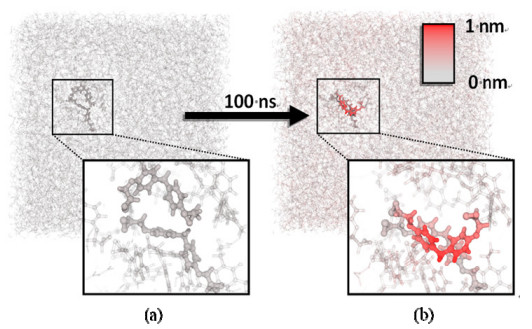
We review approaches to deriving mechanical properties from atomic simulations with a special emphasis on temperature-dependent characterization of polymer materials. The complex molecular network of such materials implies only partial, rather local ordering stemming from the entanglement of molecular moieties or covalent bonding of network nodes, whereas the polymer strands between the nodes may undergo nm-scale reorganization during thermal fluctuations. This not only leads to a strong temperature-dependence of the elastic moduli, but also gives rise to visco-elastic behavior that complicates characterization from molecular dynamics simulations. Indeed, tensile-testing approaches need rigorous evaluation of strain-rate dependences, provoking significant computational demands. Likewise, the use of fluctuations observed from unbiased constant-temperature, constant-pressure molecular dynamics simulation is not straight-forward. However, we suggest pre-processing from Fourier-filtering prior to taking Boltzmann-statistics to discriminate elastic-type vibrations of the simulation models for suitable application of linear-response theory.
Citation: Julian Konrad, Dirk Zahn. Assessing the mechanical properties of molecular materials from atomic simulation[J]. AIMS Materials Science, 2021, 8(6): 867-880. doi: 10.3934/matersci.2021053
[1] | Zhimin Cao, Chenhui Xu, Caiwei Xiao, Wei Liu, Jiaohu Huang, Wenjun Zong, Junjie Zhang, Tao Sun . Molecular dynamics study of mechanical properties of HMX–PS interface. AIMS Materials Science, 2019, 6(1): 111-118. doi: 10.3934/matersci.2019.1.111 |
[2] | Nilesh Shahapure, Dattaji Shinde, Ajit Kelkar . Atomistic modeling and molecular dynamic simulation of polymer nanocomposites for thermal and mechanical property characterization: A review. AIMS Materials Science, 2023, 10(2): 249-287. doi: 10.3934/matersci.2023014 |
[3] | Fu-Chieh Hsu, Tei-Chen Chen . Molecular dynamics simulation on mechanical behaviors of NixAl100−x nanowires under uniaxial compressive stress. AIMS Materials Science, 2019, 6(3): 377-396. doi: 10.3934/matersci.2019.3.377 |
[4] | Koufos Evan, Muralidharan Bharatram, Dutt Meenakshi . Computational Design of Multi-component Bio-Inspired Bilayer Membranes. AIMS Materials Science, 2014, 1(2): 103-120. doi: 10.3934/matersci.2014.2.103 |
[5] | Samaneh Nasiri, Michael Zaiser . Rupture of graphene sheets with randomly distributed defects. AIMS Materials Science, 2016, 3(4): 1340-1349. doi: 10.3934/matersci.2016.4.1340 |
[6] | Mica Grujicic, Jennifer S. Snipes, S. Ramaswami . Polyurea/Fused-silica interfacial decohesion induced by impinging tensile stress-waves. AIMS Materials Science, 2016, 3(2): 486-507. doi: 10.3934/matersci.2016.2.486 |
[7] | Yun-Lin Liu, Si-Yu Ren, Dong-Hua Wang, Ding-Wei Yang, Ming-Feng Kai, Dong Guo . Molecular structure and thermal conductivity of hydrated sodium aluminosilicate (N-A-S-H) gel under different Si/Al ratios and temperatures: A molecular dynamics analysis. AIMS Materials Science, 2025, 12(2): 258-277. doi: 10.3934/matersci.2025014 |
[8] | Jiyu Cai, Qian Sun, Xiangbo Meng . Novel nanostructured materials by atomic and molecular layer deposition. AIMS Materials Science, 2018, 5(5): 957-999. doi: 10.3934/matersci.2018.5.957 |
[9] | Laura J. Weiser, Erik E. Santiso . Molecular modeling studies of peptoid polymers. AIMS Materials Science, 2017, 4(5): 1029-1051. doi: 10.3934/matersci.2017.5.1029 |
[10] | Jing Chen, Ben J. Hanson, Melissa A. Pasquinelli . Molecular Dynamics Simulations for Predicting Surface Wetting. AIMS Materials Science, 2014, 1(2): 121-131. doi: 10.3934/matersci.2014.2.121 |
We review approaches to deriving mechanical properties from atomic simulations with a special emphasis on temperature-dependent characterization of polymer materials. The complex molecular network of such materials implies only partial, rather local ordering stemming from the entanglement of molecular moieties or covalent bonding of network nodes, whereas the polymer strands between the nodes may undergo nm-scale reorganization during thermal fluctuations. This not only leads to a strong temperature-dependence of the elastic moduli, but also gives rise to visco-elastic behavior that complicates characterization from molecular dynamics simulations. Indeed, tensile-testing approaches need rigorous evaluation of strain-rate dependences, provoking significant computational demands. Likewise, the use of fluctuations observed from unbiased constant-temperature, constant-pressure molecular dynamics simulation is not straight-forward. However, we suggest pre-processing from Fourier-filtering prior to taking Boltzmann-statistics to discriminate elastic-type vibrations of the simulation models for suitable application of linear-response theory.
