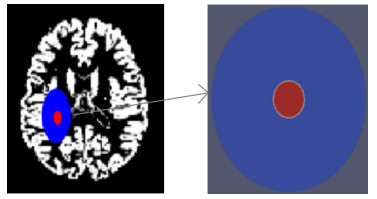
In this study, we introduced a mathematical model mimicking as much as possible the evolutions and interactions between glioma and lactate in the brain, in order to test different therapies and administration protocols. We simulated both glioma cell density evolution and lactate concentration, and considered two therapies: chemotherapy and a treatment targeting lactate production. Three different protocols for administrating the therapies were tested. We compared the efficiency of the combined therapies, depending on the administration protocols and the dosage of the drugs, in order to evaluate the importance of controlling lactate production. Results show that the use of an agent to reduce lactate concentration permits one to significantly reduce the dose of the chemotherapeutic drug.
Citation: Hussein Raad, Cyrille Allery, Laurence Cherfils, Carole Guillevin, Alain Miranville, Thomas Sookiew, Luc Pellerin, Rémy Guillevin. Simulation of tumor density evolution upon chemotherapy alone or combined with a treatment to reduce lactate levels[J]. AIMS Mathematics, 2024, 9(3): 5250-5268. doi: 10.3934/math.2024254
[1] | Ahmed J. Abougarair, Mohsen Bakouri, Abdulrahman Alduraywish, Omar G. Mrehel, Abdulrahman Alqahtani, Tariq Alqahtani, Yousef Alharbi, Md Samsuzzaman . Optimizing cancer treatment using optimal control theory. AIMS Mathematics, 2024, 9(11): 31740-31769. doi: 10.3934/math.20241526 |
[2] | Kaushik Dehingia, Hemanta Kumar Sarmah, Kamyar Hosseini, Khadijeh Sadri, Soheil Salahshour, Choonkil Park . An optimal control problem of immuno-chemotherapy in presence of gene therapy. AIMS Mathematics, 2021, 6(10): 11530-11549. doi: 10.3934/math.2021669 |
[3] | Monica Conti, Stefania Gatti, Alain Miranville . Mathematical analysis of a phase-field model of brain cancers with chemotherapy and antiangiogenic therapy effects. AIMS Mathematics, 2022, 7(1): 1536-1561. doi: 10.3934/math.2022090 |
[4] | Peter Romeo Nyarko, Martin Anokye . Mathematical modeling and numerical simulation of a multiscale cancer invasion of host tissue. AIMS Mathematics, 2020, 5(4): 3111-3124. doi: 10.3934/math.2020200 |
[5] | Folashade Agusto, Daniel Bond, Adira Cohen, Wandi Ding, Rachel Leander, Allis Royer . Optimal impulse control of West Nile virus. AIMS Mathematics, 2022, 7(10): 19597-19628. doi: 10.3934/math.20221075 |
[6] | Hsiu-Chuan Wei . Mathematical modeling of ER-positive breast cancer treatment with AZD9496 and palbociclib. AIMS Mathematics, 2020, 5(4): 3446-3455. doi: 10.3934/math.2020223 |
[7] | Xingxiao Wu, Lidong Huang, Shan Zhang, Wenjie Qin . Dynamics analysis and optimal control of a fractional-order lung cancer model. AIMS Mathematics, 2024, 9(12): 35759-35799. doi: 10.3934/math.20241697 |
[8] | Zholaman Bektemessov, Laurence Cherfils, Cyrille Allery, Julien Berger, Elisa Serafini, Eleonora Dondossola, Stefano Casarin . On a data-driven mathematical model for prostate cancer bone metastasis. AIMS Mathematics, 2024, 9(12): 34785-34805. doi: 10.3934/math.20241656 |
[9] | Houssine Zine, Abderrahim El Adraoui, Delfim F. M. Torres . Mathematical analysis, forecasting and optimal control of HIV/AIDS spatiotemporal transmission with a reaction diffusion SICA model. AIMS Mathematics, 2022, 7(9): 16519-16535. doi: 10.3934/math.2022904 |
[10] | Suprawee Lertnaweephorn, Usa Wannasigha Humphries, Amir Khan . Stability analysis and optimal control for leaf brown spot disease of rice. AIMS Mathematics, 2023, 8(4): 9602-9623. doi: 10.3934/math.2023485 |
In this study, we introduced a mathematical model mimicking as much as possible the evolutions and interactions between glioma and lactate in the brain, in order to test different therapies and administration protocols. We simulated both glioma cell density evolution and lactate concentration, and considered two therapies: chemotherapy and a treatment targeting lactate production. Three different protocols for administrating the therapies were tested. We compared the efficiency of the combined therapies, depending on the administration protocols and the dosage of the drugs, in order to evaluate the importance of controlling lactate production. Results show that the use of an agent to reduce lactate concentration permits one to significantly reduce the dose of the chemotherapeutic drug.
