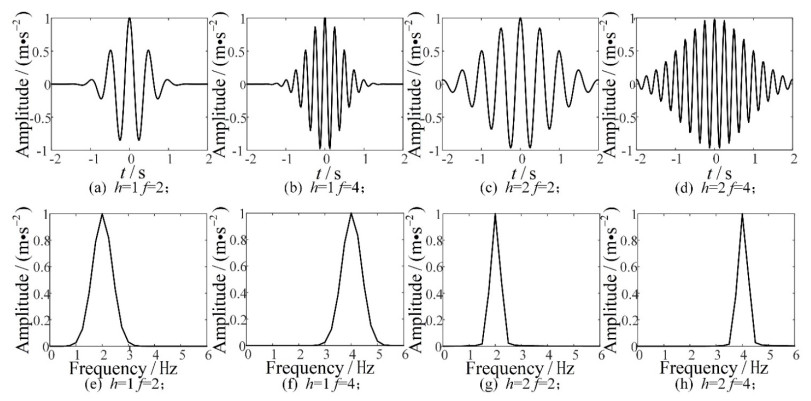
In response to the challenge of noise filtering for the impulsive vibration signals of rolling bearings, this paper presented a novel filtering method based on the improved Morlet wavelet, which has clear physical meaning and is more conducive to parameter optimization through employing Gaussian waveform width to replace the traditional Morlet wavelet shape factor. Simultaneously, the marine predation algorithm was employed and the minimum Shannon entropy was used as the parameter optimization index while optimizing the shape width and center frequency of the improved Morlet wavelet. The vibration waveform of the rolling bearing was matched perfectly by using the optimized Morlet wave. Shannon entropy was used as the evaluation index of noise filtering, and the quantitative analysis of noise filtering was realized. Through experimental validation, this method was proved to be effective in noise elimination for rolling bearing. It is significance to preprocessing of vibration signal, feature extraction and fault recognition of rolling bearing.
Citation: Yu Chen, Qingyang Meng, Zhibo Liu, Zhuanzhe Zhao, Yongming Liu, Zhijian Tu, Haoran Zhu. Research on filtering method of rolling bearing vibration signal based on improved Morlet wavelet[J]. Electronic Research Archive, 2024, 32(1): 241-262. doi: 10.3934/era.2024012
[1] | Shuang Cai, Jiwang Zhang, Cong Li, Zequn He, Zhimin Wang . A RUL prediction method of rolling bearings based on degradation detection and deep BiLSTM. Electronic Research Archive, 2024, 32(5): 3145-3161. doi: 10.3934/era.2024144 |
[2] | Xu Chen, Wenbing Chang, Yongxiang Li, Zhao He, Xiang Ma, Shenghan Zhou . Resnet-1DCNN-REA bearing fault diagnosis method based on multi-source and multi-modal information fusion. Electronic Research Archive, 2024, 32(11): 6276-6300. doi: 10.3934/era.2024292 |
[3] | Yuxia Liu, Qi Zhang, Wei Xiao, Tianguang Chu . Characteristic period analysis of the Chinese stock market using successive one-sided HP filter. Electronic Research Archive, 2023, 31(10): 6120-6133. doi: 10.3934/era.2023311 |
[4] | Ling Li, Dan He, Cheng Zhang . An image filtering method for dataset production. Electronic Research Archive, 2024, 32(6): 4164-4180. doi: 10.3934/era.2024187 |
[5] | Chunhua Chu, Yijun Chen, Qun Zhang, Ying Luo . Joint linear array structure and waveform design for MIMO radar under practical constraints. Electronic Research Archive, 2022, 30(9): 3249-3265. doi: 10.3934/era.2022165 |
[6] | Zhenzhong Xu, Xu Chen, Linchao Yang, Jiangtao Xu, Shenghan Zhou . Multi-modal adaptive feature extraction for early-stage weak fault diagnosis in bearings. Electronic Research Archive, 2024, 32(6): 4074-4095. doi: 10.3934/era.2024183 |
[7] | Tongtong Yu, Zhizhou Zhang, Yang Li, Weilong Zhao, Jinchu Zhang . Improved active disturbance rejection controller for rotor system of magnetic levitation turbomachinery. Electronic Research Archive, 2023, 31(3): 1570-1586. doi: 10.3934/era.2023080 |
[8] | Qiang Guo, Zimeng Zhou, Jie Li, Fengwei Jing . Mechanism- and data-driven algorithms of electrical energy consumption accounting and prediction for medium and heavy plate rolling. Electronic Research Archive, 2025, 33(1): 381-408. doi: 10.3934/era.2025019 |
[9] | Peng Yu, Shuping Tan, Jin Guo, Yong Song . Data-driven optimal controller design for sub-satellite deployment of tethered satellite system. Electronic Research Archive, 2024, 32(1): 505-522. doi: 10.3934/era.2024025 |
