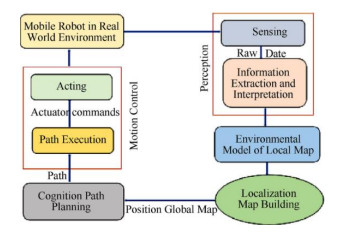
Harmonic potential fields are commonly used as guidance in a global approach for self-directed robot pathfinding. These harmonic potentials are generated using Laplace's equation solutions. The computation of these harmonic potentials often requires the use of immense amounts of computing resources. This study introduces a numerical technique called Rotated Block Accelerated Over-Relaxation (AOR), also known as Explicit Decoupled Group AOR (EDGAOR), to deal with pathfinding problem. Several robot navigation simulations were performed in a static, structured, known indoor environment to validate the efficiency of the suggested approach. The paths generated by the simulations are shown using several different starting and target positions. The performance of the proposed approach in computing harmonic potentials for solving pathfinding problems is also discussed.
Citation: A'qilah Ahmad Dahalan, Azali Saudi. Pathfinding algorithm based on rotated block AOR technique in structured environment[J]. AIMS Mathematics, 2022, 7(7): 11529-11550. doi: 10.3934/math.2022643
[1] | A'qilah Ahmad Dahalan, Azali Saudi . An iterative technique for solving path planning in identified environments by using a skewed block accelerated algorithm. AIMS Mathematics, 2023, 8(3): 5725-5744. doi: 10.3934/math.2023288 |
[2] | Li-Tao Zhang, Xian-Yu Zuo, Shi-Liang Wu, Tong-Xiang Gu, Yi-Fan Zhang, Yan-Ping Wang . A two-sweep shift-splitting iterative method for complex symmetric linear systems. AIMS Mathematics, 2020, 5(3): 1913-1925. doi: 10.3934/math.2020127 |
[3] | Peng Lv . Structured backward errors analysis for generalized saddle point problems arising from the incompressible Navier-Stokes equations. AIMS Mathematics, 2023, 8(12): 30501-30510. doi: 10.3934/math.20231558 |
[4] | Dongmei Yu, Yiming Zhang, Cairong Chen, Deren Han . A new relaxed acceleration two-sweep modulus-based matrix splitting iteration method for solving linear complementarity problems. AIMS Mathematics, 2023, 8(6): 13368-13389. doi: 10.3934/math.2023677 |
[5] | Fouad Mohammad Salama, Nur Nadiah Abd Hamid, Norhashidah Hj. Mohd Ali, Umair Ali . An efficient modified hybrid explicit group iterative method for the time-fractional diffusion equation in two space dimensions. AIMS Mathematics, 2022, 7(2): 2370-2392. doi: 10.3934/math.2022134 |
[6] | Yuanheng Wang, Bin Huang, Bingnan Jiang, Tiantian Xu, Ke Wang . A general hybrid relaxed CQ algorithm for solving the fixed-point problem and split-feasibility problem. AIMS Mathematics, 2023, 8(10): 24310-24330. doi: 10.3934/math.20231239 |
[7] | Huiling Wang, Nian-Ci Wu, Yufeng Nie . Two accelerated gradient-based iteration methods for solving the Sylvester matrix equation AX + XB = C. AIMS Mathematics, 2024, 9(12): 34734-34752. doi: 10.3934/math.20241654 |
[8] | Zhensheng Yu, Peixin Li . An active set quasi-Newton method with projection step for monotone nonlinear equations. AIMS Mathematics, 2021, 6(4): 3606-3623. doi: 10.3934/math.2021215 |
[9] | Laiq Zada, Rashid Nawaz, Sumbal Ahsan, Kottakkaran Sooppy Nisar, Dumitru Baleanu . New iterative approach for the solutions of fractional order inhomogeneous partial differential equations. AIMS Mathematics, 2021, 6(2): 1348-1365. doi: 10.3934/math.2021084 |
[10] | Nunthakarn Boonruangkan, Pattrawut Chansangiam . Convergence analysis of a gradient iterative algorithm with optimal convergence factor for a generalized Sylvester-transpose matrix equation. AIMS Mathematics, 2021, 6(8): 8477-8496. doi: 10.3934/math.2021492 |
Harmonic potential fields are commonly used as guidance in a global approach for self-directed robot pathfinding. These harmonic potentials are generated using Laplace's equation solutions. The computation of these harmonic potentials often requires the use of immense amounts of computing resources. This study introduces a numerical technique called Rotated Block Accelerated Over-Relaxation (AOR), also known as Explicit Decoupled Group AOR (EDGAOR), to deal with pathfinding problem. Several robot navigation simulations were performed in a static, structured, known indoor environment to validate the efficiency of the suggested approach. The paths generated by the simulations are shown using several different starting and target positions. The performance of the proposed approach in computing harmonic potentials for solving pathfinding problems is also discussed.
The surge of mobile robot navigation technology nowadays is rising by virtue of human desire. It is not only limited to transportation, but it is also very useful in other problems such as manufacturing, industrial, and so on. In general, the ability of a robot to navigate autonomously is a prerequisite and foundation for the development of intelligent robots. Pathfinding algorithms must be both efficient and practical to ensure the proper performance of mobile robots' various tasks.
Figure 1 illustrates the key phases related to the operation of a robot. The problem of robot pathfinding can be divided into four categories:
● Localization: Where do I go?
● Pathfinding: Where was I supposed to go? Which is the best path to be reached?
● Motion control: How can I move?
● Cognitive mapping: Where was I? What should I remember?
There is no doubt that pathfinding is a major issue in the navigation process. The pathfinding algorithm allows the robot to choose and identify the right path within the configuration space. The two major components of global path planning are the robot's representation of the world in configuration space and its ability to execute the algorithm. These two components are closely linked and have a significant impact on one another in choosing the optimum path and time for the robot to move around in the configuration space [2].
One of most difficult problem in robot applications is the construction of robust autonomous motion planning. To create a truly autonomous mobile robot, it must be able to compute its trajectory between locations quickly and accurately while also avoiding collisions with surrounding objects. The goal of this study is to use harmonic potential values to simulate point-robot pathfinding in a specified area using heat transfer theory. Laplace's equation [3] is used to model the heat transfer problem. Harmonic functions are the solutions to Laplace's equation, and they represent temperature values in the configuration space that will be exploited to simulate path generation.
Iterative methods are another way of generating harmonic functions by numerically solving the Laplace equation under Dirichlet or Neumann boundary conditions and discretizing the two-dimensional environment into a grid of discrete points [4,5,6,7,8]. Harmonic functions obey the min-max principle, thus there can be no spontaneous creation of local minima within the solution region. As a result, the only critical points that can occur are saddle points. A search in the neighbourhood of such a critical point would then reveal the exit from that point. Furthermore, any deviation or disturbance of route from such points results in a smooth path everywhere. The discretization of the Laplace equation yields a sparse linear system, in which the computing costs of iterative approaches are generally significantly greater than the analytical technique. The computational complexity is determined by the discretization resolution; hence, if a greater region of obstacles is occupied, fewer calculations and storage are required. Laplace's solutions can be numerically derived using the finite difference method. Laplace' equation is transformed into a linear system, and most of the matrix elements are zero when written in matrix notation. The resultant matrix is often extremely large and sparse, and typically requires very large computing resources. This study thereby solved the linear system by means of the iterative approach, since it avoids the need to store a very large matrix in memory.
