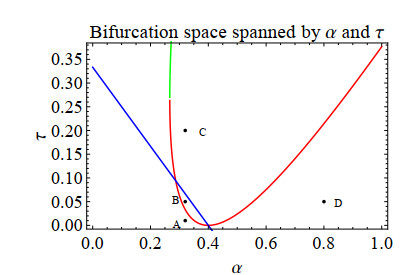
Energy consumption accounting and prediction in the medium and thick plate rolling process are crucial for controlling costs, improving production efficiency, optimizing equipment management, and enhancing the market competitiveness of enterprises. Starting from the perspective of integrating process mechanism and industrial big data, we overcame the difficulties brought by complex and highly nonlinear coupling of process variables, proposed a rolling power consumption accounting algorithm based on time slicing method, and gave a calculation method for the additional power consumption of the main motor for rough rolling and finishing rolling (auxiliary system power consumption, power loss, main motor power consumption deviation); with the help of SIMS model, forward recursion, and reverse recursion pass rolling force estimation strategies are proposed, and the rated power consumption of the main motor was predicted. Furthermore, a random forest regression model of additional power consumption based on data was established, and then a prediction algorithm for the comprehensive power consumption of billet rolling was given. Experiments showed the effectiveness of the proposed method.
Citation: Qiang Guo, Zimeng Zhou, Jie Li, Fengwei Jing. Mechanism- and data-driven algorithms of electrical energy consumption accounting and prediction for medium and heavy plate rolling[J]. Electronic Research Archive, 2025, 33(1): 381-408. doi: 10.3934/era.2025019
[1] | Anna Sun, Ranchao Wu, Mengxin Chen . Turing-Hopf bifurcation analysis in a diffusive Gierer-Meinhardt model. AIMS Mathematics, 2021, 6(2): 1920-1942. doi: 10.3934/math.2021117 |
[2] | Fethi Souna, Salih Djilali, Sultan Alyobi, Anwar Zeb, Nadia Gul, Suliman Alsaeed, Kottakkaran Sooppy Nisar . Spatiotemporal dynamics of a diffusive predator-prey system incorporating social behavior. AIMS Mathematics, 2023, 8(7): 15723-15748. doi: 10.3934/math.2023803 |
[3] | Ting Gao, Xinyou Meng . Stability and Hopf bifurcation of a delayed diffusive phytoplankton-zooplankton-fish model with refuge and two functional responses. AIMS Mathematics, 2023, 8(4): 8867-8901. doi: 10.3934/math.2023445 |
[4] | Mohamed Lamine Sahari, Abdel-Kaddous Taha, Louis Randriamihamison . Exploring three periodic point dynamics in 2D spatiotemporal discrete systems. AIMS Mathematics, 2025, 10(3): 5021-5051. doi: 10.3934/math.2025230 |
[5] | Yingyan Zhao, Changjin Xu, Yiya Xu, Jinting Lin, Yicheng Pang, Zixin Liu, Jianwei Shen . Mathematical exploration on control of bifurcation for a 3D predator-prey model with delay. AIMS Mathematics, 2024, 9(11): 29883-29915. doi: 10.3934/math.20241445 |
[6] | Ruizhi Yang, Dan Jin, Wenlong Wang . A diffusive predator-prey model with generalist predator and time delay. AIMS Mathematics, 2022, 7(3): 4574-4591. doi: 10.3934/math.2022255 |
[7] | Yougang Wang, Anwar Zeb, Ranjit Kumar Upadhyay, A Pratap . A delayed synthetic drug transmission model with two stages of addiction and Holling Type-II functional response. AIMS Mathematics, 2021, 6(1): 1-22. doi: 10.3934/math.2021001 |
[8] | Hairong Li, Yanling Tian, Ting Huang, Pinghua Yang . Hopf bifurcation and hybrid control of a delayed diffusive semi-ratio-dependent predator-prey model. AIMS Mathematics, 2024, 9(10): 29608-29632. doi: 10.3934/math.20241434 |
[9] | Changjin Xu, Chaouki Aouiti, Zixin Liu, Qiwen Qin, Lingyun Yao . Bifurcation control strategy for a fractional-order delayed financial crises contagions model. AIMS Mathematics, 2022, 7(2): 2102-2122. doi: 10.3934/math.2022120 |
[10] | Xiao-Long Gao, Hao-Lu Zhang, Xiao-Yu Li . Research on pattern dynamics of a class of predator-prey model with interval biological coefficients for capture. AIMS Mathematics, 2024, 9(7): 18506-18527. doi: 10.3934/math.2024901 |
Energy consumption accounting and prediction in the medium and thick plate rolling process are crucial for controlling costs, improving production efficiency, optimizing equipment management, and enhancing the market competitiveness of enterprises. Starting from the perspective of integrating process mechanism and industrial big data, we overcame the difficulties brought by complex and highly nonlinear coupling of process variables, proposed a rolling power consumption accounting algorithm based on time slicing method, and gave a calculation method for the additional power consumption of the main motor for rough rolling and finishing rolling (auxiliary system power consumption, power loss, main motor power consumption deviation); with the help of SIMS model, forward recursion, and reverse recursion pass rolling force estimation strategies are proposed, and the rated power consumption of the main motor was predicted. Furthermore, a random forest regression model of additional power consumption based on data was established, and then a prediction algorithm for the comprehensive power consumption of billet rolling was given. Experiments showed the effectiveness of the proposed method.
