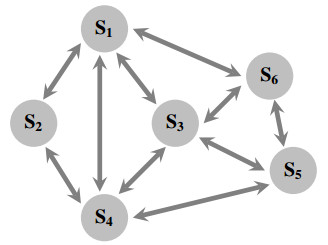
By exploiting the concept of row partitioning, we propose an efficient variant of the greedy block Kaczmarz algorithm for solving consistent large linear systems. The number of blocks is determined a priori through numerical experiments. The new algorithm works with a reduced linear system, which dramatically diminishes the computational overhead per iteration. The theoretical result validates that this method converges to the unique least-norm solution of the linear system. The effectiveness of the proposed algorithm is also justified by comparing it with some block Kaczmarz algorithms in extensive numerical experiments.
Citation: Ke Zhang, Hong-Yan Yin, Xiang-Long Jiang. An efficient variant of the greedy block Kaczmarz algorithm for solving large linear systems[J]. AIMS Mathematics, 2024, 9(1): 2473-2499. doi: 10.3934/math.2024122
[1] | Saravanan Shanmugam, Mohamed Rhaima, Hamza Ghoudi . Exponential synchronization analysis for complex dynamical networks with hybrid delays and uncertainties under given control parameters. AIMS Mathematics, 2023, 8(12): 28976-29007. doi: 10.3934/math.20231484 |
[2] | Arthit Hongsri, Wajaree Weera, Thongchai Botmart, Prem Junsawang . Novel non-fragile extended dissipative synchronization of T-S fuzzy complex dynamical networks with interval hybrid coupling delays. AIMS Mathematics, 2023, 8(12): 28601-28627. doi: 10.3934/math.20231464 |
[3] | Changping Dai, Weiyuan Ma, Ling Guo . Synchronization of generalized fractional complex networks with partial subchannel losses. AIMS Mathematics, 2024, 9(3): 7063-7083. doi: 10.3934/math.2024344 |
[4] | Pratap Anbalagan, Evren Hincal, Raja Ramachandran, Dumitru Baleanu, Jinde Cao, Chuangxia Huang, Michal Niezabitowski . Delay-coupled fractional order complex Cohen-Grossberg neural networks under parameter uncertainty: Synchronization stability criteria. AIMS Mathematics, 2021, 6(3): 2844-2873. doi: 10.3934/math.2021172 |
[5] | N. Jayanthi, R. Santhakumari, Grienggrai Rajchakit, Nattakan Boonsatit, Anuwat Jirawattanapanit . Cluster synchronization of coupled complex-valued neural networks with leakage and time-varying delays in finite-time. AIMS Mathematics, 2023, 8(1): 2018-2043. doi: 10.3934/math.2023104 |
[6] | Zhifeng Lu, Fei Wang, Yujuan Tian, Yaping Li . Lag synchronization of complex-valued interval neural networks via distributed delayed impulsive control. AIMS Mathematics, 2023, 8(3): 5502-5521. doi: 10.3934/math.2023277 |
[7] | Shuang Li, Xiao-mei Wang, Hong-ying Qin, Shou-ming Zhong . Synchronization criteria for neutral-type quaternion-valued neural networks with mixed delays. AIMS Mathematics, 2021, 6(8): 8044-8063. doi: 10.3934/math.2021467 |
[8] | Li Liu, Yinfang Song, Hong Yu, Gang Zhang . Almost sure exponential synchronization of multilayer complex networks with Markovian switching via aperiodically intermittent discrete observa- tion noise. AIMS Mathematics, 2024, 9(10): 28828-28849. doi: 10.3934/math.20241399 |
[9] | Jingyang Ran, Tiecheng Zhang . Fixed-time synchronization control of fuzzy inertial neural networks with mismatched parameters and structures. AIMS Mathematics, 2024, 9(11): 31721-31739. doi: 10.3934/math.20241525 |
[10] | Rongjie Yu, Hengguo Yu, Min Zhao . Steady states and spatiotemporal dynamics of a diffusive predator-prey system with predator harvesting. AIMS Mathematics, 2024, 9(9): 24058-24088. doi: 10.3934/math.20241170 |
By exploiting the concept of row partitioning, we propose an efficient variant of the greedy block Kaczmarz algorithm for solving consistent large linear systems. The number of blocks is determined a priori through numerical experiments. The new algorithm works with a reduced linear system, which dramatically diminishes the computational overhead per iteration. The theoretical result validates that this method converges to the unique least-norm solution of the linear system. The effectiveness of the proposed algorithm is also justified by comparing it with some block Kaczmarz algorithms in extensive numerical experiments.
It is now widely admitted by the scientific community that the increase of the human activity over the last century exerts a high pressure on the equilibrium of ecological systems, which can account for a major defaunation and a fast loss of biodiversity, often qualified as the "sixth extinction" [7,12]. One of the main causes of this biological crisis is the degradation of the ecological environments of wildlife, which takes various forms, in the forefront of which are deforestation and habitat fragmentation [8,10,13]. Facing the challenge of restoring biodiversity, while maintaining human activity at a reasonable level, a carefully considered solution consists in the implementation of ecological corridors between each component of the fragmented environment, so as to increase the connectivity of natural habitats and to avoid local extinction of several wildlife species. For example, the restoration of aquatic ecosystems by maintaining hydrological connectivity with riparian corridors is studied in [15]; different complexity and connectivity patterns are investigated at multiple scales in [21].
In this paper, our aim is precisely to propose an original mathematical model, in order to study these ecological concerns. Therefore, we consider a network of ecological systems, which is intended to model the complex dynamics of trophic chains in a degraded area. The complex network structure reproduces the heterogeneous natural environment, which is perturbed by fragmentation, by coupling several patches on which interacting wild species are living. On each patch, the ecological inter-species dynamics are modeled by a Lotka-Volterra predator-prey model with Holling type II functional response, which is able to describe several biological dynamics, such as extinction, coexistence or ecological cycles [9,17,19] (see also e.g. [3,18,23,24] for other Lotka-Volterra type models). Next, the complex network is constructed so that each patch can admit its own dynamic. In this way, the local components of the network can for instance exhibit an extinction equilibrium on some places, whereas other places can present cycles. Furthermore, migrations of biological individuals in space, between each component of the fragmented environment, are taken into account by coupling the patches of the network, as schematized in Figure 1, where the disks model the patches of the fragmented habitat, and the arrows model the ecological corridors between these patches.
With this mathematical model in hand, we analyze the effect of the couplings on the local dynamics of each patch. In particular, we investigate the possibility to modify a local dynamic of extinction of the species, by increasing the couplings with patches on which persistence, with or without oscillations, is ensured. More generally, we search for sufficient conditions of synchronization of the local dynamics, under a variation of the couplings, which roughly means that a unique local dynamic is imposed on each patch. On this point, we establish a novel theorem of near-synchronization, which guarantees that the complex network remains in a neighborhood of a synchronization state, provided the coupling strength is strong enough, even if the local behaviors are non-identical.