For crystalline materials, the prediction of elastic properties became a routine task that usually relies on energy minimization to get equilibrium structures, followed by (numerical) assessment of the second derivatives of energy upon simulation cell deformation [1,2,3]. While this approach leads to elastic moduli of quite reasonable accuracy when characterizing metals and ionic crystals, molecular materials—in particular if they are not crystalline—show strong, and often non-linear, temperature-dependence of mechanical properties [4,5]. This opposes to the zero Kelvin approximation inherent to structures derived from energy minimization. Instead, Monte-Carlo or molecular dynamics simulation methods are required to account for temperature effects to atomistic models [6,7].
A very intuitive molecular dynamics simulation approach for the assessment of mechanical properties is to essentially mimic the corresponding experiment by inducing a deformation of the simulation model and sampling the restoring forces. On this basis, each mode of deformation (tensile loading, shear, bulk compression) can be probed, and the elastic moduli attributed to the curvature of the respective stress-strain diagram. On the other hand, a very elegant route was originally suggested more than 50 years ago—by attributing the spontaneous fluctuations of a sample volume to a linear response model [8,9]. This allows for simultaneous sampling of all elastic moduli from constant-temperature, constant-pressure molecular dynamics or Monte-Carlo simulations.
Both of these approaches call for careful implementation when applied to complex molecular materials. In what follows, we will review the fundamental simulation protocols and outline strategies for error control. To demonstrate the assessment of temperature-dependent elastic moduli, we selectively picked a recent molecular dynamics simulation model that mimics an epoxy polymer [10]. This not only reflects a particularly prominent class of molecular materials, but also a rather rigorous benchmark for system complexity. Indeed, freshly cured epoxy resins are non-crystalline (and desired to remain so within most technical applications), like most polymer materials, and show little ordering beyond the range of 1–2 nm. On the other hand, there is significant local ordering at the < 1 nm scale stemming from the well-defined chemistry of binding the monomeric units into polymer strands. These strands may occur as in principle stand-alone fibers which are however twisted and entangled with each other [11]. More robust polymer materials, such as epoxy resins, are however comprised of covalently bonded networks [12,13]. As a consequence, the entire sample of the material may be considered as a single molecule. The mechanical properties dramatically depend on the degree of cross-linking which, in state-of-the-art industrial application, reaches almost 100% of the available binding sites [14]. In our benchmark model, we achieved 98% crosslinking of the base monomer (bisphenol F diglycidyl ether, BFDGE) and the linker species (4, 6-diethyl-2-methylbenzene-1, 3-diamine, DETDA) [10,15]. This could be achieved from systematically exploring the curing process from combined quantum/molecular mechanical treatment of linking reactions and extended analyses of overall network relaxation upon increasing degree of cross-linking [10,15].
