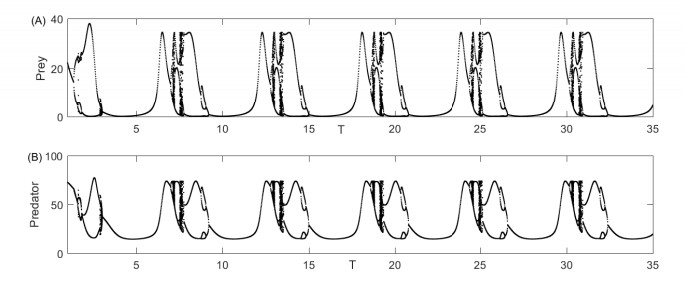
A good pulse control strategy should depend on the numbers of pests and natural enemies as determined via an integrated pest control strategy. Taking this into consideration, here, a nonlinear impulsive predator-prey model with improved Leslie-Gower and Beddington-DeAngelis functional response terms is qualitatively analyzed. The existence of a periodic solution for pest eradication has been obtained and the critical condition of global asymptotic stability has been established by using the impulsive differential equation Floquet theory. Furthermore, the conditions for the lasting survival of the system has been proved by applying a comparison theorem for differential equations. Additionally, a stable positive periodic solution has been obtained by applying bifurcation theory. To understand how nonlinear pulses affect the dynamic behavior of a system, MATLAB was used to conduct numerical simulations to show that the model has very complex dynamical behavior.
Citation: Changtong Li, Dandan Cheng, Xiaozhou Feng, Mengyan Liu. Complex dynamics of a nonlinear impulsive control predator-prey model with Leslie-Gower and B-D functional response[J]. AIMS Mathematics, 2024, 9(6): 14454-14472. doi: 10.3934/math.2024702
[1] | Heping Jiang . Complex dynamics induced by harvesting rate and delay in a diffusive Leslie-Gower predator-prey model. AIMS Mathematics, 2023, 8(9): 20718-20730. doi: 10.3934/math.20231056 |
[2] | Fatao Wang, Ruizhi Yang, Yining Xie, Jing Zhao . Hopf bifurcation in a delayed reaction diffusion predator-prey model with weak Allee effect on prey and fear effect on predator. AIMS Mathematics, 2023, 8(8): 17719-17743. doi: 10.3934/math.2023905 |
[3] | Lin Wu, Jianjun Jiao, Xiangjun Dai, Zeli Zhou . Dynamical analysis of an ecological aquaculture management model with stage-structure and nonlinear impulsive releases for larval predators. AIMS Mathematics, 2024, 9(10): 29053-29075. doi: 10.3934/math.20241410 |
[4] | Fethi Souna, Salih Djilali, Sultan Alyobi, Anwar Zeb, Nadia Gul, Suliman Alsaeed, Kottakkaran Sooppy Nisar . Spatiotemporal dynamics of a diffusive predator-prey system incorporating social behavior. AIMS Mathematics, 2023, 8(7): 15723-15748. doi: 10.3934/math.2023803 |
[5] | Jin Liao, André Zegeling, Wentao Huang . The uniqueness of limit cycles in a predator-prey system with Ivlev-type group defense. AIMS Mathematics, 2024, 9(12): 33610-33631. doi: 10.3934/math.20241604 |
[6] | Xiongxiong Du, Xiaoling Han, Ceyu Lei . Dynamics of a nonlinear discrete predator-prey system with fear effect. AIMS Mathematics, 2023, 8(10): 23953-23973. doi: 10.3934/math.20231221 |
[7] | Yao Shi, Zhenyu Wang . Bifurcation analysis and chaos control of a discrete fractional-order Leslie-Gower model with fear factor. AIMS Mathematics, 2024, 9(11): 30298-30319. doi: 10.3934/math.20241462 |
[8] | A. Q. Khan, Ibraheem M. Alsulami . Discrete Leslie's model with bifurcations and control. AIMS Mathematics, 2023, 8(10): 22483-22506. doi: 10.3934/math.20231146 |
[9] | Ruyue Hu, Chi Han, Yifan Wu, Xiaohui Ai . Analysis of a stochastic Leslie-Gower three-species food chain system with Holling-II functional response and Ornstein-Uhlenbeck process. AIMS Mathematics, 2024, 9(7): 18910-18928. doi: 10.3934/math.2024920 |
[10] | Na Min, Hongyang Zhang, Xiaobin Gao, Pengyu Zeng . Impacts of hunting cooperation and prey harvesting in a Leslie-Gower prey-predator system with strong Allee effect. AIMS Mathematics, 2024, 9(12): 34618-34646. doi: 10.3934/math.20241649 |
A good pulse control strategy should depend on the numbers of pests and natural enemies as determined via an integrated pest control strategy. Taking this into consideration, here, a nonlinear impulsive predator-prey model with improved Leslie-Gower and Beddington-DeAngelis functional response terms is qualitatively analyzed. The existence of a periodic solution for pest eradication has been obtained and the critical condition of global asymptotic stability has been established by using the impulsive differential equation Floquet theory. Furthermore, the conditions for the lasting survival of the system has been proved by applying a comparison theorem for differential equations. Additionally, a stable positive periodic solution has been obtained by applying bifurcation theory. To understand how nonlinear pulses affect the dynamic behavior of a system, MATLAB was used to conduct numerical simulations to show that the model has very complex dynamical behavior.
In recent years, predator-prey systems with integrated pest control have been extensively studied to realize the effective control of pests [1,2,3,4]. Generally, IPM (Integrated Pest Management) mainly uses chemical control, biological control and artificial control to establish an efficient comprehensive system to control pests. Among them, biological control is mainly the use of natural enemies, which involves their harvesting and capture pests, while chemical control is the use of pesticides and other chemicals to quickly kill pests in large quantities. Regarding implementing pest control, the combination of two strategies can yield an optimal control strategy [5,6].