Gliomas represent 80% of all malignant brain tumors. High grade gliomas (grade Ⅲ and Ⅳ) tends to grow back even after complete excision. Radiation and chemotherapy remain the treatment of choice, although they are not optimal. They give rise to several undesirable side effects, making it necessary to improve their efficiency and reduce their detrimental impact. Recently, the role of lactate as an important substrate to sustain tumor growth has emerged, suggesting that controlling its production by the tumor itself might be an efficient therapeutic option, especially in combination with standard chemotherapy [4,7,18,22]. Lactate can be identified and quantified at high field MRI (3T & 7T) by MRSpectroscopy during imaging monitoring.
In this study, we introduced a mathematical model mimicking as much as possible the evolutions and interactions between glioma and lactate in the brain in order to test different therapies and administration protocols. We simulated both tumor density evolution with a parabolic equation and lactate concentration using a Cahn-Hilliard type of equation when two different therapies are considered. The effect of chemotherapy alone or in combination with a treatment to target lactate production was studied following two standard clinical protocols and a theoretical protocol suggested to improve treatment efficacy. Simulations were performed for either grade Ⅲ or grade Ⅳ patients with the goal of achieving a substantial reduction of the tumor. Results show that the use of an agent to reduce lactate concentration permits one to significantly reduce the dose of the chemotherapeutic drug. Moreover, for the three protocols tested, it appears that if Monocarboxylate Transporters (MCT) inhibitors are given continuously, it allows for the greatest reduction in chemotherapeutic agent concentration while maintaining the same efficacy. In conclusion, our data suggest it would be possible to combine chemotherapy and a treatment to decrease lactate levels for reducing the dose of the chemotherapeutic agent, thus potentially reducing its side effects for the patient.
We propose a mathematical model coupling the evolution of a high grade glioma with the evolution of the intracellular brain lactate concentrations, when two different therapies are being administered: on the one hand, a conventional chemotherapeutic agent (namely Temozolomide) acting on cancer cells, and on the other hand, a drug targeting lactate production (e.g. an MCT inhibitor).
Among the many equations modeling a tumor growth in the literature (see [5,8,9,12,17,27], and the references therein), we chose the diffusion and proliferation equation (see [13])
{∂tu−div(D∇u)=ρu(1−uN)−gu,x∈Ω,t∈(0,T)D∇u⋅n|∂Ω=0 t∈(0,T) | (2.1) |
where u(=u(x,t)) is the tumor cell density, Ω a bounded and regular domain of R2 standing for the part of the brain surrounding the glioma (see Figure 1), n the outward unit vector normal to the boundary ∂Ω, and T the duration of the therapy. The evolution of the tumor cell density takes into account diffusion through the matrix D (mm2day−1), the balance between the proliferation and apoptosis through the rate ρ (per day), and the necrosis through the rate g (per day). The constant N is the carrying capacity (maximum number of cells that can fit 1 mm2 of tissue).
To our knowledge, the first brain lactate kinetics model in the literature (see [3,11,14] and the references therein) dealt with the evolution of both capillary and intracellular lactate concentrations. It consisted of two equations and numerous parameters, such as the cerebral blood flow and the arterial lactate concentrations. Since here we had in mind the identification of the equation parameters from some in vivo data, we neglected the capillary lactate concentration in order to simplify the model. More precisely, we only deal with the evolution of the intracellular lactate concentration φ(=φ(x,t)). Following a previous work from [19,23], we considered here a Cahn-Hilliard type equation. Indeed, unlike more commonly used reaction-diffusion equations, this equation allows us to take into account the heterogeneity of the lactate concentrations both in malignant and normal cells. This heterogeneity is now well established (see [16,25]). Then, the equation for the intracellular lactate concentration can be formulated as
{∂tφ+αΔ2φ−Δf(φ)+kφk′+|φ|=J,x∈Ω,t∈(0,T)∂nφ|∂Ω=∂nΔφ|∂Ω=0t∈(0,T) | (2.2) |
where k is related to the maximum transport velocity between the blood and the cell, k′ is a modified Michaelis-Menten positive constant, and α is the diffusion constant. The term J characterizes the balance between production and consumption of lactate by the cells, and implicitly takes into account the capillary lactate. The function f is the derivative of a double well polynomial function of order 4. We assume that only k and J in the lactate equation depend on the tumor cell density, whereas ρ and g in the tumor equation depend on the lactate concentration. Besides, we take into account a standard cytotoxic drug acting on tumor cells v(=v(t)) and a treatment targeting lactate production ω(=ω(t)) (see [2]). The drug ω takes values in [0,1].