Despite this high level of crosslinking, epoxy resins are best described as molecular networks with a clear distinction between strands and nodes [12,13]. The nodes that (covalently) connect 2-4 strands are separated by 1–2 nm, whereas the strands comprise of molecular moieties which atoms are bonded at ~0.15 nm distance. This structural diversity has important implications on the mechanical properties. While damaging and fracture is related to the cleavage of crosslinks or, much less frequently, rupture of strands, even the network of intact nodes gives rise to non-linear stress-strain characteristics [10]. The 1–2 nm sized strands are comparably flexible and may rotate, bend, or twist in response to mechanical load—but also by thermal fluctuations. For this reason, we find strong changes of the mechanical properties as functions of temperature. For the same reason, we however also find strong dependence of the stress-strain diagrams as functions of the strain rates used to deform the material [4,5].
The most frequently used approach to computing elastic moduli from atomic simulations is based on non-dynamic calculations [16,17]. Starting points are relaxed structures resulting from energy minimization. These are then subjected to small deformations ˉε (e.g. by linearly shifting atom positions according to ±1% uniaxial deformation ϵ11,ϵ22andϵ33 or ±1° shear in ϵ23,ϵ13andϵ12, respectively) and the corresponding stress ˉσ is related to (using Voigt notation) [18]:
ˉσ=(σ11σ22σ33σ23σ13σ12)=(c11⋯c61⋮⋱⋮c16⋯c66)(ε11ε22ε33ε23ε13ε12)=¯¯c⋅ˉε | (1) |
Despite the inherent zero Kelvin approximation, this is the method of choice for crystalline materials, provided that the melting point is far above the technically relevant temperature (usually 300 K) for which the prediction of elastic properties shall apply.
For such systems, typical calculations focus on units cells subjected to periodic boundary conditions—which implies only few explicit atoms and hence allows for high-accuracy quantum mechanical description. Using this single crystal approximation, symmetry-equivalent elastic moduli cij may be ruled out and the independent deformation modes may be either probed individually or concerted fitting of ¯¯c according to Eq 1 is performed [19]. Along this line, tensile deformations and shear are typically implemented as < 5% deformation and < 10° shear of the unit cell vectors, whereas the explicit atoms therein are allowed to relax according to energy minimization as a function of ˉε.
For simple crystals like pure metals, structure relaxation typically leads to linear atomic displacements according to ˉε, however for more complex systems such as intermetallic phases, ionic and molecular crystals the atomic displacements significantly depend on the interplay of overall unit cell deformation and the heterogeneity of local interactions. This is particularly evident for molecular crystals which feature strong covalent bonds within the molecules and much weaker interactions (H-bonds, π-stacking, van-der-Waals) between the molecules.
Inhomogeneous atomic displacements upon crystal deformation are also crucial for rationalizing the temperature-dependence of the elastic properties. The reorganization of local atomic moieties may involve significant displacements normal to the overall mode of deformation ˉε applied to the crystal. Examples are zig-zag shifts leading to the puckering of layers or rotation/slipping of molecular fragments such as polymer strands [5]. Subject to the strength of local interactions, these displacements may occur spontaneously during deformation—or involve the crossing of energy barriers ΔE before locking into a favorable configuration. While the former situation is well-described by energy minimization approaches, barrier crossing calls for explicit account of temperature T and thus requires Monte-Carlo or molecular dynamics (MD) simulations. Among these two techniques, MD simulations are widely preferred for rationalizing complex materials because of its direct insights into reorganization dynamics.
In MD simulations of material deformation, the likeliness of reorganization events triggered by the crossing an energy barrier ΔE is related to p=p0e−ΔEkBT with kB being the Boltzmann constant and p0 being a kinetic pre-factor which depends on the strain rate applied to induce deformation. Atomic displacements confined by only small barriers, say ΔE<kBT, typically occur on time scales that are much lower than the time scales at which deformation experiments are performed. Such small-barrier reorganization hence appear as elastic modes unless the deformation rate reaches the speed of sound. On the other hand, local reorganization events that require the crossing of large barriers happen only rarely. As a consequence, the material will undergo only a fraction of all possible relaxation moves. Each reorganization event lowers the stress and the overall restoring force response to a given deformation will thus depend on the available relaxation time.
As a simplified picture, such pseudo-elastic behavior may be considered as a first order kinetics assuming at least two types of local deformations, (a) linearly strained regions with comparably large potential energy and (b) domains in which atomic displacements normal to the applied deformation mode helped to lower energy. The overall stress σ will then result from a combination of type (a) and type (b) contributions, subject to the corresponding occurrences ha and hb=1−ha, respectively:
σ=ha⋅σa+(1−ha)⋅σbwithha(t)=1⋅e−t/τrelaxation | (2) |
where the characteristic relaxation time scale τrelaxation is the inverse of the a→b transition rate ra→b(T)—which is a function of temperature as given by the Arrhenius law [20].