Our choice to model the dynamics of interacting species living in a fragmented environment by a complex network structure follows a line of recent works. Indeed, synchronization in complex networks has been studied in a great number of papers, for various real-world applications, among them coupled oscillators, networks of chemical reactions, neural networks or meta-populations models (see for instance [1,2,5,14] and the references therein). Recently, complex networks of Lotka-Volterra models have been studied in [6]; however, the sufficient conditions of synchronization which have been established in this paper, correspond only to the particular case of identical dynamics. This state of the art highlights the main contributions of the present paper: it is the first time, at our knowledge, that a near-synchronization result for complex networks of non-identical systems is established at a theoretical level.
Our paper is organized as follows. In the next Section, we show how to construct a complex network of predator-prey systems, stemming from a Lotka-Volterra model embedded with a Holling type II functional response. In Section 3, we establish our main theoretical result, with Theorem 2, which establishes sufficient conditions of near-synchronization in a network of non-identical systems. Finally, in Section 4, we illustrate our theoretical statements by relevant numerical simulations.
In this section, we present the construction of a complex network of Lotka-Volterra systems, which describes the dynamics of interacting species living in a fragmented environment.
Let us consider a biological environment in which two species interact. We assume that the densities of the species are determined by a predator-prey model of Lotka-Volterra type, which can be written by:
{˙x=rx(1−x)−cxyα+x,˙y=−dy+cxyα+x. | (2.1) |
Here, x and y denote the prey and predator density, respectively; ˙x and ˙y denote their derivatives with respect to the time variable t. The parameters r, c, d and α are positive coefficients; r is the birth rate of the preys, d is the mortality rate of predators, and c, α determine the non-linear interaction between preys and predators. As mentioned in our introduction, the dynamics of the predator-prey system (2.1) have been widely studied (see for instance [11]). Depending on the values of the parameters r, c, d, α, the solutions of system (2.1) can be attracted to a coexistence equilibrium, to an extinction equilibrium or to a limit cycle. The extinction equilibrium is denoted E0=(0,0). Under the parameter conditions c>d and α<c−dd, system (2.1) admits a coexistence equilibrium E1, which implies persistence of each species, given by
E1=(αdc−d,rαc−d(1−αdc−d)). | (2.2) |
System (2.1) also admits the equilibrium E2=(1,0). Let us introduce the critical value α0 given by
α0=c−dc+d. | (2.3) |
It is well-known (see for instance [11], Chapter 3 or [4], Section 3.4.1) that system (2.1) undergoes a Hopf bifurcation at α=α0. For α<α0, a stable limit cycle bifurcates from the persistence equilibrium E1. This Hopf bifurcation is illustrated in Figure 2. Therefore, for α small enough, system (2.1) presents oscillations, which are interpreted as healthy ecological cycles.
Next, we assume that the geographical habitat of the species is perturbed by the anthropic extension, so that it is fragmented in several patches. This fragmentation is likely to alter the equilibrium of the ecological system. In order to model such a fragmented environment, we construct a complex network of predator-prey models as follows.
First, let n>0 denote the number of patches on the fragmented environment. On each patch i∈{1,…,n}, we denote by (xi,yi) the densities of preys and predators respectively. We assume that each patch i∈{1,…,n} can be connected to other patches and we denote by Ni⊂{1,…,n} the set of patches which are connected to patch i. We assume that migrations of biological individuals can occur between two connected patches, at rates σ1 for preys and σ2 for predators. In this way, the dynamics of the fragmented environment are determined by the following complex network:
{˙xi=rixi(1−xi)−cixiyiαi+xi−σ1∑j∈Ni(xi−xj),˙yi=−diyi+cixiyiαi+xi−σ2∑j∈Ni(yi−yj), | (2.4) |
for 1≤i≤n, with σ1≥0 and σ2≥0. Note that the index i ranges over the set {1,…,n}, whereas the subscript j, in the two sums which determine the couplings of the network, ranges of the set Ni of the neighbors of i.
We emphasize that the parameters ri, ci, di, αi can differ from one patch to another, which means that the ecological dynamics are non-identical within the fragmented environment. For instance, some patches could present limit cycles, whereas other patches could exhibit an extinction of both species. Note also that the couplings are symmetric, which means that if the species xi, yi of patch i can move towards some patch j, then the species xj, yj of patch j can conversely move towards patch i.
One remarkable case of fragmented environment is that of a complete graph topology, for which we have Ni={1,…,n}∖{i}; this situation means that each patch is connected to all other patches. At the opposite, if the coupling parameters σ1, σ2 are equal to 0, then no migration of individuals occur in the network.
Let us now introduce some notations. Let X=((x1,y1),…,(xn,yn))⊤∈R2n. For each i∈{1,…,n}, we denote
λi=(ri,ci,di,αi)⊤∈R4,f1(xi,yi,λi)=rixi(1−xi)−cixiyiαi+xi,f2(xi,yi,λi)=−diyi+cixiyiαi+xi,g1(xi,X,σ1)=−σ1∑j∈Ni(xi−xj),g2(yi,X,σ2)=−σ2∑j∈Ni(yi−yj). | (2.5) |
We also denote σ=(σ1,σ2)⊤∈R2 and
Λ=(λ1,…,λn)⊤∈R4n,F(X,Λ)=(f1(x1,y1,λ1),f2(x1,y1,λ1),…,f1(xn,yn,λn),f2(xn,yn,λn))⊤∈R2n,G(X,σ)=(g1(x1,X,σ1),g2(y1,X,σ2),…,g1(xn,X,σ1),g2(yn,X,σ2))⊤∈R2n. | (2.6) |
With these notations, the complex network (2.4) can be written under the following short form
˙X=F(X,Λ)+G(X,σ). | (2.7) |
Our first result guarantees that the complex network (2.7) admits global solutions, whose components are non-negative and bounded.
Theorem 1. Let X0∈(R+)2n. Then the complex network problem determined by equation (2.7) and X(0)=X0 admits a unique global solution X(t,X0) defined on [0,+∞), whose components are non-negative.
Furthermore, the flow induced by equation (2.7) admits a positively invariant region Θ which is compact in (R+)2n.
Before giving the proof of Theorem 1, we recall a Gronwall type inequality, whose proof can be found in [22] for instance.
Lemma 1. Let v denote a continuously differentiable function defined on [0,T], with T>0. Assume that v satisfies
˙v(t)+δv(t)≤γ, |
for all t∈[0,T], with δ>0 and γ>0. Then we have
v(t)≤[v(0)−γδ]e−δt+γδ,t∈[0,T]. |
We continue with the proof of Theorem 1.