The complete model finally reads
{∂tu−div(D∇u)=(ρ(φ)−v(t))u(1−uN)−ug(φ),x∈Ω,t∈(0,T)∂tφ+αΔ2φ−Δf(φ)+k(u)φk′+|φ|=(1−ω(t))J(u),x∈Ω,t∈(0,T)D∇u⋅n|∂Ω=∂nφ|∂Ω=∂nΔφ|∂Ω=0x∈∂Ω,t∈(0,T)u(0)=u0,φ(0)=φ0. | (2.3) |
Our aim is to adjust the dosage of the treatments v and ω in order to reduce the tumor and lactate concentrations, up to desired targets. Hence, we introduced the following cost functional to minimize:
J(v,ω,u,φ)=b12∫T0∫Ω(u(x,t)−ˆu(x,t))2dxdt+b22∫Ω(u(x,T)−ˆu(x,T))2dx+b32∫T0∫Ω(φ(x,t)−ˆφ(x,t))2dxdt+b42∫Ω(φ(x,T)−ˆφ(x,T))2dx+b52∫T0ω(t)2dt+b62∫T0v(t)2dt | (2.4) |
where bi, i=1,..,6, are positive constants. Here, T stands for the duration of the therapy, and the functions ˆu and ˆφ refer to prescribed targets for the tumor cell densities and the lactate concentrations respectively, in Ω×[0,T]. The last two terms of J penalize large quantities of drug in order to limit the dangerous side effects of treatments.
The mathematical analysis of problem (2.3) and of the control problem associated to (2.3) and (2.4) can be found in [24]. However, the main results are summarized in the following theorem.
Theorem 2.1. Let T>0 and (u0,φ0) be given in H1(Ω)×L2(Ω) such that 0≤u0≤N. We set Uad={(v,ω)∈L∞(Ω×[0,T])2; 0≤v≤Vmax, 0≤ω≤1}.
For all (v,ω)∈Uad, problem (2.3) has a unique weak solution (u,φ) such that 0≤u≤N and
u∈C([0,T],L2(Ω))∩L2(0,T;H1(Ω)); φ∈C([0,T];H1(Ω))∩L2(0,T;H3(Ω)). |
The control-to state map S is defined by
S:(v,ω)∈Uad↦S(v,ω)=(u,φ) |
where (u,φ) is the unique solution to (2.3) with initial data (u0,φ0) and therapy dosages (v,ω) over [0,T].
Moreover, there exists at least a pair (v∗,ω∗) such that
inf(v,ω)∈UadJ(v,ω,S(v,ω))=J(v∗,ω∗,S(v∗,ω∗)) | (2.5) |
with J defined by (2.4).
In the following, we denote by "optimal dosage" the pair (v∗,ω∗) solution to the minimization problem (2.5). This means that ω∗ and v∗ are the smallest therapy dosages allowing to reach the given targets. The optimal dosage is computed using a descent optimization algorithm based on an adjoint equation, which are both specified in the Appendix.
When we do not consider treatment for lactate, the optimal chemotherapy dosage stands for the solution of the minimization problem (2.5), taking ω=0 in (2.3) and (2.4).
During the simulations below, we tested three protocols:
● Stupp's protocol - 1 day: A chemotherapy cycle lasting 4 weeks, the chemotherapy is being administered during 1 day at the beginning of the cycle (v(t)=v>0), then v(t)=0 till the end of the cycle. Computations are performed up to 24 weeks, namely 6 cycles of chemotherapy, the first cycle starting at t=0.
● Stupp's protocol - 5 days (see [28]): A chemotherapy cycle lasting 4 weeks, the chemotherapy is being administered during 5 days at the beginning of the cycle (v(t)=v>0), then v(t)=0 till the end of the cycle. Computations are performed up to 24 weeks, namely 6 cycles of chemotherapy, the first cycle starting at t=0.
● Third Protocol (see [26]): A chemotherapy cycle lasting 6 weeks, the chemotherapy is being administered during 2 weeks at the beginning of the cycle (v(t)=v>0), then v(t)=0 till the end of the cycle. Computations are performed up to 24 weeks, namely 4 cycles of chemotherapy, the first cycle starting at t=0.
As far as the computations are concerned, we made the following assumptions:
● We chose b1=b2=10−6, b3=b4=1, in order to take into account the different orders of magnitude between the tumor cell density and the lactate concentration, and b5=b6=10−8 as Tikhonov regularization coefficients.
● We know from biological observations that k,J are increasing with respect to the tumor, and ρ,g are increasing with respect to the lactate. Without precise information provided by the literature, we assumed that the growths are linear:
k(u)=k0+k1u, J(u)=J0+J1u, with k0, J0>0 standing for normal values (in healthy tissues), and k1,J1>0.
ρ(φ)=ρ0+ρ1φ, with ρ0,ρ1>0.
g(φ)=g1φ, g1≥0.
In this section, Ω is an ellipse parametrized by x=6cosθ and y=8sinθ, with θ∈[0,2π]. Inside the domain Ω, the tumor is initially delimited by a smaller ellipse centered at (0,0) and defined with x=1.2cosθ and y=1.6sinθ. We set α=0.01, f(φ)=0.002φ(φ−2)(φ−4), N=106, g1=0 (no necrosis). Moreover, we chose:
k0=0.0764 mM/day k1=8×10−5,
J0=0.0678 mM/day J1=8×10−5,
ρ0=0.012 day−1 ρ1=0.1,
k′=0.515 mM,
D(x)=7.5×10−3(1005).
