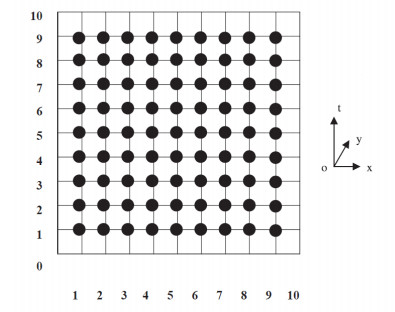
In this article, we introduce an approximation of the rotated five-point difference Crank-Nicolson R(FPCN) approach for treating the second-order two-dimensional (2D) time-fractional diffusion-wave equation (TFDWE) with damping, which is constructed from two separate sets of equations, namely transverse electric and transverse magnetic phases. Such a category of equations can be achieved by altering second-order time derivative in the ordinary diffusion damped wave model by fractional Caputo derivative of order α while 1<α<2. The suggested methodology is developed from the standard five-points difference Crank-Nicolson S(FPCN) scheme by rotating clockwise 45o with respect to the standard knots. Numerical analysis is presented to demonstrate the applicability and feasibility of the R(FPCN) formulation over the S(FPCN) technique. The stability and convergence of the presented methodology are also performed.
Citation: Ajmal Ali, Tayyaba Akram, Azhar Iqbal, Poom Kumam, Thana Sutthibutpong. A numerical approach for 2D time-fractional diffusion damped wave model[J]. AIMS Mathematics, 2023, 8(4): 8249-8273. doi: 10.3934/math.2023416
[1] | Jian-Gen Liu, Jian Zhang . A new approximate method to the time fractional damped Burger equation. AIMS Mathematics, 2023, 8(6): 13317-13324. doi: 10.3934/math.2023674 |
[2] | Krunal B. Kachhia, Jyotindra C. Prajapati . Generalized iterative method for the solution of linear and nonlinear fractional differential equations with composite fractional derivative operator. AIMS Mathematics, 2020, 5(4): 2888-2898. doi: 10.3934/math.2020186 |
[3] | Abdul-Majeed Ayebire, Saroj Sahani, Priyanka, Shelly Arora . Numerical study of soliton behavior of generalised Kuramoto-Sivashinsky type equations with Hermite splines. AIMS Mathematics, 2025, 10(2): 2098-2130. doi: 10.3934/math.2025099 |
[4] | Farman Ali Shah, Kamran, Zareen A Khan, Fatima Azmi, Nabil Mlaiki . A hybrid collocation method for the approximation of 2D time fractional diffusion-wave equation. AIMS Mathematics, 2024, 9(10): 27122-27149. doi: 10.3934/math.20241319 |
[5] | Fouad Mohammad Salama, Faisal Fairag . On numerical solution of two-dimensional variable-order fractional diffusion equation arising in transport phenomena. AIMS Mathematics, 2024, 9(1): 340-370. doi: 10.3934/math.2024020 |
[6] | Jing Li, Linlin Dai, Kamran, Waqas Nazeer . Numerical solution of multi-term time fractional wave diffusion equation using transform based local meshless method and quadrature. AIMS Mathematics, 2020, 5(6): 5813-5838. doi: 10.3934/math.2020373 |
[7] | M. Mossa Al-Sawalha, Khalil Hadi Hakami, Mohammad Alqudah, Qasem M. Tawhari, Hussain Gissy . Novel Laplace-integrated least square methods for solving the fractional nonlinear damped Burgers' equation. AIMS Mathematics, 2025, 10(3): 7099-7126. doi: 10.3934/math.2025324 |
[8] | Adnan Khaliq, Mujeeb ur Rehman . Fixed point theorem combined with variational methods for a class of nonlinear impulsive fractional problems with derivative dependence. AIMS Mathematics, 2021, 6(2): 1943-1953. doi: 10.3934/math.2021118 |
[9] | Din Prathumwan, Thipsuda Khonwai, Narisara Phoochalong, Inthira Chaiya, Kamonchat Trachoo . An improved approximate method for solving two-dimensional time-fractional-order Black-Scholes model: a finite difference approach. AIMS Mathematics, 2024, 9(7): 17205-17233. doi: 10.3934/math.2024836 |
[10] | Changdev P. Jadhav, Tanisha B. Dale, Vaijanath L. Chinchane, Asha B. Nale, Sabri T. M. Thabet, Imed Kedim, Miguel Vivas-Cortez . On solutions of fractional differential equations for the mechanical oscillations by using the Laplace transform. AIMS Mathematics, 2024, 9(11): 32629-32645. doi: 10.3934/math.20241562 |
In this article, we introduce an approximation of the rotated five-point difference Crank-Nicolson R(FPCN) approach for treating the second-order two-dimensional (2D) time-fractional diffusion-wave equation (TFDWE) with damping, which is constructed from two separate sets of equations, namely transverse electric and transverse magnetic phases. Such a category of equations can be achieved by altering second-order time derivative in the ordinary diffusion damped wave model by fractional Caputo derivative of order α while 1<α<2. The suggested methodology is developed from the standard five-points difference Crank-Nicolson S(FPCN) scheme by rotating clockwise 45o with respect to the standard knots. Numerical analysis is presented to demonstrate the applicability and feasibility of the R(FPCN) formulation over the S(FPCN) technique. The stability and convergence of the presented methodology are also performed.
In recent years, the fractional partial differential equations (PDEs) have attracted a growing attention in modeling in various applications in science and technology. The fractional derivative which is simultaneously possesses memory and non-local property can describe different non-linear phenomena more accurately and efficiently in comparison with the integer order derivative. This makes the fractional PDE a powerful tool for modeling complex dynamical systems [43]. Nowadays, fractional calculus is being used to report the non-differentiable problems for various types of fractional PDEs. In this regard, Singh et al. [45] find the non-differentiable solutions of local fractional wave equation in fractal strings by applying Local Fractional Homotopy Perturbation Laplace Transform Method (LFHPLTM). The uniqueness and convergence were also proved by the suggested method. Yang et al. [46] developed a new Boussinesq-type Model in a fractal domain which was based on the formulation of the local fractional derivative. To achieve exact traveling wave solution which can be obtained from the non-differentiable graph, first they convert the local fractional Boussinesq equation into nonlinear local fractional PDE. Fellah et al. [44] reviewed a new concept of the fractional PDE for the sound waves propagation in rigid porous materials. They experienced such a type of expression in which fractional power of frequency is related to the time derivative of fractional order that will describe the behavior of the sound waves. The results show the accuracy and capability. Kumar et al. [47] proposed a numerical algorithm to solve the vehicular fractal traffic flow problem. They solved the problem with the help of the local fractional Homotopy Perturbation Sumudu Transform method and the results were computationally sound for similar kinds of fractional PDE occurring in natural sciences. Besides this, Hajipour et al. [48] suggested a Variable-Order Fractional (VOF) model for the accurate approximate solution of reaction-diffusion equation in which they utilized a weighted shifted Grunwald derivative to solve the fractional part of the time derivative. This discretization technique was superior when compared with other methods in the literature. The variable order fractional calculus is an efficient tool to predict the compression deformation of amorphous glassy polymers. Meng et al. [49] proposed a three-regions- fitting-method and found that the VOF model is very efficient with higher accuracy.
In fractional calculus, the mathematical models are more accurately illustrate the characteristics of the real-world phenomena than the classical calculus, for example, Jajarmi and Baleanu [50] formulated a perfect model for describing the pathological behavior of HIV infection in fractional calculus that can never be investigated asymptomatic behaviors during the modeling with the integer-order derivatives. Baleanu et al. [51] examined their proposed model regarding the poor nutrition in the life cycle of humans with Mittag-Leffler nonsingular kernel in Caputo's fractional sense. The comparative numerical analysis reveals that the model based on the fractional derivative with Mittag-Leffler kernel has a different asymptotic behavior to the classic integer order derivatives. Shamasneh et al.[52] proposed a Local Fractional Entropy (LFE)-based model for kidney images enhancement where the quality of MRI images are unpredictable. They tested the model on poor-quality kidney image and found the excellent results when LFE techniques are applied. Thus, the new aspects of fractional calculus provide us with more stretchy mathematical models that help us to formulate more realistic judgments about real-world problems in a better way.
In many aspects, fractional damped wave equations (FDWEs) have taken priority over the ordinary diffusion wave equations with damping due to the numerous implementations in practical natural occurrences. In the literature, much importance has been received to the solution of FDWEs because of the mathematical concept in various branches of applied sciences [1,2,3,4,5,36,39,40,41]. In several areas of study, it is essential to find the exact or approximate solution of many types of fractional differential equations (FDEs) that will help researchers understand the mechanism and difficulty of the challenge. In the previous era, many investigators, scholars and researchers attempted to formulate the analytical solution to the challenges of various distinct forms of FDEs that were difficult assignments for them. For example, in the solution of time fractional diffusion damped wave equation (TFDWE), Schneider and Wyss [6] utilized Greens' functions and their charateristics in aspects of Fox functions to solve the diffusion wave equation, Gorenflo et al. [7] used a similar technique to solve the fractional diffusion wave equation employing Laplace transformation and found a scale-invariant solution in forms of weight functions. Many other analytical solutions of FDEs can be found in [8,9,10,11,12] utilizing the Laplace transformation, the Fourier transformation, the Green function and Homotopy perturbation techniques, etc.