Proof of Theorem 1. Let X0∈(R+)2n. Standard results of the theory of differential equations (see for instance [16]) guaranty that the Cauchy problem determined by equation (2.7) and the initial condition X(0)=X0 admits a unique local solution, which we denote X(t,X0), defined on [0,T], with T>0.
Let us now prove that the components of the local solution X(t,X0) are non-negative on [0,T]. First, we recall that the initial condition X0 belongs to (R+)2n. Next, we observe that the equations of system (2.4) can be rewritten
{˙xi=xiϕ1(xi,yi)+σ1∑j∈Nixj,˙yi=yiϕ2(x,i,yi)+σ2∑j∈Niyj, |
with 1≤i≤n and
ϕ1(xi,yi)=xi(1−xi)−ciyiαi+xi−σ1|Ni|,ϕ2(xi,yi)=−diyi+ciyiαi+xi−σ2|Ni|, |
where |Ni| denotes the cardinal of the finite set Ni. Hence, by virtue of Proposition A.17 in [20], the components (xi,yi)1≤i≤n satisfy xi(t)≥0, yi(t)≥0, for t∈[0,T].
Finally, let us prove that the flow induced by equation (2.7) admits a positively invariant region Θ, which is compact in (R+)2n; as a consequence, every local solution X(t,X0) will be global in time. We first remark that for each i∈{1,…,n}, positive coefficients ai, bi can be found such that
ris(1−s)≤ai−bis, |
for all s∈R. Afterwards, we introduce
d0=min1≤i≤ndi,a0=n∑i=1ai,b0=min1≤i≤nbi,c0=min(b0,d0), | (2.8) |
and we denote by P the total population of preys and predators in the complex network:
P(t)=n∑i=1[xi(t)+yi(t)],t∈[0,T]. | (2.9) |
Since the couplings are symmetric, the sum over i∈{1,…,n} of all equations of system (2.4) leads to
˙P(t)=n∑i=1rixi(t)[1−xi(t)]−n∑i=1diyi(t). |
Next, we write
−n∑i=1diyi(t)≤−d0n∑i=1yi(t), |
and analogously
n∑i=1rixi(t)[1−xi(t)]≤n∑i=1[ai−bixi(t)]≤a0−b0n∑i=1xi(t). |
We obtain
˙P(t)+c0P(t)≤a0. |
Applying Lemma 1 leads to
P(t)≤[P(0)−a0c0]e−c0t+a0c0, |
which implies that the region Θ defined by
Θ={X=(xi,yi)1≤i≤n∈(R+)2n;n∑i=1(xi+yi)≤a0c0} | (2.10) |
is a positively invariant and compact region. The proof is complete.
Remark 1. It is worth emphasizing that the bound a0c0 of the positively invariant region Θ defined by (2.10) does not depend on the coupling parameters σ1, σ2. This uniform bound will be of great interest in Section 3 for studying the global dynamics of the complex network (2.7).
Now that the solutions of the complex network (2.7) are proved to be well defined and global in time, we give the definition of synchronization.
Definition 1. Let i,j∈{1,…,n} such that i≠j. We say that the patches i and j of the complex network (2.7) synchronize in Θ if, for any initial condition X0∈Θ, the solution of (2.7) starting from X0 satisfies
limt→+∞(|xi(t)−xj(t)|2+|yi(t)−yj(t)|2)=0. |
We say that the complex network (2.7) synchronizes in Θ if every pair (i,j) of patches synchronizes in Θ.
Remark 2. Note that synchronization can occur in the complex network (2.7) without imposing any particular asymptotic dynamics; for example, the complex network could synchronize towards a global dynamic of extinction, towards a global dynamic of coexistence, or towards a global dynamic of limit cycles (it could even happen that a new dynamic emerges from the complex network structure). We will establish in Section 3 sufficient conditions of synchronization and discover that a complex network of non-identical instances of the predator-prey model (2.1) is likely not to fully synchronize.
In this section, we search for sufficient conditions of synchronization in the complex network (2.7). In a great number of papers, sufficient conditions of synchronization are established in the case of complex networks of identical systems. Here, we investigate the case of non-identical systems. We prove a novel theorem which shows that a complex network of non-identical systems can reach a near-synchronization state. For the sake of simplicity, we assume that the graph underlying the complex network (2.7) is complete, that is, each patch is connected to all other patches; equivalently, we have Ni={1,…,n}∖{i} for 1≤i≤n, where Ni denotes the finite set of patches which are connected to patch i. For all i,j∈{1,…,n}, we introduce the energy functions ui,j defined along the trajectories of the complex network by
ui,j(t)=12[|xi(t)−xj(t)|2+|yi(t)−yj(t)|2], | (3.1) |
and for λi=(ri,di,ci,αi),λj=(rj,dj,cj,αj)∈R4, we denote
‖λi−λj‖∞=max{|ri−rj|,|di−dj|,|ci−cj|,|αi−αj|}. |
The next theorem establishes an estimate of the energy functions ui,j defined by (3.1).
Theorem 2. There exist positive constants η, δ such that, for any initial condition X0∈Θ, the solution of the complex network (2.7), starting from X0, satisfies
˙ui,j(t)≤η‖λi−λj‖∞u1/2i,j(t)+[δ−2(n−1)˜σ]ui,j(t),t>0, | (3.2) |
where ˜σ=min{σ1,σ2}.
Furthermore, the constants η and δ do not depend on the coupling parameters σ1, σ2.
We begin with a technical lemma.
Lemma 2. There exist positive constants kr, 1≤r≤6, such that the functions f1 and f2 defined in (2.5) satisfy
|f1(xi,yi,λi)−f1(xj,yj,λj)|≤k1‖λi−λj‖∞+k2|xi−xj|+k3|yi−yj|,|f2(xi,yi,λi)−f2(xj,yj,λj)|≤k4‖λi−λj‖∞+k5|xi−xj|+k6|yi−yj|, | (3.3) |
for all (xi,yi)1≤i≤n, in the invariant region Θ defined by (2.10) and for all i,j∈{1,…,n}.
Furthermore, the constants kr, 1≤r≤6, do not depend on the coupling parameters σ1, σ2.
Proof of Lemma 2. Since (xi,yi)1≤i≤n belongs to the invariant region Θ defined by (2.10), there exists a positive constant K such that
|xi|≤K,|yi|≤K, |
for all i∈{1,…,n}.
