvC and ˆv=vC+εvC. Inequality (4.32) could be further written as
w[v−εv,v+εv]≤w[vC−εvC,vC+εvC]. | (4.33) |
According to Definition 6, vC is a centralized solution regarding system (1.6). Moreover, the WSIS regarding vC is [vC−εvC,vC+εvC]=[ˇv,ˆv].
This subsection provides an algorithm to find the centralized solution regarding a given max-min system. Moreover, some numerical examples are given for verifying our proposed algorithm.
Algorithm Ⅱ: solving the centralized solution regarding system (1.6)
Step 1. Following (4.1), calculate the indicator set Jˆvi for any i∈I.
Step 2. Following (4.2), select the indicator jˆvi from the indicator set Jˆvi for any i∈I.
Step 3. Following (4.3), construct the indicator set Iˆvj for any j∈J.
Step 4. Following (4.4), generate the vector ˇv=(ˇv1,ˇv2,⋯,ˇvn), where the ˇvj is defined by (4.4).
Step 5. Following (4.16), calculate the vectors vC and εvC. Then we find the centralized solution regarding system (1.6) as vC and the corresponding (biggest) symmetrical width as 2(εvC1∧εvC2∧⋯∧εvCn).
Computational complexity of Algorithm Ⅱ: In Algorithm Ⅱ, Step 1 requires 2mn operations for calculating the indicator sets. Moreover, Steps 2–4 have an identical calculation amount as mn. Besides, Step 5 requires 4n+2 operations. As a result, applying Algorithm Ⅱ to calculate the widest symmetrical interval solution regarding v, it requires totally
2mn+mn+mn+mn+4n=5mn+4n+2 |
operations. The computational complexity of Algorithm Ⅱ is also O(mn).
Example 3. Consider the max-min FRIs system as follows:
{0.3≤(0.2∧y1)∨(0.4∧y2)∨(0.5∧y3)∨(0.1∧y4)∨(0.6∧y5)∨(0.7∧y6)≤0.8,0.4≤(0.3∧y1)∨(0.6∧y2)∨(0.2∧y3)∨(0.8∧y4)∨(0.5∧y5)∨(0.1∧y6)≤0.9,0.1≤(0.7∧y1)∨(0.3∧y2)∨(0.4∧y3)∨(0.2∧y4)∨(0.5∧y5)∨(0.6∧y6)≤0.6,0.5≤(0.8∧y1)∨(0.1∧y2)∨(0.3∧y3)∨(0.4∧y4)∨(0.7∧y5)∨(0.2∧y6)≤0.9,0.2≤(0.5∧y1)∨(0.2∧y2)∨(0.1∧y3)∨(0.9∧y4)∨(0.6∧y5)∨(0.4∧y6)≤0.8. | (4.34) |
Try to find the centralized solution regarding the system (4.34).
Solution:
Notice that
ˆv=(0.6,1,1,0.8,1,1). |
is the maximum solution of system (4.34). Combining (4.1) and (4.2), we have
Jˆv1={j∈J|b1j∧ˆvj≥c1}={j∈J|b1j∧ˆvj≥0.3}={2,3,5,6},Jˆv2={j∈J|b2j∧ˆvj≥c2}={j∈J|b2j∧ˆvj≥0.4}={2,4,5},Jˆv3={j∈J|b3j∧ˆvj≥c3}={j∈J|b3j∧ˆvj≥0.1}={1,2,3,4,5,6},Jˆv4={j∈J|b4j∧ˆvj≥c4}={j∈J|b4j∧ˆvj≥0.5}={1,5},Jˆv5={j∈J|b5j∧ˆvj≥c5}={j∈J|b5j∧ˆvj≥0.2}={1,2,4,5,6}, |
and
jˆv1=argmax{ˆvj|j∈Jˆv1}=argmax{ˆv2,ˆv3,ˆv5,ˆv6}=2 or 3 or 5 or 6,jˆv2=argmax{ˆvj|j∈Jˆv2}=argmax{ˆv2,ˆv4,ˆv5}=2 or 5,jˆv3=argmax{ˆvj|j∈Jˆv3}=argmax{ˆv1,ˆv2,ˆv3,ˆv4,ˆv5,ˆv6}=2 or 3 or 5 or 6,jˆv4=argmax{ˆvj|j∈Jˆv4}=argmax{ˆv1,ˆv5}=5,jˆv5=argmax{ˆvj|j∈Jˆv5}=argmax{ˆv1,ˆv2,ˆv4,ˆv5,ˆv6}=2 or 5 or 6. |