The majority of the FDEs cannot be determined analytically so there is a need for approximations to find the approximate numerical solution by various iterative techniques such as the finite element approach [13,14,15,16,17], finite difference method [18], spectral approach [19] and collocation techniques [20,21,22,23,24]. Such type of iterative technique reduces the computational complexities of the algorithm. As a result, the following sparse system of linear equations is achieved: Au = b where A is a non-singular matrix that is known, b, and u are a constant vector and the unknown column vector. At this stage, the rotated iterative techniques become more significant in order to solve the discretized linear system compared to the conventional (standard) iterative methods. The basic principle of the rotated iterative scheme is the use of nearly the half of interior nodes of the solution domain and the involvement of these interior mesh points in the iterative process to achieve rapid convergence. The values on the corresponding grid points are explicitly evaluated using the finite difference formula until convergence is attained. In this way, the rotated point iterative scheme reduces total arithmetic operations used and consequently there is will be a reduction in computational complexities, execution of CPU timings (per iteration) and number of iterations of the algorithm. As a result of this concept, numerous researchers have proposed a lot of explicit group iterative techniques for solving the various types of partial differential equations (PDEs). Martins et al. [25], Othman et al. [26], Yousif and Evans [27], Ali and Kew [28], Othman and Abdullah [29], Kew and Ali [30], Evans and Haghighi [31] and Abu Arqub et al. [32,33] contributed their work with most efficient results.
Owing to their good performances to solve integer order derivatives, the efforts are now being created to implement new methodologies to solve fractional PDEs in a better way [42]. Preliminary, Balasim and Ali [34,35] published recently their work on the 2D time-fractional diffusion, advection and cable differential equations in which promising outcomes were observed for the fractional parameter α∈(0,1) when the Caputo time-fractional were combined with skewed approximations for the space fractional derivatives. In this article, we have extended our research for the numerical solution of diffusion damped wave equation of fractional order α, (1<α<2) by applying the rotated finite difference iterative method and detected the proficient results.
Various studies [28,30] have proved the rotated five-point finite difference methods provide better and excellent results than the standard five-point difference ones for the numerical solution of multi-dimensional PDEs. More precise to the rotated Crank-Nicolson method, seldom authors utilized this iterative method for the numerical solution of different types of 2D-PDEs for fast convergence and found capable results. Balasim and Ali [34,35] have shown promising results for the rotated five-point Crank-Nicolson over the standard five-point Crank-Nicolson for solution of 2D subdiffusion PDE of fractional order. Ali and Kew [28] and Kew and Ali [30] applied rotated Crank-Nicolson schemes on two and three-dimensional hyperbolic telegraph PDEs respectively and results were much better and efficient than the standard Crank-Nicolson S(C-N) method. Applying the rotated implicit difference approximation in combination with Caputo's fractional derivative on 2D-FDEs is very simple and straightforward in the case of fractional PDEs. But applying the rotated Crank-Nicolson difference R(C-N) approximation together with Caputo's fractional derivative on 2D fractional superdiffusion type PDEs (like fractional wave diffusion, fractional damped wave-diffusion and hyperbolic telegraph equation etc.) is a very difficult task for the researchers. Initially, Balasim and Ali [34,35] utilized R(C-N) technique for the numerical solution of 2D fractional subdiffusion PDEs, where the Caputo's fractional derivative is utilized to solve the fractional case. The implementation of rotated Crank-Nicolson method together with Caputo's fractional derivative on 2D fractional superdiffusion PDEs is still an open problem for the investigator in this field of research.
The organization of the article is as follows: We shall derive the (3−α) approximation from the Caputo fractional derivative at the point (ui,vj,tk+1/2) and an expression for the standard finite difference S(FPCN) in section 2. The derivation of finite difference expression R(FPCN) will be given in section 3. The stability and convergence of the R(FPCN) technique will be discussed respectively in sections 4 and 5. The numerical experiments will be performed in section 6, followed by computational complexity. In section 7, we present some final remarks about our derived results in the conclusion.
Consider the second-order 2D TFDWE as:
∂αz(u,v,t)∂tα+∂z(u,v,t)∂t=∂2z(u,v,t)∂u2+∂2z(u,v,t)∂v2+f(u,v,t) | (2.1) |
where, 1<α<2, with initial conditions
z(u,v,0)=g(u,v),zt(u,v,0)=0, |
and boundary conditions
z(0,v,t)=f1(v,t),z(L,v,t)=f2(v,t), |
z(u,0,t)=g3(u,t),z(u,L,t)=g4(u,t), |
where Ω={(u,v,t)/0<u,v<L,0≤t≤T} and f is the general source term. In Eq (2.1), the term ∂z(u,v,t)∂t is called the damped factor which usually leads initial directionality propagation of wave in a string. The motion of wave become completely random if the damped factor become zero and wave motion behavior like fractional wave-diffusion equation. We utilize the following expression of Caputo fractional derivative to solve the fractional part of Eq (2.1)
∂αz(u,v,t)∂tα=1Γ(p−α)∫t0∂zp(u,v,ζ)∂ζpdζ(t−ζ)α+1−p,p−1<α<p. | (2.2) |
Define tk=kτ,k=1,2,⋯,l, ui=ihu,i=1,2,⋯,m and vj=jhv,j=1,2,⋯,n where, τ=Tl, hu=Lm and hv=Ln. The knots in the interval are defined by (iu,jv,kτ)=(ui,vj,tk), where
(i,j,k)=(0,0,0),...,(m,n,l). |
Let Zki,j, and zki,j be exact and the approximate solution of Eq (2.1), take f(ui,vj,tk) as fki,j. We employ S(C-N) technique which is consistently based on space and time, and the Caputo fractional derivative of order α, which correctly handles initial value problems for the solution of Eq (2.1).
The second order differential operator is discretized as follows,
∂2z∂t2|ui,vj,tk=zk+1i,j−2zki,j+zk−1i,jτ2. |
Initially, to derive the required expression for Caputo time-fractional derivative ∂αz(u,v,t)∂tα, (1<α<2) at knot (ui,vj,tk+1) for Eq (2.1), consider the Eq (2.2) for p=2, we have
∂αz(ui,vj,tk+1)∂tα=1Γ(2−α)∫tk+10∂2z(ui,vj,ζ)∂ζ2dζ(tk+1−ζ)α−1=1Γ(2−α)k∑s=0∫(s+1)τsτ∂2z(ui,vj,ζ)∂ζdζ(tk+1−ζ)α−1+Rk+1τ=1Γ(2−α)k∑s=0∫(s+1)τsτ[zs+1i,j−2zsi,j+zs−1i,jτ2]dζ(tk+1−ζ)α−1+Rk+1τ=1Γ(2−α)k∑s=0[zs+1i,j−2zsi,j+zs−1i,jτ2]∫(k−s+1)τ(k−s)τ1ξα−1dξ+Rk+1τ=1Γ(2−α)k∑s=0[zk−s+1i,j−2zk−si,j+zk−s−1i,jτ2]∫(s+1)τsτ1ξα−1dξ+Rk+1τ=τ2−αΓ(2−α)k∑s=0[zk−s+1i,j−2zk−si,j+zk−s−1i,jτ2](s+1)2−α−s2−α2−α+Rk+1τ. |
Hence,
∂αz(ui,vj,tk+1)∂tα=τ−αΓ(3−α)k∑s=0bs[zk−s+1i,j−2zk−si,j+zk−s−1i,j]+Rk+1τ, | (2.3) |
where, bs=(s+1)2−α−s2−α,s=0,1,2,⋯,k and ξ=(tk+1−ζ).
In particular, for s=0, we have
z−1i,j=z1i,j−2τzt(ui,vj,0). |
Next, we find the truncation error Rk+1τ of above iterative scheme with the help of Taylor's series expansion, since
z(ui,vj,ts+1)−2z(ui,vj,ts)+z(ui,vj,ts−1)τ2=∂2z(ui,vj,ts)∂t2+τ212∂4z(ui,vj,ts)∂t4+O(τ4). |
The truncation error Rk+1τ evaluated as,
Rk+1τ=1Γ(2−α)k∑s=0∫(s+1)τsτ(tk+1−ζ)1−α[∂2z(ui,vj,ζ)∂ζ2−∂2z(ui,vj,ts)∂t2−τ212∂4z(ui,vj,ts)∂t4+O(τ4)]dζ=1Γ(2−α)k∑s=0∫(s+1)τsτ(tk+1−ζ)1−α[(ζ−ts)∂3z(ui,vj,ts)∂t3+((ζ−ts)22−τ212)∂4z(ui,vj,ts)∂t4+O(τ4)]dζΓ(2−α)k∑s=0∫(s+1)τsτ(tk+1−ζ)1−α[(ζ−ts)∂3z(ui,vj,ts)∂t3+O(τ2)]dζ. |
Taking the absolute value over the truncation error Rk+1τ, we have
|Rk+1τ|≤CMτΓ(2−α)k∑s=0∫(s+1)τsτ(tk+1−ζ)1−αdζ+O(τ2)=CMT2−ατΓ(3−α)+O(τ2), |
where, C is a constant and M is the upper bound of |∂3z(ui,vj,tk)∂t3|. Therefore,
Rk+1τ≤CT2−αΓ(3−α)maxo≤t≤T|∂3(ui,vj,tk)∂t3|τ+O(τ2). |
Therefore, the discrete form of time-fractional derivative ∂αz(u,v,t)∂tα at the knot (ui,vj,tk+1) is
∂αz(ui,vj,tk+1)∂tα=τ−αΓ(3−α)k∑s=0bs[zk−s+1i,j−2zk−si,j+zk−s−1i,j]+Rk+11, |
where, |Rk+11|≤Cτ.
Similarly, the discrete form of time-fractional derivative ∂αz(u,v,t)∂tα at (ui,vj,tk+1/2) is
∂αz(ui,vj,tk+1/2)∂tα=τ−αΓ(3−α)k∑s=0bs[zk−s+1i,j−2zk−si,j+zk−s−1i,j]+Rk+1/22, |
where, |Rk+1/22|≤Cτ3−α.
