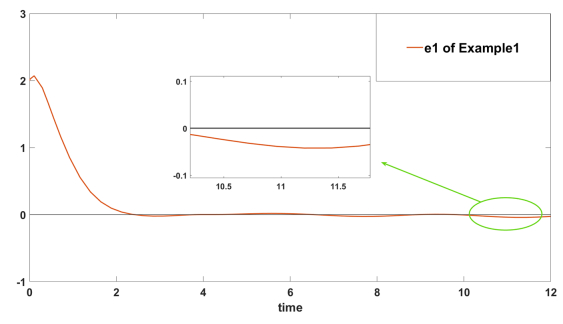
Previous works have analyzed finite/fixed-time tracking control for nonlinear systems. In these works, achieving the accurate time convergence of errors must be under the premise of known initial values and careful design of control parameters. Then, how to break through the constraints of initial values and design parameters for this issue is an unsolved problem. Motivated by this, we successfully studied prescribed-time tracking control for single-input single-output nonlinear systems with uncertainties. Specifically, we designed a state feedback controller on [0,Tp), based on the backstepping method, to make the tracking error (TE) tend to zero at Tp, in which Tp is the arbitrarily selected prescribed-time. Furthermore, on [Tp,∞), another controller, similarly to that on [0,Tp), was designed to keep TE within a precision after Tp, while TE may not stay at zero. Therefore, on [Tp,∞), another new controller, based on sliding mode control, was built to ensure that TE stays at zero after Tp.
Citation: Lichao Feng, Chunlei Zhang, Mahmoud Abdel-Aty, Jinde Cao, Fawaz E. Alsaadi. Prescribed-time trajectory tracking control for a class of nonlinear system[J]. Electronic Research Archive, 2024, 32(12): 6535-6552. doi: 10.3934/era.2024305
[1] | Qianqian Zhang, Mingye Mu, Heyuan Ji, Qiushi Wang, Xingyu Wang . An adaptive type-2 fuzzy sliding mode tracking controller for a robotic manipulator. Electronic Research Archive, 2023, 31(7): 3791-3813. doi: 10.3934/era.2023193 |
[2] | Lingling Zhang . Vibration analysis and multi-state feedback control of maglev vehicle-guideway coupling system. Electronic Research Archive, 2022, 30(10): 3887-3901. doi: 10.3934/era.2022198 |
[3] | Duhui Chang, Yan Geng . Distributed data-driven iterative learning control for multi-agent systems with unknown input-output coupled parameters. Electronic Research Archive, 2025, 33(2): 867-889. doi: 10.3934/era.2025039 |
[4] | Zhizhou Zhang, Yueliang Pan, Weilong Zhao, Jinchu Zhang, Zheng Zi, Yuan Xie, Hehong Zhang . Frequency analysis of a discrete-time fast nonlinear tracking differentiator algorithm based on isochronic region method. Electronic Research Archive, 2024, 32(9): 5157-5175. doi: 10.3934/era.2024238 |
[5] | Jian Gong, Yuan Zhao, Jinde Cao, Wei Huang . Platoon-based collision-free control for connected and automated vehicles at non-signalized intersections. Electronic Research Archive, 2023, 31(4): 2149-2174. doi: 10.3934/era.2023111 |
[6] | Chao Ma, Hang Gao, Wei Wu . Adaptive learning nonsynchronous control of nonlinear hidden Markov jump systems with limited mode information. Electronic Research Archive, 2023, 31(11): 6746-6762. doi: 10.3934/era.2023340 |
[7] | Tongtong Yu, Zhizhou Zhang, Yang Li, Weilong Zhao, Jinchu Zhang . Improved active disturbance rejection controller for rotor system of magnetic levitation turbomachinery. Electronic Research Archive, 2023, 31(3): 1570-1586. doi: 10.3934/era.2023080 |
[8] | Xingting Geng, Jianwen Feng, Yi Zhao, Na Li, Jingyi Wang . Fixed-time synchronization of nonlinear coupled memristive neural networks with time delays via sliding-mode control. Electronic Research Archive, 2023, 31(6): 3291-3308. doi: 10.3934/era.2023166 |
[9] | Ning Li, Yuequn Gao . Modified fractional order social media addiction modeling and sliding mode control considering a professionally operating population. Electronic Research Archive, 2024, 32(6): 4043-4073. doi: 10.3934/era.2024182 |
[10] | Chao Yang, Juntao Wu, Zhengyang Qiao . An improved fixed-time stabilization problem of delayed coupled memristor-based neural networks with pinning control and indefinite derivative approach. Electronic Research Archive, 2023, 31(5): 2428-2446. doi: 10.3934/era.2023123 |
Previous works have analyzed finite/fixed-time tracking control for nonlinear systems. In these works, achieving the accurate time convergence of errors must be under the premise of known initial values and careful design of control parameters. Then, how to break through the constraints of initial values and design parameters for this issue is an unsolved problem. Motivated by this, we successfully studied prescribed-time tracking control for single-input single-output nonlinear systems with uncertainties. Specifically, we designed a state feedback controller on [0,Tp), based on the backstepping method, to make the tracking error (TE) tend to zero at Tp, in which Tp is the arbitrarily selected prescribed-time. Furthermore, on [Tp,∞), another controller, similarly to that on [0,Tp), was designed to keep TE within a precision after Tp, while TE may not stay at zero. Therefore, on [Tp,∞), another new controller, based on sliding mode control, was built to ensure that TE stays at zero after Tp.