A single-species reaction-diffusion model with spatiotemporal delay which was firstly proposed by Britton [1] is considered as follows:
∂u∂t=dΔu+ru(1+αu−βu2−(1+α−β)(ψ∗u)), | (1.1) |
where u=u(x,t) for (x,t)∈R×[0,+∞) presents the density of a single-species at space x and time t, ψ is a given function and ψ∗u means a convolution in space x and time t, the parameters d,r,α and β are all positive constants and 1+α−β>0. For the biological explanations of all terms in the model (1.1), according to references [1,2], we have known that d>0 is the diffusive coefficient, the term αu is a measure of the advantage to individuals in agammaegating or grouping and βu2 represents competition for space. Besides, the spatio-temporal convolution ψ∗u, which represents competition between the individuals for food resources, is interpreted as a weighted average of the values of u over all past time and over all points in space and has the following form
(ψ∗u)(x,t)=∫R∫t−∞ψ(x−y,t−s)u(y,s)dyds, |
where ψ(x,t) is a given positive kernel function which satisfies
∫R∫+∞0ψ(x,t)dxdt=1. |
For model (1.1), a lot of work had been done by many researchers Britton [1], Gourley [3] and Billingham [4] who had shown that there exist the travelling wave front solution and the periodic travelling wave solution.
However, in the recent several decades there has been an increasing interest in the two species reaction-diffusion equations that incorporate spatially and temporally nonlocal terms in the form of the convolution of a kernel function, see [5,6,7,8,9]. Their studied results demonstrated that the predator-prey models with the nonlocal term have many bifurcation phenomena which lead to the complex spatio-temporal patterns to occur. For example, the work of [5] had shown that when the nonlocal term was introduced the more bifurcation phenomena would occur than the system without the nonlocal term. Besides, in order to explore the mechanism of emergence of complex spatiotemporal patterns, the bifurcation behaviours including Hopf bifurcation, Turing bifurcation, wave bifurcation, Turing-Hopf bifurcation an so on, are also investigated in reaction-diffusion equations by many researchers [10,11,12,13,14,15]. The definitions of these bifurcations are introduced in the references [16,17] and have the important relations with roots of the characteristic equation of the studied system. The complex spatiotemporal patterns which include the spatially inhomogeneous periodic solution, homogeneous solution [10], stationary spatial patterns [11] and quasi-periodic solution [12] are also pointed out by them, respectively.
In this paper, according to [1], we take the particular kernel functions on the basis of a random walk argument as follows
ψ(x,t)=1√4dπtexp(−|x|24dt)τexp(−τt) |
and
ψ(x,t)=1√4dπtexp(−|x|24dt)τ2texp(−τt), |
which are respectively called the weak kernel and strong kernel, where x is the spatial variable, t is the temporal variable, τ=1˜τ and ˜τ called the average time delay means the competition time for food resources. We mainly consider all kinds of bifurcation phenomena including Hopf bifurcation, Turing bifurcation and wave bifurcation for Eq (1.1) with the weak and strong kernel functions. We take the inverse of the average time delay in the kernel function as a bifurcation parameter and explore influences of the time delay on the emergence of the different bifurcation behaviours including Hopf bifurcation, Turing bifurcation and wave bifurcation. The studied results have found that there only exists Hopf bifurcation in Eq (1.1) with the weak kernel and there are Hopf bifurcation and wave bifurcation in Eq (1.1) with the strong kernel. Furthermore, we suppose that Eq (1.1) has the positive initial condition ϕ(x) for x∈R. And because there is zero population flux across the boundary tending to the infinity the following homogeneous Neumman boundary condition ∂u(x,t)∂n=0 is supposed for Eq (1.1), where n is the outward unit normal vector of the boundary which tends to infinity.