In order to establish the estimates (3.3), we first write
|diyi−djyj|≤|diyi−diyj|+|diyj−djyj|, |
which leads to
|diyi−djyj|≤d+|yi−yj|+K|di−dj|, | (3.4) |
where d+=max1≤i≤ndi. Similarly, we have
|rixi(1−xi)−rjxj(1−xj)|≤|rixi(1−xi)−rixj(1−xj)|+|rixj(1−xj)−rjxj(1−xj)|≤ri|xi(1−xi)−xj(1−xj)|+K(1+K)|ri−rj|. |
Now we write
|xi(1−xi)−xj(1−xj)|≤|xi−xj|+|x2i−x2j|≤|xi−xj|+|xi+xj|×|xi−xj|≤(1+2K)|xi−xj|, |
from which it follows that
|rixi(1−xi)−rjxj(1−xj)|≤r+(1+2K)|xi−xj|+K(1+K)|ri−rj|, | (3.5) |
where r+=max1≤i≤nri. Finally, we compute
|cixiyiαi+xi−cjxjyjαj+xj|≤|(αj+xj)cixiyi−(αi+xi)cjxjyj)(αi+xi)(αj+xj)|≤1αiαj[|αjcixiyi−αicjxjyj|+|cixixjyi−cjxixjyj|]. |
For the first term in the brackets, we write
|αjcixiyi−αicjxjyj|≤|αjcixiyi−αjcixjyj|+|αjcixjyj−αicjxjyj|≤αjci|xiyi−xjyj|+K2|αjci−αicj|≤αjci|xiyi−xiyj|+αjci|xiyj−xjyj|+K2|αjci−αicj|≤αjciK(|yi−yj|+|xi−xj|)+K2αj|ci−cj|+K2cj|αi−αj|. |
For the second term in the brackets, we have
|cixixjyi−cjxixjyj|≤|cixixjyi−cixixjyj|+|cixixjyj−cjxixjyj|≤ciK2|yi−yj|+K3|ci−cj|, |
which leads to
|cixiyiαi+xi−cjxjyjαj+xj|≤α+c+K(α−)2|xi−xj|+a+c+K+c+K2(α−)2|yi−yj|+K2α++K3(α−)2|ci−cj|+c+K2(α−)2|αi−αj|, | (3.6) |
where α+=max1≤i≤nαi, c+=max1≤i≤nci and α−=min1≤i≤nαi.
Gathering inequalities (3.5) and (3.6) leads to
|f1(xi,yi,λi)−f1(xj,yj,λj)|≤k1‖λi−λj‖∞+k2|xi−xj|+k3|yi−yj|, |
whereas gathering inequalities (3.4) and (3.6) leads to
|f2(xi,yi,λi)−f2(xj,yj,λj)|≤k4‖λi−λj‖∞+k5|xi−xj|+k6|yi−yj|, |
with positive constants kr, 1≤r≤6, which do not depend on σ neither on the number n of patches in the network. The proof of Lemma 2 is complete.
We continue with the proof of Theorem 2.
Proof of Theorem 2. We compute the derivative of ui,j(t) along a trajectory (xi(t),yi(t))1≤i≤n of the complex network (2.7), starting from an initial condition (xi,0,yi,0)1≤i≤n∈Θ. In order to lighten our notations, we omit the time dependence:
˙ui,j=(xi−xj)(˙xi−˙xj)+(yi−yj)(˙yi−˙yj)=(xi−xj)[f1(xi,yi,λi)−σ1∑k≠i(xi−xk)−f1(xj,yj,λj)+σ1∑k≠j(xj−xk)]+(yi−yj)[f2(xi,yi,λi)−σ2∑k≠i(yi−yk)−f2(xj,yj,λj)+σ2∑k≠j(yj−yk)]. |
Now, we observe that
σ1∑k≠i(xi−xk)−σ1∑k≠j(xj−xk)=σ1(n−1)(xi−xj),σ2∑k≠i(yi−yk)−σ2∑k≠j(yj−yk)=σ2(n−1)(yi−yj). |
By virtue of Lemma 2, we obtain
˙ui,j≤|xi−xj||k1‖λi−λj‖∞+k2|xi−xj|+k3|yi−yj||−σ1(n−1)(xi−xj)2+|yi−yj||k4‖λi−λj‖∞+k5|xi−xj|+k6|yi−yj||−σ2(n−1)(yi−yj)2≤max{k1,k4}‖λi−λj‖∞×[|xi−xj|+|yi−yj|]+[k2−σ1(n−1)]|xi−xj|2+[k6−σ2(n−1)]|yi−yj|2+(k3+k5)|xi−xj|×|yi−yj|. |
Next, we use the standard inequality a+b≤√2×√a2+b2, which is valid for all a,b∈R+, to write
|xi−xj|+|yi−yj|≤√2√|xi−xj|2+|yi−yj|2≤2√ui,j, |
thus we have
max{k1,k4}‖λi−λj‖∞×[|xi−xj|+|yi−yj|]≤η‖λi−λj‖∞u1/2i,j, |
with η=2×max{k1,k4}. In parallel, the Young inequality a×b≤a22+b22 yields
|xi−xj|×|yi−yj|≤|xi−xj|22+|yi−yj|22≤ui,j. |
Finally, we set δ=2max{k2,k6}+k3+k5 and obtain
˙ui,j≤η‖λi−λj‖∞u1/2i,j+[δ−2(n−1)˜σ]ui,j, |
which completes the proof of Theorem 2.
Let us now discuss on some consequences of Theorem 2. We observe that estimate (3.2) is a differential inequality, which implies that for all i,j∈{1,…,n}, the energy function ui,j is bounded by the solution w of the Bernoulli equation
˙w=η‖λi−λj‖∞w1/2+[δ−2(n−1)˜σ]w. | (3.7) |
If λi=λj, the latter differential equation can be simplified, so that estimate (3.2) becomes
˙ui,j(t)≤[δ−2(n−1)˜σ]ui,j(t), |
which directly yields the following corollary.
Corollary 1. Assume that λi=λj for some i,j∈{1,…,n}. Then the patches i and j synchronize if the following condition is fulfilled:
(n−1)˜σ>δ. | (3.8) |
If λi=λj for all i,j∈{1,…,n}, then obviously the whole network synchronizes under condition (3.8). Next, since the constant δ does not depend on the coupling parameters σ1, σ2, the sufficient condition (3.8) can easily be satisfied, provided the number n of patches in the network is sufficiently large, or provided the minimum coupling strength ˜σ=min{σ1,σ2} is sufficiently large.
From the ecological point of view, increasing the number n of patches in the network would correspond to a worse fragmentation of the habitat, which is not a reasonable strategy for our purposes. However, increasing the minimum coupling strength ˜σ can be realized by providing wider ecological corridors.
The non trivial case of Theorem 2 corresponds to a complex network of non-identical patches, for which we have λi≠λj for at least one pair (i,j)∈{1,…,n}2. In that case, the synchronization state {(xi,yi)=(xj,yj)} is likely to present a soft loss of stability. Indeed, it is well-known that the solution w of the Bernoulli equation (3.7) converges towards a positive limit given by
limt→+∞w(t)=(η‖λi−λj‖∞δ−(n−1)˜σ)2, |
provided w(0)>0. We obtain the following corollary.