The stability of automatic systems, being a significant property, has been extensively studied for several decades. Compared with asymptotic stability [1,2,3,4,5,6,7], finite-time stability is more practical, having been first introduced in [8]. Sliding mode control (SMC), a classical method, can achieve finite-time stability effectively due to its simplicity and high robustness. Furthermore, high-order sliding modes and terminal sliding modes were developed in subsequent research [9,10,11]. However, due to the discontinuous switching function, high-frequency chattering occurs in sliding mode control, restricting its practical application. To overcome this flaw, a method based on Lyapunov differential inequality was proposed to realize finite-time control [12,13]. For finite-time stability, although the system can converge to the equilibrium solution in a finite time, the estimation of the settling time always requires knowledge about the initial conditions. Nevertheless, in many real cases, the initial system conditions are unavailable, such as in state monitoring exceptions for unmanned aerial vehicles caused by sensor failure [14]. As a result, fixed-time stability (FxT stability) was introduced, in which the upper bound of settling time can be presented irrespective of initial values. The FxT control can be traced back to [15], which used polynomial feedback and modifications of the second-order SMC algorithm to achieve the stabilization of the linear system. Later, the FxT control, implemented by odd-order plus fractional-order feedback, was applied to higher-order nonlinear systems and multi-agent systems [16,17].
Although the FxT scheme can provide the upper bound of settling time by adjusting control parameters, choosing proper parameters is a complex problem. Furthermore, achieving convergence at a desired moment by FxT control is almost impossible. To overcome this, the prescribed-time (PT) stability was introduced [18] by converting the original system to a new one via a time-varying transformation; the desired convergent time was independent of arbitrarily designed parameters and initial conditions. In recent years, this interesting feature has attracted more and more attention [19,20,21,22,23,24,25,26,27,28,29,30,31,32,33], and various methods of prescribed-time control have been proposed, such as nonlinear feedback [21], extracting the characteristics of systems to design the controller [22], parametric Lyapunov equation [23,24], SMC [25], time transformation function [26], and Lyapunov differential inequality [29].
For special strict-feedback systems, our research focus, some results on PT control have been reported [27,28,30]. With the development of PT control, the PT tracking issue has emerged. By importing a new time-varying function, the PT tracking issue of nonlinear systems was achieved [31]. Based on the Barrier Lyapunov function, PT tracking control with pre-set properties for known nonlinear systems was achieved [32]. In [33], authors investigated PT tracking for completely certain systems in strict feedback form using the backstepping method. However, it should be noted that systems are not always fully observed in many cases. For systems with uncertainties, such as unknown functions and disturbance, the methods in [32,33] seem to be limited. Hence, a natural question arises: For nonlinear systems with uncertainties, can the prescribed-time tracking control be realized by the single-control approach, such as the backstepping approach, at the prescribed time and afterward? If not, another question emerges: can hybrid control approaches, such as the backstepping method and sliding mode control, be combined to achieve it? Answering these two questions is the main motivation of this paper.
Based on the above discussions, we will explore the single backstepping approach and the combined approach of backstepping and SMC to implement the PT tracking control for the single-input single-output (SISO) nonlinear system accompanied by uncertainties. The detailed contributions are listed as follows:
1) For the SISO system accompanied by unknown functions and disturbance, a state feedback controller on [0,Tp), by backstepping method, is designed to make the tracking error (TE) tend to zero at the prescribed time Tp (not dependent on initial values and designed parameters). Additionally, on [0,Tp), the controller can always be kept bounded.
2) A further controller on [Tp,∞), by backstepping method similarly to 1), is imported to keep the TE within a precision after Tp. It should be pointed out that the TE may not stay at zero after Tp, which is the motivation of 3).
3) Another further controller on [Tp,∞), by SMC, is introduced to guarantee that the TE stays at zero after Tp, which compensates the deficiency of 2).
Consider the SISO nonlinear system as follows:
{˙xj=xj+1+fj(ˉxj),j=1,...,n−1,˙xn=g(x)u+fn(x)+d(t),y=x1, | (1) |
in which xj∈Rn is the state, g(x)≠0 is a known continuous function, x=[x1,...,xn]T∈Rn and fj(ˉxj), ˉxj=[x1,…,xj]T∈Rj,j=1,…,n−1 are known nonlinear and continuous functions, fn(x) is an unknown nonlinear continuous function, u∈R denotes the control input, d(t) denotes a bounded continuous disturbance, and y∈R denotes the output.
The goal is to design a controller to allow y(t) to track the pre-set trajectory x1d(t). To implement our control scheme, the following lemma and assumptions are listed.
Lemma 1 ([34]): Consider a scalar differential equation:
˙H(s)=F(H(s),s),H(s0)=H(0), |
in which F(H(s),s) is the continuity on s and local Lipschitz continuity on H(s), for ∀s≥0, H(s)∈M⊂R. Denote [s0,T) by the maximal interval for solution H(s), and suppose H(s)∈M⊂R for ∀s∈[s0,T). Assume Q(s) is continuous with Dini upper right-hand D+Q(s),
D+Q(s)≤F(H(s),s),Q(s0)≤H(s0), |
with Q(s)∈M⊂R for ∀s∈[s0,T). Then, Q(s)≤H(s), ∀s∈[s0,T).
Assumption 1: A smooth and bounded function ˉf(x) and number λ exist for
|fn(x)|≤ˉf(x),|d(t)|≤λ. |
Assumption 2: For ∀j=1,...,n, fj(ˉxj) and x1d(t) is smooth enough to be differentiable of order n.