The rest of this paper is organized as follows. In Section 2, the bifurcation analyses of a single-species reaction-diffusion model with spatiotemporal delay including the weak kernel and the strong kernel is given, respectively. The conditions of occurrence of Hopf and wave bifurcation are also obtained. In Section 3, we investigate the effect of the time delay on the spatiotemporal patterns through the numerical method. We finally give some conclusions in Section 4.
In this section we will employ the linearized analysis to study bifurcation phenomena of Eq (1.1) as the parameter τ varies. The conditions of the emergence of Hopf bifurcation, Turing bifurcation and wave bifurcation in Eq (1.1) under the weak kernel and strong kernel are also given, respectively.
When the kernel function has the following weak form
ψ(x,t)=1√4dπtexp(−|x|24dt)τexp(−τt), |
according to the results in the references [1,18], we let
v(x,t)=ψ∗u, | (2.1) |
which leads to transform Eq (1.1) into the following form
{∂u∂t=dΔu+f1(u,v),∂v∂t=dΔv+f2(u,v), | (2.2) |
where f1(u,v)=ru(1+αu−βu2−(1+α−β)v), f2(u,v)=τ(u−v). Eq (2.2) has three equilibrium points (0,0), (1,1) and (−1β,−1β). From the viewpoints of population biology, we pay our attention to the equilibrium point E+=(1,1) which corresponds to the maximum state of the studied species. The linearized equations of system (2.2) are as follows
{∂u∂t=dΔu+a11u+a12v,∂v∂t=dΔv+a21u+a22v, | (2.3) |
where
a11=∂f1(u,v)∂u|u=1,v=1=r(α−2β),a12=∂f1(u,v)∂v|u=1,v=1=−r(1+α−β),a21=∂f2(u,v)∂u|u=1,v=1=τ,a22=∂f2(u,v)∂v|u=1,v=1=−τ. |
Let
(uv)=(c1c2)exp(λt+ik⋅r), | (2.4) |
where c1 and c2 are coefficients, k denotes the wave numbers in the X direction, and λ is the growth rate of perturbation in time t, i is the imaginary unit and i2=−1, r is the spatial vector in one-dimensional space. Thus, substituting Eq (2.4) into Eq (2.3), we get the characteristic equation which is
λ2+Tr(k2)λ+Δ(k2)=0, | (2.5) |
where Tr(k2)=2dk2−a11−a22 and Δ(k2)=(dk2−a11)(dk2−a22)−a12a21.
Assume that α>2β, from the equation Tr(0)=a11+a22=0, we obtain
τ=τHc=r(α−2β). | (2.6) |
That is to say, when τ=τHc, the characteristic Eq (2.5) for k2=0 becomes
λ2+a11a22−a12a21=0, |
which has a pair of purely imaginary roots ±√a11a22−a12a21=±√rτHc(1+β). Besides, the following transversality condition
dReλdτ|τ=τHc=−12<0 |
is also satisfied.
When k2=0, the roots of Eq (2.5) will determine the local stability of the equilibrium point E+. If the equilibrium point E+ is stable for k2=0, then for k2≠0, it is also stable because Tr(k2)=2dk2+Tr(0) and Δ(k2)=d2k4+Tr(0)k2+Δ(0) are both positive under the conditions of Tr(0) and Δ(0)>0 through using the Routh-Hurwtiz criterion. Connecting the above analyses, the following results are obtained immediately.
Theorem 2.1 Assume that f1 and f2∈C1(R2,R), α>2β, Tr0=τ−r(α−2β), Δ0=rτ(1+β) and τ=τHc is defined by (2.6).