[10] | Chengyong Chen, Fagang Chang, Li Li, Wenqiang Dou, Changjing Xu . Optimization of intelligent compaction based on finite element simulation and nonlinear multiple regression. Electronic Research Archive, 2023, 31(5): 2775-2792. doi: 10.3934/era.2023140 |
In response to the challenge of noise filtering for the impulsive vibration signals of rolling bearings, this paper presented a novel filtering method based on the improved Morlet wavelet, which has clear physical meaning and is more conducive to parameter optimization through employing Gaussian waveform width to replace the traditional Morlet wavelet shape factor. Simultaneously, the marine predation algorithm was employed and the minimum Shannon entropy was used as the parameter optimization index while optimizing the shape width and center frequency of the improved Morlet wavelet. The vibration waveform of the rolling bearing was matched perfectly by using the optimized Morlet wave. Shannon entropy was used as the evaluation index of noise filtering, and the quantitative analysis of noise filtering was realized. Through experimental validation, this method was proved to be effective in noise elimination for rolling bearing. It is significance to preprocessing of vibration signal, feature extraction and fault recognition of rolling bearing.
Rolling bearings are the key components of major equipment rotating machinery, in the national economy is known as "industrial joints" [1]. Rolling bearings usually work in high temperature, high speed, high stress and other complex and extreme working environment, its inner ring, outer ring, rolling body, retaining bracket and other parts of the failure is frequent, seriously affecting the safe operation of the whole machine and equipment [2,3]. Research on rolling bearing fault diagnosis methods, timely prediction of early bearing failure, for further enhancing the level of high-end equipment manufacturing, promote the development of intelligent manufacturing in machinery industry is of great strategic significance. However, when the rolling bearing fails, there is a large amount of noise interference in the signal acquisition, which makes it difficult to extract the fault characteristics and affects the accurate identification of the fault state, so the noise reduction of the rolling bearing fault signal is the key to realize the effective diagnosis of the bearing fault state.
Rolling bearing fault diagnosis methods are mainly divided into model-based [4,5,6,7,8] and data-driven [9,10,11,12], both model-based and data-driven fault diagnosis methods rely on high signal-to-noise ratio signals. Rolling bearing vibration signal due to bearing resonance, neighboring gear vibration, sensor vibration and other signal modulation factors, as well as environmental noise, low-frequency signal interference, cage slippage and other factors, resulting in large signal noise, low signal-to-noise ratio, seriously affecting the rolling bearing fault state identification. In order to accurately obtain the rolling bearing fault characteristic signal, reduce the noise and improve the signal-to-noise ratio, the bearing signal data need to be pre-processed for noise reduction [13]. High-quality data preprocessing lays a solid foundation for later feature extraction and screening [14].