This assumption is a general consideration of the backstepping design, see [32,33,35,36].
Before designing the controller, let us define the following n-dimensional error variables:
e1=x1−η1,ej=xj−ηj,j=2,...,n, | (2) |
where η1=x1d is the desired trajectory we will track, and ηj,j=2,...,n are the virtual controllers that will be designed later.
In this section, we will design a controller to implement the PT tracking control for system (1). The controller is divided into two phases–prescribed-time tracking controller up when 0≤t<Tp and infinite-time tracking controller ũ when t≥Tp.
Case 1 (0≤t<Tp): We use the backstepping method to design the controller at this stage. It contains n steps.
Step 1: The derivative of e1 is
˙e1=e2+η2+f1(ˉx1)−˙η1, | (3) |
According to Eq (3), we design the virtual controller η2 as
η2=−ke1Tp−t+˙η1−f1(ˉx1), | (4) |
where k>n>0, then
˙e1=e2−ke1Tp−t. | (5) |
Choose
V1=0.5e21, | (6) |
then, along Eq (3),
˙V1=−ke21Tp−t+e1e2. | (7) |
Step 2: The derivative of e2 is
˙e2=e3+η3−˙η2+f2(ˉx2), | (8) |
then the virtual controller η3 is designed as
η3=−ke2Tp−t−e1+˙η2−f2(ˉx2). | (9) |
Substituting Eq (9) into Eq (8), we can get
˙e2=e3−e1−ke2Tp−t. | (10) |
Introduce Lyapunov function V2=V1+0.5e22, then the derivative of V2 satisfies
˙V2=˙V1+e2˙e2=−ke21Tp−t−ke22Tp−t+e2e3. | (11) |
Step j(j=3,...n−1): From Eq (7) to Eq (11), we can get the derivative of ej:
˙ej=ej+1+ηj+1−˙ηj+fj(ˉxj). | (12) |
The ηj+1 can be set as
ηj+1=−kejTp−t−ej−1+˙ηj−fj(ˉxj). | (13) |
Then
˙ej=ej+1−ej−1−kejTp−t. | (14) |
Select Vj=Vj−1+0.5ej2 and its derivative satisfies
˙Vj=−kTp−t∑ji=1e2i+ejej+1. | (15) |
Step n: From Eq (2), the derivative of en is
˙en=g(x)u+fn(x)+d(t)−˙ηn. | (16) |
Choosing Lyapunov function as V=Vn−1+0.5en2, the controller up is designed
up=g(x)−1(−kenTp−t−en−1−en(ˉf(x)+λ)22δ+˙ηn), | (17) |
where δ is a positive constant.
From Eqs (15)–(17), the derivatives of en and Vn satisfy
˙en=−kenTp−t−en−1−en(ˉf(x)+λ)22δ+fn(x)+d(t), |
˙Vn=˙Vn−1−ke2nTp−t+fn(x)en+d(t)en−e2n(ˉf(x)+λ)22δ+enen−1=−kTp−t∑nj=1e2j+fn(x)en+d(t)en−e2n(ˉf(x)+λ)22δ≤−kTp−t∑nj=1e2j+ˉf(x)|en|+λ|en|−e2n(ˉf(x)+λ)22δ. | (18) |
By Young's inequality, based on Eq (18), we can get
˙Vn≤−kTp−t∑nj=1e2j+δ2=−2kVnTP−t+δ2. | (19) |
Case 2 (t≥Tp): For this case, we will design an infinite-time controller to ensure the tracking effect when t≥Tp. First, define a new serious of error variables
z1=e1=x1−η1,zj=xj− ηj,j=2,...,n. | (20) |
Step 1: Easily get
˙z1=z2+ η2−˙η1+f1(ˉx1). | (21) |
According to Eq (20), we design the new virtual controller η2 as
η2=−σz1+˙η1−f1(ˉx1), | (22) |
where σ>0 is a positive constant, then ˙z1 becomes
˙z1=z2−σz1. | (23) |
Choose
V1=0.5z12, | (24) |
then along Eq (20),
˙ V1=z1˙z1=−σz12+z1z2. | (25) |
Similarly to Case 1, we can design the subsequent backstepping as follows:
Step j (j=2,...,n): one can get
˙zj=zj+1+ ηj+1−˙ ηj+fj(ˉxj). | (26) |
The ηj+1 can be set as
ηj+1=−σzj−zj−1+˙ ηj−fj(ˉxj). | (27) |
Then
˙zj=zj+1−zj−1−σzj. | (28) |
Select Vj= Vj−1+0.5zj2, and its derivative satisfies
˙ Vj=−σ∑ji=1zi2+zjzj+1. | (29) |
Step n: From Eq (20),
˙zn=g(x)u+fn(x)+d(t)−˙ ηn. | (30) |
Choose Vn= Vn−1+0.5zn2, the controller ũ is designed as
˜u=g(x)−1(−σzn−zn−1−zn(ˉf(x)+λ)22δ+˙ ηn). | (31) |
From Eqs (29)–(31),
˙ Vn≤−σ∑nj=1zj2+δ2=−2σ Vn+δ2. | (32) |
Remark 1: We adopt different control gains in Cases 1 and 2 according to different control targets. The prescribed-time controller aims to make the error converge to zero at any desired prescribed-time Tp, hence a time-varying infinite gain function k(Tp−t)−1 is imported into the controller design of Case 1. On the other hand, in Case 2, our goal is to keep the convergence of the tracking error at Tp, so a constant σ is adopted as control gain.