Corollary 2. The energy function ui,j defined by (3.1), along with the solution of the complex network (2.7) starting, from X0∈Θ, satisfies
0≤lim supui,j(t)≤(η‖λi−λj‖∞δ−(n−1)˜σ)2. | (3.9) |
Finally, since the constants η and δ do not depend on the coupling parameters σ1, σ2, then a sufficiently large value of the minimum coupling strength ˜σ ensures that any neighborhood of the synchronization state {(xi,yi)=(xj,yj)} can be reached, which justifies the expression near-synchronization. This remark can be formalized by the following definition.
Definition 2. Let i,j∈{1,…,n} such that i≠j. We say that the patches i and j of the complex network (2.7) nearly synchronize in Θ with respect to ˜σ if, for any initial condition X0∈Θ, and for any ε>0, the solution of (2.7) starting from X0 satisfies
0≤limt→+∞(|xi(t)−xj(t)|2+|yi(t)−yj(t)|2)<ε, |
for ˜σ sufficiently large.
We say that the complex network (2.7) nearly synchronizes in Θ if every pair (i,j) of patches nearly synchronizes in Θ.
The discussion above can now be formulated as follows.
Corollary 3. The complex network (2.7) nearly synchronizes in Θ with respect to the minimum coupling strength ˜σ.
The latter corollary means that a sufficiently large minimal coupling strength ˜σ ensures that the dynamics of each patch of the complex network (2.4) will be almost identical. Furthermore, by virtue of (3.9), if the minimal coupling strength ˜σ increases, or if the number of patches n increases, then the near-synchronization state is getting closer to a synchronization state. In other words, ˜σ and n can be viewed as parameters to control the level of near-synchronization in the network.
In this section, we provide two examples that illustrate the near-synchronization pattern of a complex network, under the effect of the couplings. We consider networks with two and ten patches, with different coupling strengths, so as to highlight various emergent dynamics. For the sake of simplicity, we assume that σ1=σ2 and write σ instead of σ1, σ2. Our complete computation codes are provided in the Appendix.
We start by showing the effect of the coupling in a simple two-patches network. Although it may appear simple, the case of a two-patches network is fundamental, since it models a natural habitat which is divided by a single obstacle. Notably, the construction of a single road crossing a forest ecosystem exerts a high perturbation on the biodiversity hosted by this environment. Similarly, the establishment of a single river barrier or of a single maritime dyke profoundly modifies the behavior of a water hosted ecosystem.
Therefore, we consider the system given by
{˙x1=r1x1(1−x1)−c1x1y1α1+x1−σ(x1−x2),˙y1=−d1y1+c1x1y1α1+x1−σ(y1−y2),˙x2=r2x2(1−x2)−c2x2y2α2+x2−σ(x2−x1),˙y2=−d2y2+c2x2y2α2+x2−σ(y2−y1), | (4.1) |
with the parameter values from Table 1 and σ>0. On patch 1, the parameters are chosen so that the system converges to a coexistence steady state (α=0.5) or to a limit cycle (α=0.05): the birth rate r1 of preys and the death rate d1 of predators are of the same order; in both cases, extinction is avoided. On patch 2, the parameters are chosen so that the system converges to the extinction state (it suffices to decrease the birth rate of preys: r1=0.2).
Patch 1 | Patch 2 | |||
Parameter | Value | Parameter | Value | |
r1 | 1 | r2 | 0.2 | |
d1 | 1 | d2 | 1 | |
c1 | 2 | c2 | 3 | |
α1 | 0.5 or 0.05 | α2 | 0.05 |
The initial conditions are defined by
(x1(0),y1(0))=(0.3,0.2),(x2(0),y2(0))=(0.5,0.3). |
We show in Figure 3 the corresponding orbits of the two-patches complex network (4.1), in absence of coupling (σ=0), and in Figure 4 the orbits for a positive coupling strength (σ=0.3); two cases are investigated: in the first case, we have α1=0.5, whereas in the second case, we have α1=0.05. Let us discuss the numerical results in regard of Theorem 2.
For σ=0 and α1=0.5 (Figure 3(a)), the orbit (x1,y1) of the first patch is attracted to a persistence equilibrium, while the orbit (x2,y2) of the second patch is attracted to the extinction equilibrium. For σ=0 and α1=0.05 (Figure 3(b)), the orbit of the second patch is the same, but the orbit (x1,y1) of the first patch is now attracted to a limit cycle. We can predict, by virtue of Theorem 2, that the two-patches network will present a near-synchronization dynamic if the coupling strength σ is sufficiently large. However, we aim to describe the common dynamic which is reached under this near-synchronization pattern. Indeed, for σ=0.3, Figure 4 shows that the near-synchronization can hide various emergent dynamics that might occur. For σ=0.3 and α1=0.5 (Figure 4(a)), the two patches nearly synchronize towards a persistence equilibrium, whereas for σ=0.3 and α1=0.05 (Figure 4(b)), the two patches nearly synchronize towards a limit cycle. In both cases, the dynamics are obviously modified: the level of persistence is decreased on patch 1 for α1=0.5 (Figure 4(a)), as well as the amplitude of the oscillations on patch 1 for α1=0.05 (Figure 4(b)); however, the extinction on patch 2 is avoided. Overall, these results that show the good health of the ecosystem (persistence or ecological oscillations) can be recovered by the setting of a strong connection between the patches of the fragmented habitat.
Finally, we aim to experiment an increase of the number n of patches in the network. Therefore, we consider a ten-patches network with a complete graph topology; we choose randomly a set of parameters for each patch, such that
0≤ri≤1,0≤di≤1,0≤ci≤3,0.01≤αi≤0.41, |
for each i∈{1,2,…,10}; the initial conditions are also chosen randomly in the interval [0.1,0.6]. In this way, the patches of the complex network exhibit various local dynamics in absence of coupling. The corresponding orbits are show in Figure 5; the corresponding time series are also provided in Figure 6. For instance, the orbit (x3,y3) of patch 3 is attracted to a limit cycle; the orbit (x4,y4) of patch 4 converges to the equilibrium E2(1,0) (persistence of the preys and extinction of the predators); the orbit (x6,y6) of patch 6 converges to the extinction equilibrium.
We depict in Figure 7 the orbits of the same ten-patches complex network, with a relatively large coupling strength (σ=1); the corresponding time series are again provided in Figure 8. According to Theorem 2, we observe that the orbits nearly synchronize. The coupling strength is large enough to ensure that the near-synchronization is very closed to a synchronization state. Furthermore, the near-synchronization is characterized by a limit cycle; the amplitude of that limit cycle can slightly vary from one patch to another (for instance, y7 is less than 0.7, while y8 reaches 0.8).
From the ecological point of view, these results show again that healthy biological oscillations can be recovered by the setting of numerous and efficient connections between the patches of a fragmented environment.