The functional response is defined as the number of prey killed by a predator per unit of time, and it describes the amount of biological transfer between different trophic levels, i.e., the effects of the predator population on the prey. Many scholars have considered predator-prey models with different functional responses from many aspects. For example, the nonlinear functional response first appeared in the Lotka-Volterra [7] predation system. Also, Wang et al. [8] and Cheng et al. [9] considered Holling-Ⅰ functional responses in a predator-prey model; Luo et al. [10] and Liu et al. [11] focused on Holling type Ⅱ functional responses. Other scholars [12,13] studied the Holling-Ⅲ functional response; through their research, it was found that functional response terms not only rely on prey behavior, but they are also related to predator activity. Wang [14] researched the predator-prey model with ratio-dependent functional response terms, and by applying a ratio-dependent functional response, it was found that the predator density could not be zero, i.e., the predator and prey could not be extinct at the same time. Thus, the Beddington-DeAngelis functional response term [15,16] has garnered much attention.
To facilitate research, many scholars have set the periodic release of natural enemies in the pest-natural enemy system as an invariant constant [17]; in fact, pest outbreaks are generally transient, uncertain and unpredictable, so pest control strategies incorporate pulse disturbances to simulate the effects of the sudden change of actual factors on the state of the system. Moreover, many anthropogenic phenomena in nature can be described by impulsive differential equations, and these phenomena include fishing and stocking in fish farming, spraying pesticides in agriculture the regular release of natural enemies, insulin injection in diabetes, etc. In [18,19], the authors considered a predator-prey model with linear pulses, employing modified versions of the Leslie-Gower and Holling-Ⅱ functional responses, but these impulse effects tend to be nonlinear. However, the excessive use of pesticides or biological predators can potentially yield significant damage to the environment and economy. Nonetheless, optimal impulse control management requires that the IPM rely on the densities of both pests and natural enemies in the field; therefore, predator-prey models with nonlinear impulse control are more practical and reasonable. To investigate how nonlinear impulse control affects the dynamic behavior of a system, in [20], Li et al. studied a predator-prey model with nonlinear pulses and a Beddington-DeAngelis functional response, finding that the models with nonlinear impulse control exhibit richer dynamic behavior than those with linear pulses. The Leslie-Gower functional response is an important functional response, which means that the predator population does not unlimitedly grow because of the limitations of natural resources, and many predators do not live on just one kind of food. The authors of [21,22] studied the dynamic behavior of predatory models with Leslie-Gower and Holling-Ⅱ terms. However, these studies applied the assumption that the impulse control is linear or constant. Because the outbreak of pests and diseases occurs rapidly, it is difficult for many countries or regions, which have limited capacity for agricultural resources such as pesticides, labor, and biological resources, to effectively control an outbreak of pests. In order to take the resource limitation into account, the instant killing rate should be a monotonically increasing saturation function, and the natural enemies that are released should be based on the current pest and prey densities in the field. With the goal of realizing optimal pulse management, we studied a nonlinear impulsive control predator-prey model with a Leslie-Gower term and Beddington-DeAngelis term, which more comprehensively consider the effects of human control and environmental factors on population density:
{dxdt=(r1−bx(t)−αy(t)a1+a2x(t)+a3y(t))x(t),t≠nT,dydt=(r2−βy(t)x(t)+k)y(t),t≠nT,x(t+)=(1−δx(t)x(t)+h)x(t),t=nT,y(t+)=qy(t)+w1x(t)1+θx(t)+w2,t=nT, | (1.1) |
where x(t) and y(t) are the populations of pests and natural enemies at time t, respectively α,β,a1,a2,a3, k,h,q, θ, δ, w1,w2 are model parameters that assume only positive values, r1,r2 represent the birth rates of pests and natural enemies, b describes the intraspecific competition for pests, δ measures the maximum rate at which pests are killed by spraying insecticides (0<δ≤1), h is the semi-saturation constant, w1,w2 denote the maximum releases of predators based on prey density and predator population density, and αx(t)y(t)a1+a2x(t)+a3y(t) is the B-D functional response term; IPM strategies are adopted at each discrete time point.
First, we give some notations, definitions and lemmas which will be useful for our main results.
Assume that
R+=[0,+∞),R2+={X=(x(t),y(t))|x(t),y(t)≥0}, |
V0={V:R+×R2+→R+} |
are continuous on (nT,(n+1)T] and f=(f1,f2)T is the right-hand mapping of the first two equations of the system (1.1); obviously, the smoothness of f guarantees the existence of a unique solution. The following lemma [23,24] is given.
Lemma 2.1. Let X(t)=(x(t),y(t)) be a solution of system (1.1), and if the initial value X(0+)≥0, then X(t)≥0 for all t≥0; furthermore, if X(0+)>0, then X(t)>0 for all t≥0.
Proof. Obviously for y(t), through scaling we have the following:
{dy(t)dt=(r2−βy(t)x(t)+k)y(t),t≠nT,y(t+)≥qy(t)+w2,t=nT. | (2.1) |
It is easy to see that
y(t)≥y(0+)exp∫t0(r2−βy(s)x(s)+k)ds |
for t∈(0,T].
At time t=T, y(T+)=qy(T)+w2>qy(T)>0; thus, when t∈(T,2T], the initial value becomes y(T+)≥0. For x(t), through scaling we get the following:
{dx(t)dt=(r1−bx(t)−αy(t)a1+a2x(t)+a3y(t))x(t),t≠nT,x(t+)>(1−δ)x(t),t=nT. | (2.2) |
Thus, we have
x(t)≥x(0+)exp∫t0(r1−bx(s)−αy(s)a1+a2x(s)+a3y(s))ds, |
for t∈(0,T].
At time t=T, x(T+)>(1−δ)x(T)>0; thus, when t∈(T,2T], the initial value becomes x(T+)≥0.
Similar to the previous proof we can determine when X(0+)≥0 and X(t)≥0 for all t≥0. Similarly, when X(0+)>0 we can get that X(t)>0 for all t>0.