τrelaxation=1/ra→b=const⋅e+ΔE/kBT | (3) |
To illustrate the importance of local reorganization events, in Figure 1 we depicted snapshots from an epoxy polymer model taken from [10]. In this 100 ns MD simulation run we did not apply external loading to the model system, but only used an (anisotropic) barostat-thermostat algorithm to inspect thermal fluctuations at 1 atm and 300 K, respectively. Comparing the two snapshots, we find (i) a quite homogeneous distribution of atomic displacements in the ball-park of 0.1–0.2 nm and (ii) a strongly inhomogeneous pattern of atomic displacements up to 1 nm. Here, (i) reflects typical vibrations of atoms with respect to their lattice site. In contrast to this, the much rarer, but larger displacements of type (ii) illustrate local reorganization of polymer strands. For the latter, the system must overcome energy barriers stemming from the dissociation of strand-strand contacts and the sliding of adjacent molecular moieties [5].
In absence of external loading, such local rearrangement events occur without orientation preference and thus lead to fluctuations of the simulation cell (which we will inspect more closely in the next section). However, in a model system which experiences deformation, the rotation of polymer strands within the twisted network gets biased in favor of stress reduction. This leads to an exponential decay of σ(t) as predicted by Eq 1—and monitored within MD simulations at constant strain as illustrated in Figure 2.
The most widely spread approach to assessing elastic moduli at non-zero temperature is to perform MD simulations combined with induced deformation of the simulation cell. In analogy to the experiment, tensile testing or shearing within MD runs may be implemented by application of external stress with is ramped up linearly as a function of time. The fundamental difference here is however the large gap between experimental time scales and the ns to μs time scales available to MD simulation using force-fields of atomic resolution. This is even worse for ab-initio MD with offer electronic resolution, but barely reach the ns time scale. To get meaningful stress-strain diagrams from molecular simulation, the common route is to apply strain to the simulation cell and sample the resulting stress.
Along this line, the shape of the simulation cell is controlled externally, typically whilst maintain the overall volume of the system. In ref. [10], we used an unbiased approach for this purpose, namely via implementation of axial deformation at constant rate using ϵ11=1+˙ϵ⋅(t−t0) and fixing the length of the corresponding cell vector ⇀a to a=ϵ11⋅a(t0), whereas a 2-dimensional barostat algorithm allows relaxation in the perpendicular directions [10]. Stress-strain profiles collected in this manner will nevertheless depend on the applied rates and convergence checks from comparing a series of deformation runs of different strain rates are advised. For simple single crystalline systems, this often suffices to obtain reasonable agreement with the experiment. In turn, molecular materials—like the polymer model used as a demonstrator system in Figures 1 and 2—are however quite likely to display very slow relaxation modes [5]. Indeed, tensile testing of epoxy polymer shows strain rate dependence even in experiments performed on the time scale of up to 105 s—which exceeds the scope of MD simulations by more than 10 orders of magnitude [21].
To model such systems in a more realistic manner, MD simulations need to be redirected to mimic quasi-static conditions. For this, a series of deformed simulation cells may initially be prepared from taking snapshots from constant strain rate runs. Next, the corresponding models are inspected in parallel MD runs at constant strain. This allows investing up to μs of relaxation time to an individual data point of the stress-strain diagram. The underlying relaxation process can be followed by monitoring stress as a function of time—as illustrated in Figure 2. While the overall relaxation might require even longer time scales, already 10 ns scale runs may offer insights into partial relaxation which suffices for exponential fits of the decay in σ(t) as denoted in Eq 2. Using the asymptotic stress σ(t→∞) from this procedure for comparison to the experiment, we indeed found quite convincing agreement of the elastic part of the stress-strain diagrams and even the ultimate stress of the epoxy system before fracture [10,21].