For pathfinding method in this study, the Gradient Descent Search (GDS) technique utilizes the Laplacian potential values obtained using the iterative methods discussed in the next section as guidance during the path construction. From the current point, the GDS method examines the potential values of its eight neighbouring points and simply picks the node with the lowest Laplacian potential value. This procedure is repeated until it reaches the target point [4,9,10,11].
Recently, a real-time path planning method using harmonic functions based on the Proper Generalized Decomposition (PGD) has been suggested [12,13]. The proposed method was tested on two structured environments: an L-shaped corridor and a bug trap planning environment. The experiments showed encouraging results, in which a physical Lego Mindstorms robot was tested to validate the results. The experiments, however, were only conducted in a small and relatively simple environment. It was also stated that the offline phase of the PGD-based framework on a square environment of 150 x 150 nodes would take 14 hours using a high-performance computer to obtain the solution for every start and goal vehicle position. However, the online phase was faster and required minimal processing time.
Most path planning methods in the literature that are based on the harmonic potentials rely on the used of the standard Laplacian operator. This study presents several to evaluate the capability of a rotated block Laplacian operator, also known as Explicit Decoupled Group (EDG), in the computation of harmonic potentials. A linear system of rotating approximation equations is developed and computed via EDG Accelerated Over Relaxation (EDGAOR) by employing the rotated block operator. The results of the implemented EDGAOR algorithm are compared and analysed in terms of the number of iterations and CPU time against the existing approaches. The findings showed that the EDGAOR outperformed the existing methods.
Global planning stores the map of its environmental and then utilises that map to create a feasible path. Accordingly, global pathfinding seeks to search for a collision-free route by which the robot may move from its original position toward its intended position without colliding with any obstacles. Figure 2 illustrates the global pathfinding framework. A model of the robot workspace that includes position of the robot, obstacles and vacant spaces, must first be made. The vacant area in the workspace is then discretized to form a graph representing the connectivity of the space. The path finding algorithm is then employed to determine a feasible route for the robot to trace using the graph of connectivity in order to reach the goal.
The process of pathfinding construction in this study comprises the following steps:
Begin
Step 1. Create the map of the robot's environment.
Step 2. Establish the formulation and modelling of the iterative schemes using finite difference method.
Step 3. Develop and implement the algorithms of the proposed iterative schemes.
Step 4. Run the numerical simulations to obtain the solutions.
Step 5. Conduct the performances evaluation and complexity analysis of the algorithms.
End
Let Laplace's equation below, be satisfied by a harmonic function in a region Ω⊂ℜn
∇2U=n∑i=1∂2U∂x2i=0 | (1) |
with xi as the i-th coordinates in the Cartesian and the dimension is n. For the robot path creation, the region boundary is made up of walls, obstacle boundaries, and the target location. Since the harmonic potential complies with the min-max criterion [4] in the specified domain, it does not have local minima except the target position, and generally produces smooth path, thus making harmonic potential approach a feasible and very attractive option for robot navigation. The Laplace equation is commonly solved using standard approaches [14,15,16]. In this study, Eq (1) was solved using fast rotated block iterative technique to speed up the computational execution.
A global approach is used to compute harmonic potentials of the robot workspace by employing the analogy of heat distribution in areas in which all obstacles and boundaries (with the highest potential value) represent heat sources and target position (with the highest potential value) as heat sink. Laplace's equation is used to model this heat transfer activity and is solved numerically to obtain the heat distribution that represents the harmonic potential for each node in the graph. Utilizing the property of heat distribution that flow from high temperature to lower temperature, a gradient search can be used to generate path from any start point with high potential value to target position with the lowest potential value. The pathfinding algorithm utilizes the gradient descent search to determine a feasible route for the robot to navigate the workspace safely from the start point to reach the goal position.
This research seeks to replicate the aforementioned paradigm for pathfinding, describing the solution of Laplace's Eq (1) through the analogy of temperature (for the potential) and heat flow (for the pathway). The experiment is conducted with a two-dimensional domain with various forms of obstacles, including the inner and external walls. To solve Eq (1) and obtain the potential values for each node, the EDGAOR iterative approach is utilised. For performance comparison purposes, the existing Explicit Group Successive Over Relaxation (EGSOR), Explicit Group AOR (EGAOR), and EDGSOR were also investigated.
Abdullah [17] was the first to present the EDG iterative approach that employed block half-sweep technique on rotated grid to solve the two-dimensional Poisson equation. This approach is also used in [18,19,20,21,22,23,24,25,26] to solve partial differential equations. A modified version of this approach was investigated for the solution of the diffusion equation [24]. Meanwhile, [25] discussed early efforts on integrating SOR with other techniques. In the robotics literature, the classic GS [9] and SOR [3,26] algorithms have been utilised to solve Eq (1). To obtain the solutions of Laplace's Eq (1), this work developed a robust numerical solver using rotated block iteration approach that employ half-sweep technique.
In general, the EDG technique computes only half of all points in the region, resulting in a significant reduction in total processing time. The application of EDG iteration, which examines the interior grid points (black grid points), is compared to the conventional full-sweep (alternative name is Explicit Group, EG) technique, which iterates every grid point in the region (see Figure 3). The half-sweep iteration technique was first used in robotics in [18]. In this study, the EDG iteration is essentially subjected to the rotated 5-points finite difference approximation (5-FDA) equation to solve Eq (1). The key advantage of this iterative technique is that it reduces the computing complexity by evaluating only a portion of the total number of node grid points. Figure 4 depicts the computational molecules of the iterative techniques of full-sweep (FS) and half-sweep (HS).
All the algorithms in the Block iterative techniques family indicate that acquiring four Laplacian potentials per calculation speeds up the evaluation. In the event of computing groups of points that are close to the boundary, it will be managed as a set of a single point and two points, as illustrated in Figure 3. After all, for Block iterative techniques, the approximate values of the residual node points are computed directly by using direct methods [17,27]. It should be emphasised that all algorithms in this study are executed so long as the results meet a predefined convergence criteria denoted as ε. The stopping criterion in this study is based on ‖u(k+1)−u(k)‖⩽ε.
In general, the executions of all iterative schemes are imposed on black node points in Figure 3 until the convergence test criterion is satisfied. As depicted in Figure 3 (left), all formulations employing the EG iterative techniques compute a group of four nodes at once during the iteration process (except for certain cases near the boundary). Meanwhile, the EDG is derived from a rotated 5-FDA [17]. As shown in Figure 3 (right), the solution domain for the EDG method is divided into two types of node points, ⋅ and ∘. The solutions of each node point group can be executed by pairing the points of an equal type and can be carried out independently for each of the pairings. Due to this independency, almost half of the iteration over the solution domain is performed in either type of the points, hence saves the performance time. Furthermore, the direct method [28] is computed via Eq (3), as demonstrated in the next section, to compute sets that are close to the border, i.e., with only one or two points.