Lemma 2.2. Define g:R+×R+→R as continuous on (nT,(n+1)T] when n∈Z+,u∈R+, and
lim(t,y)→(nT+,u)g(t,y)=g(nT+,u), |
ψn:R+→R+ is a non-decreasing mapping; suppose that the following inequalities hold:
{D+V(t,x)≤g(t,V(t,x)),t≠nT,V(t,x)≤ψn(V(t,x)),t=nT, | (2.3) |
and that the impulsive differential equations given by
{du(t)dt=g(t,u(t)),t≠nT,u(t+)=ψn(u(t)),t=nT,u(0+)=u0>0, | (2.4) |
have a maximum solution r(t)∈[0,+∞); when t≥0 and V(0+,x0)≤u0, we have that V(t,x)≤r(t).
The elimination of pest populations is an important goal of IPM strategies; therefore, studying the existence and global stability of periodic solutions plays a crucial role in the investigation of their dynamic behavior. Thus, we consider the subsystem of (1.1):
{dy(t)dt=(r2−βky(t))y(t),t≠nT,y(t+)=qy(t)+w2,t=nT. | (2.5) |
Now, we shall give the basic properties of the subsystem:
Theorem 2.3. If 1−qexp(r2T)<0, system (2.5) has a globally asymptotically stable periodic solution
y∗(t)=r2y∗er2(t−nT)r2−βky∗(1−er2(t−nT)),t∈(nT,(n+1)T], | (2.6) |
where y∗=A+√A2+B with A=w22+r2(1−qer2T)2βk(1−er2T) and B=r2w2−βk(1−er2T) as two positive constants.
Proof. By applying the idea of variable separation and difference equations to solve the system (2.5), we have
y((n+1)T)=r2y(nT+)er2Tr2−βky(nT+)(1−er2T), | (2.7) |
and y((n+1)T+)=qy((n+1)T)+w2; then, the following equation is obtained
y((n+1)T+)=qy((n+1)T)+w2=qr2y(nT+)er2Tr2−βky(nT+)(1−er2T)+w2. | (2.8) |
Let y(nT+)=y((n+1)T+)=y∗. Substituting y∗ into the above equation yields the following quadratic equation:
y∗2+(−w2+r2(1−qer2T)−βk(1−er2T))y∗+r2w2βk(1−er2T)=0. | (2.9) |
We have that y∗=A+√A2+B, where
A=w22+r2(1−qer2T)2βk(1−er2T),B=r2w2−βk(1−er2T). |
Apparently when 1−qer2T<0, system (2.5) has a periodic solution y∗(t) for the case with pests.
According to the above analysis, we can easily get the expression for the unique pest-free periodic solution of system (1.1), i.e., (0,y∗(t)). In what follows, we will give the sufficient condition for the global stability of the pest-free periodic solution (0,y∗(t)) of system (1.1).
Theorem 2.4. The periodic solution (0,y∗(t)) of the system (1.1) is locally asymptotically stable for the case with pests if
T=Tmax<αr1(a1βk+a3r2)lna1r2−βka1y∗+(a1βk+a3r2)er2Ty∗a1r2+a3r2y∗. | (2.10) |
Proof. Here we first demonstrate the local stability of the periodic solution for pests.
The local stability of the periodic solution for pests may be determined by considering the behavior of small-amplitude perturbations (u(t),v(t)) of the solution. Define u(t)=x(t),v(t)=y(t)−y∗(t), where u(t),v(t) are small perturbations. After neglecting the higher-order terms, it can be written as
(u(t)v(t))=φ(t)(u(0)v(0)),0≤t<T, | (2.11) |
where φ(t) satisfies
dφ(t)dt=(r1−αy∗(t)a1+a3y∗(t)0∗r2−2βky∗(t))⋅φ(t), | (2.12) |
and φ(0)=I is the identity matrix. Hence, the fundamental solution matrix is given by
φ(t)=(e∫T0r1−αa1+a3y∗(t)⋅y∗(t)dt0∗e∫T0r2−2βky∗(t)dt). | (2.13) |
Then, linearizing the third and fourth equation of system (1.1), we have
(u(nT+)u(nT+))=(10λ1q)(u(nT)v(nT))=A(T)(u(nT)v(nT)). | (2.14) |
Let
M=A(T)φ(T)=(e∫T0r1−αa1+a3y∗(t)⋅y∗(t)dt0∗qe∫T0r2−2βky∗(t)dt). | (2.15) |
If each eigenvalue of M has an absolute value that is less than one, the solution is locally stable; note that (*) is not important, so it is not considered. Below we define
λ1=exp(∫T0r1−αa1+a3y∗(t)⋅y∗(t)dt),λ2=qexp(∫T0r2−2βky∗(t)dt), | (2.16) |
as two eigenvalues of the matrix M because
kr2β−y∗(1+er2(t−nT))<kr2β−y∗=kr2β−(w22+kr2(1−qer2T)2β(1−er2T)+√(w22+kr2(1−qer2T)2β(1−er2T))2+kr2w2β(er2T−1))<kr2β−w22−kr22β−kr22β=−w22<0,r2−2βky∗(t)=r2kr2β−y∗(1+er2(t−nT))kr2β−y∗(1−er2(t−nT))<0. | (2.17) |
It is easy to see that λ2<1, according to the Floquet theory for impulsive differential equations, (0,y∗(t)) is locally stable if
T<αa3r2+βka1lna1r2−βka1+(a2r2+βka1)er2Ty∗a1r2+a3r2y∗. | (2.18) |
Next, we will prove the global attraction of the periodic solution for pests. Just prove that the arbitrary solution (x(t),y(t)) tends to (0,y∗(t)) when t→∞.
(1) Prove that x(t)→0.