Based on the above analysis, we can get the following assertion.
Theorem 1: If Assumptions 1 and 2 hold, one can design the controllers (17) and (31) such that the system (1) can track the pre-set trajectory x1d(t) within the prescribed time Tp and keep tracking within a range (i.e., the tracking error is bounded). Controller (17) is bounded when t→Tp−.
Proof: The proof is divided into two parts due to complexity.
Part 1: Based on Eq (19), we provide the proof of prescribed-time convergence for ˙Vn=−2kVnTP−t+δ2, and then apply Lemma 1 to the case of ˙Vn<−2kVnTP−t+δ2.
It can be obtained by ˙Vn=−2kVnTP−t+δ2 that
Vn(t)=C(1−tTp)2k+δ2(2k−1)(Tp−t), | (33) |
where C=Vn(ej(0))Tp2k−δ2(2k−1)Tp2k−1 is a constant. It can easily be found that limt→Tp−Vn(t)=0. For Vn=12∑nj=1e2j, it means limt→Tp−ej=0, j=1,...,n.
Then, we will verify the boundness of the virtual controllers ηj and u.
Based on Eq (5), we can get
e1(t)=e1(0)(1−tTp)k+(Tp−t)k∫t0e2(s)(Tp−s)kds, | (34) |
then we have
e1(t)Tp−t=e1(0)Tp−1(1−tTp)k−1+(Tp−t)k−1∫t0e2(s)(Tp−s)kds. | (35) |
By L'Hôpital's rule for (Tp−t)k−1∫t0e2(s)(Tp−s)kds, we can obtain
limt→Tp−e1(t)Tp−t=limt→Tp−e2(t)k−1=0, | (36) |
which implies η2 is bounded when t→Tp− from Eq (4). According to Eqs (5) and (36), we know limt→TP−˙e1(t)=0.
By the same method, we can get
ej(t)=ej(0)(1−tTp)k+(Tp−t)k∫t0ej+1(s)−ej−1(s)(Tp−s)kds,j=2,...,n−1,en(t)=en(0)Tp−k(Tp−t)kω(t)−1+(Tp−t)kω(t)−1∫t0(fn(x(s))+d(s)−en−1(s))ω(s)(Tp−s)kds,limt→Tp−ej(t)Tp−t=limt→Tp−ej+1(t)−ej−1(t)k−1=0,j=2,…,n−1,limt→Tp−en(t)Tp−t=limt→TP−(fn(x(t))+d(t)−en−1(t))ω(t)k−1≤(ˉf(x(Tp))+λ)ω(Tp)k−1, | (37) |
where ω(t)=e∫t0(ˉf(x(s))+λ)22δds, which means that all virtual controllers ηj and up are bounded when time t→Tp− and limt→TP−˙ej(t)=0, j=2,...n.
Part 2: From Eqs (34) and (37), using L'Hôpital's rule, we can get
limt→Tp−e1(t)(Tp−t)m=limt→Tp−1k−me2(t)(Tp−t)m−1,limt→Tp−ej(t)(Tp−t)m=limt→Tp−1k−m[ej+1(t)(Tp−t)m−1−ej−1(t)(Tp−t)m−1],j=2,...,n−1, | (38) |
for any integer m∈(0,k).
From Eq (38), we have
limt→Tp−e1(t)Tp−t=limt→Tp−e2(t)k−1=0,limt→Tp−e1(t)(Tp−t)2=limt→Tp−1k−2e2(t)(Tp−t)=0,limt→Tp−e1(t)(Tp−t)3=limt→Tp−1k−3e2(t)(Tp−t)2=limt→Tp−1k−31k−2[e3(t)Tp−t−e1(t)Tp−t]=limt→Tp−1k−31k−2e3(t)(Tp−t)=0. | (39) |
Then, we can easily obtain
limt→Tp−e1(t)(Tp−t)n=limt→Tp−(k−m)!(k−1)!en(t)(Tp−t). | (40) |
Due to the fact that limt→Tp−en(t)Tp−t is bounded, from Assumption 2, using L'Hôpital's rule to Eq (40), we can get
limt→Tp−e1(t)(j)(n−j)!(−1)jn!(Tp−t)n−j=0,j=1,...,n−1, | (41) |
which means limt→Tp−e1(t)(j)=limt→Tp−z1(t)(j)=0,j=1,...,n−1. From Eq (23), we can get
˙z1(Tp)=z2(Tp)−σz1(Tp)=0, | (42) |
then z2(Tp)=0. From Eq (28), we can get
¨z1(Tp)=z3(Tp)−z1(Tp)−σz2(Tp)−σ˙z1(Tp)=0, | (43) |
then z3(Tp)=0. Similarly, we can obtain zj(Tp)=0, j=4,...,n, which implies Vn(Tp)=0.
According to Lemma 1, from Eq (32),
Vn(t)≤( Vn(Tp)−δ4σ)e−2σ(t−Tp)+δ4σ≤δ4σ, | (44) |
which implies z1≤√δ2σ when t≥Tp. The proof is completed.
Remark 2: The designed controller based on the backstepping approach up can make the system error converge to zero at Tp, which is consistent with the effect achieved in [33]. That means we can achieve tracking control of nonlinear systems with uncertain function and disturbance by the backstepping approach. Nevertheless, we must point out that the effect of the unknown function fn(x) and disturbance d(t) cannot be precisely eliminated, and that the tracking error cannot be maintained at zero after Tp, which can be later illustrated by our example. Of course, we can adjust the control parameters to keep the tracking error within a controllable range. This gives a direct answer to the first question mentioned above.