The "normal" values k0, J0, and k′ were identified on the basis of lactate data from the University Hospital of Poitiers (see [23] for details). The parameter ρ0 was taken from [17,26]. Without any information in the literature about the other parameters, we chose data leading to biologically coherent simulations. We used the following initial conditions and targets:
φ0={4.37mMinside the tumor0.8mMoutside, ˆφ={1.2mMinside the tumor0.8mMoutside,
u0={4×105inside the tumor0outside, ˆu={104inside the tumor0outside.
We emphasize that 0.8 mM is a normal value for lactate in healthy tissues. Inside the tumor area, we chose initial data for lactate and tumor consistent with a patient of grade 3 and we searched for a substantial but not complete reduction of the tumor within 24 weeks.
The figures below display the trajectories of lactate concentrations and tumor cell densities with respect to time, at a point of the brain localized in the center of the tumor. Our purpose is to compare the optimal chemotherapy dosages required for attaining the target for the tumor, under the different delivery protocols, with or without additional treatment for lactate.
In Figure 2 the chemotherapy is administered following the Stupp's 1-day protocol, without treatment for lactate. The target for the tumor cell density is obtained with a large optimal dosage of the chemotherapy, namely v∗=127.1day−1. The lactate concentration still increases during the therapy. But, it stabilizes at the end due to the decrease of the tumor cell density.
In Figure 3, the chemotherapy and the treatment for lactate are administered concomitantly, following the Stupp's 1-day protocol. Even though the target for lactate cannot be attained (ω∗=1 means that the production of lactate is entirely disrupted), the concentration is reduced, compared to Figure 2. The chemotherapy dosage is very slightly reduced (v∗=124.1day−1).
In Figure 4, the chemotherapy still follows the Stupp's 1-day protocol, but the treatment for lactate is administered continuouly (every day). This time, both the targets for lactate and the tumor are achieved (ω∗=0.44 and v∗=108.7day−1). We emphasize that the optimal chemotherapy dosage is reduced by 14.5%, in comparison to the results of Figure 2.
We carried out again the same tests as above, the only difference being that, this time, the chemotherapy is administered following the Stupp's 5-days protocol. As expected, the order of magnitude of the chemotherapy quantity administered per day is much smaller with this protocol.
The results are similar to those of section 3.1: With chemotherapy alone, lactate still increases and stabilizes at the end (see Figure 5); with a concomitant treatment, the target for lactate cannot be attained (see Figure 6), whereas it is achieved when the treatment for lactate is administered continuously (see Figure 7). The optimal dosage for chemotherapy with continuous therapy is reduced by 11% with an everyday treatment for lactate.
We carried out again the same three tests as above, but now the chemotherapy is administered according to the third protocol (see Figures 8, 9, 10). We emphasize that, this time, with a concomitant treatment the target for lactate is almost attained; it is achieved when the treatment is administered continuously. The optimal dosages for chemotherapy are very similar, whatever the way the treatment for lactate is administered (v∗=8.14day−1 or v∗=8.19day−1). Moreover, combining the two therapies leads to a reduction of the optimal chemotherapy dosage of about 11%.
For the simulations in this section, Ω is an ellipse parametrized by x=6cosθ and y=8sinθ, with θ∈[0,2π]. Inside Ω, the tumor is (initially) delimited by a smaller ellipse centered at (0,0) and defined with x=1.5cosθ and y=2sinθ. We set α=0.01, f(φ)=0.002φ(φ−2)(φ−4), and N=106. Moreover, we take:
k0=0.0374 mM/day k1=5×10−5,
J0=0.0134 mM/day J1=5×10−5,
ρ0=0.012 day−1 ρ1=0.2,
k′=1.31 mM g1=0.002,
D(x)=7.5×10−3(20010).
As before, the "normal" values k0, J0, and k′ were identified on the basis of lactate data from the University Hospital of Poitiers (see [23] for details). The parameter ρ0 was taken from the literature [17,26]. Without any information about the other parameters, we chose data which lead to consistent simulations. We used the following initial conditions and targets:
φ0={8.54 mMinside the tumor0.8 mMoutside ˆφ={2 mMinside the tumor0.8 mMoutside,
u0={7×105inside the tumor0outside ˆu={105inside the tumor0outside.
We chose initial data for lactate and tumors consistent with a patient of grade 4 and we searched for a substantial but not complete reduction of the tumor within 24 weeks.
Again, the figures below display the trajectories of the lactate concentration and tumor cell densities with respect to time, at a point of the brain localized in the center of the tumor. We compare the optimal chemotherapy dosages required for attaining the targets. Since Stupp's 1-day protocol is not used by oncologists for patients of grade 4, we only tested Stupp's 5-days protocol and the third protocol.
In Figure 11, the chemotherapy is administered following Stupp's 5-days protocol, without treatment for lactate. The target for the tumor cell density is obtained with the optimal dosage v∗=21.9day−1. The lactate concentration still increases during the therapy. But, at the end, the decrease of the tumor cell density leads to a decrease of lactate.