Since x=r1b, it follows that dxdt<0; then 0<x<r1b. Take a small enough ε>0 so that
ηΔ=exp(∫T0r1−α(y∗(t)−ε)a1+a2(k+ε)+a3(y∗(t)−ε))dt<1. | (2.19) |
It follows from the system (1.1) that
dx(t)dt=(r1−bx(t)−αy(t)a1+a2x(t)+a3y(t))x(t)≤(r1−αy(t)a1+a2x(t)+a3y(t))x(t)≤(r1−α(y∗(t)−ε)a1+a2(k+ε)+a3(y∗(t)−ε))x(t), | (2.20) |
further, we consider the following impulsive differential equation:
{dx(t)dt≤(r1−α(y∗(t)−ε)a1+a2(k+ε)+a3(y∗(t)−ε))x(t),t≠nT,x(t+)=(1−δx(t)x(t)+h)x(t),t=nT. | (2.21) |
By applying the impulsive differential equation theorem and the comparison theorem, within the interval ((nT),(n+1)T), we get
x((n+1)T)≤x(nT)exp(∫T0r1−α(y∗(t)−ε)a1+a2(k+ε)+a3(y∗(t)−ε)dt)≤x(nT)η. | (2.22) |
Then, x(nT)≤x(0+)ηn and x(nT)→0 as n→∞. Therefore x(t)→0 as t→0.
(2) Prove that y(t)→y∗(t).
From above x(t)→0 as t→∞ for any ε1>0. Additionally, there must exist a T1>0, such that 0<x(t)<ε1 and t>T1. Without loss of generality, we may assume that 0<x(t)<ε1 for all t>0; from system (2.5) we have
y(t)(r2−βy(t)k)<dy(t)dt=(r2−βy(t)x(t)+k)y(t)<y(t)(r2−βy(t)ε1+k). | (2.23) |
Then we consider the following equations
{dz1(t)dt=(r2−βkz1(t))z1(t),t≠nT,z1(t+)=qz1(t)w2,t=nT, | (2.24) |
and
{dz2(t)dt=(r2−βε1+kz2(t))z2(t),t≠nT,z2(t+)=qz2(t)w2,t=nT, | (2.25) |
where
z∗1(t)=y∗(t)=r2z∗1(1−er2(t−nT))r2−βkz∗1(1−er2(t−nT)),z1∗=A1+√A12+B1, |
A1=w22+kr2(1−qer2T)2β(1−er2T),B1=−kr2w2β(1−er2T), |
and
z∗2(t)=r2z∗2er2(t−nT))r2−βε1+kz∗2(1−er2(t−nT)),z2∗=A2+√A22+B2, |
A2=w22+(k+ε1)r2(1−qer2T)2β(1−er2T),B2=−(k+ε1)r2w2β(1−er2T). |
For arbitrarily small ε2>0, according to the comparison theorem we have
z1∗(t)−ε2<y(t)<z2∗(t)+ε2. |
Let ε1→0 as t→∞; we have that y∗(t)−ε2<y(t)<y∗(t)+ε2; hence, y(t)→y∗(t) for t large enough. Thus the proof is completed.
In order to understand the long-term survival of the prey and predator populations, it is important to investigate the permanence of system (1.1). First, we give the definition of permanence as follows:
Definition 3.1. The system (1.1) is permanent if there exists M≥m>0 for any solution (x(t),y(t)) of (1.1) with x0>0 such that
m≤limt→∞infx(t)≤limt→∞supx(t)≤M,m≤limt→∞infy(t)≤limt→∞supy(t)≤M. | (3.1) |
Theorem 3.2. System (1.1) is permanent if
T>αr1(a1βk+a3r2)lna1r2−βka1y∗+(a1βk+a3r2)er2Ty∗a1r2+a3r2y∗. | (3.2) |
Proof. Suppose that (x(t),y(t)) is any solution of system (1.1). Apparently, given the above analysis, we have that x<M1<r1b for t large enough. For convenience, let x(t)≤M for any t>0. Then, from Theorem 2.4, we can easily obtain that y∗(t)−ε2<y(t)<y∗(t)+ε2 for t large enough. Let m2=y∗(t)−ε2 and M2=y∗(t)+ε2; then we have that m2≤y(t)≤M2.
Assume that y(t)≥m2 for any t>0. We need to prove that there exists a positive constant m1 such that x(t)≥m1 when t is large enough. We will prove it in two steps:
1) Take a positive number m3>0 and ε3>0 arbitrarily small enough. Let
η2=exp((r−bm3−αε3a1)T−αa1k+a3βlnr2−βk+m3z∗3(1−exp(r2T))r2)>1, | (3.3) |
where
z∗3=√A23+B3 |
and
A=w22+r2(1−qer2T)2βk+m3(1−er2T),B=r2w2−βk+m3(1−er2T). |
Next we will prove that there must exist a t3>0. Set x(t3)≥m3 for t>t3. Otherwise, from Theorem 2.4, we obtain that y(t)≤z3(t)andz3(t)→z∗3(t) as t→∞, where z3(t) is the solution of system (3.4).
{dz3(t)dt=z3(t)(r2−βk+m3z3(t)),t≠nT,z3(t+)≤qz3(t)+w1m31+θm3+w2,t=nT, | (3.4) |
and
z∗3(t)=r2z∗3exp(r2(t−nT))r2−βk+m3z∗3(1−exp(r2(t−nT))),t∈(nT,(n+1)T),n∈Z+. | (3.5) |
Thus there is a T1 such that y(t)≤z3(t)≤z∗3(t)+ε3 as t>T1. Therefore we establish (3.6) as below:
{dx(t)dt≥x(t)(r1−bm3−α(z∗3(t)+ε3)a1),t≠nT,x(t+)=(1−δx(t)x(t)+h)x(t),t=nT. | (3.6) |
Let N∈Z+,NT>T; integrating (3.6) on (nT,(n+1)T), n≥N, we have
∫(n+1)TnTdx(t)x(t)dt≥∫(n+1)TnT(r1−bm3−α(z∗3(t)+ε3)a1)dt,lnx((n+1)T)−lnx(nT)≥∫(n+1)TnT(r1−bm3−α(z∗3(t)+ε3)a1)dt,lnx((n+1)T)x(nT)≥∫(n+1)TnT(r1−bm3−α(z∗3(t)+ε3)a1)dt,x((n+1)T)≥x(nT)exp(∫(n+1)TnT(r1−bm3−α(z∗3(t)+ε3)a1)dt)≥x(nT)exp((r−bm3−αε3a1)T−αa1k+a3βlnr2−βk+m3z∗3(1−exp(r2T))r2)). | (3.7) |
Then, given (3.3), substituting the right-hand side of (3.7) with η2, we get that x((n+1)T)≥x(nT)η2. Then x((N+n)T)>x(nT)ηn2→∞ as n→∞, which is a contradiction; hence, there must exist a t3>0 such that x(t3)≥m3.