A very elegant way of assessing elastic properties of materials is to take use of spontaneous fluctuations from the equilibrium geometry [8,22]. For a given set of temperature and pressure conditions, we can describe the equilibrium cell of a simulation model by the length of the box edges a0,b0,c0 and the angles between the cell vectors α0,β0,γ0, respectively, and expand a Taylor series for describing the energy cost ΔE of deviations Δa,Δα,Δb,Δβ,ΔcandΔγ, respectively. For the example of bulk compression ΔV, we thus get:
E(ΔV)=E0+∂E∂V|V0⋅ΔV+12∂2E∂V2|V0⋅(ΔV)2+16∂3E∂V3|V0⋅(ΔV)3+124∂4E∂V4|V0⋅(ΔV)4+.. | (4) |
whereas full analogous expansions ΔE(Δa), ΔE(Δα) etc. are obtained for tensile and shear deformation modes, respectively. Note that all derivatives are taken with respect to the equilibrium geometry which causes the first derivatives in Eq 4 to vanish.
Per definition, elastic deformation implies a linear response of the restoring stress as a function of strain, namely:
p=−ΔEΔV=−K⋅ΔV | (5) |
with
K={∂2E∂V2|V0(idealelastic)∂2E∂V2|V0+12∂3E∂V3|V0⋅(ΔV)+16∂4E∂V4|V0⋅(ΔV)2+..(non−linearelastic) | (6) |
In absence of external loading, the deviations of simulation cell volume ΔV=V(t)−V0 (and likewise Δa,Δα etc.) only stem from thermal fluctuations and are hence as small as actually possible at non-zero temperature. As a consequence, the elastic behavior is typically well described by the idealized linear formulation of Eq 5 with K=∂2E∂V2|V0 being a constant. While the implementation of so-called non-linear elastic moduli is straight-forward [23], in what follows we focus on the ideal elastic case.
After sufficient relaxation of a material under investigation to ensure thermodynamic equilibrium by means of constant-temperature, constant-pressure MD simulation, the fluctuations of the simulation cell are simultaneously obtained by monitoring the cell vectors as functions of time [22]. Taking use of Boltzmann statistics, the occurrence profile h of spontaneously observed deformations related to a given mode can directly be related to the corresponding energy profile. For the example of volume fluctuationsΔV=V(t)−V0 this implies
h(ΔV)=h0⋅e−E(ΔV)kBT=h0⋅e−E0+12K(ΔV)2kBT=const⋅e−(ΔV)22dΔV | (7) |
where the right part of Eq 7 refers to the fitting of a Gaussian distribution to the occurrence statistics of ΔV=V(t)−⟨V⟩t with V0=⟨V⟩t and dV=dΔV being the standard deviation of ΔV(t). Likewise, Gaussian fits may be performed for the occurrence profiles of cell vector dimensions, e.g. Δa(t) or the cell angles, e.g. Δα(t) to provide the corresponding standard deviations daanddα, respectively. Thus, the analyses of a single MD run at thermodynamic equilibrium offers the entire set of elastic constants:
K=kBT(dV)2⋅⟨V⟩t (bulk modulus) Ya=kBT(da)2⋅(⟨a⟩t)2⟨V⟩t (Youngs modulus in a direction) Gab=kBT(dγ)2⋅1⟨V⟩t(abshear modulus) | (8) |
Despite the elegant simplicity of this approach, practical application to characterizing complex materials is complicated by the overlapping of elastic modes with other processes such as visco-elastic deformation. Indeed, for molecular materials models subjected to direct sampling of occurrence statistics we typically find the elastic moduli from Eq 8 to be underestimated. This is nicely illustrated by our beforehand discussed benchmark system modelling an epoxy polymer [10].
To assess the Youngs modulus for deformations along a, we find a superposition of elastic and visco-elastic fluctuations than can formally be written as:
a(t)=a0+Δaelastic(t)+Δavisco(t) | (9) |
As a consequence, the standard deviation taken from the overall occurrence statistics da is equal or larger than that of the purely elastic deformation models, Figure 3 clearly indicates da>daelastic in the epoxy model.
Ideally, the elastic constants should be derived from an exclusive statistics of Δaelastic(t). For this, we however must discriminate elastic from non-elastic deformation which is far from trivial in a complex molecular material. The only guide for such differentiation is given by the different time-scales of elastic deformation (fastest modes) and the usually much slower visco-elastic modes. Indeed, when sampling the occurrence profiles over short (here 5 ns) time intervals we found the standard deviations da in line with experimental values of the Youngs modulus [10].