By utilizing Crank-Nicolson difference technique for the space derivatives, Caputo time-fractional derivative from Eq (2.3) and central difference approximation for first order differential operator leads Eq (2.1) to the following expression,
zk+1i,j−2zki,j+zk−1i,j+k∑s=1bs(zk−s+1i,j−2zk−si,j+zk−s−1i,j)+m1(zk+1i,j−zk−1i,j)=r12(zk+1i−1,j−2zk+1i,j+zk+1i+1,j+zki−1,j−2zki,j+zki+1,j)+r22(zk+1i,j−1−2zk+1i,j+zk+1i,j+1+zki,j−1−2zki,j+zki,j−1)+mofk+1/2i,j, |
for i=1,2,⋯,m−1, j=1,2,⋯,n−1 and k=0,1,2,⋯,l, where
mo=ταΓ(3−α),r1=mo(hu)2,r2=mo(hv)2andm1=mo2τ. |
Rewrite the above equation as,
zk+1i,j=1(1+m1+r1+r2)[r12(zk+1i−1,j+zk+1i+1,j)+r22(zk+1i,j−1+zk+1i,j+1)+r12(zki−1,j+zki+1,j)+r22(zki,j−1+zki,j+1)+(2−r1−r2)zki,j+(m1−1)zk−1i,j−k∑s=1bs(zk−s+1i,j−2zk−si,j+zk−s−1i,j)+mofk+1/2i,j], | (2.4) |
for i=1,2,⋯,m−1, j=1,2,⋯,n−1 and k=0,1,2,⋯,l.
Finally, the S(FPCN) can be obtained as follows:
zk+1i,j=1(1+m1+r1+r2)[r12(zk+1i−1,j+zk+1i+1,j)+r22(zk+1i,j−1+zk+1i,j+1)+r12(zki−1,j+zki+1,j)+r22(zki,j−1+zki,j+1)+(2−b1−r1−r2)zki,j+m1zk−1i,j+(2bk−bk−1)z0i,j−bkz1i,j−k−1∑s=1(bs−1−2bs+bs+1)zk−si,j+mofk+1/2i,j], | (2.5) |
for i=1,2,⋯,m−1, j=1,2,⋯,n−1 and k=0,1,2,⋯,l, with initial conditions: z0i,j=g(ui,vj), and boundary conditions:
zk0,j=f1(vj,t),zkL,j=f2(vj,t), |
zki,0=g3(uj,t),zki,L=g4(uj,t), |
0≤u,v≤L,0≤t≤T.
The technique defined in Eq (2.5) is a point-dependent technique on the computational domain that utilizes all the points for the knot convergence as illustrated in Figure 1. All knots of the solution rectangular domain are considered as iterative knots in the iterative procedure at each temporal level until the knot converged solutions are obtained for some predetermined convergence criterion as well as for some tolerance factor. After achieving grid converged solutions, the predicted knot solutions are used as a beginning prediction for the next time level.
The R(FPCN) can be developed by utilization of R(C-N) difference technique, the Caputo time fractional derivative of order α from Eq (2.3) and central difference approximation for first order derivative. This will leads Eq (2.1) to the following expression,
zk+1i,j−2zki,j+zk−1i,j+k∑s=1bs(zk−s+1i,j−2zk−si,j+zk−s−1i,j)+m1(zk+1i,j−zk−1i,j)=r14(zk+1i−1,j+1−2zk+1i,j+zk+1i+1,j−1+zki−1,j+1−2zki,j+zki+1,j−1)+r24(zk+1i−1,j−1−2zk+1i,j+zk+1i+1,j+1+zki−1,j−1−2zki,j+zki+1,j+1)+mofk+1/2i,j, |
for i=1,2,⋯,m−1, j=1,2,⋯,n−1 and k=0,1,2,⋯,l.
Finally, we get the R(FPCN) as follows,
zk+1i,j=1(1+m1+r1/2+r2/2)[r14(zk+1i−1,j+1+zk+1i+1,j−1)+r24(zk+1i−1,j−1+zk+1i+1,j+1)+r14(zki−1,j+1+zki+1,j−1)+r24(zki−1,j−1+zki+1,j+1)+(2−b1−r1/2−r2/2)zki,j+m1zk−1i,j+(2bk−bk−1)z0i,j−bkz1i,j−k−1∑s=1(bs−1−2bs+bs+1)zk−si,j+mofk+1/2i,j], | (3.1) |
for i=1,2,⋯,m−1, j=1,2,⋯,n−1 and k=0,1,2,⋯,l, with initial conditions: z0i,j=g(ui,vj), and boundary conditions:
zk0,j=f1(vj,t),zkL,j=f2(vj,t),zki,0=g3(uj,t),zki,L=g4(uj,t), |
0≤u,v≤L,0≤t≤T.
The main purpose of the rotated grid scheme is to evaluate the values of the half of nodes of the discretized domain to get quick convergence. On the other hand, the remaining grid points can be determined by the standard grid scheme.
The technique in Eq (3.1) is a half grid dependent technique on the solution rectangular domain that employs half of the knots for the grid convergence. In the half grid dependent technique, we divide knots of entire discretized domain into sets of two knots, one type of knot is recognized as iterative knots ∙ while the other types of knots are recognized as direct knots ∘ as shown in the Figure 2. This procedure divides in two parts, in first part the approximation in Eq (3.1) iterates only on iterative knots ∙ of the suggested domain until a certain convergence is attained. In the second part, when convergence is attained, the values on direct points ∘ calculated by approximation defined in Eq (2.5). By sweeping half of the knots, we decrease approximately half of the algorithm's computational difficulty, resulting in lower CPU timings for each iteration.
Lemma 1. The coefficients bs, s=0,1,2,⋯ shown in Eq (2.3) fulfill the following characteristics:
(1) b0=1, bs>0, ∀s=0,1,2,⋯.
(2) bs>bs+1, ∀s=0,1,2,⋯.
The stability of the suggested finite difference technique using the Fourier method will be discussed in this part. Consider ξki,j be the approximated solution of Eq (3.1) and the error is defined as:
ξki,j=Zki,j−zki,j, |
for i=1,2,⋯,m−1, j=1,2,⋯,n−1 and k=0,1,2,⋯,l, and
ξk=[ξk1,1,ξk1,2,⋯ξk1,m−1,ξk2,1,ξk2,2,⋯ξk2,m−1,ξkm−1,1,ξkm−1,2,⋯ξkm−1,m−1]T. |
This error satisfies Eq (3.1) and we have,
−r14(ξk+1i−1,j+1+ξk+1i+1,j−1)+(1+m1+r1/2+r2/2)ξk+1i,j−r24(ξk+1i−1,j−1+ξk+1i+1,j+1)=r14(ξki−1,j+1+ξki+1,j−1)+(2−b1−r1/2−r2/2)ξki,j+r24(ξki−1,j−1+ξki+1,j+1)+m1zk−1i,j+(2bk−bk−1)ξ0i,j−bkξ1i,j−k−1∑s=1(bs−1−2bs+bs+1)ξk−si,j, | (4.1) |
for all 1≤i≤m−1, 1≤j≤n−1, 0≤k≤l, with the initial and boundary conditions are
ξk0=ξkL=ξ0i,j=0. |
For k=0,1,2,⋯,l, define the grid function as,
ξk(u,v)={ξki,j,ui−hu2<u≤ui+hu2,vj−hv2<v≤vj+hv20,0≤u≤hu2orL−hu2≤u≤L0,0≤v≤hv2orL−hv2≤v≤L. |
The ξk(u,v) can be expressed in the Fourier series as follows:
ξk(u,v)=∞∑l1=−∞∞∑l2=−∞λk(l1,l2)e2π√−1(l1u/L+l2v/L), |
where,
λk(l1,l2)=1L2∫L0∫L0ξk(u,v)e−2π√−1(l1u/L+l2v/L)dudv. |
By the l2 error norm definition and Parseval's equality, we have
∥ξk∥22=m−1∑i=1n−1∑j=1huhv|ξki,j|2=∞∑l1=−∞∞∑l2=−∞|λk(l1,l2)|2. |
Let the error Eq (4.1) has the solution of the following form
ξki,j=λke√−1(σ1ihu+σ2jhv) |
where, σ1=2πl1/L and σ2=2πl2/L.
Using the above expression in Eq (4.1) we obtain,
λk+1=11+m1+μ[(2−b1−μ)λk+m1λk−1+(2bk−bk−1)λ0−bkλ1−k−1∑s=1(bs−1−2bs+bs+1)λk−s], | (4.2) |
where
μ=r2sin2(σ1ihu+σ2jhv2)+r1sin2(σ1ihu−σ2jhv2). |
Proposition 1. The R(FPCN) define in Eq (3.1) is unconditionally stable.
Proof. First, we utilize the mathematical induction to satisfy the following result.
If λk(k=1,2,⋯,l) satisfy Eq (4.2), then |λk|≤|λ0|. For k=0, from Eq (4.2), we have
|λ1|=2−b1−μ2+μ|λ0|. |
Since, μ≥0 and 0<b1<1, therefore, we have
|λ1|≤|λ0|. |
Now assume that |λm|≤|λ0| for m=1,2,⋯,k, we have
|λk+1|≤11+m1−μ[(2−b1−μ)|λk|+m1|λk−1|+(2bk−bk−1)|λ0|−bk|λ1|−k−1∑s=1(bs−1−2bs+bs+1)|λk−s|]=11+m1+μ[(2−b1−μ)+m1+(2bk−bk−1)−bk−k−1∑s=1(bs−1−2bs+bs+1)]|λ0|=11+m1+μ[(2−b1−μ)+m1+bk−bk−1−(b0−b1−bk−1+bk)]|λ0|=1−m1−μ1+m1+μ|λ0|, |
i.e.,
|λk+1|≤|λ0|. |
Now utilizing the preceding result and Parseval's equality, we obtain the following inequality,
∥ξk∥22=∞∑l1=−∞∞∑l2=−∞|λk(l1,l2)|2≤∞∑l1=−∞∞∑l2=−∞|λ0(l1,l2)|2=∥ξ0∥22, |
that is,
∥ξk∥≤∥ξ0∥, |
for all k=1,2,⋯,l.