Take jˆv1=2, jˆv2=5, jˆv3=3, jˆv4=5, jˆv5=6. Combining (4.3) and (4.4), we can obtain that
Iˆv1={i∈I|jˆvi=1}=∅,Iˆv2={i∈I|jˆvi=2}={1},Iˆv3={i∈I|jˆvi=3}={3},Iˆv4={i∈I|jˆvi=4}=∅,Iˆv5={i∈I|jˆvi=5}={2,4},Iˆv6={i∈I|jˆvi=6}={5}, |
and
ˇv1=0,ˇv2=⋁i∈Iˆv2ci=c1=0.3,ˇv3=⋁i∈Iˆv3ci=c3=0.1, |
ˇv4=0,ˇv5=⋁i∈Iˆv5ci=c2∨c4=0.5,ˇv6=⋁i∈Iˆv6ci=c5=0.2. |
Then we find the vector ˇv=(0,0.3,0.1,0,0.5,0.2). In accordance with (4.16), we further have
vC=ˆv+ˇv2=(0.3,0.65,0.55,0.4,0.75,0.6),εvC=ˆv−ˇv2=(0.3,0.35,0.45,0.4,0.25,0.4). |
Then it holds that vC=(0.3,0.65,0.55,0.4,0.75,0.6) is a centralized solution regarding system (4.34). The corresponding widest symmetrical interval solution regarding vC is
[vC−εvC,vC+εvC]=([0,0.6],[0.3,1],[0.1,1],[0,0.8],[0.5,1],[0.2,1]), | (4.35) |
with the (biggest) symmetrical width w[vC−εvC,vC+εvC]=0.5.
Remark 3. In fact, the centralized solution regarding a given system with max-min FRIs might not be unique. For example, it can be easily examined that
(0.35,0.75,0.75,0.55,0.75,0.75) |
is indeed another centralized solution regarding system (4.34).
Example 4. Consider the max-min FRIs system as follows:
{0.37≤(0.35∧y1)∨(0.48∧y2)∨(0.87∧y3)∨(0.63∧y4)∨(0.26∧y5)∨(0.74∧y6)≤0.82,0.34≤(0.28∧y1)∨(0.92∧y2)∨(0.76∧y3)∨(0.31∧y4)∨(0.53∧y5)∨(0.19∧y6)≤0.87,0.25≤(0.89∧y1)∨(0.76∧y2)∨(0.42∧y3)∨(0.18∧y4)∨(0.56∧y5)∨(0.75∧y6)≤0.79,0.36≤(0.33∧y1)∨(0.85∧y2)∨(0.27∧y3)∨(0.35∧y4)∨(0.93∧y5)∨(0.35∧y6)≤0.85,0.41≤(0.59∧y1)∨(0.28∧y2)∨(0.18∧y3)∨(0.38∧y4)∨(0.83∧y5)∨(0.91∧y6)≤0.89,0.32≤(0.48∧y1)∨(0.23∧y2)∨(0.31∧y3)∨(0.26∧y4)∨(0.56∧y5)∨(0.28∧y6)≤0.86. | (4.36) |
Try to find the centralized solution regarding system (4.36).
Solution: After calculation, the maximum solution of system (3.26) can be obtained as ˆv=(0.79,0.87,0.82,1,0.85,0.89). Next, we apply our proposed Algorithm Ⅱ to find the centralized solution regarding (4.36).
Step 1. Following (4.1), we have
Jˆv1={2,3,4,6},Jˆv2={2,3,5},Jˆv3={1,2,3,5,6},Jˆv4={2,5},Jˆv5={1,5,6},Jˆv6={1,5}. |
Step 2. Following (4.2), we have
jˆv1=argmax{vj|j∈Jˆv1}=4,jˆv2=argmax{vj|j∈Jˆv2}=2,jˆv3=argmax{vj|j∈Jˆv3}=6,jˆv4=argmax{vj|j∈Jˆv4}=2,jˆv5=argmax{vj|j∈Jˆv5}=6,jˆv6=argmax{vj|j∈Jˆv6}=5. |
Step 3. Following (4.3), we have
Iˆv1={i∈I|jˆvi=1}=∅,Iˆv2={i∈I|jˆvi=2}={2,4},Iˆv3={i∈I|jˆvi=3}=∅,Iˆv4={i∈I|jˆvi=4}={1},Iˆv5={i∈I|jˆvi=5}={6},Iˆv6={i∈I|jˆvi=6}={3,5}. |
Step 4. Following (4.4), we have
ˇv1=0,ˇv2=⋁i∈Iˇv2ci=c2∨c4=0.36,ˇv3=0,ˇv4=⋁i∈Iˇv4ci=c1=0.37,ˇv5=⋁i∈Iˇv5ci=c6=0.32,ˇv6=⋁i∈Iˇv6ci=c3∨c5=0.41. |
Hence, we obtain the vector ˇv=(0,0.36,0,0.37,0.32,0.41).