In case of extended sampling over longer periods we therefore suggested to sample da in 5 ns intervals, and then use the overall average ⟨da⟩ as input to Eq 8 [10,15]. While this lead to excellent agreement of bulk, Youngs and shear moduli in line with the experiment, the obvious downside of this approach is the somewhat arbitrary choice of the time intervals. Moreover, few but still some of these intervals include larger scale deformation stemming from viscous modes of molecular movements. Anyway, we argue that fragmenting the statistics in time intervals converges to ⟨da⟩≅⟨daelastic⟩ for sufficiently short sampling periods, whereas the direct sampling of overall statistics implies ⟨da⟩=⟨daelastic⟩+⟨davisco⟩ and thus inadequate inputs to Eq 8. In ref. [10], the choice of 5 ns was motivated by choosing the sampling intervals short, whilst still providing smooth occurrence profiles for fitting the Gaussians reliably.
From a materials science perspective, the separation of (ideal) elastic and pseudo/visco-elastic modes reflects the spatial and time-depend fluctuations of elastic constants in polymer materials at the nm (ns) length (and time) scales, respectively [5,24]. From a mathematical viewpoint, the separation of scales is routinely performed by means of Fourier filtering. To bring this together, we analyzed the Fourier transforms of the simulation cell shape and dimensions as shown in Figure 4.
The intensities of the Fourier transforms of a(t),α(t)andV(t) show a clear peak at low frequencies ν<0.05−0.1ns−1, subject to the mode of deformation. In turn, a broader spectrum is found for the faster vibrational modes. To separate elastic and visco-elastic type fluctuations, we suggest Fourier-filtering by means of a cut-off frequency delimiter νcut:
a(t)=aelastic(t)+avisco(t)=FT−1[FT[a(t)],ν≥νcut]+FT−1[FT[a(t)],ν<νcut] | (10) |
with
aelastic(t)≅FT−1[FT[a(t)],ν≥νcut] | (11a) |
avisco(t)≅FT−1[FT[a(t)],ν<νcut] | (11b) |
Likewise, analogous filtering of α(t)andV(t) is obtained, however using individual frequency delimiters νcut to account for the different dynamics of tensile (νcut=0.1ns−1), shear (νcut=0.05ns−1) and bulk volume (νcut=0.04ns−1) deformations.
On the basis of these approximations, we sampled the fluctuations daelastic and davisco of merely elastic aelastic(t) and viscous avisco(t) deformation modes of the simulation cell as illustrated in Figure 5. This separation of time scales leads to more reliable sampling of the elastic-type fluctuations daelastic as compared to the statistics taken from a series of time intervals we discussed earlier. This is also reflected by comparing our modeling results to the experimental assessment of the Youngs, shear and bulk moduli as functions of temperature, respectively.
For such benchmarking, we decided to focus on the study of Littell et al. in which a consistent series based on a constant experimental setup was used (Figure 6) [21]. Likewise, we analyzed the elastic properties as a function of temperature using the same atomic configuration as starting point for MD runs at 300,350,400 and 450 K, respectively. While the modeling data obtained at 300 and 350 K nicely agrees with the experimental data available, we find that a clear-cut separation of time scales for elastic/viscous deformation modes may not be achieved for MD runs at 400 K or even larger temperatures. This limitation is in line with the intrinsic difficulty in discriminating elastic and viscous deformations in polymers near their glass transition temperature (~440 K) [10].
Molecular modeling and simulation approaches are continuously catching up with the complexity of modern molecular materials. While early simulation studies of polymer networks mainly coped with the appropriate description of molecular interactions [11], current computational resources allow all-atom modeling based on robust empirical interaction potentials such as the OPLS or GAFF force-fields [27,28]. Clearly, the length scales inherent to proper modeling of complex polymer networks still oppose a full ab-initio description, in particular when it come to dynamics calculations. However, reactive force-field approaches and combined quantum/classical techniques help to provide near ab-initio accuracy where needed, namely for the cross-links between the polymer precursors [10,15,29,30,31].
This not only boosted the quality of assessing the atomic interactions of a given polymer model, but also greatly helped to create the model itself. Indeed, for non-crystalline networks of molecules it is far from trivial to provide realistic starting structures and extensive relaxation of the simulation systems is needed to ensure convergence. With typically dimensions exceeding the 10000 atoms scale and relaxation times beyond the 10 ns scale, the use of smart molecular mechanics models is likely to prevail the state-of-the-art also in the nearer future.