Therefore, the R(FPCN) in Eq (3.1) is unconditionally stable.
To investigate the convergence of the R(FPCN) iterative technique defined in Eq (3.1). Suppose that z(ui,vj,tk+1/2) is the exact solution illustrated by the Taylor series. Then the truncation error at point z(ui,vj,tk+1/2) is denoted by Rk+1/2i,j and is defined as,
Rk+1/2i,j=1ταΓ(3−α)k∑s=0bs(z(ui,vj,tk+1−s)−2z(ui,vj,tk−s)+z(ui,vj,tk−s−1)+m1(z(ui,vj,tk+1)−z(ui,vj,tk−1))−14(hu)2[z(ui−1,vj+1,tk+1)−2z(ui,vj,tk+1)+z(ui+1,vj−1,tk+1)+z(ui−1,vj+1,tk)−2z(ui,vj,tk)+z(ui+1,vj−1,tk)]−14(hv)2[z(ui−1,vj−1,tk+1)−2z(ui,vj,tk+1)+z(ui+1,vj+1,tk+1)+z(ui−1,vj−1,tk)−2z(ui,vj,tk)+z(ui+1,vj+1,tk)]−mof(ui,vj,tk+1/2), | (5.1) |
for all i=1,2,⋯,m−1, j=1,2,⋯,n−1 and k=0,1,2,⋯,l.
From Eq (2.1), we have
Rk+1/2i,j=1ταΓ(3−α)k∑s=0(z(ui,vj,tk+1−s)−2z(ui,vj,tk−s)+z(ui,vj,tk−s−1)−∂αz(ui,vj,tk+1)∂tα+a(ui,vj,tk+1)[∂2z(ui,vj,tk+1)∂u2−14(hu)2{z(ui−1,vj+1,tk+1)−2z(ui,vj,tk+1)+z(ui+1,vj−1,tk+1)+z(ui−1,vj+1,tk)−2z(ui,vj,tk)+z(ui+1,vj−1,tk)}]+b(ui,vj,tk+1)[∂2z(ui,vj,tk+1)∂v2−14(hv)2{z(ui−1,vj−1,tk+1)−2z(ui,vj,tk+1)+z(ui+1,vj+1,tk+1)+z(ui−1,vj−1,tk)−2z(ui,vj,tk)+z(ui+1,vj+1,tk)}]=O(τ3−α+(hu)2+(hv)2). |
There is a constant C1 for all i=1,2,⋯,m−1, j=1,2,⋯,n−1 and k=0,1,2,⋯,l such that,
Rk+1/2i,j≤C1(τ3−α+(hu)2+(hv)2), | (5.2) |
where,
C1=max1≤i≤m−1,1≤j≤n−1,0≤k≤l{Cki,j}. |
Define the function,
ϕki,j=z(ui,vj,tk)−zki,j. |
From Eq (5.1), we have
−r14(z(ui−1,vj+1,tk+1)+z(ui+1,vj−1,tk+1))+(1+m1+r1/2+r2/2)z(ui,vj,tk+1)−r24(z(ui−1,vj−1,tk+1)+z(ui+1,vj+1,tk+1))=r14(z(ui−1,vj+1,tk)+z(ui+1,vj−1,tk))+(2−b1−r1/2−r2/2)z(ui,vj,tk)+r24(z(ui−1,vj−1,tk)+z(ui+1,vj+1,tk))+m1z(ui,vj,tk−1)+(2bk−bk−1)z(ui,vj,t0)−bkz(ui,vj,t1)−k−1∑s=1(bs−1−2bs+bs+1)z(ui,vj,tk−s)+mof(ui,vj,tk+1/2). | (5.3) |
In order to get the error equation subtract Eq (5.3) from Eq (3.1), we have
−r14(ϕk+1i−1,j+1+ϕk+1i+1,j−1)+(1+m1+r1/2+r2/2)ϕk+1i,j−r24(ϕk+1i−1,j−1+ϕk+1i+1,j+1)=r14(ϕki−1,j+1+ϕki+1,j−1)+(2−b1−r1/2−r2/2)ϕki,j+r24(ϕki−1,j−1+ϕki+1,j+1)+m1ϕk−1i,j+(2bk−bk−1)ϕ0i,j−bkϕ1i,j−k−1∑s=1(bs−1+2bs+bs+1)ϕk−si,j+moRk+1/2i,j, | (5.4) |
for all
1≤i≤n−1,1≤j≤m−1,0≤k≤l−1. |
This equation verifies the boundary conditions
ϕki,0=ϕki,m=0,1≤i≤n−1,0≤k≤l−1, |
ϕk0,j=ϕkn,j=0,1≤j≤m−1,0≤k≤l−1, |
and initial conditions
ϕ0i,j=0,0≤i≤n,1≤j≤m. |
For k=0,1,2,⋯,l, define the grid function
ϕk(u,v)={ϕki,j,ui−hu2<u≤ui+hu2,vj−hv2<v≤vj+hv20,0≤u≤hu2orL−hu2≤u≤L0,0≤v≤hv2orL−hv2≤v≤L |
and
Rk(u,v)={Rki,j,ui−hu2<u≤ui+hu2,vj−hv2<v≤vj+hv20,0≤u≤hu2orL−hu2≤u≤L0,0≤v≤hv2orL−hv2≤v≤L |
for all 1≤i≤m−1, 1≤j≤n−1, 0≤k≤l.
The functions ϕk(u,v) and Rk(u,v) can be defined in the Fourier series,
ϕk(u,v)=∞∑l1=−∞∞∑l2=−∞ηk(l1,l2)e2π√−1(l1u/L+l2v/L), |
Rk(u,v)=∞∑l1=−∞∞∑l2=−∞ζk(l1,l2)e2π√−1(l1u/L+l2v/L), |
where,
ηk(l1,l2)=1L2∫L0∫L0ϕk(u,v)e−2π√−1(l1u/L+l2v/L)dudv, | (5.5) |
ζk(l1,l2)=1L2∫L0∫L0Rk(u,v)e−2π√−1(l1u/L+l2v/L)dudv. | (5.6) |
By the l2 error norm and Parseval's equality, we have
∥ϕk∥22=m−1∑i=1n−1∑j=1huhv|ϕki,j|2=∞∑l1=−∞∞∑l2=−∞|ηk(l1,l2)|2, | (5.7) |
∥Rk∥22=m−1∑i=1n−1∑j=1huhv|Rki,j|2=∞∑l1=−∞∞∑l2=−∞|ζk(l1,l2)|2, | (5.8) |
where,
ϕk=[ϕk1,1,ϕk1,2,⋯ϕk1,m−1,ϕk2,1,ϕk2,2,⋯ϕk2,m−1,ϕkm−1,1,ϕkm−1,2,⋯ϕkm−1,m−1]T, |
Rk=[Rk1,1,Rk1,2,⋯Rk1,m−1,Rk2,1,Rk2,2,⋯Rk2,m−1,Rkm−1,1,Rkm−1,2,⋯Rkm−1,m−1]T. |
Suppose that the solutions have the following forms,
ϕki,j=ηke√−1(σ1ihu+σ2jhv), |
Rki,j=ζke√−1(σ1ihu+σ2jhv), |
where, σ1=2πl1/L and σ2=2πl2/L.
Using the above equation in Eq (5.4) we have,
ηk+1=11+m1+μ[(2−b1−μ)ηk+m1ηk−1+(2bk−bk−1)η0−bkη1−k−1∑s=1(bs−1−2bs+bs+1)ηk−s+moζk+1/2], | (5.9) |
where, μ is defined in section (4).
Proposition 2. Assume that ηk(k=1,2,⋯,l) is the solution of Eq (5.9), then there is a positive constant C2, so that |ηk|≤C2|ζ1/2|,k=1,2,⋯,l.
Proof. From Eq (5.5), noticing that ϕ0=0, we have, η0(l1,l2)=0.
For convergence the series, there is a positive constant C2>0 of the right side of Eq (5.8) such that,
|ζk|=|ζk(l1,l2)|≤Ck|ζ1/2(l1,l2)|=C2|ζ1/2|,k=1,2,⋯,l, | (5.10) |
where, C2=max1≤k≤l{Ck}. We will verify this by mathematical induction.
For k=0, from Eq (5.9) we have,
η1=mo2+m1+μζ1/2. |
Since, μ≥0 and mo<2+m1+μ, from Eq (5.10), we get thus,
|η1|≤C2|ζ1/2|. |
Assume that |ηm|≤C2|ζ1/2|,m=1,2,⋯,k. We prove that it is true for n=k+1. Again from Eq (5.9) we have,
|ηk+1|≤11+m1+μ[(2−b1−μ)|ηk|+m1|ηk−1|−bk|η1|−k−1∑s=1(bs−1−2bs+bs+1)|ηk−s|+mo|ζ1/2|]=11+m1+μ[2−b1−μ+m1−bk−k−1∑s=1(bs−1−2bs+bs+1)+mo]C2|ζ1/2|=11+m1+μ[2−b1−μ+m1−bk−(b0−b1−bk−1+bk)+mo]C2|ζ1/2|=11+m1+μ[1−μ+m1−2bk+bk−1+mo]C2|ζ1/2|. |
From Lemma 1(2), we have 1=b0>b1>b2>⋯>bk−1>bk and also we have mo=(τ)αΓ(3−α), m1=(τ)α−1Γ(3−α). In both cases, as τ→0 (i.e. increase the number of time levels) implies that bk−1,bk,mo,m1→0 thus, we have
|ηk+1|≤1−μ1+μC2|ζ1/2|≤C2|ζ1/2|. |
This completes the proof.