The current rolling bearing filtering and noise reduction methods include mathematical morphology filtering [15], EEMD [16,17], wavelet filtering [18], etc. Due to the good local time-frequency characteristics of wavelet analysis, wavelet filtering methods have attracted much attention in rolling bearing filtering and noise reduction [19,20,21]. The types of wavelet functions used in wavelet analysis are diverse and non-unique, including Haar wavelet, Gabor wavelet, db wavelet, Morlet wavelet and so on. And the time domain waveform of Morlet wavelet has the characteristic of fluctuating and decaying from the center of wavelet amplitude to both ends and tends to zero. With the rolling bearing fault vibration signal has the shock attenuation waveform characteristics are very similar, can greatly improve the "waveform" matching degree, so Morlet wavelet is often used for rolling bearing signal noise reduction filtering [22]. Currently, the related literature for traditional Morlet wavelet filtering and noise reduction, most of them are to determine the optimal Morlet wavelet by determining the bandwidth parameter and the center frequency to realize the filtering and noise reduction of the bearing signal. Liang et al. [23] used Morlet wavelet combined with singular value decomposition to achieve effective noise cancellation of mechanical test signals, but the article did not optimize the wavelet parameters, so it is difficult to ensure the stability of the results. Lin and Qu [24] proposed to use Shannon entropy method to optimize the bandwidth parameter of Morlet wavelet to improve the noise reduction effect, but the method did not take into account the center frequency optimization, which made the matching degree of Morlet wavelet with the shock component greatly reduced. Zhang et al. [25] used the entropy value as an index to determine the Morlet wavelet bandwidth parameter and center frequency and used the mixed-wash frog jump algorithm to obtain the optimal Morlet wavelet to realize the filtering and noise reduction of the bearing signal. Tse and Wang [26] used the sparsity of the filtered envelope signal as a fitness function to optimize the center frequency and bandwidth parameters of the Morlet wavelet filter using genetic algorithm to achieve filtering and noise reduction of the signal. Jiang et al. [27] designed a denoising method to achieve optimal matching with impulse signals, in which the improved Shannon wavelet entropy is used to optimize the shape and bandwidth parameters of the conventional Morlet wavelet and the singular value decomposition is also required to select the appropriate transform scale, which in turn achieves the filtering of the signal. Behzad et al. [28] proposed a new Morlet wavelet optimization criterion for the diagnosis of rolling bearings, which optimizes the wavelet parameters according to the amplitude of the bearing eigenfrequency by proposing a new criterion for adjusting the scaling parameters of the continuous wavelet transform. Gu et al. [29] used a complex Morlet wavelet filter for composite fault detection in rolling bearings, in which the envelope spectrum was used to optimize the wavelet parameters and achieve noise reduction on the bearing signals. Zhang et al. [30] used traditional Morlet wavelets for noise reduction of bearing vibration signals, and the optimization process was complicated by determining the bandwidth and center frequency of the Morlet wavelet through local mean decomposition and Shannon entropy criterion. Su et al. [31] proposed a hybrid method combining optimal Morlet wavelet and autocorrelation enhancement algorithms for enhancing periodic impulse features and removing residual noise. Han et al. [32] proposed a fault feature recognition method based on small wavelet filter optimized by genetic algorithm and empirical modal decomposition, in which genetic algorithm is used to optimize the bandwidth parameter and the center frequency of Morlet wavelet filter and singular value decomposition is used to determine the scale of the transformation, to obtain the optimal Morlet wavelet, which can then realize the filtering of vibration signals. All of the above are using traditional Morlet wavelet to achieve bearing signal filtering noise reduction, due to the fuzzy physical meaning of the traditional Morlet wavelet waveform factor, resulting in the complexity of the optimization process of the filtering parameters of the bearing signal, the optimization efficiency is low and so on.
In 2019, Cohen [33] proposed an improved Morlet wavelet using Gaussian waveform width instead of the traditional Morlet wavelet shape factor, which further clarified the physical significance of the Morlet wavelet waveform parameters. And only the improved Morlet wavelet waveform width and center comment rate need to be optimized, which simplifies the bearing signal filtering process. Therefore, this paper adopts the improved Morlet wavelet for noise reduction filtering of rolling bearing vibration signals to realize the optimal matching between the Morlet wavelet waveform and the effective signal waveform of rolling bearing fault vibration. This method can effectively filter out a large number of noise signals and invalid signals, which greatly simplifies the filtering process and realizes the purpose of noise reduction filtering for rolling bearing fault vibration signals.