(i) When k2=0, the equilibrium point E+ is asymptotically stable for τ>τHc and unstable for 0≤τ<τHc, and Eq (2.2) undergoes Hopf bifurcation at τ=τHc which leads to the spatially homogeneous periodic solution to occur;
(ii) When k2≠0, the equilibrium point E+ of Eq (2.2) is also stable because roots of the characteristic Eq (2.5) have the negative real parts under the conditions of Tr(k2)=2dk2+Tr0, Δ(k2)=d2k4+Tr0k2+Δ0, Tr0>0 and Δ0>0. That is to say, there are not Turing bifurcation and wave bifurcation to occur.
When the kernel function has the following strong form
ψ(x,t)=1√4dπtexp(−|x|24dt)τ2texp(−τt), |
we use the same symbols as the subsection 2.1 for the convenience of our studies and let
{v(x,t)=∫R∫t−∞1√4dπ(t−s)exp(−|x−y|24d(t−s))τ2(t−s)exp(−τ(t−s))u(y,s)dyds,w(x,t)=∫R∫t−∞1√4dπ(t−s)exp(−|x−y|24d(t−s))τexp(−τ(t−s))u(y,s)dyds, | (2.7) |
according to the results in the references [1,18], which lead to (1.1) to transform into the following form
{∂u∂t=dΔu+g1(u,v,w),∂v∂t=dΔv+g2(u,v,w),∂w∂t=dΔw+g3(u,v,w), | (2.8) |
where g1(u,v,w)=ru(1+αu−βu2−(1+α−β)v), g2(u,v,w)=τ(w−v) and g3(u,v,w)=τ(u−w). Similar to Eq (2.2), Eq (2.8) has three equilibrium points (0,0,0), (1,1,1) and (−1β,−1β,−1β).
The linearized equations of (2.8) at E+=(1,1,1) are
{∂u∂t=dΔu+b11u+b12v+b13w,∂v∂t=dΔv+b21u+b22v+b23w,∂w∂t=dΔw+b31u+b32v+b33w, | (2.9) |
where
b11=∂g1(u,v,w)∂u|u=1,v=1,w=1=r(α−2β),b12=∂g1(u,v,w)∂v|u=1,v=1,w=1=−r(1+α−β),b13=∂g1(u,v,w)∂w|u=1,v=1,w=1=0,b21=∂g2(u,v,w)∂u|u=1,v=1,w=1=0,b22=∂g2(u,v,w)∂v|u=1,v=1,w=1=−τ,b23=∂g2(u,v,w)∂v|u=1,v=1,w=1=τ,b31=∂g3(u,v,w)∂u|u=1,v=1,w=1=τ,b32=∂g3(u,v,w)∂v|u=1,v=1,w=1=0,b33=∂g3(u,v,w)∂v|u=1,v=1,w=1=−τ. |
Similarly, we can get the characteristic equation as follows
λ3+m1(k2)λ2+m2(k2)λ+m3(k2)=0, | (2.10) |
where
m1(k2)=3dk2−b11+2τ,m2(k2)=(dk2+τ)2+2(dk2+τ)(dk2−b11),m3(k2)=(dk2+τ)2(dk2−b11)−τ2b12. |
According to the Routh-Hurwitz criterion, the conditions of the emergence of Hopf bifurcation, Turing bifurcation and wave bifurcation have the relation with the distribution of roots of Eq (2.10) at k2=0 and k2≠0, respectively. That is to say, we need to discuss whether the following results hold.
For k2=0, if m1(0)>0, m3(0)>0 and m1(0)m2(0)−m3(0)>0 are satisfied, then the roots of Eq (2.10) have the negative real parts which lead the equilibrium point E+ to be asymptotically stable.
For k2=0, if m1(0)>0 and m1(0)m2(0)−m3(0)=0 hold, then Eq (2.10) has a pair of purely imaginary roots which lead to Hopf bifurcation to occur at the equilibrium point E+.
For k2≠0, when the characteristic Eq (2.10) has a zero root and the other roots with negative real parts under the conditions of m1(0)>0,m2(0)>0 and m3(0)>0, there is possible Turing bifurcation to occur in Eq (2.8).
For k2≠0, when the characteristic Eq (2.10) has a pair of purely imaginary roots and the another root with negative real part under the conditions of m1(0)>0,m2(0)>0 and m3(0)>0, there possibly exist wave bifurcation in Eq (2.8).
According to the above discussions of the root distributions of Eq (2.10) the following results are obtained immediately.