In Figure 12, chemotherapy and the treatment for lactate are administered concomitantly, following Stupp's 5-days protocol. Even though the target for lactate cannot be attained (ω∗=0.99), its concentration is reduced. Compared to the sole chemotherapy treatment (Figure 11), the optimal chemotherapy dosage v∗=18.6day−1 has decreased by about 15%.
In Figure 13, the dosage for chemotherapy is fixed (v=21.9day−1). We tested three dosages for the treatment targeting the lactates (ω=0, ω=0.48, and ω=0.9) and compared the resulting trajectories for the lactate concentration and tumor cell density. As expected, the decrease of the tumor cell density is enhanced with the combination of the two therapies; the lactate concentration is stabilized.
In Figure 14, the chemotherapy still follows Stupp's 5-days protocol, but the treatment for lactate is administered continuouly (every day). This time, both the targets for lactate and the tumor are attained (ω∗=0.48 and v∗=14.4day−1). The optimal chemotherapy dosage is reduced by 34% in comparison to the results of Figure 11 thanks to the additional therapy for lactate.
In Figure 15, the dosage for the treatment targeting the lactates is fixed (ω=0.48) and we tested two dosages for the chemotherapy (v=21.9day−1 and v=14.4day−1), and the combination of ω=0.48 and v=14.4day−1 was determined to be optimal (see Figure 14). As expected, the decrease of the tumor is improved with the higher chemotherapy dosage v=21.9day−1. However, the evolution of the lactate concentration may be surprising at first sight: the therapy for lactate seems to be more efficient for higher cell density tumors (i.e. for smaller dosage of chemotherapy). This observation might be explained by the higher production of lactate by high density tumors, which accounts for their aggressiveness. This result seems consistent with in vivo observations from patients.
We compare again the optimal treatment dosages required to attain the targets for the tumor cell density and lactate concentration, according to the third protocol (see Figures 16, 17, 18). We emphasize that, with this protocol, the target for lactate is almost attained with the concomitant delivery of treatment (Figure 17), and attained with continuous delivery (Figure 18). In both cases, the reduction of the optimal chemotherapy dosage is substantial (around 30%).
Figure 19 displays the evolution of the tumor cell density before, at the middle, and at the end of the optimal therapy, in the case of continuous lactate therapy (ω∗=0.49, v∗=7.46 day−1).
In the various simulations carried out on grade Ⅲ and Ⅳ gliomas, as well as on the more theoretical one based on Swanson's growth model (close to diffuse low-grade glioma, WHO Ⅱ), the following common elements were observed.
(i) Complementary "anti-lactate" treatment, such as anti-MCT 1 and 4, enhances the efficiency of conventional treatment in all the scenarios studied above (see summary in Table 1). These findings, confirmed by results obtained in vivo on WHO Ⅲ and Ⅳ gliomas using proton spectroscopy at 3 and 7 Teslas, are consistent with the now well-known role of lactate as an important alternative energetic and biosynthetic substrate for oxidative metabolism in glioma and its capacity to sustain their growth under conditions of glucose deprivation [21].
Grade | Protocol | Only chemotherapy | Concomitant therapy | Continuous therapy |
3 | Stupp 1 | v∗=127.1 | ω∗=1, v∗=124.1 | ω∗=0.44, v∗=108.7 |
3 | Stupp 5 | v∗=17.8 | ω∗=1, v∗=16.6 | ω∗=0.43, v∗=15.8 |
3 | Third | v∗=9.17 | ω∗=1, v∗=8.14 | ω∗=0.43, v∗=8.19 |
4 | Stupp 5 | v∗=21.9 | ω∗=0.99, v∗=18.6 | ω∗=0.48, v∗=14.4 |
4 | Third | v∗=11.15 | ω∗=1, v∗=8.02 | ω∗=0.49, v∗=7.46 |
More recently, 13C MRS studies have demonstrated a drastic reduction in lactate production by IDH1 mutated gliomas, unaffected by Temozolomide (TMZ) treatment. Conversely, mutant IDH1 cells and tumors produced significantly less hyperpolarized [1–13C] lactate compared to glioblastoma, consistent with their metabolic reprogramming, thus leading to less growth and less invasiveness observed in IDH1-mutated glioma [10]. Finally, lactate is involved in remodeling the tumor microenvironment and glial growth [20]. This simulation could therefore be a realistic adjuvant treatment option, as a pharmacological MCT inhibition strategy in cancer is now reaching the clinical stage [15].
(ii) Continuous, daily administration results in a faster decline in metabolic activity than sequential, monthly administration concomitant with conventional chemotherapy. The simulations presented suggest an increase in treatment efficacy, and/or a reduction in chemotherapy doses. The possibility of combining more conventional chemotherapy with monocarboxylate transporter inhibitors to reverse tumor progression has not only been considered previously but it has been tested with a certain success in various pre-clinical cancer models [4,7,18,22]. However, in order to implement this approach at the clinical level, it is mandatory to be able to determine for each patient the exact doses and regimen to aim for the greatest efficacy while reducing potential side effects. Here we provide evidence of its feasibility based on a modeling approach.