In order to achieve the best match between the Morlet wavelet and the rolling bearing vibration waveform, the waveform width and center frequency of the improved Morlet wavelet [33] need to be optimized. As intelligent optimization algorithms are intelligent and universal, they are able to maintain high solution accuracy and optimization efficiency when solving many complex optimization problems, among other advantages [34], while the Marine predator algorithm [35] is a meta-heuristic algorithm proposed by Faramarzi et al. in 2020, which has the characteristics of fast convergence speed and balancing the global search capability and local search capability. It also has the advantages of simple structure, few parameters, easy implementation as well as the ability to obtain the optimal solution at a low computational cost, so in this paper, we will introduce the ocean predation algorithm to optimize the parameters of the improved Morlet wavelet waveform width and center frequency. Reasonable selection of the fitness function is a key link in the optimization of the marine predation algorithm to the improved Morlet wavelet parameters. The actual signals of rolling bearings mostly belong to sparse distribution, while the noise belongs to non-sparse distribution [36]. Shannon entropy is a measure of the sparsity of a signal, and its size can reflect the sparse characteristics of the signal intuitively. If the after-filtering signal contains rich regular fault signals, both the signal periodicity characteristics are obvious, then the signal shows strong sparse characteristics, Shannon entropy is smaller, that is, at this time Morlet wavelet filtering parameters optimization is reasonable. If the signal after filtering contains a large number of noise signals or irregular signals, the signal does not contain important fault information, showing weak sparse characteristics, Shannon entropy is larger, that is, at this time, Morlet wavelet filtering parameters are not set reasonably. Nikolaou and Ioannis [37] used Shannon entropy as an indicator to optimize the wavelet waveform parameters for demodulation of rolling bearing vibration signals. The results of Kankar et al. [38] showed that the wavelet optimization method was developed using the minimum Shannon entropy criterion approach, which helps in rolling bearing feature extraction and wavelet parameter optimization. A comparative analysis of wavelet coefficients based on entropy measurements was carried out by Dubey et al. [39]. The results show that using Shannon entropy and average Shannon entropy the best wavelet can be selected among the discrete wavelets. Dong et al. [40] used local average decomposition and its Shannon entropy to analyze the collected bearing vibration signals, effectively extracted the eigenvalues and fault characteristics and completed the fault diagnosis with good results. All in all, it is due to the fact that Shannon entropy is less affected by local noise and interference. Shannon entropy can better represent the similarity between the wavelet function and the signal being analyzed, and using Shannon entropy to optimize the wavelet parameters produces good results. Therefore, in this paper, the minimum Shannon entropy is selected as the fit of the function of the ocean predation algorithm and the improved Morlet wavelet waveform width and center frequency are jointly optimized. Therefore, the two parameter combinations are better matched, reducing the degree of mismatch of the single parameter optimization and then used in combination and increasing the reliability of parameter optimization.
In summary, for the problems of unclear physical significance of traditional Morlet wavelet waveform factors and complicated optimization process, this paper applies the improved Morlet wavelet to the noise reduction filtering of rolling bearing vibration signals. Its use of Gaussian waveform width instead of the traditional Morlet wavelet shape factor makes its physical meaning clear and more conducive to waveform parameter optimization. At the same time, the marine predation algorithm is introduced as the optimization framework and the minimum Shannon entropy is used as the optimization index of Morlet wavelet width and center frequency parameters of the marine predation algorithm to realize the adaptive optimization of Morlet wavelet parameters, simplify the optimization process of Morlet wavelet parameters and improve the rolling bearing fault signal and the optimal Morlet wavelet matching degree, effectively filtering out a large number of noise signals and invalid signals. Thus, the noise reduction filtering of rolling bearing faulty vibration signals is realized.