The issue of structural complexity, and the time-dependent diversity of local ordering in molecular materials is also reflected in the analysis and the understanding of mechanical properties. The techniques reviewed in the present work offer a versatile toolbox for this purpose. Among these methods, the computational demand differs quite significantly. Temperature-dependent analyses of molecular dynamics simulation runs are clearly the most expensive option—but allow exciting atomic scale insights into complex network dynamics in parallel to computing macroscopic properties. Thus, for the actual understanding of molecular materials we consider molecular simulations as an important, if not indispensable extension to the experiment.
This work was funded by the Graduiertenkolleg GRK 2423, "Frascal" of the Friedrich-Alexander-Universität Erlangen-Nürnberg.
The authors declare no conflict of interest.
[1] |
Kresse G, Furthmüller J (1996) Efficiency of ab-initio total energy calculations for metals and semiconductors using a plane-wave basis set. Comp Mater Sci 6: 15-50. doi: 10.1016/0927-0256(96)00008-0
![]() |
[2] |
Giannozzi P, Baseggio O, Bonfà P, et al. (2020) Quantum ESPRESSO toward the exascale. J Chem Phys 152: 154105. doi: 10.1063/5.0005082
![]() |
[3] |
Gale JD (2005) GULP: Capabilities and prospects. Z Krist-Cryst Mater 220: 552-554. doi: 10.1524/zkri.220.5.552.65070
![]() |
[4] | Treloar LRG (1975) The Physics of Rubber Elasticity, Oxford: Oxford University Press. |
[5] | DoiM, Edwards SF (1986) The Theory of Polymer Dynamics, Oxford: Clarendon Press. |
[6] | Rapaport DC (1996) The Art of Molecular Dynamics Simulation, Cambridge University Press. |
[7] | Frenkel D, Smit B (2002) Understanding Molecular Simulation: From Algorithms to Applications, Elsevier. |
[8] | Landau LD, Lifshitz EM (1958) Statistical physics: Theory of the condensed state, In: Sykes JB, Kearsley MJ, Course of Theoretical Physics, Pergamon Press. |
[9] |
Lebowitz JL, Percus JK, Verlet L (1967) Ensemble dependence of fluctuations with application to machine computations. Phys Rev 153: 250. doi: 10.1103/PhysRev.153.250
![]() |
[10] | Konrad J, Meissner RH, Bitzek E, Zahn D (2021) A molecular simulation approach to bond reorganization in epoxy resins: From curing to deformation and fracture (in press). ACS Polym Au. |
[11] |
Everaers R, Kremer K (1996) Elastic properties of polymer networks. J Mol Model 2: 293-299. doi: 10.1007/s0089460020293
![]() |
[12] | Lee H, Neville K (1987) Chemistry and Technology of Epoxy Resins, 2 Eds., CRC Press. |
[13] | Ellis B (1993) Chemistry and Technology of Epoxy Resins, Springer Science + Business Media. |
[14] |
Cai H, Li P, Sui G, et al. (2008) Curing kinetics study of epoxy resin/flexible amine toughness systems by dynamic and isothermal DS. Thermochim Acta 473: 101-105. doi: 10.1016/j.tca.2008.04.012
![]() |
[15] |
Meissner RH, Konrad J, Boll B, et al. (2020) Molecular simulation of thermosetting polymer hardening: Reactive events enabled by controlled topology transfer. Macromolecules 53: 9698-9705. doi: 10.1021/acs.macromol.0c02222
![]() |
[16] |
Fast L, Wills JM, Johansson B, et al. (1995) Elastic constants of hexagonal transition metals: Theory. Phys Rev B 51: 17431. doi: 10.1103/PhysRevB.51.17431
![]() |
[17] |
Karki BB, Stixrude L, Clark SJ, et al. (1997) Structure and elasticity of MgO at high pressure. Am Mineral 82: 51-60. doi: 10.2138/am-1997-1-207
![]() |
[18] | Nye JF (1985) Physical Properties of Crystals: Their Representation by Tensors and Matrices, Oxford: Oxford University Press. |
[19] |
Yu R, Zhu J, Ye HQ (2010) Calculations of single-crystal elastic constants made simple. Comput Phys Commun 181: 671-675. doi: 10.1016/j.cpc.2009.11.017