Theorem 1. The R(FPCN) defined by Eq (3.1) is L2 convergent of order τ3−α+(hu)2+(hv)2.
Proof. Apply the Proposition 2 and using Eqs (5.7) and (5.8), we have
∥ϕk∥22=∞∑l1=−∞∞∑l2=−∞|ηk(l1,l2)|2≤∞∑l1=−∞∞∑l2=−∞C22|ζ1/2(l1,l2)|2=C22∥R1/2∥22. |
There is a positive constant C1 as in inequality Eq (5.2) such that
‖ϕk‖2≤C2‖R1/2‖2≤C2C1(τ3−α+(hu)2+(hv)2). |
Therefore, ‖ϕk‖2≤C(τ3−α+(hu)2+(hv)2) where C=C1C2.
To demonstrate the effectiveness and simplicity of the R(FPCN) technique in solving the 2D TFDWE, the numerical examples were performed on a PC with a Core 2 Duo 2.8 GHz processor and 2GB of RAM running with Window XP SP3 operating system in Mathematica 11. To get accurate results, the numerical examples were shown at three time levels for various mesh sizes 4, 8, 12 and 16. For the space discretization, we assume that h=hu=hv in both u and v directions and different time steps 1/4, 1/8, 1/12 and 1/16 are considered for the time discretization (0<t<1) in the suggested domain. The Gauss-Seidel technique was used throughout our computations for convenience with relaxation factor ωe set to 1. The l∞ norm was utilized for the convergence with the tolerance factor ε=10−5.
The computational difficulty of any iterative numerical technique is entirely determined by the number of arithmetic operations performed per iteration. The higher the computational difficulty of iterative technique, the more operation performs by the algorithm denoting the slow convergence rate. To assess the computational complexity of both techniques suppose that the suggested domain is discretized with grid size n, and that the internal grid knots will be λ2 where λ=n−1. Table 1 describes the computational complexity of the S(FPCN) and the R(FPCN) iterative methods before and after convergence, whereas Table 2 summarizes the total number of arithmetic used. The results of conducted numerical experiments of both the iterative methods are shown in Table 3 where the promising results of R(FPCN) indicates that this method is more efficient than S(FPCN) in the forms of execution time (in seconds), number of iterations (Ite.) and the total number of mathematical operations at various values of h, τ and α. Moreover, R(FPCN) technique has 40–45% less computational difficulty than S(FPCN), whereas the calculating time and the number of iterations were observed almost 30–35% and 20–25% less, with the same degree of accuracy.
Method | Each iteration | After convergence | ||
(+/-) | (×/÷) | (+/-) | (×/÷) | |
S(FPCN) | (16+3k)λ2 | (8+2k)λ2 | - | - |
R(FPCN) | (16+3k)λ2+12 | (8+2k)λ2+12 | (16+3k)λ2−12 | (8+2k)λ2−12 |
Methods | Total operations |
S(FPCN) | (24+5k)λ2∗Ite |
R(FPCN) | (12+2.5k)(λ2+1)∗Ite+(12+2.5k)(λ2−1) |
α=1.25 | |||||||
Δt | h−1 | Method | Time (s) | Iteration | Ave error | Max error | Total operations |
1/4 | 4 | S(FPCN) | 0.04680 | 12 | 1.23889×10−2 | 1.73310×10−2 | 4,752 |
R(FPCN) | 0.03120 | 7 | 1.39199×10−2 | 2.18245×10−2 | 1,716 | ||
1/8 | 8 | S(FPCN) | 0.99840 | 26 | 1.02852×10−3 | 1.99684×10−3 | 81,536 |
R(FPCN) | 0.37440 | 15 | 1.06050×10−3 | 2.05820×10−3 | 25,536 | ||
1/12 | 12 | S(FPCN) | 6.95764 | 38 | 2.00135×10−4 | 4.56704×10−4 | 386,232 |
R(FPCN) | 2.37122 | 22 | 2.17520×10−4 | 4.78263×10−4 | 117,768 | ||
1/16 | 16 | S(FPCN) | 27.2222 | 49 | 3.99971×10−5 | 1.24839×10−4 | 1,146,600 |
R(FPCN) | 9.09486 | 28 | 5.90195×10−5 | 1.53416×10−4 | 340,704 | ||
α=1.50 | |||||||
Δt | h−1 | Method | Time (s) | Iteration | Ave error | Max error | Total operations |
1/4 | 4 | S(FPCN) | 0.03120 | 11 | 1.44048×10−2 | 2.05458×10−2 | 4,356 |
R(FPCN) | 0.015600 | 7 | 1.60035×10−2 | 2.55940×10−2 | 1,716 | ||
1/8 | 8 | S(FPCN) | 0.79560 | 21 | 1.10424×10−3 | 2.18167×10−3 | 65,856 |
R(FPCN) | 0.32760 | 13 | 1.13615×10−3 | 2.28299×10−3 | 22,336 | ||
1/12 | 12 | S(FPCN) | 5.36643 | 30 | 2.04407×10−4 | 4.88386×10−4 | 304,920 |
R(FPCN) | 1.91881 | 18 | 2.23152×10−4 | 5.14603×10−4 | 97,272 | ||
1/16 | 16 | S(FPCN) | 20.5921 | 38 | 4.79959×10−5 | 1.40112×10−4 | 889,200 |
R(FPCN) | 7.19165 | 23 | 6.11451×10−5 | 1.58162×10−4 | 281,944 | ||
α=1.75 | |||||||
Δt | h−1 | Method | Time (s) | Iteration | Ave error | Max error | Total operations |
1/4 | 4 | S(C-N) | 0.04680 | 10 | 1.96933×10−2 | 2.84240×10−2 | 3,960 |
R(C-N) | 0.03120 | 6 | 2.17247×10−2 | 3.51605×10−2 | 1,496 | ||
1/8 | 8 | S(C-N) | 0.68640 | 18 | 1.54832×10−3 | 3.18035×10−3 | 56,376 |
R(C-N) | 0.29640 | 11 | 1.58320×10−3 | 3.30433×10−3 | 19,136 | ||
1/12 | 12 | S(C-N) | 4.14963 | 23 | 3.08254×10−4 | 7.36312×10−4 | 233,772 |
R(C-N) | 1.70041 | 15 | 3.20382×10−4 | 7.54646×10−4 | 81,900 | ||
1/16 | 16 | S(C-N) | 15.0697 | 27 | 9.07687×10−5 | 2.41195×10−4 | 631,800 |
R(C-N) | 5.52244 | 17 | 9.91180×10−5 | 2.53874×10−4 | 211,432 |
Example 1. Consider the following TFDWE [37],
∂αz(u,v,t)∂tα+∂z(u,v,t)∂t=∂2z(u,v,t)∂u2+∂2z(u,v,t)∂v2+f(u,v,t), |
where,
f(u,v,t)=2t2−α(u+v−2)(u+v)(α−2)Γ(2−α)+4t2+2t(−u−v+2)(u+v). |
The initial conditions are, z(u,v,0)=g(u,v)=0, zt(u,v,0)=0, and boundary boundary conditions
z(0,v,t)=f1(v,t)=t2v(2−v),z(1,v,t)=f2(v,t)=t2(1−v2), |
z(u,0,t)=g3(u,t)=t2u(2−u),z(u,1,t)=g4(u,t)=t2(1−u2). |
The exact analytical solution is z(u,v,t)=t2(u+v)(2−u−v).
The diagram in Figure 3 represents the graphs of total number of operations, number of iterations and execution of timings (in sec.) of S(FPCN) and R(FPCN) iterative methods against the mesh sizes at different values of α. It can easily be seen from these graphs, the quantity of elapsed time, number of iterations and total number of operations of R(FPCN) iterative scheme are considerable less as compare to S(FPCN) iterative scheme.
Table 4 shows the temporal convergence order of the numerical data obtained from the proposed rotated Crank-Nicolson scheme for different time nodes τ and α, and for fixed space node h=π60, when end time is taken Tend=1. It is observed that R(FPCN) iterative scheme generates (3−α) temporal convergence order. Table 5 demonstrates spatial convergence order for different space nodes h and α, and for fixed time node τ=0.01, when space end point is chosen Lend=1. This shows that R(FPCN) iterative scheme attains second order spatial accuracy. Therefore convergence rate of both tables verify the theoretical derivation of convergence order of our proposed R(FPCN) iterative scheme as derived in Theorem 1.
h/τ | α = 1.25 | α = 1.50 | α = 1.75 | ||||
h | τ | L2 norm | γ1−order | L2 norm | γ1−order | L2 norm | γ1−order |
π60 | 1/25 | 5.85372×10−4 | - | 5.86342×10−4 | - | 7.97263×10−5 | - |
1/50 | 3.47837×10−4 | 1.3692 | 3.09532×10−4 | 1.7518 | 4.24843×10−5 | 1.8663 | |
1/75 | 7.94632×10−5 | 1.5861 | 8.95312×10−5 | 1.8703 | 4.57934×10−6 | 1.9030 | |
1/100 | 4.93682×10−5 | 1.7938 | 5.97534×10−5 | 1.9094 | 2.84623×10−6 | 1.9986 |
h/τ | α = 1.25 | α = 1.50 | α = 1.75 | ||||
τ | h | L2 norm | γ2−order | L2 norm | γ2−order | L2 norm | γ2−order |
0.01 | π20 | 9.76342×10−4 | - | 8.75324×10−5 | - | 7.41940×10−5 | - |
π40 | 5.86453×10−4 | 1.5563 | 5.85309×10−5 | 1.7610 | 4.06432×10−5 | 1.9132 | |
π60 | 5.45790×10−5 | 1.6734 | 2.07536×10−5 | 1.8310 | 6.06421×10−6 | 1.9976 | |
π80 | 3.97521×10−5 | 1.7859 | 4.68310×10−6 | 1.9928 | 8.75312×10−7 | 2.0001 |
We calculate the error e(τ,h) in L2 norm as e(τ,h)=‖Zexact−zapprox.‖2 and temporal and spatial convergence order of the proposed method by the following formulas [38]:
γ1−order≈log(e1/e2)log(τ1/τ2), | (6.1) |
where, e1 and e2 are the errors correspond to the time step with size τ1 and τ2, and
γ2−order≈log(e′1/e′2)log(h1/h2) | (6.2) |
where, e′1 and e′2 are the errors correspond to the space step with size h1 and h2.