Step 5. Following (4.16), we can calculate the vectors vC and εvC. After calculation, we have
vC=ˆv+ˇv2=(0.395,0.615,0.41,0.685,0.585,0.65). |
εvC=ˆv−ˇv2=(0.395,0.255,0.41,0.315,0.265,0.24). |
Hence, the centralized solution regarding system (4.36) is vC=(0.395,0.615,0.41,0.685,0.585,0.65), with the corresponding (biggest) symmetrical width 2(εvC1∧εvC2∧⋯∧εvC6)=0.48.
Recently, some researchers adopted the max-min FRIs or FREs system to model a P2P educational information sharing system. Any feasible scheme in the P2P network system was found to be a solution to the max-min system, e.g., system (1.6). As a result, we employed the concept of the widest symmetrical interval solution to represent the stability for a feasible scheme. Moreover, reflecting the most stable feasible scheme, the concept of a centralized solution of system (1.6) was further defined. Effective resolution methods were proposed for the widest symmetrical interval solution regarding a provided solution and the centralized solution regarding system (1.6), respectively. The proposed resolution methods have been demonstrated and examined through the numerical examples. In future works, one might further generalize the widest symmetrical interval solution and centralized solution to the addition-min FRIs system, or even to the classical max-t-norm fuzzy relational system.
Miaoxia Chen: Validation, Investigation, Writing-original draft; Guocheng Zhu: Validation, Methodology; Shayla Islam: Conceptualization, Supervision; Xiaopeng Yang: Conceptualization, Supervision, Funding acquisition, Writing-review & editing. All authors have read and agreed to the published version of the manuscript.
The authors declare they have not used Artificial Intelligence (AI) tools in the creation of this article.
We would like to express our appreciation to the editor and the anonymous reviewers for their valuable comments, which have been very helpful in improving the paper. This work was supported by the National Natural Science Foundation of China (12271132), the Guangdong Basic and Applied Basic Research Foundation (2024A1515010532, 2024ZDZX1027) and the NSF of Hanshan Normal University (XYN202303, 2023KQNCX041, XSPY202405). This work was supported by the Guangdong Province Quality Project (Data Science Innovation and Entrepreneurship Laboratory).
The authors declare that they have no conflicts of interest.
[1] | E. Sanchez, Resolution of composite fuzzy relation equations, Inf. Control, 30 (1976), 38–48. |
[2] | W. Pedrycz, Granular Computing: Analysis and Design of Intelligent Systems, Boca Raton: CRC Press, 2018. |
[3] | A. Di Nola, W. Pedrycz, S. Sessa, P. Z. Wang, Fuzzy relation equation under a class of triangular norms: A survey and new results, In: Readings in Fuzzy Sets for Intelligent Systems, Amsterdam: Elsevier, 1993,166–189. https://doi.org/10.1016/B978-1-4832-1450-4.50018-3 |
[4] |
M. Miyakoshi, M. Shimbo, Solutions of composite fuzzy relational equations with triangular norms, Fuzzy Sets Syst., 16 (1985), 53–63. https://doi.org/10.1016/S0165-0114(85)80005-1 doi: 10.1016/S0165-0114(85)80005-1
![]() |
[5] | W. Pedrycz, Fuzzy relational equations with triangular norms and their resolutions, BUSEFAL, 11 (1982), 24–32. |
[6] |
W. Pedrycz, On generalized fuzzy relational equations and their applications, J. Math. Anal. Appl., 107 (1985), 520–536. https://doi.org/10.1016/0022-247X(85)90329-4 doi: 10.1016/0022-247X(85)90329-4
![]() |