2) Assume that x(t)≥m3 for all t>t3 and prove that the conclusion holds; otherwise, let t∗=inft>t3,x(t)<m3, and we have that x(t)≥m3 as t∈[t3,t∗]. It is divided into the following two cases: points in time of impulse and no impulse.
Case (1): t∗=nT,n1∈Z+. We have that q1m1≤q1x(t∗)=x(t∗+)≤m3. Select n2,n3∈Z+ such that
(1−δh)n2exp(ρn2T)ηn3>1, |
where ρ=r1−bm3−αa1M2<0. Let T′=n2T+n3T; there must exist a t4∈(t∗,t∗+T′) such that x(t4)≥m3. Otherwise, assume that x(t)<m3 for any t∈(t∗,t∗+T′); since (3.4) has an initial solution z3(t∗+)=y(t∗+), we can get
y(t)<z3(t)≤z3∗(t)+ε3,t∈[t∗+n2T,t∗+T′]. |
According to the above, we know that
x(t∗+T′)≥x(t∗+n2T)ηn3. |
Since y(t)≤M2, when t∈[t∗,t∗+n2T], we have
{dx(t)dt≥x(t)(r−bm3−αa1M2),t≠nT,x(t+)=(1−δx(t)x(t)+h)x(t),t=nT. | (3.8) |
Integrating (3.8) on the interval [t∗,t∗+n2T], we obtain
x(t∗+n2T)≥(1−δh)n2−1exp(ρn2T)x(t∗+)=(1−δh)n2exp(ρn2T)x(t∗)≥m3(1−δh)n2exp(ρn2T). | (3.9) |
So,
x(t∗+T′)≥x(t∗+n2T)ηn3=m3(1−δh)n2exp(ρn2T)ηn3>m3, | (3.10) |
which contradicts the above assumption and means that there must exist a t4∈(t∗,t∗+T′) such that x(t4)>m3.
Let ˉt=inf{t>t∗,x(t)≥m3}; we have that x(t)<m3 when t∈(t∗,ˉt] and x(ˉt)=m3. Assume that ˉt=inf{t>t∗,x(t)≥m3}; from (3.8), it follows that
x(t)≥x(t∗+)(1−δh)k−1exp(ρ(t−t∗))≥m3(1−δh)n2+n3exp(ρ(n2+n3)T)=m′1,t∈(t∗,ˉt). | (3.11) |
Therefore we get that x(t)≥m1′ for t∈(t∗,ˉt] when t>ˉt. We can still prove that the conclusion x(ˉt)≥m3 holds by employing the same method as above.
Case (2): t∗≠nT,n∈Z+. Then x(t)≥m3 for t∈[t3,t∗) and x(t∗)=m3. Assume that
t∗∈(n′1T,(n′1+1)T],n′1∈Z+ for t∈((t∗,(n′1+1)T)). |
There are two possible cases for x(t).
(H1): If x(t)<m3 for all t∈(t∗(n′1+1)T], it is similar to Case (1). We can prove that there exists a t5∈[(n′1+1)T,(n′1+1)T+T′] such that x(t5)≥m3. Otherwise assume that x(t)<m3 for all t∈[(n′1+1)T,(n′1+1)+T′]. Consider the initial solution z3((n′1+1)T∗)=y((n′1+1)T∗) of (3.4) with the initial values on [t∗,(n′1+1+n2+n3)T+T′]; we get that
x((n′1+1+n2+n3)T)≥x((n′1+1+n2)T)η2n3. | (3.12) |
Integrating (3.8), we have
x((n′1+1+n2)T)η2n3≥m3(1−δh)n2exp(ρ(1+n2)T). | (3.13) |
Therefore,
x((n′1+1+n2+n3)T)≥m3(1−δh)n2exp(ρ(1+n2)T)>m3. | (3.14) |
This contradicts the assumption.
Let ˆt=inf{t>t∗,x(t)>m3}. We have that x(t)<m3 when t∈(t∗,ˆt) and x(ˆt)=m3. For t∈(t∗,ˆt), suppose that
t∈(t∗+(l−1)T,t∗+lT),l≤n2+n3,l∈Z+. |
We have
x(t)≥m3(1−δh)l−1exp(ρlT)≥m3(1−δh)n2+n3exp(ρ(1+n2+n3)T)=m1<m′1. | (3.15) |
Thus, x(ˆt)≥m3 for t>ˆt. By repeating the above steps we can obtain that x(t)≥m1 as t≥t3.
(H2): If there exists a t∈(t∗,(n′1+1)T), we have that x(t)≥m3. Let t′=inf{t>t∗,x(t)>m3} such that x(t)<m3,t∈[t∗,t′), but x(t′)=m3 when t∈[t∗,t′); thus, we get
x(t)≥x(t∗)exp((t−t∗)ρ)≥m3exp(Tρ)>m1, |
since x(ˆt)≥m3. By repeating the steps above we can obtain that x(t)≥m1 as t>t3. In summary, when t≥t3, we can prove that x(t)≥m3.
Next, we will research the existence of a nontrivial solution near the pest-eradication solution by applying the bifurcation theorem of Rabinowitz.
Theorem 4.1. System (1.1) has a positive nontrivial periodic solution when T=T∗, and it is supercritical if αa2≤4ba1a3.
Proof. It is computationally convenient to exchange x and y and change the period T to τ.