Consider the 2-dimensional equation of Laplace in Eq (1), which is rewritten as
∇2U=∂2U∂x2+∂2U∂y2=0 | (2) |
The Laplacian operator is denoted as ∇2. To solve Eq (2), the system needs to be discretised via finite difference method before it can be computed efficiently using the numerical method. The simplest 5-FDA for the 2D Laplacian, ∇2, is given as
∇2U(x,y)≈u(x+h,y)+u(x−h,y)+u(x,y+h)+u(x,y−h)−4u(x,y)h2, |
where U is a function to satisfy the equation of Laplace, u is the potential node at point (x,y), and h is the distance between node points for each direction. The above equation can be termed as a second-order equation since its error term is of order two, h2. By using the central difference formula, it will then be deduced using Taylor series approximation into the second derivative, and be expressed as follows (which represents FS iteration case)
∇2U(x,y)=1h2[u(x+h,y)+u(x−h,y)+u(x,y+h)+u(x,y−h)−4u(x,y)]. |
Meanwhile, the approximation that is based on the cross-orientation operator is formed upon rotating the x−y plane by 45∘ in the clockwise direction [29,30]. This produces the rotated (skewed) 5-point approximation, also known as HS iteration, denoted as
∇2U(x,y)=12h2[u(x−h,y−h)+u(x+h,y−h)+u(x−h,y+h)+u(x+h,y+h)−4u(x,y)]. |
This also can be shown in matrix form as
∇2U=1h2[0101−41010] |
and
∇2U=12h2[1010−40101], |
for FS and HS iteration cases respectively.
By letting Uij approaches the solution of u for Laplace's Eq (2) along the grid point (xi,yj), the discretization of these Laplace equation via conventional 5-point stencil is
Ui−1,j+Ui+1,j+Ui,j−1+Ui,j+1−4Ui,j=0, | (3) |
for the FS case. Meanwhile, for HS iteration holds that
Ui−1,j−1+Ui−1,j+1+Ui+1,j−1+Ui+1,j+1−4Ui,j=0. | (4) |
Then, by employing Eqs (3) and (4) to the 2-dimensional Laplace's problem based on Eq (2), it will eventually generate a linear system. This system can be formed in matrix notation and produce large and sparse matrices. The aforementioned linear system is displayed as
Au=b. | (5) |
The coefficient A and b are both identified, where A is in matrix form and b is a vector. Whereas coefficient u is an unknown vector. For the FS iteration case, the matrix A is said to be a block triangle matrix with order (N−1)×(N−1), and signified as
A=[TIITIITI⋱⋱⋱ITIIT](N−1)×(N−1). |
From matrix A, each element of block T is represented as
T=[−411−411−41⋱⋱⋱1−411−4](N−1)×(N−1), |
while I is an identity matrix with the same order. Meanwhile, coefficients u and b from Eq (5) may be defined as
uk=[u1,ku2,k⋯uN−2,kuN−1,k]T,bk=[b1,kb2,k⋯bN−2,kbN−1,k]T, |
where k=1,2,…,N−2,N−1.
Meanwhile, for the HS iteration case, matrix A from the linear system Eq (5) can be described as
A=[TPQSQPTPQSQ⋱⋱⋱QSQPT](N−1)×(N−1), |
where
T=[−400−400−400−40⋱⋱⋱0−400−4](N2)×(N2), |
S=[−400−400−400−40⋱⋱⋱0−400−4](N2−1)×(N2−1), |
P=[10110110⋱⋱⋱110111](N2)×(N2−1), |
Q=[11011011⋱⋱⋱011011](N2−1)×(N2). |
Vectors u and b from Eq (5) for the HS iteration case are defined respectively as
ui=[u1,iu3,i⋯uN−3,iuN−1,i]T,uj=[u2,ju4,j⋯uN−4,juN−2,j]T,bi=[b1,ib3,i⋯bN−3,ibN−1,i]T,bj=[b2,jb4,j⋯bN−4,jbN−2,j]T, |
with i=1,3,5,…,N−3,N−1 and j=2,4,6,…,N−4,N−2.
As stated previously, the system of linear Eq (5) generates a sparse and large matrix. Consequently, the iterative methods can be solved using either Point or Block iterative techniques because the proposed methods are ideal for solving this problem [17]. Hence, considering the finite difference of Eqs (3) and (4), the Gauss-Seidel iterative schemes for FS and HS cases can be rewritten and denoted respectively as follows,
U(k+1)i,j=14[U(k+1)i−1,j+U(k)i+1,j+U(k+1)i,j−1+U(k)i,j+1], | (6) |
U(k+1)i,j=14[U(k+1)i−1,j−1+U(k+1)i+1,j−1+U(k)i−1,j+1+U(k)i+1,j+1]. | (7) |
As previously stated, this study concentrates on the family of Block iterative schemes for the proposed solvers, i.e., SOR and AOR, using EG and EDG iterative methods. With the intention of implementing the Block scheme, the Point iteration scheme for corresponding methods is required. The FSSOR method is, in fact, the standard SOR method that applies traditional FS iteration via a 5-point discretization scheme [31]. The iterative scheme for the Point SOR method is given below. It is referring to Eq (6), by inserting a weighted parameter, ω via SOR [31].
U(k+1)i,j=ω4[U(k+1)i−1,j+U(k)i+1,j+U(k+1)i,j−1+U(k)i,j+1]+(1−ω)U(k)i,j. | (8) |
Meanwhile, the HSSOR iterative method is formulated by employing the HS concept [17]. From Eq (7), the Point rotated SOR iterative scheme is given as
U(k+1)i,j=ω4[U(k+1)i−1,j−1+U(k+1)i+1,j−1+U(k)i−1,j+1+U(k)i+1,j+1]+(1−ω)U(k)i,j. | (9) |
The difference between Eqs (6) and (7) to Eqs (8) and (9) are clearly on the addition of weighted parameters ω. The basic idea of the SOR iterative method comes from Gauss-Seidel iterative schemes with weighted parameters [32,33]. Note that if the value of ω is equal to 1, then the SOR scheme is effectively simplified to the standard Gauss-Seidel scheme.
The AOR method belongs to the family of over-relaxation methods. It is a generalisation of the SOR method with two parameters. For this study, these two relaxation parameters are denoted as r and ω. Both parameters can be utilised to generate iterative methods that can speed up the convergence rates, and AOR are more flexible and suitable than any other methods in this family. To perform the AOR iterative scheme proposed in [34], replacing u(k+1)i−1,j−1 and u(k+1)i+1,j−1 with u(k)i−1,j−1 and u(k)i+1,j−1, respectively, as well as appending the nodes of r(u(k+1)i−1,j−1−u(k)i−1,j−1)4 and r(u(k+1)i+1,j−1−u(k)i+1,j−1)4 into Eq (8) are required. Thus, the Point AOR iterative scheme is
U(k+1)i,j=r4[U(k+1)i−1,j−U(k)i−1,j+U(k+1)i,j−1−U(k)i,j−1]+ω4[U(k)i−1,j+U(k)i+1,j+U(k)i,j−1+U(k)i,j+1]+(1−ω)U(k)i,j. | (10) |
Whereas, based on the explanation above and implementing every replacement of terms into Eq (9), the rotated Point AOR schemes are stated as
U(k+1)i,j=r4[U(k+1)i−1,j−1−U(k)i−1,j−1+U(k+1)i+1,j−1−U(k)i+1,j−1]+ω4[U(k)i−1,j−1+U(k)i+1,j−1+U(k)i−1,j+1+U(k)i+1,j+1]+(1−ω)U(k)i,j. | (11) |
Based on Laplace Eq (2), all of the proposed iterative techniques simply replace each node's value from the average of four neighbours of the corresponding node. The node values that indicate the boundaries, obstacles, and target position in this study remains constant.