It should be noted that, from Theorem 1, the single backstepping approach can only ensure that the error is within a controllable range after the prescribed time rather than staying at zero. However, some practical applications need to ensure accurate tracking, such as the tracking control of spacecraft [25]. This means that, other than the backstepping approach, other approaches need to be imported to guarantee the tracking error remains at zero after the prescribed time. Considering that SMC has a great advantage in countering disturbances through the input channel, SMC method is imported to the design controller, so as to achieve zero error tracking when t≥Tp. The controller design is as follows.
When 0≤t<Tp, the controller design based on the backstepping method can be referred to Case 1 of Section 3.1. Then, we mainly design the controller using SMC to ensure that the tracking error is kept at zero.
First, we use e1(t) and its derivatives to build the following system for the SMC design:
ξ1(t)=e1(t)=x1(t)−x1d(t),ξj(t)=˙ξj−1(t)=e1(t)(j−1),j=2,...,n. | (45) |
The SMC variable is set as
s(t)=∑nj=1ξj(t),j=1,...,n, | (46) |
then
˙s=∑nj=2xj+∑n−1j=1fj(ˉxj)−∑nj=1x1d(j)+∑n−1l=1∑n−lj=1fj(ˉxj)(l)+g(x)u+fn(x)+d(t). | (47) |
The controller u is designed as
us=g(x)−1[−(ˉf(x)+λ)sgn(s)−ϵs−∑nj=2xj−∑n−1j=1fj(ˉxj)+∑nj=1x1d(j)−∑n−1l=1∑n−lj=1fj(ˉxj)(l)], | (48) |
where ϵ>0 is a constant.
Theorem 2: If Assumptions 1 and 2 hold, one can design the controllers (17) and (48) such that the system (1) can track the pre-set trajectory x1d(t) within the prescribed time Tp and keep the tracking error stay at zero after Tp, and controller (17) is bounded when t→Tp−.
Proof: Substituting Eq (48) into Eq (47), we can obtain
˙s=fn(x)+d(t)−(ˉf(x)+λ)sgn(s)−ϵs. | (49) |
Choose Vs=0.5s2, then from Assumption 2, the derivative of Vs satisfies
˙Vs=(fn(x)+d(t))s−(ˉf(x)+λ)ssgn(s)−ϵs2≤0. | (50) |
From assertions Eq (38) to Eq (41), we have ξ1(Tp)(j−1)=ξj(Tp)=˙ξj−1(Tp)=0, j=2,...,n, which means error system (45) converge to zero when t=Tp. Additionally, from Eq (50), we can obtain that error ξ1(t) stays at zero when t≥Tp. The proof is completed.
Remark 3: It can be shown from Theorems 1 and 2 that, compared with the infinite time controller (31), the sliding mode controller (48) introduces a sign function sign(.) to forcibly cancel the effects of unknown functions and perturbation, and then zero tracking error is achieved. Of course, the sliding mode controller (48) requests greater control costs due to the introduction of a sign function. Therefore, in the application, we can choose the appropriate controller, whether infinite controller (31) or sliding mode controller (48), according to the practiced requirements.
Remark 4: On the FxT tracking control scheme, the tracking error can converge to zero without relying on the initial state. However, it is necessary to adjust the design parameters carefully to achieve arbitrary time convergence through the FxT method, see [35,36]. In our control scheme, the error convergence can be realized at any desired time without considering any initial conditions and designed parameters, which is simpler and more convenient. Contrary to [18,19], the prescribed-time control method here is feasible for t∈[0,∞) rather than only valid for [0,Tp). Prescribed-time attitude tracking control of spacecraft was proposed by SMC [25], where the upper bound of the uncertainty is a constant. Compared to [25] and [33], our assumptions are broader, which makes our method applicable to more general systems.
Remark 5: Compared with predefined-time control giving an upper bound on settling time [37], prescribed-time control can give an exact settling time. The prescribed-time tracking control, as an application of prescribed-time control, also gives an exact settling time. For the difference between predefined-time control and prescribed-time control, readers are referred to [38]. Prescribed performance control, such as [39], always refers to a control method with system state and convergence speed as expected, with no explicit requirement for settling time. The advantage of our proposed method lies in that it can give an exact settling time that does not depend on control parameters and initial values.
Consider a nonlinear system as follows
{˙x1=x2+x12/3−0.5x1,˙x2=(x12+1)u+ax1+bsin(x1x2)+ccost,y=x1, | (51) |
where ax1+bsin(x1x2) and ccos(t) represent the uncertain function fn(x) and disturbance d(t), respectively, g(x)=x12+1. The target trajectory is selected as x1d=sin(t). Here, we select the parameters as a=0.1, b=1, c=0.2. Then, we can obtain the upper bound of fn(x) and d(t) as follows:
ˉf(x)=0.1|x1|+1, |
λ=0.2. |
Based on the proposed method, the prescribed-time controller up can be designed as
up=1x12+1[−ke2Tp−t−e1+˙η2−e2(1+0.1|x1|+0.2)22δ], | (52) |
where η2=−k(x1−x1d)Tp−t+˙x1d−x12/3+0.5x1, e1=x1−x1d, e2=x2−η2.
The infinite time controller ũ is
˜u=−σz2+˙ η2−z1−z2(1+0.1|x1|+0.2)22δ, | (53) |
where η2=−σz2+˙x1d−x12/3+0.5x1, z1=x1−x1d, z2=x2− η2.