The integration of these simulations, based on real data obtained in vivo, makes it possible to envisage a different approach to treatment based on the interaction of numerical simulations and in vivo measurements, aimed at (ⅰ) limiting the toxicity of certain treatments and (ⅱ) better predicting their efficacy, even before they are administered. This approach could enable innovative therapeutics to be tested upstream of clinical experimentation. It is therefore a promising path towards personalized medicine and the digital twin.
The authors declare they have not used Artificial Intelligence (AI) tools in the creation of this article.
The authors wish to thank the anonymous referees for their careful reading of the paper and helpful comments.
The authors declare that there is no conflict of interest in this paper.
For the sake of completeness, we describe the descent optimization algorithm mentioned in section 2 for the computation of the optimal dosage of treatment. To this aim, we first give the adjoint equations associated with the state problem (2.3):
Let (u∗,φ∗)=S(v∗,ω∗). Find (ξ,π,y) the solution to
{−∂tξ+αΔπ−f′(φ∗)π+k(u∗)h′(φ∗)ξ−a′(φ∗)u∗(1−u∗N)y+P′(φ∗)u∗y−b3(φ∗−ˆφ)=0,on (0,T)×Ωπ=Δξ,on (0,T)×Ω−∂ty−div(D∇y)+k′(u∗)h(φ∗)ξ−(1−ω∗)J′(u∗)ξ−(a(φ∗)−v∗)(1−2u∗N)y+P(φ∗)y−b1(u∗−ˆu)=0,on (0,T)×Ω∂νξ=∂νπ=D∇y.ν=0on (0,T)×Γξ(T)=b4(ˆφ(T)−φ∗(T)),y(T)=b2(ˆu(T)−u∗(T))in Ω, |
with h(φ)=φk′+|φ|. The state and adjoint equations were spatially discretized by a finite element method. The mesh was obtained by dividing Ω into 31,735 triangles. For the time discretization, a Crank-Nicolson scheme was considered for the state equations (2.3), and an implicit Euler scheme for the adjoint equations (A.1). The time step was taken as δt=0.01.
The descent optimization algorithm is summarized below.
Algorithm 1 Descent optimization algorithm |
Require: n=0, v0=0 and ω0=0, E=1 |
while E>10−8 do |
Solve the state equation (2.3) with v=vn and ω=ωn on [0,T] and get un and φn |
Evaluate the objective functional J(ωn,vn,φn,un) |
Find (ξn,πn,yn) solution of the adjoint equation (A.1) with u∗=un, φ∗=φn, v∗=vn and ω∗=ωn. |
Evaluate the direction of descent by: |
dnv=−b6∫T0vndt+∫T0∫Ωun(1−unN)yndxdtdnω=−b5∫T0ωndt+∫T0∫ΩJ(un)ξndxdt |
Search the descent step σn by Armijo's rule (see [6]) |
Update the control parameter: |
vn+1=vn+σndnvωn+1=ωn+σndnω |
Evaluate the error E=|J(vn+1,ωn+1,un+1,φn+1)−J(vn,ωn,un,φn)||J(vn+1,ωn+1,un+1,φn+1)| |
n=n+1 |
end while |
[1] |
J. C. L. Alfonso, K. Talkenberger, M. Seifert, B. Klink, A. Hawkins-Daarud, K. R. Swanson, et al., The biology and mathematical modelling of glioma invasion: a review, J. R. Soc. Interface, 14 (2017), 20170490. https://doi.org/10.1098/rsif.2017.0490 doi: 10.1098/rsif.2017.0490
![]() |
[2] |
H. Alsayed, H. Fakih, A. Miranville, A. Wehbe, On an optimal control problem describing lactate production inhibition, Appl. Anal., 102 (2023), 1711–1731. https://doi.org/10.1080/00036811.2021.1999418 doi: 10.1080/00036811.2021.1999418
![]() |
[3] |
A. Aubert, R. Costalat, P. J. Magistretti, L. Pellerin, Brain lactate kinetics: modeling evidence for neuronal lactate uptake upon activation, Proceedings of the National Academy of Sciences of the United States of America, 102 (2005), 16448–16453. https://doi.org/10.1073/pnas.0505427102 doi: 10.1073/pnas.0505427102
![]() |
[4] |
D. Benjamin, M. N. Hall, Combining metformin with lactate transport inhibitors as a treatment modality for cancer - recommendation proposal, Front. Oncol., 12 (2022), 1034397. https://doi.org/10.3389/fonc.2022.1034397 doi: 10.3389/fonc.2022.1034397
![]() |
[5] |
M. U. Bogdańska, M. Bodnar, J. Belmonte-Beita, M. Murek, P. Schucht, J. Beck, et al., A mathematical model of low grade gliomas treated with temozolomide and its therapeutical implications, Math. Biosci., 288 (2017), 1–13. https://doi.org/10.1016/j.mbs.2017.02.003 doi: 10.1016/j.mbs.2017.02.003