The main contributions of this paper can be summarized as follows:
In the actual working environment, the obtained rolling bearing fault characteristics signal is mostly a non-smooth sequence of signals, which inevitably contains a large degree of noise, masking the original fault characteristics signal. In order to accurately obtain the rolling bearing fault characteristic signal, reduce the noise and improve the signal-to-noise ratio, it is necessary to carry out data noise reduction preprocessing. In this paper, the improved Morlet wavelet is innovatively applied to the noise reduction filtering of rolling bearing vibration signals. A new way of taking the value of waveform width is proposed, in which the width of single pulse vibration of bearing failure is taken as the upper limit of the waveform width and the minimum value of the improved Morlet waveform width [33] is taken as the lower limit of the waveform width. This method clarifies the specific physical meaning of the wavelet waveform parameters. At the same time, the marine predation algorithm is used as an optimization framework for the improved Morlet wavelet waveform parameters by taking advantage of its excellent global search capability, outstanding local search capability and fast convergence rate. And combined with the ability of Shannon entropy to directly quantify the sparsity of the bearing signal and the magnitude of the signal-to-noise ratio of the response signal, the best optimization of the parameters of the improved Morlet wavelet waveform is achieved. The optimal combination of waveform parameters is used to match the improved Morlet wavelet waveform with the effective signal waveform of rolling bearing fault vibration. The experimental results show that a large number of noise signals and invalid signals can be effectively filtered out, which greatly simplifies the filtering process of the Morlet wavelet and provides a new realization method for the pre-processing of rolling bearing vibration signals.
In this paper, waveform matching of rolling bearing signals based on improved Morlet wavelet and further noise reduction filtering will be realized. The improved Morlet wavelet utilizes a Gaussian waveform width instead of the traditional wavelet shape factor, which is a key parameter used to balance the wavelet time accuracy and frequency accuracy. The improved Morlet wavelet mathematical expression [33] is as follows:
W=e2πifte−4ln(2)t2h2 | (1) |
where h is the width of the modified Morlet wavelet and f is the center frequency.
The Fourier transform of the improved Morlet wavelet W is performed to study the effect of h and f on the time domain and frequency domain characteristics of the improved Morlet wavelet. The results of the simulation are shown in Figure 1.
From the time-domain plots (a)–(d), when h is kept constant and f is increased, the wavelet fluctuation is intensified within the same time width; and when f is kept constant and h is increased, the wavelet oscillation decay time is prolonged. From the frequency domain plots (e)–(h), when f is kept constant and h is increased, the width of the waveform decreases in the frequency domain, indicating a prolonged decay time of the wavelet oscillations. From the above analysis results, it can be seen that the changes of h and f, whether in the time domain or frequency domain, will have an important impact on the improved Morlet wavelet waveform and also affect the improved Morlet wavelet. Furthermore, the rolling bearing fault signal waveform can achieve the best matching of the key parameters. Therefore, the optimization of h and f is the key to achieve the optimal noise reduction filtering for rolling bearings, which is also the focus of the research in this paper.
The improved Morlet wavelet is used for noise reduction filtering of rolling bearing fault vibration signals, which is essentially a wavelet convolution feature to match the bearing vibration signal with the improved Morlet wavelet Gaussian window sine wave, and the result is a time series of "similarity" between the bearing signal and the wavelet. The result is a time series of "similarity" between the bearing signal and the wavelet, so the process of convolution of the bearing signal with the improved Morlet wavelet in the frequency domain is the noise reduction filtering process of the bearing signal. Let x(t) characterize the rolling bearing signal, then the noise reduction filtering process of rolling bearing using improved Morlet wavelet can be expressed as
R{x(t)′}=F−1{X(f)∗ψ(f)} | (2) |
X(f)=FFT(x(t)) | (3) |
ψ(f)=FFT(W) | (4) |
where R{x(t)'} is the signal after x(t) noise reduction filtering, F-1 denotes Fourier inverse transform, * denotes convolution and FFT stands for fast Fourier transform.