The sliding mode controller us is designed as
us=1x12+1[−(1.2+0.1|x1|)sgn(s)−ϵs+˙x1d−x2+¨x1d−x12/3+0.5x1−(23x1−1/3+0.5˙)x1], | (54) |
where s=ξ1+˙ξ1,ξ1=x1−x1d. We design two examples with different initial states and control parameters for simulation.
Example 1: The controller parameters are selected as k=4, δ=0.09, σ=0.5, Tp=4, ϵ=0.1 and the initial state is [2,2]; then, the track error under the action of ũ satisfies |e1|≤0.3 when t≥4.
Example 2: The controller parameters are selected as k=3, δ=0.04, σ=2, Tp=4, ϵ=0.2 and the initial state is [3,2.5]; then, the track error under the action of ũ satisfies |e1|≤0.1 when t≥4.
The simulation results of the two examples under the action of the prescribed-time controller up and the infinite time controller ũ are shown in Figures 1–4.
As we can see from Figures 1 and 3, although the initial conditions and designed parameters of two examples are different, all tracking errors converge to zero at Tp=4, and the output y keeps tracking x1d(t) after Tp with the precision being less than √δ∕2σ under the single action of backstepping control, which is consistent with Remark 2. Additionally, Figures 2 and 4 illustrate that all control inputs u are bounded for t→Tp.
In order to verify the effectiveness of the sliding mode controller us, Figures 5–8 provide the simulation results of two examples under the action of the prescribed-time controller up and the sliding mode controller us.
Figures 5 and 7 show that all tracking errors converge to zero at Tp and stay at zero after Tp under the hybrid action of backstepping controller and sliding mode controller. At the same time, from Figures 6 and 8, the control input remains bounded and has discontinuity after t≥4 due to the introduction of the sign function, which is consistent with Remark 3. Numerical simulations are demonstrated to verify the proposed theory.
In our manuscript, a PT tracking control for the SISO nonlinear system is proposed. Under the prescribed-time controller and infinite controller designed by the backstepping method in Theorem 1, the system output can track the expected trajectory at any desired time, and the tracking error can be limited to a range, which gives an answer to the first question in the Introduction. Additionally, we design a controller in Theorem 2 combining the backstepping method and sliding mode method to make the tracking error stay at zero rather than in a range, which gives an answer to the second question. Although an infinite gain function is introduced, the control behavior exhibits boundedness over the entire time domain. It is worth mentioning that the convergence time does not depend on initial values and designed parameters. Future work may focus on prescribed-time control for other nonlinear systems, such as network control systems [40].
The authors declare they have not used Artificial Intelligence (AI) tools in the creation of this article.
This project was jointly supported by the National Natural Science Foundation of China (61833005), the Humanities and Social Science Fund of Ministry of Education of China (23YJAZH031), the Natural Science Foundation of Hebei Province of China (A2023209002, A2019209005), the Tangshan Science and Technology Bureau Program of Hebei Province of China (24130201C), and the Fundamental Research Funds for Hebei Province Universities: North China University of Science and Technology (No. JJC2024045). This project was funded by the Deanship of Scientific Research (DSR) at King Abdulaziz University, Jeddah, Saudi Arabia, under grant no. (GPIP: 1798-611-2024). The authors, therefore, acknowledge with thanks DSR for technical and financial support.
Jinde Cao is one of the special issue editors for Electronic Research Archive, and was not involved in the editorial review or the decision to publish this article. All authors declare that there are no competing interests.
[1] | A. Y. Aleksandrov, E. B. Aleksandrova, A. P. Zhabko, On the asymptotic stability with respect to a part of variables of solutions of nonlinear systems with delay, in 2016 International Conference Stability and Oscillations of Nonlinear Control Systems, IEEE, (2016), 1–3. https://doi.org/10.1109/STAB.2016.7541155 |
[2] |
M. Bucolo, A. Buscarino, L. Fortuna, S. Gagliano, A new asymptotic stability criterion for linear discrete-time systems, IEEE Trans. Circuits Syst. II Express Briefs, 69 (2022), 4994–4998. https://doi.org/10.1109/TCSII.2022.3187864 doi: 10.1109/TCSII.2022.3187864
![]() |
[3] |
F. Zhang, Z. Zeng, Asymptotic stability and synchronization of fractional-order neural networks with unbounded time-varying delays, IEEE Trans. Syst. Man Cybern.: Syst., 51 (2021), 5547–5556. https://doi.org/10.1109/TSMC.2019.2956320 doi: 10.1109/TSMC.2019.2956320