{dxdt=(r2−βx(t)y(t)+k)x(t),t≠nT,dy(t)dt=(r1−by(t)−αx(t)a1+a2y(t)+a3x(t))y(t),t≠nT,x(t+)=qx(t)+w1y(t)1+θy(t)+w2,t=nT,y(t+)=(1−δy(t)y(t)+h)y(t),t=nT, | (4.1) |
let Φ be the solution of the non-impulsive system associated with system (4.1); also, we get that X(t)=Φ(t,X0) with X0=X(0). Define the mappings Θ1,Θ2:R2→R2 by
Θ1(x,y)=qx+w1y1+θy+w2,Θ2(x,y)=(1−δyy+h)y,ζ(t)=(y∗(t),0), | (4.2) |
and the mappings F1,F2:R2→R2 by
F1(x,y)=(r2−βx(t)y(t)+k)x(t),F2(x,y)=(r1−by(t)−αx(t)a1+a2y(t)+a3x(t))y(t). | (4.3) |
Thus, by computation, we get
d′0=1−(∂Θ2∂y⋅∂Φ2∂y)(τ0,X0)=1−(1−δy(y+2h)(y+h)2)exp(∫τ00r1−αy∗(t)a1+a3y∗(t)dt), | (4.4) |
where τ0 is the root of d0′=0. In fact, it is easy to see that d0′ is equivalent to τ0=T∗.
a′0=1−(∂Θ1∂x⋅∂Φ1∂x)(τ0,X0)=1−qexp(∫τ00r2−2βy∗(t)kdt)>0,b′0=−(∂Θ1∂x⋅∂Φ1∂y+∂Θ2∂y⋅∂Φ2∂y)(τ0,X0)=−(q∂Φ1∂y+1(1+θy)2∂Φ2∂y),∂2Φ2(τ0,X0)∂x∂y=∫τ00exp(∫τ0s∂F2(ζ(t))∂ydt)∂2F2(ζ(t))∂x∂yexp(∫s0∂F2(ζ(t))∂ydt)ds=−∫τ00exp(∫τ0s∂F2(ζ(t))∂ydt)αa1(a1+a3y∗(t))2exp(∫s0∂F2(ζ(t))∂ydt)ds<0. | (4.5) |
By the same method, we have
∂2Φ2(τ0,X0)∂y2=∫τ00exp(∫τ0s∂F2(ζ(t))∂ydt)∂2F2(ζ(t))∂y2exp(∫s0∂F2(ζ(t))∂ydt)ds+∫τ00(exp(∫τ0s∂F2(ζ(t))∂ydt)∂2F2(ζ(s))∂y∂x×∫s0exp(∫sv∂F1(ζ(t))∂xdt)∂F1(ζ(s))∂yexp(∫v0∂F2(ζ(t))∂ydt)dv)ds=∫τ00exp(∫τ0s∂F2(ζ(t))∂ydt)(−2b+2αa2y∗(t)(a1+a3y∗(t))2)exp(∫s0∂F2(ζ(t))∂ydt)ds−∫τ00exp(∫τ0s∂F2(ζ(t))∂ydt)αa1(a1+a3y∗(t))2×∫s0exp(∫sv∂F1(ζ(t))∂xdt)βy∗(t)2k2exp(∫v0∂F2(ζ(t))∂ydt)dv,∂2Φ2(τ0,X0)∂τ∂y=∂F2(ζ(τ0))∂yexp(∫τ00∂F2(ζ(t))∂ydt)=(r1−αy∗(t)a1+a3y∗(t))exp(∫τ00(r1−αy∗(t)a1+a3y∗(t))), | (4.6) |
so we can get
B=∂2Θ2∂x∂y(∂Φ1(τ0,X0)∂τ+∂Φ1(τ0,X0)∂x1a0′∂Θ1(τ0,X0)∂τ)∂Φ2(τ0,X0)∂y−∂Θ2∂y(∂2Φ2(τ0,X0)∂x∂y1a0′∂Θ1∂x∂Φ1(τ0,X0)∂τ+∂2Φ2(τ0,X0)∂τ∂y)=−(1−yδ(y+2h)(y+h)2)(∂2Φ2(τ0,X0)∂x∂y1a0′qy∗′(τ0)+(r1−αy∗(t)a1+a3y∗(t))exp(∫τ00(r1−αy∗(t)a1+a3y∗(t)))),C=−2∂2Θ2∂x∂y(a0′b0′∂Φ1(τ0,X0)∂x+∂Φ1(τ0,X0)∂y)∂Φ2(τ0,X0)∂y−∂2Θ2∂y2(∂Φ2(τ0,X0)∂y)2+2a0′b0′∂Θ2∂y∂2Φ2(τ0,X0)∂x∂y−∂Θ2∂y∂2Φ2(τ0,X0)∂y2)=−2δh(exp(∫t0∂F2(ζ(t))∂ydt))2+2a0′b0′(1−δy(y+2h)(y+h)2)∂2Φ2∂x∂y−(1−δy(y+2h)(y+h)2)∂2Φ2∂y2. | (4.7) |
To determine the sign of B and C, first assume that
ρ(t)=r1−αy∗(t)a1+a3y∗(t) |
which implies that
ρ′(t)=αa1y∗′(t)(a1+a3y∗(t))2>0. |
Then, ρ(t) is a strictly increasing function. Given that d0′=0, since
∫τ00ρ(t)dt=ln(1/(1−δy(y+2h)(y+h)2))>0, |
it is obvious that ρ(τ0)>0; so, ∂2Φ2(τ0,X0)∂y∂τ0>0 and B<0. Through analysis, we define the following:
ϖ(t)=−2b+2αa2t(a1+a3t)2. |
Apparently −2b+2αa2t(a1+a3t)2≤0 only such that αa2≤4ba1a3. This means that
ϖ(y∗(t))=−2b+2αa2y∗(t)(a1+a3y∗(t))2≤0 |
for all y∗(t)>0. So we get
∂2Φ(τ0,X0)∂y2<0. |
From (4.7) we have that C>0.
In summary, BC<0, and there is a supercritical bifurcation when T=T∗.
In order to confirm our theoretical results and facilitate interpretation, we will focus on the complex dynamics. The properties of dynamic systems can be obtained by conducting bifurcation analysis.