Theorem 2.2 Assume that g1,g2 and g3∈C1(R3,R), 17β−9α−1<0, m1(0)>0, m3(0)>0 and τ=τshc±, τ=τswc are defined by (2.13) and (2.19), respectively.
(i) For k2=0, the constant equilibrium point E+ is asymptotically stable for 0≤τ<τshc− and τ>τshc+ and unstable for τshc−<τ<τshc+, and Eq (2.8) undergoes the Hopf bifurcation at τ=τshc±;
(ii) For k2≠0, under the conditions of m1(0)>0, m3(0)>0 and m1(0)m2(0)−m3(0)>0, there does not exist the Turing bifurcation in Eq (2.8);
(iii) For k2≠0, under the conditions of conditions of m1(0)>0, m3(0)>0 and m1(0)m2(0)−m3(0)>0, Eq (2.8) undergoes the wave bifurcation at τ=τswc at the critical wave number k=kwc=b11−τswc2d.
Proof: (i) For k2=0, the characteristic equation becomes into
λ3+m1(0)λ2+m2(0)λ+m3(0)=0, | (2.11) |
where m1(0)=2τ−b11, m2(0)=τ2−2τb11 and m3(0)=−τ2(b11+b12). If Eq (2.8) exists the Hopf bifurcation, the sufficient conditions are that Eq (2.11) has a pair of purely imaginary roots ±iω0(ω0>0) and the another root has the negative real part. Substituting λ=±iω0 into the Eq (2.11), we get
m1(0)m2(0)−m3(0)=0. | (2.12) |
Through the detailed calculations about Eq (2.12), under the condition of 17β−9α−1<0 the critical value of the bifurcation parameter τ is as follows
τ={τshc+=4b11−b12+√b212−8b11b124,τshc−=4b11−b12−√b212−8b11b124. | (2.13) |
And the another root of Eq (2.11) is λ=−m1(0). In order to obtain the transversality condition, we need to differentiate about the parameter τ on the two sides of Eq (2.11) and get
dλdτ=−λ2dm1(0)dτ−λdm2(0)dτ−dm3(0)dτ3λ2+2m1(0)λ+m2(0). | (2.14) |
Connecting
dm1(0)dτ=2,dm2(0)dτ=2τ−2b11anddm3(0)dτ=−2τ(b11+b12), |
we have
dλdτ=−2λ2−2λ(τ−b11)+2τ(b11+b12)3λ2+2m1(0)λ+m2(0). |
So when τ=τshc±,
dλdτ|τ=τshc±=ω20+τshc(b11+b12)−ω0(τshc−b11)i−ω20+ω0(2τshc−b11)i. |
And the transversality condition of occurrence of Hopf bifurcation
dReλdτ|τ=τshc±=3(τshc−b11)2ω20+(2τshc−b11)2>0 |
is also satisfied.
(ii) For k2≠0, the conditions of Eq (2.8) undergoing Turing bifurcation are that Eq (2.10) has a zero root and the equilibrium point E+ is asymptotically stable for k2=0. These conditions need the following results simultaneously hold
{m3(k2)=0,m1(0)>0,m3(0)>0,m1(0)m2(0)−m3(0)>0. | (2.15) |
Let z=k2, then
m3(k2)=m3(z)=(dz+τ)2(dz−b11−τ2b12)=d3z3+d2(2τ−b11)z2+dτ(τ−2b11)z−τ2(b11+b12). |
The derivative function of m3(z) about the variable z is
dm3(z)dz=3d3z2+2d2(2τ−b11)z+dτ(τ−2b11)=3d3(z+2τ−b113d)2−d(τ+b11)23. | (2.16) |
Because the zero points of the derivative function dm3(z)dz correspond the local extreme value of m3(z), we get the two zero points z1=−τd and z2=2b11−τ3d from dm3(z)dz=0. Because z=k2 is positive, we can obtain the only zero point z=z2=2b11−τ3d=k2>0 which leads to 2b11−τ>0. And connecting the equality m2(0)=τ(τ−2b11), we get m2(0)<0. Then combining with the inequalities m1(0)>0 and m3(0)>0, we have m1(0)m2(0)−m3(0)<0. There exists a contradiction with m1(0)m2(0)−m3(0)>0. This contradiction demonstrates that for k2≠0 there does not exist Turing bifurcation in Eq (2.8).