![]() |
[6] | F. Bonnans, J.-C. Gilbert, C. Lemaréchal, C. Sagastizábal, Optimisation Numérique: Aspects théoriques et pratiques (Mathématiques et Applications), Springer, 1997. |
[7] |
H. E. Bridgewater, E. M. Bolitho, I. Romero-Canelón, P. J. Sadler, J. P. C. Coverdale, Targeting lactate metabolism with synergistic combinations of synthetic catalysts and monocarboxylate transporter inhibitors, J. Biol. Inorg. Chem., 28 (2023), 345–353. https://doi.org/10.1007/s00775-023-01994-3 doi: 10.1007/s00775-023-01994-3
![]() |
[8] |
L. E. B. Cabrales, J. I. Montijano, M. Schonbek, A. R. S. Casta˜neda, A viscous modified Gompertz model for the analysis of the kinetics of tumors under electrochemical therapy, Mathematics and Computers in Simulations, 151 (2018), 96–110. https://doi.org/10.1016/j.matcom.2018.03.005 doi: 10.1016/j.matcom.2018.03.005
![]() |
[9] |
A. R. S. Casta˜neda, J. M. Pozo, E. E. Ramirez-Torres, E. J. R. Oria, S. B. Vaillant, J. I. Montijano, et al., Spatio temporal dynamics of direct current in treated anisotropic tumors, Mathematics and Computers in Simulations, 203 (2023), 609–632. https://doi.org/10.1016/j.matcom.2022.07.004 doi: 10.1016/j.matcom.2022.07.004
![]() |
[10] |
M. M. Chaumeil, M. Radoul, C. Najac, P. Eriksson, P. Viswanath, M. D. Blough, et al., Hyperpolarized 13C MR imaging detects no lactate production in mutant IDH1 gliomas: Implications for diagnosis and response monitoring, Neuroimage Clin., 12 (2016), 180–189. https://doi.org/10.1016/j.nicl.2016.06.018 doi: 10.1016/j.nicl.2016.06.018
![]() |
[11] |
L. Cherfils, S. Gatti, C. Guillevin, A. Miranville, R. Guillevin, On a tumor growth model with brain lactate kinetics, Math. Med. Biol., 39 (2022), 382–409. https://doi.org/10.1093/imammb/dqac010 doi: 10.1093/imammb/dqac010
![]() |
[12] |
P. Colli, H. Gomez, G. Lorenzo, G. Marinoschi, A. Reali, E. Rocca, Mathematical analysis and simulation study of a phase-field model of prostate cancer growth with chemotherapy and antiangiogenic therapy effects, Math. Mod. Meth. Appl. Sci., 30 (2020), 1253–1295. https://doi.org/10.1142/S0218202520500220 doi: 10.1142/S0218202520500220
![]() |
[13] |
H. Gomez, Quantitative analysis of the proliferative-to-invasive transition of hypoxic glioma cells, Integr. Biol., 9 (2017), 257–262. https://doi.org/10.1039/C6IB00208K doi: 10.1039/C6IB00208K
![]() |
[14] |
C. Guillevin, R. Guillevin, A. Miranville, A. Perillat-Mercerot, Analysis of a mathematical model for brain lactate kinetics, Math. Biosci. Eng., 15 (2018), 1225–1242. https://doi.org/10.3934/mbe.2018056 doi: 10.3934/mbe.2018056
![]() |
[15] |
S. Halford, G. J. Veal, S. R. Wedge, G. S. Payne, C. M. Bacon, P. Sloan, et al., A Phase I Dose-escalation Study of AZD3965, an Oral Monocarboxylate Transporter 1 Inhibitor, in Patients with Advanced Cancer, Clin. Cancer Res., 29 (2023), 1429–1439. https://doi.org/10.1158/1078-0432.CCR-22-2263 doi: 10.1158/1078-0432.CCR-22-2263
![]() |
[16] |
P. Jacquet, A. Stéphanou, Metabolic Reprogramming, Questioning, and Implications for Cancer, Biology, 10 (2021), 129. https://doi.org/10.3390/biology10020129 doi: 10.3390/biology10020129
![]() |
[17] |
S. Jbabdi, E. Mandonnet, H. Duffau, L. Capelle, K. R. Swanson, M. Pélégrini-Issac, et al., Simulation of anisotropic growth of low-grade gliomas using diffusion tensor imaging, Magn. Reson. Med., 54 (2005), 616–624. https://doi.org/10.1002/mrm.20625 doi: 10.1002/mrm.20625
![]() |
[18] |
S. Kumstel, T. Schreiber, L. Goldstein, J. Stenzel, T. Lindner, M. Joksch, et al., Targeting pancreatic cancer with combinatorial treatment of CPI-613 and inhibitors of lactate metabolism, PLoS ONE, 17 (2022), e0266601. https://doi.org/10.1371/journal.pone.0266601 doi: 10.1371/journal.pone.0266601