From the above equation, it can be seen that the realization of the improved Morlet wavelet on the rolling bearing vibration signal filtering noise reduction only needs to carry out the corresponding Fourier transform of the original rolling bearing signal x(t) and Morlet wavelet W. Then, after the Fourier inverse transform, convolution processing can be quickly realized on the noise reduction filtering of rolling bearing vibration signal.
From the previous analysis, it is clear that the values of h and f directly affect the improved Morlet wavelet's filtering characteristics. In order to make the improved Morlet wavelet better, extract the impact feature components in the vibration signal and improve the rolling bearing fault signal noise reduction filtering effect, it is necessary to jointly optimize the combination of the improved Morlet wavelet width h and the center frequency f, so as to obtain the optimal matching parameters of the rolling bearing waveform and achieve the best filtering effect. That the marine predation algorithm has the advantages of simple structure, few parameters, is easy to implement and is able to obtain the optimal solution at a low computational cost [35]. In this paper, the marine predation algorithm is introduced to construct the prey matrix of the marine predation algorithm using the parameters of improved Morlet wavelet waveform width h and center frequency f. Then, the minimum Shannon entropy is used as the value of adaptive degree. After the optimization operation of the marine predation algorithm in the three stages of exploration–coexistence of exploration and exploitation and exploitation–the optimal combination of the waveform width h and the center frequency f is obtained, which realizes the optimal matching of the improved Morlet wavelet and the waveforms of the vibration signals of the rolling bearings.
As the wavelet waveform width, the value of h is closely related to the width of the single impulse vibration waveform of the bearing fault, so it can be based on the width of the single impulse vibration of the bearing fault as a reference for the h value. According to [33], the lower limit value of wavelet waveform width h optimization can be set as 1/fs, where fs is the sampling frequency and its upper limit value can be set as two times of the theoretical fault vibration period of the rolling bearing to ensure that h is sufficient for the optimization range. The center frequency f roughly reflects the vibration of the waveform within the width of h. In order to expand the range of f optimization, the range of f can be directly set to 0 < f < fs/2, which makes the range of values of h and f as follows:
{f∈(0,fs/2)h∈(1/fs,2/fz) | (5) |
where fz is the theoretical failure characteristic frequency of rolling bearings, which can usually be calculated according to the bearing geometry parameters.
According to the marine predation algorithm [20], the constructed prey matrix position initialization can be calculated according to the following equation
{h0n,d=1/fs+rand×(2/fz−1/fs)f0n,d=rand×fs/2 | (6) |
where rand is a random number uniformly distributed in the range 0 to 1 and the superscript 0 indicates the initial position.
After many iterations of the marine predation algorithm, the prey matrix and elite matrix formed in the t-th iteration can be expressed as follows:
Preyt=[ht1,1ft1,2ht2,1ft2,2⋮⋮htN,1ftN,2],Elite=[hI1,1fI1,2hI2,1fI2,2⋮⋮hIN,1fIN,2] | (7) |
where the first number of the subscript denotes the individual and the second number denotes the dimension, e.g., hn, 1t denotes the first dimension coordinate value of the nth individual; fn, 2t denotes the second dimension coordinate value of the nth individual. The elite matrix is obtained by replicating h1, 1I and f1, 2I N times, where I denotes up to the current t-th iteration and h1, 1I and f1, 2I is a combination that minimizes the adaptation value.
Because rolling bearing vibration signals exhibit super-Gaussian or sparse distributions, noise is non-sparse compared to the effective signal components of bearing vibration. Entropy quantifies the sparseness of a signal's distribution. The smaller the entropy value, the sparser the signal distribution, the more organized the signal is, and the less noise there is [36,37,38,39,40]. And Shannon entropy is the total amount of information in the variable, which can reflect the sparseness of the rolling bearing signal. The smaller the Shannon entropy value, the sparser and more ordered the bearing signal is and the less noise the bearing signal contains. Therefore, the Shannon entropy can be used as a fitness value for the marine predation algorithm in the process of optimizing the improved Morlet wavelet parameters. The Shannon entropy expression is given as
SE(x(t))=−n∑i=1p(xi)log2p(xi) | (8) |
n=1+log2(N) | (9) |
where N is the total number of rolling bearing signal data points, n is the total number of segments and p(xi) is the probability that a bearing signal data point falls within xi.