To facilitate the formulation of the Block EGSOR method, observe a set of four node points (4×4) in Figure 3 (left). By examining Eqs (3) and (8), the scheme generally can be expressed as [17,28,30].
[4−1−10−140−1−104−10−1−14][Ui,jUi+1,jUi,j+1Ui+1,j+1]=[S1S2S3S4], | (12) |
where
S1=Ui−1,j+Ui,j−1,S2=Ui+2,j+Ui+1,j−1,S3=Ui−1,j+1+Ui,j+2,S4=Ui+2,j+1+Ui+1,j+2. |
Apparently, Eq (12) may also be converted into the form of a system of linear Eq (5). It will then be translated upon establishing the inverse of a matrix to coefficient A, as shown below
[Ui,jUi+1,jUi,j+1Ui+1,j+1]=124[6S1+Sa6S2+Sb6S3+Sb6S4+Sa], | (13) |
where
Sa=2(S2+S3)+S1+S4,Sb=2(S1+S4)+S2+S3. |
Now, the Block EGSOR scheme for Eq (13) is indicated as
[Ui,jUi+1,jUi,j+1Ui+1,j+1](k+1)=ω24[6S1+Sa6S2+Sb6S3+Sb6S4+Sa]+(1−ω)[Ui,jUi+1,jUi,j+1Ui+1,j+1](k). | (14) |
Meanwhile, the formulation of the Block EGAOR method also considers the node points in Figure 3 (left), but assessing the approximation equation from Eq (3) and (10). Then, the AOR block scheme of AOR is stated as [35]
[4−100−1400004−100−14][Ui,jUi+1,j+1Ui+1,jUi,j+1]=[S1S2S3S4], | (15) |
with
S1=r(U(k+1)i−1,j−U(k)i−1,j+U(k+1)i,j−1−U(k)i,j−1)+ω(U(k)i−1,j+U(k)i,j−1),S2=r(U(k+1)i+1,j−1−U(k)i+1,j−1)+ω(U(k)i+1,j−1+U(k)i+2,j),S3=r(U(k+1)i−1,j+1−U(k)i−1,j+1)+ω(U(k)i−1,j+1+U(k)i,j+2),S4=ω(U(k)i+2,j+1+U(k)i+1,j+2). |
Again, Eq (15) may also be transformed into a system of linear Eq (5) and translated as below, by initiating the inverse of matrix A
[Ui,jUi+1,j+1Ui+1,jUi,j+1]=124[6S1+Sa6S2+Sb6S3+Sb6S4+Sa], | (16) |
with
Sa=2(S2+S3)+S1+S4,Sb=2(S1+S4)+S2+S3. |
Finally, the Block EGAOR iterative scheme for Eq (16) is presented as
[Ui,jUi+1,j+1Ui+1,jUi,j+1](k+1)=ω24[6S1+Sa6S2+Sb6S3+Sb6S4+Sa]+(1−ω)[Ui,jUi+1,j+1Ui+1,jUi,j+1](k). | (17) |
The EDG approximation equation is on the basis of the cross-orientation operator, which allows it to be formed upon rotating or turning the i−j plane by 45∘ in clockwise direction. Now, to simplify the formulation of the Block EDGSOR scheme, a set of four node points (see Figure 3 (right)) is examined in order to construct a (4×4) algebraic linear system [17,27,36], expressed by Eq (15), where
S1=Ui−1,j−1+Ui−1,j+1+Ui+1,j−1,S2=Ui,j+2+Ui+2,j+2+Ui+2,j, | (18) |
and
S3=Ui,j−1+Ui+2,j−1+Ui+2,j+1,S4=Ui−1,j+Ui−1,j+2+Ui+1,j+2. | (19) |
The linear system equation in matrix form as in Eq (15), can be reduced to Eqs (18) and (19) and solved individually using two (2×2) linear algebraic equations as follows
[Ui,jUi+1,j+1]=115[4114][S1S2], | (20) |
[Ui+1,jUi,j+1]=115[4114][S3S4]. | (21) |
The Block EDGSOR scheme for both Eqs (20) and (21) can be defined independently as shown below, respectively,
[Ui,jUi+1,j+1](k+1)=ω15[4S1+S2S1+4S2]+(1−ω)[Ui,jUi+1,j+1](k), | (22) |
[Ui+1,jUi,j+1](k+1)=ω15[4S3+S4S3+4S4]+(1−ω)[Ui+1,jUi,j+1](k). | (23) |
The algorithm for Block EDGSOR iterative scheme can be implemented by using either Eq (22) or (23). Both equations can be used to obtain solution.
Applying similar approach to EGAOR, by using the rotated AOR formula (7) to the Block scheme gives rise to a new formula called EDGAOR [37]. As a result, the AOR scheme for the EDG iteration is stated as
[Ui,jUi+1,j+1](k+1)=r15[4S2S2]+ω15[4S1+S3S1+4S3]+(1−ω)[Ui,jUi+1,j+1](k), | (24) |
where
S1=U(k)i−1,j−1+U(k)i−1,j+1+U(k)i+1,j−1,S2=U(k+1)i−1,j−1+U(k+1)i−1,j+1+U(k+1)i+1,j−1−S1,S3=U(k)i,j+2+U(k)i+2,j+2+U(k)i+2,j. |
Contrasting from SOR, no generic formula exists for determining the optimum r and ω values that result in the minimal iteration counts. According to [34], the r value is often set to be close to the SOR ω value, and the numerical analysis is then repeated for a certain range of ω, with 1⩽ω⩽2. As some values do not converge in certain cases, r and ω values for each full- sweep and half-sweep cases are different in order to obtain the optimal value. The effect of complexity on discovering parameter values to overall computation does not change, since the value of each parameter is set before the execution/computation. The optimal values used throughout the experiments are listed in Table 1.
Methods | ω | r |
EGSOR | 1.82 | - |
EGAOR | 1.83 | 1.82 |
EDGSOR | 1.81 | - |
EDGAOR | 1.82 | 1.84 |
The implementation of Block EDGAOR scheme based on Eq (24) into solving the two-dimensional Laplace's problem, as expressed in Eq (2), is stated in Algorithm 1.