Figure 4 represents graphs of temporal and spatial convergence rates of our proposed scheme at different values of α. It can be observed from both graphs as the time or space steps (i.e. h or τ) decreases the temporal and spatial parameters converges to second order accuracy in time and space respectively, at different values of α. Figure 5 illustrates the graph of absolute errors at different values of α. It can easily be observed that the absolute error is significantly small at α=1.75 compared with α=1.25.
In this paper, we formulate two numerical iterative schemes S(FPCN) and R(FPCN) by utilizing the (3−α)-Caputo approximation for the time fractional derivative, and standard and rotated five points Crank-Nicolson approximations for the space derivatives in solving the diffusion damped wave equation of fractional order. The developed schemes are tested with a numerical example and results are compared. The numerical results revealed that the computational cost of R(FPCN) method is reduced as compared to S(FPCN) method and it also reduces the total number of operations, iteration numbers and CPU-timings which assist the algorithm in attaining rapid convergence. The derived numerical data sports our theoretical derivations. There are a lot of open problems that need to be solved by grouping strategies based on rotated finite difference approximations for several types of FDEs especially for three-dimensional case.
This research project is supported by Thailand Science Research and Innovation (TSRI) Basic Research Fund: Fiscal year 2023 under project number FRB660073/0164.
The authors declare that they have no conflicts of interest.
[1] | Y. Povstenko, Linear fractional diffusion-wave equation for scientists and engineers, Birkhäuser Cham, 2015. https://doi.org/10.1007/978-3-319-17954-4 |
[2] |
O. P. Agrawal, O. Defterli, D. Baleanu, Fractional optimal control problems with several state and control variables, J. Vib. Control., 16 (2010), 1967–1976. https://doi.org/10.1177/1077546309353361 doi: 10.1177/1077546309353361
![]() |
[3] | I. Podlubny, Fractional differential equations, New York: Academic Press, 1999. |
[4] |
O. A. Arqub, S. Nabil, Application of reproducing kernel algorithm for solving Dirichlet time-fractional diffusion-Gordon types equations in porous media, J. Porous Med., 22 (2019), 411–434. https://doi.org/10.1615/JPorMedia.2019028970 doi: 10.1615/JPorMedia.2019028970
![]() |
[5] | O. A. Arqub, Application of residual power series method for the solution of time-fractional Schrodinger equations in one-dimensional space, Fund. Inf., 166 (2019), 87–110. |
[6] |
W. R. Schneider, W. Wyss, Fractional diffusion and wave equation, J. Math. Phys., 30 (1989), 134–144. https://doi.org/10.1063/1.528578 doi: 10.1063/1.528578
![]() |
[7] |
R. Gorenflo, Y. Luchko, F. Mainardi, Wright function as scale-invariant solution of the wave equation, J. Comput. Appl. Math., 118 (2000), 175–191. https://doi.org/10.1016/S0377-0427(00)00288-0 doi: 10.1016/S0377-0427(00)00288-0
![]() |
[8] |
G. Jumarie, Laplace's transform of fractional order via the Mittag-Leffler function and modified Riemann-Liouville derivative, Appl. Math. Lett., 22 (2009), 1659–1664. https://doi.org/10.1016/j.aml.2009.05.011 doi: 10.1016/j.aml.2009.05.011
![]() |
[9] |
C. M. Chen, F. Liu, I. Turner, V. A. Anh, A Fourier method for the fractional diffusion equation describing sub-diffusion, J. Comput. Phys., 227 (2007), 886–897. https://doi.org/10.1016/j.jcp.2007.05.012 doi: 10.1016/j.jcp.2007.05.012
![]() |
[10] |
S. Wang, M. Xu, X. Li, Green's function of time fractional diffusion equation and its applications in fractional quantum mechanics, Nonlinear Anal.: Real World Appl., 10 (2009), 1081–1086. https://doi.org/10.1016/j.nonrwa.2007.11.024 doi: 10.1016/j.nonrwa.2007.11.024
![]() |
[11] |
X. Zhang, J. Zhao, J. Liu, B. Tang, Homotopy perturbation method for two dimensional time-fractional wave equation, Appl. Math. Model., 38 (2014), 5545–5552. https://doi.org/10.1016/j.apm.2014.04.018 doi: 10.1016/j.apm.2014.04.018
![]() |
[12] |
J. Chen, F. Liu, V. Anh, S. Shen, Q. Liu, C. Liao, The analytical solution and numerical solution of the fractional diffusion-wave equation with damping, Appl. Math. Comput., 219 (2012), 1737–1748. https://doi.org/10.1016/j.amc.2012.08.014 doi: 10.1016/j.amc.2012.08.014
![]() |
[13] | A. K. Pani, J. Y. Yuan, Mixed finite element method for a strongly damped wave equation, Numer. Methods Partial Differ. Equ., 17 (2001), 105–119. |
[14] |
Z. G. Shi, Y. M. Zhao, F. Liu, Y. F. Tang, F. L. Wang, Y. H. Shi, High accuracy analysis of an H1-Galerkin mixed finite element method for two-dimensional time fractional diffusion equations, Comput. Math. Appl., 74 (2017), 1903–1914. https://doi.org/10.1016/j.camwa.2017.06.057 doi: 10.1016/j.camwa.2017.06.057
![]() |
[15] |
K. Mihály, L. Stig, L. Fredrik, Weak convergence of finite element approximations of linear stochastic evolution equations with additive noise Ⅱ. Fully discrete schemes, BIT Numer. Math., 53 (2013), 497–525. https://doi.org/10.1093/geront/gnt023 doi: 10.1093/geront/gnt023
![]() |
[16] |
G. Matthias, K. Mihály, L. Stig, Rate of weak convergence of the finite element method for the stochastic heat equation with additive noise, BIT Numer. Math., 49 (2009), 343–356. https://doi.org/10.1007/s10543-009-0227-y doi: 10.1007/s10543-009-0227-y
![]() |
[17] |
A. Iqbal, N. N. Hamid, A. I. Ismail, M. Abbas, Galerkin approximation with quintic B-spline as basis and weight functions for solving second order coupled nonlinear Schrödinger equations, Math. Comput. Simul., 187 (2021), 1–16. https://doi.org/10.3917/parl2.hs16.0187 doi: 10.3917/parl2.hs16.0187
![]() |
[18] |
Y. Zhang, A finite difference method for fractional partial differential equation, Appl. Math. Comput., 215 (2009), 524–529. https://doi.org/10.1016/j.amc.2009.05.018 doi: 10.1016/j.amc.2009.05.018
![]() |
[19] |
A. Kadem, Y. Luchko, D. Baleanu, Spectral method for solution of the fractional transport equation, Rep. Math. Phys., 66 (2010), 103–115. https://doi.org/10.1016/S0034-4877(10)80026-6 doi: 10.1016/S0034-4877(10)80026-6
![]() |
[20] |
T. Akram, M. Abbas, A. I. Izani, An extended cubic B-spline collocation scheme for time fractional sub-diffusion equation, AIP Conf. Proc., 2184 (2019), 060017. https://doi.org/10.1063/1.5136449 doi: 10.1063/1.5136449
![]() |
[21] |
T. Akram, M. Abbas, A. Ali, A. Iqbal, D. Baleanu, A numerical approach of a time fractional reaction-diffusion model with a non-singular kernel, Symmetry, 12 (2020), 1653. https://doi.org/10.3390/sym12101653 doi: 10.3390/sym12101653
![]() |
[22] |
T. Akram, M. Abbas, M. B. Riaz, A. I. Ismail, N. M. Ali, Development and analysis of new approximation of extended cubic B-spline to the non-linear time fractional Klein-Gordon equation, Fractals, 28 (2020), 2040039. https://dx.doi.org/10.1142/S0218348X20400393 doi: 10.1142/S0218348X20400393
![]() |
[23] |