According to the principle of marine predation algorithm, the designed waveform matching optimization flow of rolling bearing signal based on improved Morlet wavelet is shown in Figure 2, where T denotes the number of iterations.
First, the h and f search ranges are set respectively, where the upper and lower limits of h and f can be assigned according to Eq (5) and the prey initial matrix is constructed according to Eq (6) to complete the initialization of its position; taking each individual (h, f) in the prey initial matrix as an improved Morlet wavelet parameter, according to Eqs (2)–(4) of the rolling bearing vibration signal filtering noise reduction and the Shannon entropy of the filtered signal is calculated according to Eqs (8) and (9). This Shannon entropy is used as the adaptive degree value of the marine predation algorithm and the elite matrix is constructed with the prey individual corresponding to the minimum adaptive degree value. Second, the elite and prey matrices are updated based on the current iteration number t at the stage in the whole iteration cycle and based on the iterative process of the marine predation algorithm's exploration optimization process, hybrid exploration, development co-optimization process, and development optimization process. Finally, after each completed update, its impact on the fitness value is evaluated based on the FADs effect, and if the fitness value of the prey position under the current iteration is lower than that of the previous iteration, the new prey position will replace the previous one, completing the prey matrix update, and vice versa unchanged. That is, the (h, f) combination corresponding to the minimum fitness value within the iteration number T steps is obtained, i.e., the optimal matching parameter between the faulty vibration waveform of the rolling bearing and the improved Morlet wavelet.
In this section, XJTU-SY rolling bearing accelerated life test dataset [41] is used to experimentally validate the method of this paper, the shaft speed fr is 37.5 Hz, the radial force is 11 KN, the sampling frequency fs is 25.6 kHz and the model of rolling bearing is LDK UER204, and its specific geometric parameters are shown in Table 1.
Bearing type | Inside diameter (DI/mm) | Outside diameter (DO/mm) | Ball diameter (d/mm) | Number of rolling elements (Z) | Angle of load from radial plane (a/rad) |
Pitch diameter (D/mm) |
LDK UER204 Ball bearing |
29.3 | 39.8 | 7.92 | 8 | 0 | 34.55 |
The theoretical failure frequency of rolling bearings is related to shaft speed, bearing geometry and defect location. The theoretical failure frequency fIR of the inner ring of the bearing, the theoretical failure frequency fOR of the outer ring of the bearing and the theoretical failure frequency fFTF of the bearing cage are calculated as follows:
fIR=zfr2(1+dDcos(α)) | (10) |
fOR=zfr2(1−dDcos(α)) | (11) |
fFTF=fr2(1−dDcos(α)) | (12) |
where fr is the shaft speed, D is the pitch diameter, d is the ball diameter, Z is the number of rolling elements and α is the angle of the load from the radial plane. The calculated theoretical failure frequency of rolling bearings is shown in Table 2.
Experimental condition | Data set | Sample size | Failure location | Selected csv files | Theoretical failure frequency (fz/Hz) |
Rotation speed: 37.5 Hz Radial force: 11 KN |
Bearing2_1 | 491 | Inner ring | EF: 418.csv; MF: 451.csv; TF: 476.csv; |
184.39 |
Bearing2_2 | 161 | Outer ring | EF: 136.csv; MF: 148.csv; TF: 156.csv; |
115.61 | |
Bearing2_3 | 533 | Cage | EF: 453.csv; MF: 489.csv; TF: 517.csv; |
14.45 |
According to the results of bearing degradation related research [42], the bearing motion state is normal in less than 80% of the operating time period during the full-life operation of the bearing. In 80–90% of the operating time period, the bearing will be in early failure (EF); in 90–95% of the operating time period, the bearing will be in mid-term failure (MF) and in the remaining operating time period, the bearing will be in terminal failure (TF). According to the bearing full life cycle operation law, respectively take the bearing early, middle and late failure corresponding to the data file samples, its intercepted sample data information is shown in Table 2.