[7] | B. De Baets, Analytical Solution Methods for Fuzzy Relational Equations, : Fundamentals of Fuzzy Sets, Boston: Springer, 2000,291–340. https://doi.org/10.1007/978-1-4615-4429-6_7 |
[8] | A. Di Nola, E. Sanchez, W. Pedrycz, S. Sessa, Fuzzy relation equations and their applications to knowledge engineering, Dordrecht: Springer, 1989. https://doi.org/10.1007/978-94-017-1650-5 |
[9] | P. K. Li, S. C. Fang, On the resolution and optimization of a system of fuzzy relational equations with sup-t composition, Fuzzy Opt. Decis. Making, 7 (2008), 169–214. |
[10] |
B.-S. Shieh, Solutions of fuzzy relation equations based on continuous t-norms, Inf. Sci., 177 (2007), 4208–4215. https://doi.org/10.1016/j.ins.2007.04.006 doi: 10.1016/j.ins.2007.04.006
![]() |
[11] |
S. Wang, H. Li, Column stacking approach to resolution of systems of fuzzy relational inequalities, J. Franklin Inst., 356 (2019), 3314–3332. https://doi.org/10.1016/j.jfranklin.2019.02.007 doi: 10.1016/j.jfranklin.2019.02.007
![]() |
[12] |
J. Drewniak, Fuzzy relation equations and inequalities, Fuzzy Sets Syst., 14 (1984), 237–247. https://doi.org/10.1016/0165-0114(84)90084-8 doi: 10.1016/0165-0114(84)90084-8
![]() |
[13] |
P. Z. Wang, D. Z. Zhang, E. Sanchez, E. S. Lee, Latticized linear programming and fuzzy relation inequalities, J. Math. Anal. Appl., 159 (1991), 72–87. https://doi.org/10.1016/0022-247X(91)90222-L doi: 10.1016/0022-247X(91)90222-L
![]() |
[14] |
F. F. Guo, L. P. Pang, D. Meng, Z. Q. Xia, An algorithm for solving optimization problems with fuzzy relational inequality constraints, Inf. Sci., 252 (2013), 20–31. https://doi.org/10.1016/j.ins.2011.09.030 doi: 10.1016/j.ins.2011.09.030
![]() |
[15] | J. X. Li, S. J. Yang, Fuzzy relation equalities about the data transmission mechanism in bittorrent-like peer-to-peer file sharing systems, Proceedings of the 2012 9th International Conference on Fuzzy Systems and Knowledge Discovery, 2012. Available from: https://doi.org/10.1109/FSKD.2012.6233956 |
[16] |
X. P. Yang, H. T. Lin, X. G. Zhou, B. Y. Cao, Addition-min fuzzy relation inequalities with application in BitTorrent-like Peer-to-Peer file sharing system, Fuzzy Sets Syst., 343 (2018), 126–140. https://doi.org/10.1016/j.fss.2017.04.002 doi: 10.1016/j.fss.2017.04.002
![]() |
[17] | M. Li, X. Wang, Minimal solutions of fuzzy relation inequalities with addition-min composition and their applications, IEEE Trans. Fuzzy Syst., 31 (2023), 1665–1675. https://ieeexplore.ieee.org/document/9917301/ |
[18] |
X. P. Yang, X. G. Zhou, B. Y. Cao, Min-max programming problem subject to addition-min fuzzy relation inequalities, IEEE Trans. Fuzzy Syst., 24 (2016), 111–119. https://doi.org/10.1109/TFUZZ.2015.2428716 doi: 10.1109/TFUZZ.2015.2428716
![]() |
[19] |
Y. K. Wu, S. M. Guu, Solving minimal-optimal solutions for the generalized min-max programming problem with addition-min composition, Fuzzy Sets Syst., 477 (2024), 108825. https://doi.org/10.1016/j.fss.2023.108825 doi: 10.1016/j.fss.2023.108825
![]() |
[20] |
X. Yang, Leximax minimum solution of addition-min fuzzy relation inequalities, Inf. Sci., 524 (2020), 184–198. https://doi.org/10.1016/j.ins.2020.03.047 doi: 10.1016/j.ins.2020.03.047
![]() |
[21] |
X. Yang, Random-term-absent addition-min fuzzy relation inequalities and their lexicographic minimum solutions, Fuzzy Sets Syst., 440 (2022), 42–61. https://doi.org/10.1016/j.fss.2021.08.007 doi: 10.1016/j.fss.2021.08.007
![]() |
[22] |
J. Qiu, X. Yang, Min-max programming problem with constraints of addition-min-product fuzzy relation inequalities, Fuzzy Opt. Decis. Making, 21 (2022), 291–317. https://doi.org/10.1007/s10700-021-09368-7 doi: 10.1007/s10700-021-09368-7