Algorithm 1: EDGAOR method | |
(i). | Setup the configuration space with a specified start and goal position. |
(ii). | Initialising the starting point U,ε←10−15,iteration←0. |
(iii). | Set the variables S1←U(k)i−1,j−1+U(k)i−1,j+1+U(k)i+1,j−1,S2←U(k+1)i−1,j−1+U(k+1)i−1,j+1+U(k+1)i+1,j−1−S1,S3←U(k)i,j+2+U(k)i+2,j+2+U(k)i+2,j. |
(iv). | For all non-occupied node points of type ⋅ using Eq (24), calculate U(k+1)i,j←r154S2+ω15[4S1+S3]+(1−ω)U(k)i,j,U(k+1)i+1,j+1←r15S2+ω15[S1+4S3]+(1−ω)U(k)i+1,j+1. |
(v). | Compute the remaining group of points (with one or two points) near to the boundary via direct method by using Eq (11). Then, evaluate the remaining points of type ∘ using Eq (6) U(k+1)i,j←14[U(k+1)i−1,j+U(k)i+1,j+U(k+1)i,j−1+U(k)i,j+1]. |
(vi). | Check the convergence test for ε←10−15. If yes, go to step (vii). Otherwise go back to step (iii). |
(vii). | Execute GDS to generate the path from start to goal point. |
The implementation of the EDG iterative method computes approximately half of the group of node points in the block scheme (see Figure 3) during the iteration process. Therefore, it will drastically reduce the computational complexity, i.e., approximately 50%. With the purpose of measuring the performance of these algorithms, the simulations are conducted on four configuration regions in three different sizes, i.e., 300×300, 600×600, and 900×900. Their performances are examined using three criteria:
(i). Number of iteration count,
(ii). CPU time, and
(iii). Success of pathfinding.
The number of iterations and CPU time required by each algorithm is captured when the tolerance rate of the iteration process is met. The computed Laplacian potentials are then used by the GDS algorithm to ensure that a path from the initial to the target position can be successfully created.
A variety of obstacles of various shapes are placed in the given region. In the preliminary configuration, the Dirichlet boundary condition was used, where the boundaries and obstacles are fixed at high-temperature values. The target position was placed at the lowest temperature reading, whereas no initial value was assigned at the start position. Other grid nodes remained at zero temperature. The execution had been performed using a computer, operating with a 2.50GHz processor and 8GB of RAM. The iterative procedure of numerical computation continued until the stopping condition was satisfied. The loop is supposed to be halted if potential values have not changed further and the changes within Laplacian potentials at the computation of current (k) and next (k+1) iterations are very small, i.e., 1.0−10. This level of precision was required to avoid flat areas in the solutions, often known as saddle points, which means the sudden non-existence of descending gradient patterns. As a result, the algorithm simply stops searching and gets stuck in flat areas at the lowest point. Tables 2 and 3 indicate the number of iterations along with CPU time (in seconds) for each numerical method compared in the tests. Clearly, the EDGAOR approach showed to be faster than other approaches tested. On average, the EDGAOR successfully reduced the number of iterations compared to EDGSOR by 19%, approximately 24% for EGAOR, and 33% for EGSOR. In terms of CPU time reduction, the EDGAOR averagely reduces the execution by 16%, 36%, and 40% against EDGSOR, EGAOR, and EGSOR respectively.
Techniques | N x N | |||
300 | 600 | 900 | ||
Sample 1 | EGSOR | 1258 | 5899 | 12844 |
EGAOR | 1042 | 4994 | 10928 | |
EDGSOR | 953 | 4495 | 9916 | |
EDGAOR | 766 | 3730 | 8200 | |
Sample 2 | EGSOR | 1729 | 6782 | 14874 |
EGAOR | 1610 | 6368 | 13953 | |
EDGSOR | 1324 | 5599 | 13252 | |
EDGAOR | 1188 | 4767 | 10490 | |
Sample 3 | EGSOR | 2666 | 11076 | 24519 |
EGAOR | 2480 | 10389 | 22995 | |
EDGSOR | 2035 | 10243 | 24864 | |
EDGAOR | 1835 | 7741 | 17131 | |
Sample 4 | EGSOR | 1629 | 6487 | 14194 |
EGAOR | 1392 | 5648 | 12367 | |
EDGSOR | 1238 | 5958 | 13501 | |
EDGAOR | 1116 | 4223 | 9369 |
Techniques | N x N | |||
300 | 600 | 900 | ||
Sample 1 | EGSOR | 6.88 | 163.72 | 871.66 |
EGAOR | 6.05 | 137.87 | 751.78 | |
EDGSOR | 4.34 | 110.88 | 603.41 | |
EDGAOR | 3.67 | 95.08 | 498.19 | |
Sample 2 | EGSOR | 7.67 | 199.59 | 1009.48 |
EGAOR | 8.25 | 185.36 | 926.49 | |
EDGSOR | 4.70 | 142.64 | 787.53 | |
EDGAOR | 4.17 | 122.85 | 624.48 | |
Sample 3 | EGSOR | 13.24 | 315.87 | 1602.81 |
EGAOR | 13.83 | 301.27 | 1633.35 | |
EDGSOR | 8.00 | 261.74 | 1529.74 | |
EDGAOR | 7.67 | 203.61 | 1072.61 | |
Sample 4 | EGSOR | 7.80 | 187.33 | 990.20 |
EGAOR | 7.56 | 167.65 | 891.51 | |
EDGSOR | 4.33 | 152.73 | 828.24 | |
EDGAOR | 4.53 | 109.96 | 615.38 |
After determining the configuration region's potential values, a smooth path is formed by following the potentials distribution using the gradient descent method, in which the algorithm follows the descending gradient from the initial position to the next sequential points at lower potentials until it reaches the lowest potential (the target) point. Figure 5 depicts the pathways that were efficiently generated in a given stationary environment using the numerically computed potentials distribution. Every starting location (green/square point) reached the designated target location (red/circle point), avoiding every obstacle placed in the environments. The solution can be calculated and repeated as often as desired in a stationary situation where the target point and obstacles are set. It must be recalculated only if the obstacles or the target position are changed. The trajectory of the paths can be very quick, as they involve only the gradient evaluation of precomputed Laplacian potential [9]. The flow diagram of the pathfinding technique of this study is shown in Figure 6.
The performance graphs in terms of iteration numbers (see Figure 7) and CPU time (see Figure 8) are also illustrated. By referring to both graphs, it can be seen that the EDGAOR had outperformed their corresponding methods, either in terms of iteration counts or CPU time. The EDGAOR method gave the best performance. This idea can be clearly seen in Tables 2 and 3. As we can see from the table of results, the graphs for the number of iterations as well as CPU time, shows the same pattern.
This study demonstrated that the paths can be obtained from the GDS algorithm by utilising the Laplacian potentials computed using the presented EDG iterative schemes. After succeeding in obtaining the potential values present in the environment, the path of the navigating robot can be constructed. It follows the principle of heat distribution by using the steepest descent method. The algorithms follow the temperature descent flow to the sink, which in this case is the goal point, thus providing a path line for the navigation. The path line can be generated using different starting and goal points by computing the Laplacian potentials numerically. The generated path line had successfully avoided any obstacles while navigating from the start green point to the target red point.