A. Ali, N. H. M. Ali, On numerical solution of fractional order delay differential equation using Chebyshev collocation method, New Trends Math. Sci., 6 (2018), 8–17. http://dx.doi.org/10.20852/ntmsci.2017.240 doi: 10.20852/ntmsci.2017.240
![]() |
[24] |
A. Ali, N. H. M. Ali, On numerical solution of multi-terms fractional differential equations using shifted Chebyshev poynomials, Int. J. Pur. Appl. Math., 120 (2018), 111–125. https://doi.org/10.1016/j.critrevonc.2018.03.012 doi: 10.1016/j.critrevonc.2018.03.012
![]() |
[25] | M. Martins, W. S. Yousif, D. J. Evans, Explicit group AOR method for solving elliptic partial differential equations, Neural Parallel Sci. Comput., 10 (2002), 411–421. |
[26] |
M. Othman, A. R. Abdullah, D. J. Evans, A parallel four points modified explicit group algorithm on shared memory multiprocessors, Parallel Algorithms Appl., 19 (2004), 1–9. https://doi.org/10.1080/1063719042000208818 doi: 10.1080/1063719042000208818
![]() |
[27] |
W. S. Yousif, D. J. Evans, Explicit group over-relaxation methods for solving elliptic partial differential equations, Math. Comput. Simul., 28 (1986), 453–466. https://doi.org/10.1016/0378-4754(86)90040-6 doi: 10.1016/0378-4754(86)90040-6
![]() |
[28] |
N. H. M. Ali, L. M. Kew, New explicit group iterative methods in the solution of two dimensional hyperbolic equations, J. Comput. Phys., 231 (2012), 6953–6968. https://doi.org/10.1016/j.jcp.2012.06.025 doi: 10.1016/j.jcp.2012.06.025
![]() |
[29] |
M. Othman, A. R. Abdullah, The halfsweeps multigrid method as a fast multigrid Poisson solver, Int. J. Comput. Math., 69 (1998), 319–329. https://doi.org/10.1080/00207169808804726 doi: 10.1080/00207169808804726
![]() |
[30] |
L. M. Kew, N. H. M. Ali, New explicit group iterative methods in the solution of three dimensional hyperbolic equations, J. Comput. Phys., 294 (2015), 382–404. https://doi.org/10.1016/j.jcp.2015.03.052 doi: 10.1016/j.jcp.2015.03.052
![]() |
[31] |
D. J. Evans, R. S. Haghighi, Explicit group versus implicit line iterative methods, Int. J. Comput. Math., 16 (1984), 261–316. https://doi.org/10.1080/00207168408803442 doi: 10.1080/00207168408803442
![]() |
[32] |
O. A Arqub, Z. Odibat, M. Al-Smadi, Numerical solutions of time-fractional partial integrodifferential equations of Robin functions types in Hilbert space with error bounds and error estimates, Nonlinear Dyn., 94 (2018), 1819–1834 https://doi.org/10.1007/s11071-018-4459-8 doi: 10.1007/s11071-018-4459-8
![]() |
[33] |
O. A Arqub, M. Al-Smadi, An adaptive numerical approach for the solutions of fractional advection-diffusion and dispersion equations in singular case under Riesz's derivative operator, Phys. A: Stat. Mech. Appl., 540 (2020), 123257. https://doi.org/10.1016/j.physa.2019.123257 doi: 10.1016/j.physa.2019.123257
![]() |
[34] |
A. T. Balasim, N. H. M. Ali, The solution of 2-D time-fractional diffusion equation by the fractional modified explicit group iterative method, AIP Conf. Proc., 1775 (2016), 030014. https://doi.org/10.1063/1.4965134 doi: 10.1063/1.4965134
![]() |
[35] |
A. T. Balasim, N. H. M. Ali, Group iterative methods for the solution of two-dimensional time-fractional diffusion equation, AIP Conf. Proc., 1750 (2016), 030003. https://doi.org/10.1063/1.4954539 doi: 10.1063/1.4954539
![]() |
[36] |
M. Uddin, Kamran, A. Ali, A localized transform-based meshless method for solving time fractional wave-diffusion equation, Eng. Anal. Bound. Elem., 92 (2018), 108–113. https://doi.org/10.1016/j.enganabound.2017.10.021 doi: 10.1016/j.enganabound.2017.10.021
![]() |
[37] |
V. R. Hosseini, E. Shivanian, W. Chen, Local radial point interpolation (MLRPI) method for solving time fractional diffusion-wave equation with damping, J. Comput. Phys., 312 (2016), 307–332. https://doi.org/10.1016/j.jcp.2016.02.030 doi: 10.1016/j.jcp.2016.02.030
![]() |
[38] |
L. Li, D. Xu, M. Luo, Alternating direction implicit Galerkin finit element method for the two-dimensional fractional diffusion-wave quation, J. Comput. Phys., 255 (2013), 471–485. https://doi.org/10.1016/j.jcp.2013.08.031 doi: 10.1016/j.jcp.2013.08.031
![]() |
[39] |
X. Cao, H. Liu, Determining a fractional Helmholtz equation with unknown source and scattering potential, Commun. Math. Sci., 17 (2019), 1861–1876. https://doi.org/10.4310/CMS.2019.v17.n7.a5 doi: 10.4310/CMS.2019.v17.n7.a5
![]() |
[40] |
X. Cao, Y. Lin, H. Liu, Simultaneously recovering potentials and embedded obstacles for anisotropic fractional Schrödinger operators, Inverse Probl. Imag., 13 (2019), 197–210. https://doi.org/10.3934/ipi.2019011 doi: 10.3934/ipi.2019011
![]() |
[41] |
Z. Bai, H. Diao, H. Liu, Q. Meng, Stable determination of an elastic medium scatterer by a single far-field measurement and beyond, Calc. Var. Partial Differ. Equ., 61 (2022), 170–223. https://doi.org/10.1007/s00120-022-01787-7 doi: 10.1007/s00120-022-01787-7
![]() |
[42] |
S. Yang, Y. Liu, H. Liu, C. Wang, Numerical methods for semilinear fractional diffusion equations with time delay, Adv. Appl. Math. Mech., 14 (2022), 56–78. https://doi.org/10.4208/aamm.OA-2020-0387 doi: 10.4208/aamm.OA-2020-0387
![]() |
[43] |
D. Baleanu, A. Jajarmi, M. Hajipour, On the nonlinear dynamical systems within the generalized fractional derivatives with Mittag-Leffler kernel, Nonlinear Dyn., 94 (2018), 397–414. https://doi.org/10.1007/s11071-018-4367-y doi: 10.1007/s11071-018-4367-y
![]() |
[44] | Z. E. A. Fellah, C. Depollier, M. Fellah, Application of fractional calculus to the sound waves propagation in rigid porous materials: validation via ultrasonic measurements, Acta Acust. United Ac., 88 (2002), 34–39. |
[45] |
J. Singh, D. Kumar, D. Baleanu, S. Rathore, On the local fractional wave equation in fractal strings, Math. Methods Appl. Sci., 42 (2019), 1588–1595. https://doi.org/10.1002/mma.5458 doi: 10.1002/mma.5458
![]() |
[46] |
X. J. Yang, T. A. Machado, D. Baleanu, Exact traveling-wave solution for local fractional Boussinesq equation in fractal domain, Fractals, 25 (2017), 17400060. https://doi.org/10.1142/S0218348X17400060 doi: 10.1142/S0218348X17400060
![]() |
[47] |
D. Kumar, F. Tchier, J. Singh, D. Baleanu, An efficient computational technique for fractal vehicular traffic flow, Entropy, 20 (2018), 259. https://doi.org/10.3390/e20040259 doi: 10.3390/e20040259
![]() |
[48] |
M. Hajipour, A. Jajarmi, D. Baleanu, H. S. Sun, On an accurate discretization of variable-order fractional reaction-diffusion equation, Commun. Nonlinear Sci. Numer. Simul., 69 (2019), 119–133. https://doi.org/10.1016/j.cnsns.2018.09.004 doi: 10.1016/j.cnsns.2018.09.004
![]() |
[49] |
R. Meng, D. Yin, C. S. Drapaca, Variable-order fractional description of compression deformation of amorphous glassy polymers, Comput. Mech., 64 (2019), 163–171. https://doi.org/10.1007/s00466-018-1663-9 doi: 10.1007/s00466-018-1663-9
![]() |
[50] |