![]() |
[4] |
L. Feng, S. Li, X. Mao, Asymptotic stability and boundedness of stochastic functional differential equations with Markovian switching, J. Franklin Inst., 353 (2016), 4924–4949. https://doi.org/10.1016/j.jfranklin.2016.09.017 doi: 10.1016/j.jfranklin.2016.09.017
![]() |
[5] |
L. Feng, L. Liu, J. Cao, L. Rutkowski, G. Lu, General decay stability for non-autonomous neutral stochastic systems with time-varying delays and Markovian switching, IEEE Trans. Cybern., 52 (2022), 5441–5453. https://doi.org/10.1109/TCYB.2020.3031992 doi: 10.1109/TCYB.2020.3031992
![]() |
[6] |
H. Lin, H. Zeng, X. Zhang, W. Wang, Stability analysis for delayed neural networks via a generalized reciprocally convex inequality, IEEE Trans. Neural Networks Learn. Syst., 34 (2023), 7491–7499. https://doi.org/10.1109/TNNLS.2022.3144032 doi: 10.1109/TNNLS.2022.3144032
![]() |
[7] |
T. Peng, H. Zeng, W. Wang, X. Zhang, X. Liu, General and less conservative criteria on stability and stabilization of T–S fuzzy systems with time-varying delay, IEEE Trans. Fuzzy Syst., 31 (2023), 1531–1541. https://doi.org/10.1109/TFUZZ.2022.3204899 doi: 10.1109/TFUZZ.2022.3204899
![]() |
[8] |
L. Weiss, E. Infante, Finite time stability under perturbing forces and on product spaces, IEEE Trans. Autom. Control, 12 (1967), 54–59. https://doi.org/10.1109/TAC.1967.1098483 doi: 10.1109/TAC.1967.1098483
![]() |
[9] |
Y. Wu, X. Yu, Z. Man, Terminal sliding mode control design for uncertain dynamic systems, Syst. Control Lett., 34 (1998), 281–287. https://doi.org/10.1016/S0167-6911(98)00036-X doi: 10.1016/S0167-6911(98)00036-X
![]() |
[10] |
A. Levant, Homogeneity approach to high-order sliding mode design, Automatica, 41 (2005), 823–830. https://doi.org/10.1016/j.automatica.2004.11.029 doi: 10.1016/j.automatica.2004.11.029
![]() |
[11] |
M. Chen, Q. Wu, R. Cui, Terminal sliding mode tracking control for a class of SISO uncertain nonlinear systems, ISA Trans., 52 (2013), 198–206. https://doi.org/10.1016/j.isatra.2012.09.009 doi: 10.1016/j.isatra.2012.09.009
![]() |
[12] |
S. Bhat, D. Bernstein, Finite-time stability of continuous autonomous systems, SIAM J. Control Optim., 38 (2000), 751–766. https://doi.org/10.1137/S0363012997321358 doi: 10.1137/S0363012997321358
![]() |
[13] |
Y. Shen, Y. Huang, Global finite-time stabilisation for a class of nonlinear systems, Int. J. Syst. Sci., 43 (2012), 73–78. https://doi.org/10.1080/00207721003770569 doi: 10.1080/00207721003770569
![]() |
[14] |
M. Ahmad, M. Akram, M. Mohsan, K. Saghar, R. Ahmad, W. Butt, Transformer-based sensor failure prediction and classification framework for UAVs, Expert Syst. Appl., 248 (2024), 123415. https://doi.org/10.1016/j.eswa.2024.123415 doi: 10.1016/j.eswa.2024.123415
![]() |
[15] |
A. Polyakov, Nonlinear feedback design for fixed-time stabilization of linear control systems, IEEE Trans. Autom. Control, 57 (2012), 2106–2110. https://doi.org/10.1109/TAC.2011.2179869 doi: 10.1109/TAC.2011.2179869
![]() |
[16] |
C. Chen, Z. Sun, Fixed-time stabilisation for a class of high order non-linear systems, IET Control Theory Appl., 12 (2018), 2578–2587. https://doi.org/10.1049/iet-cta.2018.5053 doi: 10.1049/iet-cta.2018.5053
![]() |
[17] |
B. Ning, Q. L. Han, Z. Zuo, L. Ding, Q. Lu, X. Ge, Fixed-time and prescribed-time consensus control of multi-agent systems and its applications: A survey of recent trends and methodologies, IEEE Trans. Ind. Inf., 19 (2023), 1121–1135. https://doi.org/10.1109/TII.2022.3201589 doi: 10.1109/TII.2022.3201589
![]() |
[18] |
Y. Song, Y. Wang, J. Holloway, M. Krstic, Time-varying feedback for regulation of normal-form nonlinear systems in prescribed finite time, Automatica, 83 (2017), 243–251. https://doi.org/10.1016/j.automatica.2017.06.008 doi: 10.1016/j.automatica.2017.06.008
![]() |
[19] |
J. Holloway, M. Krstic, Prescribed-time observers for linear systems in observer canonical form, IEEE Trans. Autom. Control, 64 (2019), 3905–3912. https://doi.org/10.1109/TAC.2018.2890751 doi: 10.1109/TAC.2018.2890751
![]() |
[20] |
N. Espitia, D. Steeves, W. Perruquetti, M. Krstic, Sensor delay compensated prescribed-time observer for LTI systems, Automatica, 135 (2022), 110005. https://doi.org/10.1016/j.automatica.2021.110005 doi: 10.1016/j.automatica.2021.110005
![]() |
[21] |
H. Ye, Y. Song, Prescribed-time control for linear systems in canonical form via nonlinear feedback, IEEE Trans. Syst. Man Cybern.: Syst., 53 (2023), 1126–1135. https://doi.org/10.1109/TSMC.2022.3194908 doi: 10.1109/TSMC.2022.3194908
![]() |
[22] |