Harmonic functions offer a rapid approach to generating paths in a structured environment. The approach avoids the local minimum problem at some cost in computational speed for very large environments. However, given that the proposed solver is well suited for parallel processing, it can be applied to quickly solve Laplace's equation. The authors feel that this is not a serious drawback. Moreover, most inner and outer boundaries of an indoor structured environment remain static most of the time, so only the regions occupied by moving obstacles and people need to be re-computed. The computation of harmonic functions for these dynamic regions can be completed very quickly. In the future, the suggested Block iteration procedure such as EDGAOR can be extended using the log-space approach as demonstrated in [38], where the parallel implementation of the proposed approach showed great performance improvement.
The experiments conducted in this research revealed that numerical computation of Laplace's equation to solve the problem of robot pathfinding is notably appealing and practical due to recent developments and newly discovered numerical techniques, as well as the current availability of fast machines. The EDGAOR iterative approach proved to be much faster than the previous SOR and AOR approaches. An increase in the number of obstacles has no negative effect on performance; in fact, the calculation becomes faster considering the regions occupied by obstacles are ignored throughout the computation. In the future work, studies on half-sweep and quarter-sweep [36,39,40,41] iterations, would be explored in order to further improve the rate of convergence of the proposed approach.
The authors acknowledge the National Defence University of Malaysia for the funding of this article.
The researchers declare that there is no conflict of interest regarding the publication of this study.
[1] |
B. Patle, G. Babu L, A. Pandey, D. Parhi, A. Jagadeesh, A review: on path planning strategies for navigation of mobile robot, Def. Technol., 15 (2019), 582-606. http://dx.doi.org/10.1016/j.dt.2019.04.011 doi: 10.1016/j.dt.2019.04.011
![]() |
[2] | N. Buniyamin, N. Sariff, W. Wan Ngah, Z. Mohamad, Robot global path planning overview and a variation of ant colony system algorithm, International Journal of Mathematics and Computers in Simulation, 5 (2011), 9-16. |
[3] |
S. Sasaki, A practical computational technique for mobile robot navigation, Proceedings of the IEEE International Conference on Control Applications, 1998, 1323-1327. http://dx.doi.org/10.1109/CCA.1998.721675 doi: 10.1109/CCA.1998.721675
![]() |
[4] |
C. Connolly, R. Gruppen, The applications of harmonic functions to robotics, J. Robotic Syst., 10 (1993), 931-946. http://dx.doi.org/10.1002/rob.4620100704 doi: 10.1002/rob.4620100704
![]() |
[5] |
O. Khatib, Real-time obstacle avoidance for manipulators and mobile robots, Proceedings of IEEE International Conference on Robotics and Automation, 1985,500-505. http://dx.doi.org/10.1109/ROBOT.1985.1087247 doi: 10.1109/ROBOT.1985.1087247
![]() |
[6] |
S. Waydo, R. Murray, Vehicle motion planning using stream functions, Proceedings of IEEE International Conference on Robotics and Automation, 2003, 2484-2491. http://dx.doi.org/10.1109/ROBOT.2003.1241966 doi: 10.1109/ROBOT.2003.1241966
![]() |
[7] |
H. Ghassemi, S. Panahi, A. Kohansal, Solving the Laplace's equation by the FDM and BEM using mixed boundary conditions, American Journal of Applied Mathematics and Statistics, 4 (2016), 37-42. http://dx.doi.org/10.12691/ajams-4-2-2 doi: 10.12691/ajams-4-2-2
![]() |
[8] |
P. Kuo, C. Wang, H. Chou, J. Liu, A real-time streamline-based obstacle avoidance system for curvature-constrained nonholonomic mobile robots, Proceedings of 6th International Symposium on Advanced Control of Industrial Processes, 2017,618-623. http://dx.doi.org/10.1109/ADCONIP.2017.7983851 doi: 10.1109/ADCONIP.2017.7983851
![]() |
[9] |
C. Connolly, J. Burns, R. Weiss, Path planning using Laplace's equation, Proceedings of IEEE International Conference on Robotics and Automation, 1990, 2102-2106. http://dx.doi.org/10.1109/ROBOT.1990.126315 doi: 10.1109/ROBOT.1990.126315
![]() |
[10] |
J. Barraquand, B. Langlois, J. Latombe, Numerical potential field techniques for robot path planning, IEEE T. Syst. Man Cyb., 22 (1992), 224-241. http://dx.doi.org/10.1109/21.148426 doi: 10.1109/21.148426
![]() |
[11] | M. Karnik, B. Dasgupta, V. Eswaran, A comparative study of Dirichlet and Neumann conditions for path planning through harmonic functions, In: Lecture notes in computer science, Berlin: Springer, 2002,442-451. http://dx.doi.org/10.1007/3-540-46080-2_46 |
[12] |
N. Montes, F. Chinesta, M. Mora, A. Falco, L. Hilario, N. Rosillo, E. Nadal, Real-time path planning based on harmonic functions under a proper generalized decomposition-based framework, Sensors, 21 (2021), 3943. http://dx.doi.org/10.3390/s21123943 doi: 10.3390/s21123943
![]() |
[13] |
A. Falco, L. Hilario, N. Montes, M. Mora, E. Nadal, A path planning algorithm for a dynamic environment based on proper generalized decomposition, Mathematics, 8 (2020), 2245. http://dx.doi.org/10.3390/math8122245 doi: 10.3390/math8122245
![]() |
[14] | K. Al-Khaled, Numerical solutions of the Laplace's equation, Appl. Math. Comput., 170 (2005), 1271-1283. http://dx.doi.org/10.1016/j.amc.2005.01.018 |
[15] |
K. Shivaram, H. Jyothi, Finite element approach for numerical integration over family of eight node linear quadrilateral element for solving Laplace equation, Materials Today: Proceedings, 46 (2021), 4336-4340. http://dx.doi.org/10.1016/j.matpr.2021.03.437 doi: 10.1016/j.matpr.2021.03.437