![]() |
[19] |
L. Li, A. Miranville, R. Guillevin, Cahn-Hilliard models for glial cells, Appl. Math. Optim., 84 (2021), 1821–1842. https://doi.org/10.1007/s00245-020-09696-x doi: 10.1007/s00245-020-09696-x
![]() |
[20] |
L. Longhitano, N. Vicario, S. Forte, C. Giallongo, G. Broggi, R. Caltabiano, et al. Lactate modulates microglia polarization via IGFBP6 expression and remodels tumor microenvironment in glioblastoma, Cancer Immunol. Immun., 72 (2023), 1–20. https://doi.org/10.1007/s00262-022-03215-3 doi: 10.1007/s00262-022-03215-3
![]() |
[21] |
N. Minami, K. Tanaka, T. Sasayama, E. Kohmura, H. Saya, O. Sampetrean, Lactate Reprograms Energy and Lipid Metabolism in Glucose-Deprived Oxidative Glioma Stem Cells, Metabolites, 11 (2021), 325. https://doi.org/10.3390/metabo11050325 doi: 10.3390/metabo11050325
![]() |
[22] |
R. A. Noble, H. Thomas, Y. Zhao, L. Herendi, R. Howarth, I. Dragoni, et al., Simultaneous targeting of glycolysis and oxidative phosphorylation as a therapeutic strategy to treat diffuse large B-cell lymphoma, Brit. J. Cancer, 127 (2022), 937–947. https://doi.org/10.1038/s41416-022-01848-w doi: 10.1038/s41416-022-01848-w
![]() |
[23] | H. Raad, C. Allery, L. Cherfils, R. Guillevin, Optimal control of a model for brain lactate kinetics, Asymptotic Anal., Preprint (2023), 1–32. https://doi.org/10.3233/ASY-221823 |
[24] | H. Raad, C. Allery, L. Cherfils, A. Miranville, R. Guillevin, Optimal control of therapies on a tumor growth model, submitted for publication. |
[25] |
P. Sonveaux, F. Végran, T. Schroeder, M. C. Wergin, J. Verrax, Targeting lactate-fueled respiration selectively kills hypoxix tumor cells in mice, J. Clin. Invest., 118 (2008), 3930–3942. https://doi.org/10.1172/JCI36843 doi: 10.1172/JCI36843
![]() |
[26] |
K. R. Swanson, E. C. Alvord, J. D. Murray, Quantifying efficacy of chemotherapy of brain tumors with homogeneous and heterogeneous drug delivery, Acta Biotheor., 50 (2002), 223–237. https://doi.org/10.1023/A:1022644031905 doi: 10.1023/A:1022644031905
![]() |
[27] |
K. R. Swanson, R. C. Rostornily, E. C. Alvord, A mathematical modelling tool for predicting survival of individual patients following resection of glioblastoma: a proof of principle, Br. J. Cancer, 98 (2007), 113–119. https://doi.org/10.1038/sj.bjc.6604125 doi: 10.1038/sj.bjc.6604125
![]() |
[28] |
R. Stupp, W. P. Mason, M. J. van den Bent, M. Weller, B. Fisher, M. J. B. Taphoorn, et al., Radiotherapy plus Concomitant and Adjuvant Temozolomide for Glioblastoma, The New England journal of Medecine, 352 (2005), 987–996. https://doi.org/10.1056/NEJMoa043330 doi: 10.1056/NEJMoa043330
![]() |
1. | Zholaman Bektemessov, Laurence Cherfils, Cyrille Allery, Julien Berger, Elisa Serafini, Eleonora Dondossola, Stefano Casarin, On a data-driven mathematical model for prostate cancer bone metastasis, 2024, 9, 2473-6988, 34785, 10.3934/math.20241656 |
Grade | Protocol | Only chemotherapy | Concomitant therapy | Continuous therapy |
3 | Stupp 1 | v∗=127.1 | ω∗=1, v∗=124.1 | ω∗=0.44, v∗=108.7 |
3 | Stupp 5 | v∗=17.8 | ω∗=1, v∗=16.6 | ω∗=0.43, v∗=15.8 |
3 | Third | v∗=9.17 | ω∗=1, v∗=8.14 | ω∗=0.43, v∗=8.19 |
4 | Stupp 5 | v∗=21.9 | ω∗=0.99, v∗=18.6 | ω∗=0.48, v∗=14.4 |
4 | Third | v∗=11.15 | ω∗=1, v∗=8.02 | ω∗=0.49, v∗=7.46 |
Grade | Protocol | Only chemotherapy | Concomitant therapy | Continuous therapy |
3 | Stupp 1 | v∗=127.1 | ω∗=1, v∗=124.1 | ω∗=0.44, v∗=108.7 |
3 | Stupp 5 | v∗=17.8 | ω∗=1, v∗=16.6 | ω∗=0.43, v∗=15.8 |
3 | Third | v∗=9.17 | ω∗=1, v∗=8.14 | ω∗=0.43, v∗=8.19 |
4 | Stupp 5 | v∗=21.9 | ω∗=0.99, v∗=18.6 | ω∗=0.48, v∗=14.4 |
4 | Third | v∗=11.15 | ω∗=1, v∗=8.02 | ω∗=0.49, v∗=7.46 |