(iii) For k2≠0, the conditions of Eq (2.8) undergoing wave bifurcation are that Eq (2.10) has a pair of purely imaginary roots and a root with the negative real and the equilibrium point E+ is asymptotically stable for k2=0. These conditions need also the following results simultaneously hold
{m1(k2)m2(k2)−m3(k2)=0,m1(0)>0,m3(0)>0,m1(0)m2(0)−m3(0)>0. | (2.17) |
In order to get the critical value of the bifurcation parameter τ about the wave bifurcation, we firstly let z=k2 and F(z)=m1(z)m2(z)−m3(z). By the detailed calculations, we get the expression of function F(z) about the variable z as follows
F(z)=8d3z3+8d2(2τ−b11)z2+2d(τ−b11)(5τ−b11)z+τ(2τ−b11)(τ−2b11)+τ2(b11+b12). |
The derivative function of F(z) about the variable z is
dF(z)dz=24d3z2+16d2(2τ−b11)z+2d(τ−b11)(5τ−b11). | (2.18) |
Letting dF(z)dz=0, we obtain that the zero points of the derivative function dF(z)dz are zmin=b11−5τ6d and zmax=b11−τ2d which correspond the local extreme maximum value and local minimum value of the function F(z), respectively. Let the local extreme minimum F(zmax) of the function F(z) be equal to zero, we get the critical value of the bifurcation parameter
τswc=b11b11+b12, | (2.19) |
and the corresponding critical wave number k2wc=zmax=b11−τswc2d. In order to obtain the transversality condition, we need to differentiate about the parameter τ on the two sides of Eq (2.10) and get
dλdτ=−λ2dm1(k2)dτ−λdm2(k2)dτ−dm3(k2)dτ3λ2+2m1(k2)λ+m2(k2). | (2.20) |
Connecting
dm1(k2)dτ=2,dm2(k2)dτ=2(dk2+τ)+2(dk2−b11)anddm3(k2)dτ=2(dk2−b11)(dk2+τ)−2τb12, |
we have
dλdτ=p13λ2+2m1(k2)λ+m2(k2), |
where
p1=−2λ2−2λ((dk2+τ)+(dk2−b11))−2((dk2−b11)(dk2+τ)−τb12). |
So when τ=τswc and k2=k2wc,
dλdτ|τ=τswc=p2q, |
where
p2=ω20−((dk2wc−b11)(dk2wc+τswc)−τswcb12)+ω0((dk2wc+τswc)+(dk2wc−b11))i |
and
q=−ω20+m1(k2wc)ω0i. |
The transversality condition of the wave bifurcation
dReλdτ|τ=τswc=τshcr(1+α−β)ω20+m21(k2wc)>0 |
is also satisfied. The proof is completed.
In this section we will give some numerical results when this single-species reaction-diffusion model with the strong kernel function. According to the results in Theorem 2.2 of subsection 2.2, we fix d=1,r=2,β=0.2 and take the parameters τ and α as variables. The pictures of Hopf bifurcation curve and wave bifurcation curve are given in the parameter plane spanned by τ and α, see Figure 1.
Next, we fix the parameters α=0.32 and obtain the critical value of τshc−=0.333939 about Hopf bifurcation and the critical value of τswc=0.066667 about wave bifurcation, respectively. If we take τ=0.01, τ=0.05 and τ=0.2, respectively corresponding to the points A,B and C, we would get the spatiotemporal evolution pictures of u with the initial conditions u(0,x)=1−0.1cos(1.3x),v(0,x)=1−0.1cos(1.3x) and w(0,x)=1−0.1cos(1.3x), see Figure 2 (A–C).
Because of τ=0.01<0.333939=τshc−, Figure 2 (A) presents that the state of u will tend to the stable state E+=(1,1,1) as the time varies.
Because of τ=0.05 and τshc−=0.333939<0.05<0.066667=τswc, Figure 2 (B) describes that there exists the spatially homogeneous and temporally periodic state induced by Hopf bifurcation.
Because of τ=0.2>0.066667=τswc, Figure 2 (C) shows that there exists the temporally oscillation pattern induced by the interactions of Hopf bifurcation and wave bifurcation. Because the Hopf bifurcation is much more prominent than the wave bifurcation, we would obtain the seriously oscillatory pattern in the temporal direction.