First, the influence of impulse period T on the dynamic characteristics of the system was studied. From Figure 1, we can ascertain that dynamic behaviors of system (1.1) become increasingly complicated as parameter T increases, and these include behaviors such as period-doubling bifurcation, period-halving bifurcation, chaotic bands, meanwhile, multiple attractors may coexist with the same T. Figure 2 shows that the pest free periodic solution has global stability when the impulse period stays below some threshold value in (A, B), and that different attractors can coexist with the same T=2.5 when we select different initial values i.e., (x0,y0)=(1.3,2.1),(x0,y0)=(3.5,6.8) in (C, D, E, F). The different solutions coexist for a wide range of parameters, which suggests that the final steady state of pest and predator populations depends on their initial densities, and these results confirm that changes in the impulse period T can greatly alter the dynamics of the system (1.1).
It can be ascertained from Figure 3 that the nonlinear impulse parameter w1 affect the dynamics of the system (1.1), and that with the increase of parameter w1, the system (1.1) undergoes period-doubling bifurcation, chaotic bifurcation, period-halving bifurcation and multi-stability. Figure 4 shows that attractors with different amplitudes appeared at w1=10.2. Therefore, the initial values of both pest and predator populations are critical.
The results shown in Figure 5 reveal how the maximal release of predators, denoted by w2 affects the dynamics of system (1.1). The properties of the positive periodic solution are maintained until w2=2.9, at which point a period-doubling bifurcation occurs. Additionally, as w2 increases, a series of period bifurcations cause the system (1.1) to move from periodicity to chaos. However, when w2≥20.2, the chaos disappears.
Regarding the predator-prey systems with an integrated pest management scheme, the impulse control strategies in many previous studies were linear, while ignoring the influence of nonlinear impulse on pest control such as the effects of limited resources. The number of natural enemies to be released must be based on the densities of the pest and predator populations in the field, so we have provided a nonlinear impulsive control predator-prey model with Leslie-Gower and B-D terms. The global stability of the pest extinction periodic solution of system (1.1) has been studied by applying the Floquet theory for differential equations and the comparison theorem, and the conditions for the permanence of system (1.1) has been obtained in detail. We have also proven that a nontrivial periodic solution can be obtained via a supercritical bifurcation once a threshold condition is reached within the context of bifurcation theory. In order to reveal how nonlinear impulses affect successful pest control strategies, we performed numerical simulations to show that system (1.1) has very complex dynamic behaviors, such as period-halving bifurcations, period-doubling bifurcation, chaos, and non-unique attractors. Comparing the bifurcation diagrams for parameters w1 and w2, it was found that small perturbations can cause periodic oscillations of different amplitudes and periods in the populations of pests and natural enemies, that is, the influence of nonlinear impulses on the dynamic behavior of the model is very sensitive, which shows that the predator-prey models with nonlinear impulses have richer dynamic properties. These bifurcation diagrams show that impulse period, insecticide dose, and initial population densities are critical to successful control strategies.
In view of these results, we believe that systems with nonlinear impulsive control are more reasonable and have more complex dynamic behavior than systems with linear impulsive control. Furthermore, nonlinear impulsive control should be taken into account when investigating predator-prey systems with IPM. However, a more realistic case is that the density dependent releasing function should depend on the densities of the prey and predator populations, i.e., the higher the density of pests or the lower the density of predators in the field, the higher the number of predators that should be released and vice versa. We will work on this in the near future.
The authors declare they have not used Artificial Intelligence (AI) tools in the creation of this article.
This work was partially supported by the National Natural Science Foundation of China (Nos. 11901370, 12001425); Natural Science Basic Research Plan in Shaanxi Province of China (No. 2020JM-569); Shaanxi Province Natural Science Basic Research Program (No. 2023JCYB258); Shaanxi Ecospatial Governance Key Research Project (No. 2022HZ1809); and Key Project of Postgraduate Education Reform of Xi'an Technological University (No. XAGDYJ220106).
The authors declare that there is no conflict of interest regarding the publication of this paper.
[1] |
P. Kalra, M. Kaur, Stability analysis of an eco-epidemiological SIN model with impulsive control strategy for integrated pest management considering stage-structure in predator, Int. J. Math. Model. Numer. Optim., 12 (2022), 43–68. https://doi.org/10.1504/IJMMNO.2022.119779 doi: 10.1504/IJMMNO.2022.119779
![]() |
[2] |
M. He, Z. Li, Stability of a fear effect predator-prey model with mutual interference or group defense, J. Biol. Dyn., 16 (2022), 480–498. https://doi.org/10.1080/17513758.2022.2091800 doi: 10.1080/17513758.2022.2091800
![]() |
[3] |
W. Gao, S. Tang, The effects of impulsive releasing methods of natural enemies on pest control and dynamical complexity, Nonlinear Anal.: Hybrid Syst., 5 (2011), 540–553. https://doi.org/10.1016/j.nahs.2010.12.001 doi: 10.1016/j.nahs.2010.12.001