![]() |
[16] |
Y. Liu, C. Fan, W. Yeih, C. Ku, C. Chu, Numerical solutions of two-dimensional Laplace and biharmonic equations by the localized Trefftz method, Comput. Math. Appl., 88 (2021), 120-134. http://dx.doi.org/10.1016/j.camwa.2020.09.023 doi: 10.1016/j.camwa.2020.09.023
![]() |
[17] |
A. Abdullah, The four point explicit decoupled group (EDG) method: a fast poison solver, Int. J. Comput. Math., 38 (1991), 61-70. http://dx.doi.org/10.1080/00207169108803958 doi: 10.1080/00207169108803958
![]() |
[18] | A. Saudi, J. Sulaiman, Half-sweep Gauss-Seidel (HSGS) iterative method for robot path planning, Proceedings of the 3rd International Conference on Informatics and Technology, 2009, 34-39. |
[19] |
M. Muthuvalu, J. Sulaiman, Half-sweep arithmetic mean method with composite trapezoidal scheme for solving linear Fredholm integral equations, Appl. Math. Comput., 217 (2011), 5442-5448. http://dx.doi.org/10.1016/j.amc.2010.12.013 doi: 10.1016/j.amc.2010.12.013
![]() |
[20] |
M. Muthuvalu, T. Htun, E. Aruchunan, M. Ali, J. Sulaiman, Performance analysis of half-sweep successive over-relaxation iterative method for solving four-point composite closed newton-cotes system, Proceedings of 7th International Conference on Intelligent Systems, Modelling and Simulation, 2016,375-379. http://dx.doi.org/10.1109/ISMS.2016.84 doi: 10.1109/ISMS.2016.84
![]() |
[21] |
S. Matsui, H. Nagahara, R. Taniguchi, Half-sweep imaging for depth from defocus, Image Vision Comput., 32 (2014), 954-964. http://dx.doi.org/10.1016/j.imavis.2014.09.001 doi: 10.1016/j.imavis.2014.09.001
![]() |
[22] | F. Muhiddin, J. Sulaiman, A. Sunarto, Solving time-fractional parabolic equations with the four point-HSEGKSOR iteration, In: Lecture notes in electrical engineering, Singapore: Springer, 2021,281-293. http://dx.doi.org/10.1007/978-981-33-4069-5_24 |
[23] | A. Dahalan, A. Saudi, Rotated TOR-5P Laplacian iteration path navigation for obstacle avoidance in stationary indoor simulation, In: Advances in intelligent systems and computing, Cham: Springer, 2021,285-295. http://dx.doi.org/10.1007/978-3-030-70917-4_27 |
[24] | J. Sulaiman, M. Hassan, M. Othman, The half-sweep iterative alternating decomposition explicit (HSIADE) method for diffusion equation, In: Lecture notes in computer sciences, Berlin: Springer, 2004, 57-63. http://dx.doi.org/10.1007/978-3-540-30497-5_10 |
[25] | J. Sulaiman, M. Othman, M. Hassan, Nine point-EDGSOR iterative method for the finite element solution of 2D Poisson equations, In: Lecture notes in computer sciences, Berlin: Springer, 2009,764-774. http://dx.doi.org/10.1007/978-3-642-02454-2_59 |
[26] |
A. Saudi, J. Sulaiman, Robot path planning using Laplacian behaviour-based control (LBBC) via half-sweep SOR, Proceedings of the International Conference on Technological Advances in Electrical, Electronics and Computer Engineering, 2013,424-429. http://dx.doi.org/10.1109/TAEECE.2013.6557312 doi: 10.1109/TAEECE.2013.6557312
![]() |
[27] |
A. Ibrahim, A. Abdullah, Solving the two dimensional diffusion equation by the four point explicit decoupled group (EDG) iterative method, Int. J. Comput. Math., 58 (1995), 253-263. http://dx.doi.org/10.1080/00207169508804447 doi: 10.1080/00207169508804447
![]() |
[28] |
D. Evans, Group explicit iterative methods for solving large linear systems, Int. J. Comput. Math., 17 (1985), 81-108. http://dx.doi.org/10.1080/00207168508803452 doi: 10.1080/00207168508803452
![]() |
[29] | G. Dahlquist, A. Bjorck, Numerical methods, New Jersey: Prentice Hall, 1974. |
[30] |
W. Yousif, D. Evans, Explicit group over-relaxation methods for solving elliptic partial differential equations, Math. Comput. Simulat., 28 (1986), 453-466. http://dx.doi.org/10.1016/0378-4754(86)90040-6 doi: 10.1016/0378-4754(86)90040-6
![]() |
[31] | D. Young, Iterative methods for solving partial difference equations of elliptic type, PhD Thesis, Harvard University, 1950. |
[32] |
D. Young, Iterative methods for solving partial difference equations of elliptic type, Trans. Amer. Math. Soc., 76 (1954), 92-111. http://dx.doi.org/10.2307/1990745 doi: 10.2307/1990745
![]() |
[33] | D. Young, Iterative solution of large linear systems, London: Academic Press, 1971. |
[34] |
A. Hadjidimos, Accelerated overrelaxation method, Math. Comput., 32 (1978), 149-157. http://dx.doi.org/10.2307/20006264 doi: 10.2307/20006264
![]() |
[35] | M. Martins, W. Yousif, D. Evans, Explicit group AOR method for solving elliptic partial differential equations, Neural, Parallel & Scientific Computations, 10 (2002), 411-422. |
[36] |
M. Othman, A. Abdullah, An efficient four points modified explicit group poisson solver, Int. J. Comput. Math., 76 (2000), 203-217. http://dx.doi.org/10.1080/00207160008805020 doi: 10.1080/00207160008805020
![]() |
[37] |
N. Ali, S. Lee, Group accelerated OverRelaxation methods on rotated grid, Appl. Math. Comput., 191 (2007), 533-542. http://dx.doi.org/10.1016/j.amc.2007.02.131 doi: 10.1016/j.amc.2007.02.131
![]() |
[38] |
K. Wray, D. Ruiken, R. Grupen, S. Zilberstein, Log-space harmonic function path planning, Proceedings of IEEE/RSJ International Conference on Intelligent Robots and Systems, 2016, 1511-1516. http://dx.doi.org/10.1109/IROS.2016.7759245 doi: 10.1109/IROS.2016.7759245
![]() |
[39] |
J. Navnit, The application of sixth order accurate parallel quarter sweep alternating group explicit algorithm for nonlinear boundary value problems with singularity, Proceedings of International Conference on Methods and Models in Computer Science, 2010, 76-80. http://dx.doi.org/10.1109/ICM2CS.2010.5706722 doi: 10.1109/ICM2CS.2010.5706722