A. K. Pal, S. Kamal, S. K. Nagar, B. Bandyopadhyay, L. Fridman, Design of controllers with arbitrary convergence time, Automatica, 112 (2020), 108710. https://doi.org/10.1016/j.automatica.2019.108710 doi: 10.1016/j.automatica.2019.108710
![]() |
[23] |
B. Zhou, Finite-time stability analysis and stabilization by bounded linear time-varying feedback, Automatica, 121 (2020), 109191. https://doi.org/10.1016/j.automatica.2020.109191 doi: 10.1016/j.automatica.2020.109191
![]() |
[24] |
B. Zhou, Y. Shi, Prescribed-time stabilization of a class of nonlinear systems by linear time-varying feedback, IEEE Trans. Autom. Control, 66 (2021), 6123–6130. https://doi.org/10.1109/TAC.2021.3061645 doi: 10.1109/TAC.2021.3061645
![]() |
[25] |
Z. Chen, X. Ju, Z. Wang, Q. Li, The prescribed time sliding mode control for attitude tracking of spacecraft, Asian J. Control, 24 (2022), 1650–1662. https://doi.org/10.1002/asjc.2569 doi: 10.1002/asjc.2569
![]() |
[26] |
D. Tran, T. Yucelen, Finite-time control of perturbed dynamical systems based on a generalized time transformation approach, Syst. Control Lett., 136 (2020), 104605. https://doi.org/10.1016/j.sysconle.2019.104605 doi: 10.1016/j.sysconle.2019.104605
![]() |
[27] |
C. Hua, P. Ning, K. Li, Adaptive prescribed-time control for a class of uncertain nonlinear systems, IEEE Trans. Autom. Control, 67 (2022), 6159–6166. https://doi.org/10.1109/TAC.2021.3130883 doi: 10.1109/TAC.2021.3130883
![]() |
[28] |
C. Hua, H. Li, K. Li, P. Ning, Adaptive prescribed-time control of time-delay nonlinear systems via a double time-varying gain approach, IEEE Trans. Cybern., 53 (2023), 5290–5298. https://doi.org/10.1109/TCYB.2022.3192250 doi: 10.1109/TCYB.2022.3192250
![]() |
[29] |
Y. Song, J. Su, A unified Lyapunov characterization for finite time control and prescribed time control, Int. J. Robust Nonlinear Control, 33 (2021), 2930–2949. https://doi.org/10.1002/rnc.6544 doi: 10.1002/rnc.6544
![]() |
[30] |
H. F. Ye, Y. D. Song, Prescribed-time control of uncertain strict-feedback-like systems, Int. J. Robust Nonlinear Control., 31 (2021), 5281–5297. https://doi.org/10.1002/rnc.5541 doi: 10.1002/rnc.5541
![]() |
[31] | Y. Cao, Y. D. Song, Practical prescribed time tracking control with user pre-determinable precision for uncertain nonlinear systems, in 2020 59th IEEE Conference on Decision and Control (CDC), IEEE, (2020), 3526–3530. https: //doi.org/10.1109/CDC42340.2020.9304143 |
[32] |
R. Ma, L. Fu, J. Fu, Prescribed-time tracking control for nonlinear systems with guaranteed performance, Automatica, 146 (2022), 110573. https://doi.org/10.1016/j.automatica.2022.110573 doi: 10.1016/j.automatica.2022.110573
![]() |
[33] |
Y. Li, R. Ma, X. Tian, Prescribed-time tracking with prescribed performance for a class of strict-feedback nonlinear systems, J. Franklin Inst., 361 (2024), 106638. https://doi.org/10.1016/j.jfranklin.2024.01.039 doi: 10.1016/j.jfranklin.2024.01.039
![]() |
[34] | H. K. Khalil, Nonlinear Systems (3rd edition), Prentice-Hal, 2002. |
[35] |
H. Wang, B. Su, Y. Wang, J. Gao, Adaptive sliding mode fixed-time tracking control based on fixed-time sliding mode disturbance observer with dead-zone input, Complexity, 2019 (2019), 8951382. https://doi.org/10.1155/2019/8951382 doi: 10.1155/2019/8951382
![]() |
[36] |
M. Chen, Y. Li, H. Wang, K. Peng, L. Wu, Adaptive fixed-time tracking control for nonlinear systems based on finite-time command-filtered backstepping, IEEE Trans. Fuzzy Syst., 31 (2023), 1604–1613. https://doi.org/10.1109/TFUZZ.2022.3206507 doi: 10.1109/TFUZZ.2022.3206507
![]() |
[37] |
D. Cui, M. Chali, Z. Xiang, Fuzzy fault-tolerant predefined-time control for switched systems: A singularity-free method, IEEE Trans. Fuzzy Syst., 32 (2024), 1223–1232. https://doi.org/10.1109/TFUZZ.2023.3321688 doi: 10.1109/TFUZZ.2023.3321688
![]() |
[38] |
Y. Song, H. Ye, F. Lewis, Prescribed-time control and its latest developments, IEEE Trans. Syst. Man Cybern.: Syst., 53 (2023), 4102–4116. https://doi.org/10.1109/TSMC.2023.3240751 doi: 10.1109/TSMC.2023.3240751
![]() |
[39] |
D. Cui, C. K. Ahn, Y. Sun, Z. Xiang, Mode-dependent state observer-based prescribed performance control of switched systems, IEEE Trans. Circuits Syst. II Express Briefs, 71 (2024), 3810–3814. https://doi.org/10.1109/TCSII.2024.3370865 doi: 10.1109/TCSII.2024.3370865
![]() |
[40] |
H. Zeng, Z. Zhu, T. Peng, W. Wang, X. Zhang, Robust tracking control design for a class of nonlinear networked control systems considering bounded package dropouts and external disturbance, IEEE Trans. Fuzzy Syst., 32 (2024), 3608–3617. https://doi.org/10.1109/TFUZZ.2024.3377799 doi: 10.1109/TFUZZ.2024.3377799
![]() |