![]() |
[4] |
J. Liu, Q. Qi, B. Liu, S. Gao, Pest control switching models with instantaneous and non-instantaneous impulsive effects, Math. Comput. Simul., 205 (2023), 926–938. https://doi.org/10.1016/j.matcom.2022.10.027 doi: 10.1016/j.matcom.2022.10.027
![]() |
[5] |
Y. Xiao, F. Van Den, The dynamics of an eco-epidemic model with biological control, Ecol. Model., 168 (2003), 203–214. https://doi.org/10.1016/S0304-3800(03)00197-2 doi: 10.1016/S0304-3800(03)00197-2
![]() |
[6] |
X. Zhang, Y. Wang, Y. Liu, P. Dai, J. Dong, Z. Yu, Approaches biological control of pests of through landscape regulation: theory and practice, J. Ecol. Rural Environ., 31 (2015), 617–624. https://doi.org/10.11934/j.issn.1673-4831.2015.05.001 doi: 10.11934/j.issn.1673-4831.2015.05.001
![]() |
[7] |
B. Liu, Y. Zhang, L. Chen, The dynamical behaviors of a Lotka-Volterra predator-prey model concerning integrated pest management, Nonlinear Anal., 6 (2005), 227–243. https://doi.org/10.1016/j.nonrwa.2004.08.001 doi: 10.1016/j.nonrwa.2004.08.001
![]() |
[8] |
J. Wang, H. Cheng, X. Meng, B. G. S. A. Pradeep, Geometrical analysis and control optimization of a predator-prey model with multi state-dependent impulse, Adv. Differ. Equ., 2017 (2017), 252. https://doi.org/10.1186/s13662-017-1300-5 doi: 10.1186/s13662-017-1300-5
![]() |
[9] |
H. Cheng, F. Wang, T. Zhang, Multi-state dependent impulsive control for Holling Ⅰ predator-prey model, Discrete Dyn. Nat. Soc., 2012 (2012), 181752. https://doi.org/10.1155/2012/181752 doi: 10.1155/2012/181752
![]() |
[10] |
D. Luo, Global bifurcation for a reaction-diffusion predator-prey model with Holling-Ⅱ functional response and prey-taxis, Chaos Soliton. Fract., 147 (2021), 110975. https://doi.org/10.1016/j.chaos.2021.110975 doi: 10.1016/j.chaos.2021.110975
![]() |
[11] |
M. Liu, C. Du, M. Deng, Persistence and extinction of a modified Leslie-Gower Holling-type Ⅱ stochastic predator-prey model with impulsive toxicant input in polluted environments, Nonlinear Anal.: Hybrid Syst., 27 (2018), 177–190. https://doi.org/10.1016/j.nahs.2017.08.001 doi: 10.1016/j.nahs.2017.08.001
![]() |
[12] |
L. Niu, X. Wang, Periodic solution for a delayed Leslie-Gower and Holling-Type Ⅲ predator-prey model incorporating prey cannibalism, Adv. Appl. Math., 9 (2020), 1170–1176. https://doi.org/10.12677/AAM.2020.98136 doi: 10.12677/AAM.2020.98136
![]() |
[13] |
Z. He, B. Li, Complex dynamic behavior of a discrete-time predator-prey system of Holling-Ⅲ type, Adv. Differ. Equ., 2014 (2014), 180. https://doi.org/10.1186/1687-1847-2014-180 doi: 10.1186/1687-1847-2014-180
![]() |
[14] |
M. Wang, Stationary patterns for a prey-predator model with prey-dependent and ration-dependent functional responses and diffusion, Phys. D: Nonlinear Phenom., 196 (2004), 172–192. https://doi.org/10.1016/j.physd.2004.05.007 doi: 10.1016/j.physd.2004.05.007
![]() |
[15] |
X. Feng, C. Sun, Y. Wang, C. Li, Dynamics of a predator-prey model with nonlinear growth rate and B-D functional response, Nonlinear Anal., 70 (2023), 103766. https://doi.org/10.1016/j.nonrwa.2022.103766 doi: 10.1016/j.nonrwa.2022.103766
![]() |
[16] |
X. Feng, M. Liu, Y. Jiang, D. Li, Dynamics and stability of a fractional-order Tumor-Immune interaction model with B-D functional response and immunotherapy, Fractal Fract., 7 (2023), 200. https://doi.org/10.3390/fractalfract7020200 doi: 10.3390/fractalfract7020200
![]() |
[17] |
Z. Xiang, X. Song, The dynamical behaviors of a food chain model with impulsive effect and Ivlev functional response, Chaos Soliton. Fract., 39 (2009), 2282–2293. https://doi.org/10.1016/j.chaos.2007.06.124 doi: 10.1016/j.chaos.2007.06.124
![]() |
[18] |
Z. Zhao, L. Yang, L. Chen, Impulsive perturbations of a predator-prey system with modified Leslie-Gower and Holling type Ⅱ schemes, J. Appl. Math. Comput., 35 (2011), 119–134. https://doi.org/10.1007/s12190-009-0346-2 doi: 10.1007/s12190-009-0346-2
![]() |
[19] |
H. Guo, X. Song, An impulsive predator-prey system with modified Leslie-Gower and Holling type Ⅱ schemes, Chaos Soliton. Fract., 36 (2008), 1320–1331. https://doi.org/10.1016/j.chaos.2006.08.010 doi: 10.1016/j.chaos.2006.08.010
![]() |
[20] |
C. Li, X. Feng, Y. Wang, X. Wang, Complex dynamics of Beddington-DeAngelis-Type predator-prey model with nonlinear impulsive control, Complexity, 2020 (2020), 8829235. https://doi.org/10.1155/2020/8829235 doi: 10.1155/2020/8829235
![]() |
[21] |
M. A. Aziz-Alaoui, M. Daher Okiye, Boundedness and global stability for a predator-prey model with modified Leslie-Gower and Holling-type Ⅱ type schemes, Appl. Math. Lett., 16 (2003), 1069–1075. https://doi.org/10.1016/S0893-9659(03)90096-6 doi: 10.1016/S0893-9659(03)90096-6
![]() |
[22] |
A. F. Nindjin, M. A. Aziz-Alaoui, M. Cadivel, Analysis of a predator-prey model with modified Leslie-Gower and Holling-type Ⅱ schemes with time delay, Nonlinear Anal., 7 (2006), 1104–1108. https://doi.org/10.1016/j.nonrwa.2005.10.003 doi: 10.1016/j.nonrwa.2005.10.003
![]() |
[23] |
I. U. Khan, S. Tang, The impulsive model with pest density and its change rate dependent feedback control, Discrete Dyn. Nat. Soc., 2020 (2020), 4561241. https://doi.org/10.1155/2020/4561241 doi: 10.1155/2020/4561241
![]() |
[24] |
C. Li, S. Tang, Analyzing a generalized pest-natural enemy model with nonlinear impulsive control, Open Math., 16 (2018), 1390–1411. https://doi.org/10.1515/math-2018-0114 doi: 10.1515/math-2018-0114
![]() |
1. | Changtong Li, Tongtong Dang, Xiaozhou Feng, Lifeng Wang, Dynamic complexity of a predator-prey model with nonlinear pulse and periodic factor, 2025, 2025, 2731-4235, 10.1186/s13662-025-03922-y |