![]() |
[23] |
Y. K. Wu, S. M. Guu, F. H. Yang, K. M. Chang, On min-max optimization for systems with addition-min-product fuzzy relational inequalities, Int. J. Fuzzy Syst., 24 (2022), 2631–2642. https://doi.org/10.1007/s40815-022-01316-w doi: 10.1007/s40815-022-01316-w
![]() |
[24] | Y. Zhong, G. Xiao, X. Yang, Fuzzy relation lexicographic programming for modelling P2P file sharing system, Soft Comput., 23 (2019), 3605–3614. |
[25] | G. Xiao, K. Hayat, X. Yang, Evaluation and its derived classification in a Server-to-Client architecture based on the fuzzy relation inequality, Fuzzy Opt. Decis. Making, 22 (2023), 213–245. |
[26] |
X. Yang, Evaluation model and approximate solution to inconsistent max-min fuzzy relation inequalities in P2P file sharing system, Complexity, 2019 (2019), 6901818. https://doi.org/10.1155/2019/6901818 doi: 10.1155/2019/6901818
![]() |
[27] |
X. B Yang, X. P Yang, K. Hayat, A new characterisation of the minimal solution set to max-min fuzzy relation inequalities, Fuzzy Inf. Eng., 9 (2017), 423–435. https://doi.org/10.1016/j.fiae.2017.12.002 doi: 10.1016/j.fiae.2017.12.002
![]() |
[28] |
G. Xiao, T. Zhu, Y. Chen, X. Yang, Linear searching method for solving approximate solution to system of max-min fuzzy relation equations with application in the instructional information resources allocation, IEEE Access, 7 (2019), 65019–65028. https://doi.org/10.1109/ACCESS.2019.2912217 doi: 10.1109/ACCESS.2019.2912217
![]() |
[29] |
C. Wen, Y. Wu, Z. Li, Algebraic formulae for solving systems of max-min inverse fuzzy relational equations, Inf. Sci., 622 (2023), 1162–1183. https://doi.org/10.1016/j.ins.2022.11.123 doi: 10.1016/j.ins.2022.11.123
![]() |
[30] | X. P. Yang, Linear programming method for solving semi-latticized fuzzy relation geometric programming with max-min composition, Int. J. Uncertainty, Fuzziness Knowl.-Based Syst., 23 (2015), 781–804. |
[31] |
X. G. Zhou, X. P. Yang, B. Y. Cao, Posynomial geometric programming problem subject to max-min fuzzy relation equations, Inf. Sci., 328 (2016), 15–25. https://doi.org/10.1016/j.ins.2015.07.058 doi: 10.1016/j.ins.2015.07.058
![]() |
[32] | X. Yang, X. Zhou, B. Cao, Y. Hong, Variable substitution method for solving single-variable term fuzzy relation geometric programming problem and its application, Int. J. Uncertainty, Fuzziness Knowl.-Based Syst., 27 (2019), 537–557. |
[33] |
Y. B. Ma, X. B. Yang, B. Y. Cao, Fuzzy-Relation-Based lexicographic minimum solution to the P2P network system, IEEE Access, 8 (2020), 195447–195458. https://doi.org/10.1109/ACCESS.2020.3034279 doi: 10.1109/ACCESS.2020.3034279
![]() |
[34] |
Y. Chen, X. Liu, L. Zhang, Interval solution to fuzzy relation inequality with application in P2P educational information resource sharing systems, IEEE Access, 9 (2021), 96166–96175. https://doi.org/10.1109/ACCESS.2021.3092745 doi: 10.1109/ACCESS.2021.3092745
![]() |
[35] |
L. Zhang, Max-min fuzzy bi-level programming: resource sharing system with application, Appl. Math. Sci. Eng., 32 (2024), 2335319. https://doi.org/10.1080/27690911.2024.2335319 doi: 10.1080/27690911.2024.2335319
![]() |
[36] |
L. Zhang, Optimal symmetric interval solution of fuzzy relation inequality considering the stability in P2P educational information resources sharing system, Fuzzy Sets Syst., 478 (2024), 108835. https://doi.org/10.1016/j.fss.2023.108835 doi: 10.1016/j.fss.2023.108835
![]() |