Besides, if we take the parameters α=0.8 and τ=0.05 corresponding to the point D, we get the spatiotemporal pictures about wave bifurcation which leads to the spatially heterogeneous pattern to occur, see Figure 2 (D).
The bifurcation behaviours resulting in the occurrence of all kinds of patterns in the reaction-diffusion equations have been focused in the mathematical biology. It is well known that these bifurcation behaviors including Hopf bifurcation, Turing bifurcation, wave bifurcation and interactions of them are reported by many researchers. Recently, the spatiotemporal patterns in the reaction-diffusion equations due to the presence of the nonlocal reaction have been a fascinating subject on the study of the pattern formation [5,8]. What's more, the effect of the kernel functions on the spatial pattern formation are also discussed by [19]. In this paper, we mainly pay our attention to influences of the different kernel function, which are respectively called weak kernel and strong kernel, on pattern formation in a single-species reaction-diffusion equation. We have found that when the weak kernel function is introduced there only exists Hopf bifurcation which induces the spatially homogeneous pattern to occur, but when the strong kernel is introduced there are Hopf bifurcation and wave bifurcation which can induce the spatially homogeneous pattern and the spatially heterogeneous pattern to occur, respectively. It is shown that the rich spatiotemporal patterns occur when the different kernel functions are introduced.
We would like to thank the reviewers for their valuable comments and suggestions, which can significantly improve the quality of our paper indeed. This work is supported by grants from Natural Science Basic Research Program of Shaanxi (No.2019JM-444).
The authors declare that they have no known competing financial interests or personal relationships that could have appeared to influence the work reported in this paper.
[1] | Z. Han, Research on Strategies to Enhance the Competitiveness of China's Steel Industry, ph.D thsis, Chinese Academy of Social Sciences, 2014. |
[2] | C. Zhang, Research on Energy Efficiency of My Country's Steel Enterprises Based on Circular Economy, ph.D thsis, University of Science and Technology Beijing, 2018. |
[3] |
L. Malka, F. Bidaj, A. Kuriqi, A. Jaku, R. Roçi, A. Gebremedhin, Energy system analysis with a focus on future energy demand projections: the case of Norway, Energy, 272 (2023), 127107. https://doi.org/10.1016/j.energy.2023.127107 doi: 10.1016/j.energy.2023.127107
![]() |
[4] |
X. Liu, S. Yuan, H. Yu, Z. Liu, How ecological policy stringency moderates the influence of industrial innovation on environmental sustainability: The role of renewable energy transition in BRICST countries, Renewable Energy, 207 (2023), 194–204. https://doi.org/10.1016/j.renene.2023.01.045 doi: 10.1016/j.renene.2023.01.045
![]() |
[5] |
G. P. Perunov, Y. V. Inatovich, N. A. Strashkova, The power consumption optimization for thin-sheet rolling on a reversing mill, Steel Transl., 50 (2020), 897–901. https://doi.org/10.3103/S0967091220120116 doi: 10.3103/S0967091220120116
![]() |
[6] |
M. Tan, H. Yang, B. Duan, Y. Su, F. He, Optimizing production scheduling of steel plate hot rolling for economic load dispatch under time‐of‐use electricity pricing, Math. Probl. Eng., 2017 (2017), 1048081. https://doi.org/10.1155/2017/1048081 doi: 10.1155/2017/1048081
![]() |
[7] |
L. Giorleo, E. Ceretti, C. Giardini, Energy consumption reduction in Ring Rolling processes: A FEM analysis, Int. J. Mech. Sci., 74 (2013), 55–64. https://doi.org/10.1016/j.ijmecsci.2013.04.008 doi: 10.1016/j.ijmecsci.2013.04.008
![]() |
[8] |
J. Paralikas, K. Salonitis, G. Chryssolouris, Energy efficiency of cold roll forming process, Int. J. Adv. Manuf. Technol., 66 (2013), 1271–1284. https://doi.org/10.1007/s00170-012-4405-8 doi: 10.1007/s00170-012-4405-8