A. Jajarmi, D. Baleanu, A new fractional analysis on the interaction of HIV with CD4+ T-cells, Chaos Solitons Fract., 113 (2018), 221–229. https://doi.org/10.1016/j.chaos.2018.06.009 doi: 10.1016/j.chaos.2018.06.009
![]() |
[51] |
D. Baleanu, A. Jajarmi, E. Bonyah, M. Hajipour, New aspects of poor nutrition in the life cycle within the fractional calculus, Adv. Differ. Equ., 2018 (2018), 230. https://doi.org/10.1186/s13662-018-1684-x doi: 10.1186/s13662-018-1684-x
![]() |
[52] |
A. R. Shamasneh, H. A. Jalab, S. Palaiahnakote, U. H. Obaidellah, R. W. Ibrahim, M. T. El-Melegy, A new local fractional entropy-based model for kidney MRI image enhancement, Entropy, 20 (2018), 344. https://doi.org/10.3390/e20050344 doi: 10.3390/e20050344
![]() |
Method | Each iteration | After convergence | ||
(+/-) | (×/÷) | (+/-) | (×/÷) | |
S(FPCN) | (16+3k)λ2 | (8+2k)λ2 | - | - |
R(FPCN) | (16+3k)λ2+12 | (8+2k)λ2+12 | (16+3k)λ2−12 | (8+2k)λ2−12 |
Methods | Total operations |
S(FPCN) | (24+5k)λ2∗Ite |
R(FPCN) | (12+2.5k)(λ2+1)∗Ite+(12+2.5k)(λ2−1) |
α=1.25 | |||||||
Δt | h−1 | Method | Time (s) | Iteration | Ave error | Max error | Total operations |
1/4 | 4 | S(FPCN) | 0.04680 | 12 | 1.23889×10−2 | 1.73310×10−2 | 4,752 |
R(FPCN) | 0.03120 | 7 | 1.39199×10−2 | 2.18245×10−2 | 1,716 | ||
1/8 | 8 | S(FPCN) | 0.99840 | 26 | 1.02852×10−3 | 1.99684×10−3 | 81,536 |
R(FPCN) | 0.37440 | 15 | 1.06050×10−3 | 2.05820×10−3 | 25,536 | ||
1/12 | 12 | S(FPCN) | 6.95764 | 38 | 2.00135×10−4 | 4.56704×10−4 | 386,232 |
R(FPCN) | 2.37122 | 22 | 2.17520×10−4 | 4.78263×10−4 | 117,768 | ||
1/16 | 16 | S(FPCN) | 27.2222 | 49 | 3.99971×10−5 | 1.24839×10−4 | 1,146,600 |
R(FPCN) | 9.09486 | 28 | 5.90195×10−5 | 1.53416×10−4 | 340,704 | ||
α=1.50 | |||||||
Δt | h−1 | Method | Time (s) | Iteration | Ave error | Max error | Total operations |
1/4 | 4 | S(FPCN) | 0.03120 | 11 | 1.44048×10−2 | 2.05458×10−2 | 4,356 |
R(FPCN) | 0.015600 | 7 | 1.60035×10−2 | 2.55940×10−2 | 1,716 | ||
1/8 | 8 | S(FPCN) | 0.79560 | 21 | 1.10424×10−3 | 2.18167×10−3 | 65,856 |
R(FPCN) | 0.32760 | 13 | 1.13615×10−3 | 2.28299×10−3 | 22,336 | ||
1/12 | 12 | S(FPCN) | 5.36643 | 30 | 2.04407×10−4 | 4.88386×10−4 | 304,920 |
R(FPCN) | 1.91881 | 18 | 2.23152×10−4 | 5.14603×10−4 | 97,272 | ||
1/16 | 16 | S(FPCN) | 20.5921 | 38 | 4.79959×10−5 | 1.40112×10−4 | 889,200 |
R(FPCN) | 7.19165 | 23 | 6.11451×10−5 | 1.58162×10−4 | 281,944 | ||
α=1.75 | |||||||
Δt | h−1 | Method | Time (s) | Iteration | Ave error | Max error | Total operations |
1/4 | 4 | S(C-N) | 0.04680 | 10 | 1.96933×10−2 | 2.84240×10−2 | 3,960 |
R(C-N) | 0.03120 | 6 | 2.17247×10−2 | 3.51605×10−2 | 1,496 | ||
1/8 | 8 | S(C-N) | 0.68640 | 18 | 1.54832×10−3 | 3.18035×10−3 | 56,376 |
R(C-N) | 0.29640 | 11 | 1.58320×10−3 | 3.30433×10−3 | 19,136 | ||
1/12 | 12 | S(C-N) | 4.14963 | 23 | 3.08254×10−4 | 7.36312×10−4 | 233,772 |
R(C-N) | 1.70041 | 15 | 3.20382×10−4 | 7.54646×10−4 | 81,900 | ||
1/16 | 16 | S(C-N) | 15.0697 | 27 | 9.07687×10−5 | 2.41195×10−4 | 631,800 |
R(C-N) | 5.52244 | 17 | 9.91180×10−5 | 2.53874×10−4 | 211,432 |
h/τ | α = 1.25 | α = 1.50 | α = 1.75 | ||||
h | τ | L2 norm | γ1−order | L2 norm | γ1−order | L2 norm | γ1−order |
π60 | 1/25 | 5.85372×10−4 | - | 5.86342×10−4 | - | 7.97263×10−5 | - |
1/50 | 3.47837×10−4 | 1.3692 | 3.09532×10−4 | 1.7518 | 4.24843×10−5 | 1.8663 | |
1/75 | 7.94632×10−5 | 1.5861 | 8.95312×10−5 | 1.8703 | 4.57934×10−6 | 1.9030 | |
1/100 | 4.93682×10−5 | 1.7938 | 5.97534×10−5 | 1.9094 | 2.84623×10−6 | 1.9986 |
h/τ | α = 1.25 | α = 1.50 | α = 1.75 | ||||
τ | h | L2 norm | γ2−order | L2 norm | γ2−order | L2 norm | γ2−order |
0.01 | π20 | 9.76342×10−4 | - | 8.75324×10−5 | - | 7.41940×10−5 | - |
π40 | 5.86453×10−4 | 1.5563 | 5.85309×10−5 | 1.7610 | 4.06432×10−5 | 1.9132 | |
π60 | 5.45790×10−5 | 1.6734 | 2.07536×10−5 | 1.8310 | 6.06421×10−6 | 1.9976 | |
π80 | 3.97521×10−5 | 1.7859 | 4.68310×10−6 | 1.9928 | 8.75312×10−7 | 2.0001 |
Method | Each iteration | After convergence | ||
(+/-) | (×/÷) | (+/-) | (×/÷) | |
S(FPCN) | (16+3k)λ2 | (8+2k)λ2 | - | - |
R(FPCN) | (16+3k)λ2+12 | (8+2k)λ2+12 | (16+3k)λ2−12 | (8+2k)λ2−12 |
Methods | Total operations |
S(FPCN) | (24+5k)λ2∗Ite |
R(FPCN) | (12+2.5k)(λ2+1)∗Ite+(12+2.5k)(λ2−1) |
α=1.25 | |||||||
Δt | h−1 | Method | Time (s) | Iteration | Ave error | Max error | Total operations |
1/4 | 4 | S(FPCN) | 0.04680 | 12 | 1.23889×10−2 | 1.73310×10−2 | 4,752 |
R(FPCN) | 0.03120 | 7 | 1.39199×10−2 | 2.18245×10−2 | 1,716 | ||
1/8 | 8 | S(FPCN) | 0.99840 | 26 | 1.02852×10−3 | 1.99684×10−3 | 81,536 |
R(FPCN) | 0.37440 | 15 | 1.06050×10−3 | 2.05820×10−3 | 25,536 | ||
1/12 | 12 | S(FPCN) | 6.95764 | 38 | 2.00135×10−4 | 4.56704×10−4 | 386,232 |
R(FPCN) | 2.37122 | 22 | 2.17520×10−4 | 4.78263×10−4 | 117,768 | ||
1/16 | 16 | S(FPCN) | 27.2222 | 49 | 3.99971×10−5 | 1.24839×10−4 | 1,146,600 |
R(FPCN) | 9.09486 | 28 | 5.90195×10−5 | 1.53416×10−4 | 340,704 | ||
α=1.50 | |||||||
Δt | h−1 | Method | Time (s) | Iteration | Ave error | Max error | Total operations |
1/4 | 4 | S(FPCN) | 0.03120 | 11 | 1.44048×10−2 | 2.05458×10−2 | 4,356 |
R(FPCN) | 0.015600 | 7 | 1.60035×10−2 | 2.55940×10−2 | 1,716 | ||
1/8 | 8 | S(FPCN) | 0.79560 | 21 | 1.10424×10−3 | 2.18167×10−3 | 65,856 |
R(FPCN) | 0.32760 | 13 | 1.13615×10−3 | 2.28299×10−3 | 22,336 | ||
1/12 | 12 | S(FPCN) | 5.36643 | 30 | 2.04407×10−4 | 4.88386×10−4 | 304,920 |
R(FPCN) | 1.91881 | 18 | 2.23152×10−4 | 5.14603×10−4 | 97,272 | ||
1/16 | 16 | S(FPCN) | 20.5921 | 38 | 4.79959×10−5 | 1.40112×10−4 | 889,200 |
R(FPCN) | 7.19165 | 23 | 6.11451×10−5 | 1.58162×10−4 | 281,944 | ||
α=1.75 | |||||||
Δt | h−1 | Method | Time (s) | Iteration | Ave error | Max error | Total operations |
1/4 | 4 | S(C-N) | 0.04680 | 10 | 1.96933×10−2 | 2.84240×10−2 | 3,960 |
R(C-N) | 0.03120 | 6 | 2.17247×10−2 | 3.51605×10−2 | 1,496 | ||
1/8 | 8 | S(C-N) | 0.68640 | 18 | 1.54832×10−3 | 3.18035×10−3 | 56,376 |
R(C-N) | 0.29640 | 11 | 1.58320×10−3 | 3.30433×10−3 | 19,136 | ||
1/12 | 12 | S(C-N) | 4.14963 | 23 | 3.08254×10−4 | 7.36312×10−4 | 233,772 |
R(C-N) | 1.70041 | 15 | 3.20382×10−4 | 7.54646×10−4 | 81,900 | ||
1/16 | 16 | S(C-N) | 15.0697 | 27 | 9.07687×10−5 | 2.41195×10−4 | 631,800 |
R(C-N) | 5.52244 | 17 | 9.91180×10−5 | 2.53874×10−4 | 211,432 |
h/τ | α = 1.25 | α = 1.50 | α = 1.75 | ||||
h | τ | L2 norm | γ1−order | L2 norm | γ1−order | L2 norm | γ1−order |
π60 | 1/25 | 5.85372×10−4 | - | 5.86342×10−4 | - | 7.97263×10−5 | - |
1/50 | 3.47837×10−4 | 1.3692 | 3.09532×10−4 | 1.7518 | 4.24843×10−5 | 1.8663 | |
1/75 | 7.94632×10−5 | 1.5861 | 8.95312×10−5 | 1.8703 | 4.57934×10−6 | 1.9030 | |
1/100 | 4.93682×10−5 | 1.7938 | 5.97534×10−5 | 1.9094 | 2.84623×10−6 | 1.9986 |
h/τ | α = 1.25 | α = 1.50 | α = 1.75 | ||||
τ | h | L2 norm | γ2−order | L2 norm | γ2−order | L2 norm | γ2−order |
0.01 | π20 | 9.76342×10−4 | - | 8.75324×10−5 | - | 7.41940×10−5 | - |
π40 | 5.86453×10−4 | 1.5563 | 5.85309×10−5 | 1.7610 | 4.06432×10−5 | 1.9132 | |
π60 | 5.45790×10−5 | 1.6734 | 2.07536×10−5 | 1.8310 | 6.06421×10−6 | 1.9976 | |
π80 | 3.97521×10−5 | 1.7859 | 4.68310×10−6 | 1.9928 | 8.75312×10−7 | 2.0001 |