In this paper, we proposed a complex network to model a heterogeneous geographical habitat of species which is perturbed by an anthropic extension, being fragmented in several patches, where the fragmentation is likely to alter the equilibrium of the ecological system. The complex network was constructed by coupling several patches on which interacting wild species are living and where, for each patch, the ecological inter-species dynamics were modeled by a Lotka-Volterra predator-prey model with Holling type II functional response. An important feature of the complex network is that each patch can admit its own dynamic and migrations of biological individuals in space, between each component of the fragmented environment, are taken into account by coupling the patches of the network. We proved new sufficient conditions for the near-synchronization of the complex network, which guarantees that the complex network remains in a neighborhood of a synchronization state, provided the coupling strength is strong enough, even if the local behaviors are non-identical. This result allows us to modify the local dynamic of extinction of the species, by increasing the couplings with patches on which persistence, with or without oscillations, is ensured.
When proving the sufficient conditions of synchronization, we discovered that a complex network of non-identical instances of the predator-prey model (2.1) is likely not to fully synchronize. This feature motivates the setting of an optimal control problem, so as to exert a command on the dynamics of the complex network (2.7) and to reach a synchronization state, even in the case of non-identical patches. As future work, we intend to apply optimal control theory to remedy the default of synchronization, where the coupling strengths will be represented by external controls acting on the dynamics of the network.
Another interesting research perspective corresponds to the fact that the dispersion of the species from one patch to another could be modeled at a refined level by adding time delays in the coupling terms; in particular, it will be natural to investigate the impact of time delays on the near synchronization dynamic that we have established in the present paper.
This work was partially supported by Portuguese funds through CIDMA, The Center for Research and Development in Mathematics and Applications of University of Aveiro, and the Portuguese Foundation for Science and Technology (FCT–Fundação para a Ciência e a Tecnologia), within project UIDB/04106/2020. Silva was also supported by the FCT Researcher Program CEEC Individual 2018 with reference CEECIND/00564/2018.
All authors declare no conflicts of interest in this paper.
In this appendix, we provide the computation codes of our numerical simulations. These codes are written in Python3 and require the scientific libraries matplotlib, numpy and scipy.
Computation code for a two-patches network
#!/usr/bin/env python3 # Scientific libraries from matplotlib import pyplot as plt import numpy as np from scipy.integrate import odeint from random import random # Parameters r1 = 1; d1 = 1; c1 = 2; alpha1 = 0.05 # or 0.5 sigma = 0.3 # or 0 r2 = 0.2; d2 = 1; c2 = 3; alpha2 = 0.05 def lotka(X, t): x1, y1, x2, y2 = X dx1 = r1*x1*(1-x1) - c1*x1*y1/(alpha1+x1) - sigma*(x1-x2) dy1 = -d1*y1 + c1*x1*y1/(alpha1+x1) - sigma*(y1-y2) dx2 = r2*x2*(1-x2) - c2*x2*y2/(alpha2+x2) + sigma*(x1-x2) dy2 = -d2*y2 + c2*x2*y2/(alpha2+x2) + sigma*(y1-y2) return [dx1, dy1, dx2, dy2] # Phase portrait time = np.arange(0, 50, 0.01) plt.figure() x10 = 0.3; y10 = 0.2; x20 = 0.5; y20 = 0.3 orbit = odeint(lotka, [x10, y10, x20, y20], time) x1, y1, x2, y2 = orbit.T plt.plot(x1, y1, 'b', lw = 0.5) plt.plot(x2, y2, 'r', lw = 1) plt.show() |
Computation code for a ten-patches network
#!/usr/bin/env python3 # Scientific libraries from matplotlib import pyplot as plt import numpy as np from scipy.integrate import odeint from random import random # Parameters r1 = random(); d1 = random(); c1 = 3*random(); alpha1 = 0.01 + 0.4*random() r2 = random(); d2 = random(); c2 = 3*random(); alpha2 = 0.01 + 0.4*random() r3 = random(); d3 = random(); c3 = 3*random(); alpha3 = 0.01 + 0.4*random() r4 = random(); d4 = random(); c4 = 3*random(); alpha4 = 0.01 + 0.4*random() r5 = random(); d5 = random(); c5 = 3*random(); alpha5 = 0.01 + 0.4*random() r6 = random(); d6 = random(); c6 = 3*random(); alpha6 = 0.01 + 0.4*random() r7 = random(); d7 = random(); c7 = 3*random(); alpha7 = 0.01 + 0.4*random() r8 = random(); d8 = random(); c8 = 3*random(); alpha8 = 0.01 + 0.4*random() r9 = random(); d9 = random(); c9 = 3*random(); alpha9 = 0.01 + 0.4*random() r10 = random(); d10 = random(); c10 = 3*random(); alpha10 = 0.01 + 0.4*random() def lotka(X, t): x1, y1, x2, y2, x3, y3, x4, y4, x5, y5, x6, y6, x7, y7, x8, y8, x9, y9, x10, y10 = X dx1 = r1*x1*(1-x1) - c1*x1*y1/(alpha1+x1) - sigma*(9*x1-x2-x3-x4-x5-x6-x7-x8-x9-x10) dy1 = -d1*y1 + c1*x1*y1/(alpha1+x1) - sigma*(9*y1-y2-y3-y4-y5-y6-y7-y8-y9-y10) dx2 = r2*x2*(1-x2) - c2*x2*y2/(alpha2+x2) - sigma*(9*x2-x1-x3-x4-x5-x6-x7-x8-x9-x10) dy2 = -d2*y2 + c2*x2*y2/(alpha2+x2) - sigma*(9*y2-y1-y3-y4-y5-y6-y7-y8-y9-y10) dx3 = r3*x3*(1-x3) - c3*x3*y3/(alpha3+x3) - sigma*(9*x3-x1-x2-x4-x5-x6-x7-x8-x9-x10) dy3 = -d3*y3 + c3*x3*y3/(alpha3+x3) - sigma*(9*y3-y2-y1-y4-y5-y6-y7-y8-y9-y10) dx4 = r4*x4*(1-x4) - c4*x4*y4/(alpha4+x4) - sigma*(9*x4-x1-x2-x3-x5-x6-x7-x8-x9-x10) dy4 = -d4*y4 + c4*x4*y4/(alpha4+x4) - sigma*(9*y4-y2-y3-y1-y5-y6-y7-y8-y9-y10) dx5 = r5*x5*(1-x5) - c5*x5*y5/(alpha5+x5) - sigma*(9*x5-x2-x3-x4-x1-x6-x7-x8-x9-x10) dy5 = -d5*y5 + c5*x5*y5/(alpha5+x5) - sigma*(9*y5-y2-y3-y4-y1-y6-y7-y8-y9-y10) dx6 = r6*x6*(1-x6) - c6*x6*y6/(alpha6+x6) - sigma*(9*x6-x2-x3-x4-x5-x1-x7-x8-x9-x10) dy6 = -d6*y6 + c6*x6*y6/(alpha6+x6) - sigma*(9*y6-y2-y3-y4-y5-y1-y7-y8-y9-y10) dx7 = r7*x7*(1-x7) - c7*x7*y7/(alpha7+x7) - sigma*(9*x7-x2-x3-x4-x5-x6-x1-x8-x9-x10) dy7 = -d7*y7 + c7*x7*y7/(alpha7+x7) - sigma*(9*y7-y2-y3-y4-y5-y6-y1-y8-y9-y10) dx8 = r8*x8*(1-x8) - c8*x8*y8/(alpha8+x8) - sigma*(9*x8-x2-x3-x4-x5-x6-x7-x1-x9-x10) dy8 = -d8*y8 + c8*x8*y8/(alpha8+x8) - sigma*(9*y8-y2-y3-y4-y5-y6-y7-y1-y9-y10) dx9 = r9*x9*(1-x9) - c9*x9*y9/(alpha9+x9) - sigma*(9*x9-x2-x3-x4-x5-x6-x7-x8-x1-x10) dy9 = -d9*y9 + c9*x9*y9/(alpha9+x9) - sigma*(9*y9-y2-y3-y4-y5-y6-y7-y8-y1-y10) dx10 = r10*x10*(1-x10) - c10*x10*y10/(alpha10+x10) - sigma*(9*x10-x2-x3-x4-x5-x6-x7-x8-x9-x1) dy10 = -d10*y10 + c10*x10*y10/(alpha10+x10) - sigma*(9*y10-y2-y3-y4-y5-y6-y7-y8-y9-y1) return [dx1, dy1, dx2, dy2, dx3, dy3, dx4, dy4, dx5, dy5, dx6, dy6, dx7, dy7, dx8, dy8, dx9, dy9, dx10, dy10] # Phase portrait time = np.arange(0,200, 0.01) plt.figure() X0 = [0.1 + 0.5*random() for k in range(20)] sigma = 0 # or 1 orbit = odeint(lotka, X0, time) x1, y1, x2, y2, x3, y3, x4, y4, x5, y5, x6, y6, x7, y7, x8, y8, x9, y9, x10, y10 = orbit.T plt.plot(x1, y1) plt.plot(x2, y2) plt.plot(x3, y3) plt.plot(x4, y4) plt.plot(x5, y5) plt.plot(x6, y6) plt.plot(x7, y7) plt.plot(x8, y8) plt.plot(x9, y9) plt.plot(x10, y10) plt.show() |
[1] | A. Agaskar, C. Wang, Y. M. Lu, Randomized Kaczmarz algorithms: Exact MSE analysis and optimal sampling probabilities, 2014 IEEE Global Conference on Signal and Information Processing (Global-SIP), 389–393. https://doi.org/10.1109/GlobalSIP.2014.7032145 |
[2] |
Z. Z. Bai, X. G. Liu, On the Meany inequality with applications to convergence analysis of several row-action iteration methods, Numer. Math., 124 (2013), 215–236. https://doi.org/10.1007/s00211-012-0512-6 doi: 10.1007/s00211-012-0512-6
![]() |
[3] |
Z. Z. Bai, L. Wang, On convergence rates of Kaczmarz-type methods with different selection rules of working rows, Appl. Numer. Math., 186 (2023), 289–319. https://doi.org/10.1016/j.apnum.2023.01.013 doi: 10.1016/j.apnum.2023.01.013
![]() |
[4] |
Z. Z. Bai, W. T. Wu, On convergence rate of the randomized Kaczmarz method, Linear Algebra Appl., 553 (2018), 252–269. https://doi.org/10.1016/j.laa.2018.05.009 doi: 10.1016/j.laa.2018.05.009
![]() |
[5] |
Z. Z. Bai, W. T. Wu, On greedy randomized Kaczmarz method for solving large sparse linear systems, SIAM J. Sci. Comput., 40 (2018), A592–A606. https://doi.org/10.1137/17M1137747 doi: 10.1137/17M1137747
![]() |
[6] |
Z. Z. Bai, W. T. Wu, On relaxed greedy randomized Kaczmarz methods for solving large sparse linear systems, Appl. Math. Lett., 83 (2018), 21–26. https://doi.org/10.1016/j.aml.2018.03.008 doi: 10.1016/j.aml.2018.03.008
![]() |
[7] |
Z. Z. Bai, W. T. Wu, Randomized Kaczmarz iteration methods: Algorithmic extensions and convergence theory, Jpn. J. Ind. Appl. Math., 40 (2023), 1421–1443. https://doi.org/10.1007/s13160-023-00586-7 doi: 10.1007/s13160-023-00586-7
![]() |
[8] | E. Bodewig, Bericht über die verschiedenen Methoden zur Lösung eines System linearer Gleichungen mit reellen Koeffizienten, Cons. Naz. Ric., 324 (1948), 441–452. |
[9] |
C. Byrne, A unified treatment of some iterative algorithms in signal processing and image reconstruction, Inverse Probl., 20 (2004), 103–120. https://doi.org/10.1088/0266-5611/20/1/006 doi: 10.1088/0266-5611/20/1/006
![]() |
[10] |
J. Q. Chen, Z. D. Huang, On a fast deterministic block Kaczmarz method for solving large-scale linear systems, Numer. Algorithms, 89 (2022), 1007–1029. https://doi.org/10.1007/s11075-021-01143-4 doi: 10.1007/s11075-021-01143-4
![]() |
[11] |
T. A. Davis, Y. Hu, The university of Florida sparse matrix collection, ACM T. Math. Software, 38 (2011), 1–25. https://doi.org/10.1145/2049662.2049663 doi: 10.1145/2049662.2049663
![]() |
[12] |
K. Du, W. T. Si, X. H. Sun, Randomized extended average block Kaczmarz for solving least squares, SIAM J. Sci. Comput., 42 (2020), A3541–A3559. https://doi.org/10.1137/20M1312629 doi: 10.1137/20M1312629
![]() |
[13] |
Y. C. Eldar, D. Needell, Acceleration of randomized Kaczmarz methods via the Johnson-Lindenstrauss lemma, Numer. Algorithms, 58 (2011), 163–177. https://doi.org/10.1007/s11075-011-9451-z doi: 10.1007/s11075-011-9451-z
![]() |
[14] |
T. Elfving, Block-iterative methods for consistent and inconsistent linear equations, Numer. Math., 35 (1980), 1–12. https://doi.org/10.1007/BF01396365 doi: 10.1007/BF01396365
![]() |
[15] | G. E. Forsythe, Solving linear algebraic equations can be interesting, Bull. Amer. Math. Soc., 59 (1953), 299–329. |
[16] |