![]() |
[20] | Arrhenius S (1889) Über die Reaktionsgeschwindigkeit bei der Inversion von Rohrzucker durch Säuren. Z Phys Chem 4: 226. |
[21] |
Littell JD, Ruggeri CR, Goldberg RK, et al. (2008) Measurement of epoxy resin tension, compression, and shear stress-strain curves over a wide range of strain rates using small test specimens. J Aerospace Eng 21: 162-173. doi: 10.1061/(ASCE)0893-1321(2008)21:3(162)
![]() |
[22] |
Parrinello M, Raman A (1982) Strain fluctuations and elastic constants. J Chem Phys 76: 2662-2666. doi: 10.1063/1.443248
![]() |
[23] |
Duan G, Lind ML, Demetriou MD, et al. (2006) Strong configurational dependence of elastic properties for a binary model metallic glass. Appl Phys Lett 89: 151901. doi: 10.1063/1.2360203
![]() |
[24] | Muliana AH (2021) Spatial and temporal changes in physical properties of epoxy during curing and their effects on the residual stresses and properties of cured epoxy and composites. Appl Eng Sci 7: 100061. |
[25] |
Bajpai A, Alapati AK, Klingler A, et al. (2018) Tensile properties, fracture mechanics properties and toughening mechanisms of epoxy systems modified with soft block copolymers, rigid TiO2 nanoparticles and their hybrids. J Compos Sci 2: 72. doi: 10.3390/jcs2040072
![]() |
[26] |
Kallivokas SV, Sgouros AP, Theodorou DN (2019) Molecular dynamics simulations of EPON-862/DETDA epoxy networks: Structure, topology, elastic constants, and local dynamics. Soft Matter 15: 721-733. doi: 10.1039/C8SM02071J
![]() |
[27] |
Jorgensen WL, Maxwell DS, Tirado-Rives J (1996) Development and testing of the OPLS all-atom force field on conformational energetics and properties of organic liquids. J Am Chem Soc 118: 11225-11236. doi: 10.1021/ja9621760
![]() |
[28] |
Wang J, Wolf RM, Caldwell JW, et al. (2004) Development and testing of a general amber force field. J Comput Chem 25: 1157-1174. doi: 10.1002/jcc.20035
![]() |
[29] |
Varshney V, Patnaik SS, Roy AK, et al. (2008) A molecular dynamics study of epoxy-based networks: Cross-linking procedure and prediction of molecular and material properties. Macromolecules 41: 6837-6842. doi: 10.1021/ma801153e
![]() |
[30] |
Nouri N, Ziaei-Rad S (2011) A molecular dynamics investigation on mechanical properties of cross-linked polymer networks. Macromolecules 44: 5481-5489. doi: 10.1021/ma2005519
![]() |
[31] |
Li C, Strachan A (2010) Molecular simulations of crosslinking process of thermosetting polymers. Polymer 51: 6058-6070. doi: 10.1016/j.polymer.2010.10.033
![]() |
1. | Julian Konrad, Sebastian Pfaller, Dirk Zahn, Multi-Scale Modelling of Plastic Deformation, Damage and Relaxation in Epoxy Resins, 2022, 14, 2073-4360, 3240, 10.3390/polym14163240 | |
2. | Thomas G. Mason, Benny D. Freeman, Ekaterina I. Izgorodina, Influencing Molecular Dynamics Simulations of Ion-Exchange Membranes by Considering Comonomer Propagation, 2023, 56, 0024-9297, 1263, 10.1021/acs.macromol.2c01743 | |
3. | Julian Konrad, Paolo Moretti, Dirk Zahn, Molecular Simulations and Network Analyses of Surface/Interface Effects in Epoxy Resins: How Bonding Adapts to Boundary Conditions, 2022, 14, 2073-4360, 4069, 10.3390/polym14194069 | |
4. | Julian Konrad, Dirk Zahn, Bottom-to-top modeling of epoxy resins: From atomic models to mesoscale fracture mechanisms, 2024, 160, 0021-9606, 10.1063/5.0180355 | |
5. | Julian Konrad, Dirk Zahn, Interfaces in reinforced epoxy resins: from molecular scale understanding towards mechanical properties, 2023, 29, 1610-2940, 10.1007/s00894-023-05654-w | |
6. | Janina Mittelhaus, Julian Konrad, Julius Jacobs, Phil Röttger, Robert Meißner, Bodo Fiedler, Load‐Induced Shear Band Formation in Microscale Epoxy Materials, 2025, 2642-4150, 10.1002/pol.20241162 |