![]() |
[9] | V. Chubenko, A. Khinotskaya, T. Yarosh, L. Saithareiev, Sustainable development of the steel plate hot rolling technology due to energy-power process parameters justification, in E3S Web of Conferences, EDP Sciences, 166 (2020), 06009. |
[10] |
J. Qiu, J. Feng, X. Huang, Z. Huang, Optimization of reheating furnace rolling delay strategies based on a minimum energy consumption principle, J. Iron. Steel Res. Int., 2024 (2024), 1–13. https://doi.org/10.1007/s42243-024-01227-0 doi: 10.1007/s42243-024-01227-0
![]() |
[11] | X. Chen, Y. Yang, Data-driven multi-objective optimization of power consumption and quality for finishing rolling process, in 2024 IEEE 13th Data Driven Control and Learning Systems Conference (DDCLS), IEEE, (2024), 1262–1266. https://doi.org/10.1109/DDCLS61622.2024.10606881 |
[12] |
Y. Zhong, J. Wang, J. Xu, S. Wu, J. Rao, K. Dang, Prediction of energy consumption in horizontal roughing process of hot rolling strip based on TDADE algorithm, IEEE Trans. Autom. Sci. Eng., 21 (2023), 555–568. https://doi.org/10.1109/TASE.2023.3236306 doi: 10.1109/TASE.2023.3236306
![]() |
[13] |
Y. T. Kim, B. J. Kim, S. W. Kim, Multi-level stacked regression for predicting electricity consumption of hot rolling mill, Expert Syst. Appl., 201 (2022), 117040. https://doi.org/10.1016/j.eswa.2022.117040 doi: 10.1016/j.eswa.2022.117040
![]() |
[14] | X. Xiong, D. Deng, Y. Xiao, Q. Guo, Y. Zhang, Research on prediction method of finish rolling power consumption of multi-specific strip steel based on random forest optimization model, in 2020 39th Chinese Control Conference (CCC), IEEE, (2020), 5977–5984. https://doi.org/10.23919/CCC50068.2020.9188937 |
[15] |
S. Behzadipour, A. Khajepour, J. G. Lenard, J. Biglou, A new shape change quantification method for estimation of power in shape rolling, J. Mater. Process. Technol., 148 (2004), 353–361. https://doi.org/10.1016/j.jmatprotec.2004.02.047 doi: 10.1016/j.jmatprotec.2004.02.047
![]() |
[16] |
G. A. Orcajo, J. Rodríguez, P. Ardura, J. M. Cano, J. G. Norniella, T. R. Llera, et al., Dynamic estimation of electrical demand in hot rolling mills, IEEE Trans. Ind. Appl., 52 (2016), 2714–2723. https://doi.org/10.1109/TIA.2016.2533483 doi: 10.1109/TIA.2016.2533483
![]() |
[17] | Y. Sun, Model and Control of Hot Strip Rolling, Metallurgical Industry Press, Beijing, 2007. |
[18] |
R. B. Sims, The calculation of roll force and torque in hot rolling, Proc. Inst. Mech. Eng., 168 (1954), 191–200. https://doi.org/10.1243/PIME_PROC_1954_168_023_02 doi: 10.1243/PIME_PROC_1954_168_023_02
![]() |
[19] |
J. Guo, X. Wang, W. Xue, Y. Zhao, System identification with binary-valued observations under data tampering attacks, IEEE Trans. Autom. Control, 66 (2021), 3825–3832. https://doi.org/10.1109/TAC.2020.3029325 doi: 10.1109/TAC.2020.3029325
![]() |
[20] |
J. Guo, Q. Zhang, Y. Zhao, Identification of FIR Systems with binary-valued observations under replay attacks, Automatica, 172 (2025), 112001. https://doi.org/10.1016/j.automatica.2024.112001 doi: 10.1016/j.automatica.2024.112001
![]() |
[21] | H. Valecha, A. Varma, I. Khare, A. Sachdeva, M. Goyal, Prediction of consumer behaviour using random forest algorithm, in 2018 5th IEEE Uttar Pradesh Section International Conference on Electrical, Electronics and Computer Engineering (UPCON), IEEE, (2018), 1–6. https://doi.org/10.1109/UPCON.2018.8597070 |
1. | Osmin Ferrer, José Guerra, Alberto Reyes, Transcritical bifurcation in a multiparametric nonlinear system, 2022, 7, 2473-6988, 13803, 10.3934/math.2022761 | |
2. | Yong-Li Song, Gao-Xiang Yang, Theoretical mechanism of boundary-driven instability of the reaction–diffusion population system, 2025, 18, 1793-5245, 10.1142/S1793524523500912 |