R. Gordon, R. Bender, G. T. Herman, Algebraic reconstruction techniques (ART) for three-dimensional electron microscopy and X-ray photography, J. Theoret. Biol., 29 (1970), 471–481. https://doi.org/10.1016/0022-5193(70)90109-8 doi: 10.1016/0022-5193(70)90109-8
![]() |
[17] |
R. M. Gower, D. Molitor, J. Moorman, D. Needell, On adaptive sketch-and-project for solving linear systems, SIAM J. Matrix Anal. A., 42 (2021), 954–989. https://doi.org/10.1137/19M1285846 doi: 10.1137/19M1285846
![]() |
[18] |
R. M. Gower, P. Richtárik, Randomized iterative methods for linear systems, SIAM J. Matrix Anal. A., 36 (2015), 1660–1690. https://doi.org/10.1137/15M1025487 doi: 10.1137/15M1025487
![]() |
[19] |
M. Griebel, P. Oswald, Greedy and randomized versions of the multiplicative Schwarz method, Linear Algebra Appl., 437 (2012), 1596–1610. https://doi.org/10.1016/j.laa.2012.04.052 doi: 10.1016/j.laa.2012.04.052
![]() |
[20] | G. T. Herman, Fundamentals of computerized tomography: Image reconstruction from projections, 2 Eds., Springer, Dordrecht, 2009. https://doi.org/10.1007/978-1-84628-723-7 |
[21] |
X. L. Jiang, K. Zhang, J. F. Yin, Randomized block Kaczmarz methods with k-means clustering for solving large linear systems, J. Comput. Appl. Math., 403 (2022), 113828. https://doi.org/10.1016/j.cam.2021.113828 doi: 10.1016/j.cam.2021.113828
![]() |
[22] | S. Kaczmarz, Angenäherte Auflösung von systemen linearer gleichungen, Bull. Int. Acad. Pol. Sci. Lett. A, 35 (1937), 355–357. |
[23] |
S. P. Kolodziej, M. Aznaveh, M. Bullock, J. David, T. A. Davis, M. Henderson et al., The SuiteSparse matrix collection website interface, J. Open Source Softw., 4 (2019), 1244–1248. https://doi.org/10.21105/joss.01244 doi: 10.21105/joss.01244
![]() |
[24] |
Y. Liu, C. Q. Gu, On greedy randomized block Kaczmarz method for consistent linear systems, Linear Algebra Appl., 616 (2021), 178–200. https://doi.org/10.1016/j.laa.2021.01.024 doi: 10.1016/j.laa.2021.01.024
![]() |
[25] |
C. Q. Miao, W. T. Wu, On greedy randomized average block Kaczmarz method for solving large linear systems, J. Comput. Appl. Math., 413 (2022), 114372. https://doi.org/10.1016/j.cam.2022.114372 doi: 10.1016/j.cam.2022.114372
![]() |
[26] |
I. Necoara, Faster randomized block Kaczmarz algorithms, SIAM J. Matrix Anal. A., 40 (2019), 1425–1452. https://doi.org/10.1137/19M1251643 doi: 10.1137/19M1251643
![]() |
[27] |
D. Needell, J. A. Tropp, Paved with good intentions: Analysis of a randomized block Kaczmarz method, Linear Algebra Appl., 441 (2014), 199–221. https://doi.org/10.1016/j.laa.2012.12.022 doi: 10.1016/j.laa.2012.12.022
![]() |
[28] |
Y. Q. Niu, B. Zheng, A greedy block Kaczmarz algorithm for solving large-scale linear systems, Appl. Math. Lett., 104 (2020), 106294. https://doi.org/10.1016/j.aml.2020.106294 doi: 10.1016/j.aml.2020.106294
![]() |
[29] |
T. Strohmer, R. Vershynin, A randomized Kaczmarz algorithm with exponential convergence, J. Fourier Anal. Appl., 15 (2009), 262–278. https://doi.org/10.1007/s00041-008-9030-4 doi: 10.1007/s00041-008-9030-4
![]() |
[30] | C. Tompkins, Projection methods in calculation of some linear problems, Bull. Amer. Math. Soc., 55 (1949), 520. |
[31] |
L. Wen, F. Yin, Y. M. Liao, G. X. Huang, A greedy average block Kaczmarz method for the large scaled consistent system of linear equations, AIMS Math., 7 (2022), 6792–6806. https://doi.org/10.3934/math.2022378 doi: 10.3934/math.2022378
![]() |
[32] | G. Wu, Q. Chang, Semi-randomized block Kaczmarz methods with simple random sampling for large-scale linear systems, arXiv: 2212.08797, 2023. https://doi.org/10.48550/arXiv.2212.08797 |
[33] |
A. Q. Xiao, J. F. Yin, N. Zheng, On fast greedy block Kaczmarz methods for solving large consistent linear systems, Comput. Appl. Math., 42 (2023), 119. https://doi.org/10.1007/s40314-023-02232-x doi: 10.1007/s40314-023-02232-x
![]() |
[34] |
F. Yin, B. Y. Zhang, G. X. Huang, A partially block randomized extended Kaczmarz method for solving large overdetermined inconsistent linear systems, AIMS Math., 8 (2023), 18512–18527. https://doi.org/10.3934/math.2023941 doi: 10.3934/math.2023941
![]() |
[35] |
K. Zhang, F. T. Li, X. L. Jiang, Multi-step greedy Kaczmarz algorithms with simple random sampling for solving large linear systems, Comput. Appl. Math., 41 (2022), 332. https://doi.org/10.1007/s40314-022-02044-5 doi: 10.1007/s40314-022-02044-5
![]() |
[36] |
Y. Zhang, H. Li, Block sampling Kaczmarz-Motzkin methods for consistent linear systems, Calcolo, 58 (2021), 39. https://doi.org/10.1007/s10092-021-00429-2 doi: 10.1007/s10092-021-00429-2
![]() |
[37] |
Y. Zhang, H. Li, Randomized block subsampling Kaczmarz-Motzkin method, Linear Algebra Appl., 667 (2023), 133–150. https://doi.org/10.1016/j.laa.2023.03.003 doi: 10.1016/j.laa.2023.03.003
![]() |
1. | M A Aziz-Alaoui, Guillaume Cantin, Alexandre Thorel, Synchronization of Turing patterns in complex networks of reaction–diffusion systems set in distinct domains, 2024, 37, 0951-7715, 025011, 10.1088/1361-6544/ad1a48 |
Patch 1 | Patch 2 | |||
Parameter | Value | Parameter | Value | |
r1 | 1 | r2 | 0.2 | |
d1 | 1 | d2 | 1 | |
c1 | 2 | c2 | 3 | |
α1 | 0.5 or 0.05 | α2 | 0.05 |