![]() |
[40] |
A. Sunarto, J. Sulaiman, Investigation of fractional diffusion equation via QSGS iterations, J. Phys. Conf. Ser., 1179 (2019), 012014. http://dx.doi.org/10.1088/1742-6596/1179/1/012014 doi: 10.1088/1742-6596/1179/1/012014
![]() |
[41] |
J. Lung, J. Sulaiman, On quarter-sweep finite difference scheme for one-dimensional porous medium equations, International Journal of Applied Mathematics, 33 (2020), 439-450. http://dx.doi.org/10.12732/ijam.v33i3.6 doi: 10.12732/ijam.v33i3.6
![]() |
1. | A'qilah Ahmad Dahalan, Azali Saudi, An iterative technique for solving path planning in identified environments by using a skewed block accelerated algorithm, 2022, 8, 2473-6988, 5725, 10.3934/math.2023288 | |
2. | Zhen Pan, Feipeng Huang, Xin Lin, Ming Yu, Construction of new energy consumption optimization model based on improved pathfinder algorithm, 2024, 7, 2520-8942, 10.1186/s42162-024-00330-6 |
Methods | ω | r |
EGSOR | 1.82 | - |
EGAOR | 1.83 | 1.82 |
EDGSOR | 1.81 | - |
EDGAOR | 1.82 | 1.84 |
Algorithm 1: EDGAOR method | |
(i). | Setup the configuration space with a specified start and goal position. |
(ii). | Initialising the starting point U,ε←10−15,iteration←0. |
(iii). | Set the variables S1←U(k)i−1,j−1+U(k)i−1,j+1+U(k)i+1,j−1,S2←U(k+1)i−1,j−1+U(k+1)i−1,j+1+U(k+1)i+1,j−1−S1,S3←U(k)i,j+2+U(k)i+2,j+2+U(k)i+2,j. |
(iv). | For all non-occupied node points of type ⋅ using Eq (24), calculate U(k+1)i,j←r154S2+ω15[4S1+S3]+(1−ω)U(k)i,j,U(k+1)i+1,j+1←r15S2+ω15[S1+4S3]+(1−ω)U(k)i+1,j+1. |
(v). | Compute the remaining group of points (with one or two points) near to the boundary via direct method by using Eq (11). Then, evaluate the remaining points of type ∘ using Eq (6) U(k+1)i,j←14[U(k+1)i−1,j+U(k)i+1,j+U(k+1)i,j−1+U(k)i,j+1]. |
(vi). | Check the convergence test for ε←10−15. If yes, go to step (vii). Otherwise go back to step (iii). |
(vii). | Execute GDS to generate the path from start to goal point. |
Techniques | N x N | |||
300 | 600 | 900 | ||
Sample 1 | EGSOR | 1258 | 5899 | 12844 |
EGAOR | 1042 | 4994 | 10928 | |
EDGSOR | 953 | 4495 | 9916 | |
EDGAOR | 766 | 3730 | 8200 | |
Sample 2 | EGSOR | 1729 | 6782 | 14874 |
EGAOR | 1610 | 6368 | 13953 | |
EDGSOR | 1324 | 5599 | 13252 | |
EDGAOR | 1188 | 4767 | 10490 | |
Sample 3 | EGSOR | 2666 | 11076 | 24519 |
EGAOR | 2480 | 10389 | 22995 | |
EDGSOR | 2035 | 10243 | 24864 | |
EDGAOR | 1835 | 7741 | 17131 | |
Sample 4 | EGSOR | 1629 | 6487 | 14194 |
EGAOR | 1392 | 5648 | 12367 | |
EDGSOR | 1238 | 5958 | 13501 | |
EDGAOR | 1116 | 4223 | 9369 |
Techniques | N x N | |||
300 | 600 | 900 | ||
Sample 1 | EGSOR | 6.88 | 163.72 | 871.66 |
EGAOR | 6.05 | 137.87 | 751.78 | |
EDGSOR | 4.34 | 110.88 | 603.41 | |
EDGAOR | 3.67 | 95.08 | 498.19 | |
Sample 2 | EGSOR | 7.67 | 199.59 | 1009.48 |
EGAOR | 8.25 | 185.36 | 926.49 | |
EDGSOR | 4.70 | 142.64 | 787.53 | |
EDGAOR | 4.17 | 122.85 | 624.48 | |
Sample 3 | EGSOR | 13.24 | 315.87 | 1602.81 |
EGAOR | 13.83 | 301.27 | 1633.35 | |
EDGSOR | 8.00 | 261.74 | 1529.74 | |
EDGAOR | 7.67 | 203.61 | 1072.61 | |
Sample 4 | EGSOR | 7.80 | 187.33 | 990.20 |
EGAOR | 7.56 | 167.65 | 891.51 | |
EDGSOR | 4.33 | 152.73 | 828.24 | |
EDGAOR | 4.53 | 109.96 | 615.38 |
Methods | ω | r |
EGSOR | 1.82 | - |
EGAOR | 1.83 | 1.82 |
EDGSOR | 1.81 | - |
EDGAOR | 1.82 | 1.84 |
Algorithm 1: EDGAOR method | |
(i). | Setup the configuration space with a specified start and goal position. |
(ii). | Initialising the starting point U,ε←10−15,iteration←0. |
(iii). | Set the variables S1←U(k)i−1,j−1+U(k)i−1,j+1+U(k)i+1,j−1,S2←U(k+1)i−1,j−1+U(k+1)i−1,j+1+U(k+1)i+1,j−1−S1,S3←U(k)i,j+2+U(k)i+2,j+2+U(k)i+2,j. |
(iv). | For all non-occupied node points of type ⋅ using Eq (24), calculate U(k+1)i,j←r154S2+ω15[4S1+S3]+(1−ω)U(k)i,j,U(k+1)i+1,j+1←r15S2+ω15[S1+4S3]+(1−ω)U(k)i+1,j+1. |
(v). | Compute the remaining group of points (with one or two points) near to the boundary via direct method by using Eq (11). Then, evaluate the remaining points of type ∘ using Eq (6) U(k+1)i,j←14[U(k+1)i−1,j+U(k)i+1,j+U(k+1)i,j−1+U(k)i,j+1]. |
(vi). | Check the convergence test for ε←10−15. If yes, go to step (vii). Otherwise go back to step (iii). |
(vii). | Execute GDS to generate the path from start to goal point. |
Techniques | N x N | |||
300 | 600 | 900 | ||
Sample 1 | EGSOR | 1258 | 5899 | 12844 |
EGAOR | 1042 | 4994 | 10928 | |
EDGSOR | 953 | 4495 | 9916 | |
EDGAOR | 766 | 3730 | 8200 | |
Sample 2 | EGSOR | 1729 | 6782 | 14874 |
EGAOR | 1610 | 6368 | 13953 | |
EDGSOR | 1324 | 5599 | 13252 | |
EDGAOR | 1188 | 4767 | 10490 | |
Sample 3 | EGSOR | 2666 | 11076 | 24519 |
EGAOR | 2480 | 10389 | 22995 | |
EDGSOR | 2035 | 10243 | 24864 | |
EDGAOR | 1835 | 7741 | 17131 | |
Sample 4 | EGSOR | 1629 | 6487 | 14194 |
EGAOR | 1392 | 5648 | 12367 | |
EDGSOR | 1238 | 5958 | 13501 | |
EDGAOR | 1116 | 4223 | 9369 |
Techniques | N x N | |||
300 | 600 | 900 | ||
Sample 1 | EGSOR | 6.88 | 163.72 | 871.66 |
EGAOR | 6.05 | 137.87 | 751.78 | |
EDGSOR | 4.34 | 110.88 | 603.41 | |
EDGAOR | 3.67 | 95.08 | 498.19 | |
Sample 2 | EGSOR | 7.67 | 199.59 | 1009.48 |
EGAOR | 8.25 | 185.36 | 926.49 | |
EDGSOR | 4.70 | 142.64 | 787.53 | |
EDGAOR | 4.17 | 122.85 | 624.48 | |
Sample 3 | EGSOR | 13.24 | 315.87 | 1602.81 |
EGAOR | 13.83 | 301.27 | 1633.35 | |
EDGSOR | 8.00 | 261.74 | 1529.74 | |
EDGAOR | 7.67 | 203.61 | 1072.61 | |
Sample 4 | EGSOR | 7.80 | 187.33 | 990.20 |
EGAOR | 7.56 | 167.65 | 891.51 | |
EDGSOR | 4.33 | 152.73 | 828.24 | |
EDGAOR | 4.53 | 109.96 | 615.38 |