Early failure vibration data (EF: 136.csv) of the outer ring was used to validate the effectiveness of noise reduction filtering of the method in this paper. fs is 25.6 kHz and fz is 115.61 Hz, the search range of the improved Morlet wavelet width h is [3.91e-5, 1.73e-2] and the search range of f is [0, 12800], which is calculated according to Eq (5). For the marine predation algorithm, population size is usually 30–50 and the maximum number of iterations T is usually 100–300. In order to realize the optimal h and f optimization results, this paper sets the population size to 50, the maximum number of iterations T to 300 and sets the FADs to 0.2.
Based on the waveform matching optimization process of rolling bearing vibration signal with improved Morlet wavelet, the outer ring early failure vibration data (EF: 136.csv) is subjected to noise reduction filtering operation and the iterative curve of waveform matching optimization is shown in Figure 3. The Shannon entropy SE shows a trend of gradual convergence, with a minimum SE of 2.01 at the completion of the iteration.
Filtering noise reduction results are shown in Figure 4(b), the filtered signal compared with the original signal impact components increased significantly, the filtered signal noise compared with the original signal noise is significantly reduced and the filtered bearing failure vibration impact signal has a certain periodicity, verifying the effectiveness of this paper's filtering noise reduction method.
The envelope analysis [43] of the filtered signal is shown in Figure 5. The fundamental frequency of the actual eigenfrequency of the outer ring fault obtained by the envelope analysis is similar to the theoretical fault frequency. Factors such as environmental noise and cage slippage may be the main reasons for the deviation between the actual and theoretical frequencies.
In addition, we can clearly see the outer ring theoretical failure characteristic frequency of the base frequency of 2 times the frequency (230.4 Hz), 3 times the frequency (345.6 Hz), etc., which can be judged at this time bearings occur in the outer ring failure.
The synthesis of the above analysis results shows that the method proposed in this paper is effective in noise reduction filtering of early fault signals in the outer ring.
In order to further illustrate the advantages of the method proposed in this paper and compare the method of this paper with other wavelet filtering noise reduction methods, the outer ring early failure vibration data (EF: 136.csv) is subjected to wavelet soft-threshold filtering for noise reduction, and the obtained results are shown in Figure 4(c). The vibration impact component of the signal after wavelet soft threshold filtering is not obvious compared with the filtering results of the proposed method, and the Shannon entropy of the signal after wavelet soft threshold filtering is further calculated to be 2.40, which is greater than the Shannon entropy value of the signal filtered by the method in this paper 2.01, indicating that the signal noise component after wavelet soft threshold noise reduction is more. The envelope analysis of the filtered signal is performed and the results are shown in Figure 6.
The difference between the base frequency of the actual outer ring fault eigenfrequency (116.4 Hz) and the theoretical fault frequency (115.6 Hz) is 0.8 Hz, while the base frequency of the outer ring fault eigenfrequency (115.2 Hz) obtained by the method of this paper differs from the theoretical fault frequency only by 0.4 Hz, which further demonstrates that the method of this paper has better filtering and noise reduction filtering effect.
Using the rolling bearing vibration signal filtering method based on improved Morlet wavelet proposed in this paper, all the data samples selected in Table 2 are sequentially subjected to noise reduction filtering and the results are shown in Table 3. In order to further verify the applicability of this method for different fault types and fault degrees of rolling bearings, the signal Shannon entropy before and after filtering is subjected to the calculation of impairment, and the formula is shown in Eq (13). When the rolling bearing's calculated S value is larger in a certain kind of fault period, that in this case the method of bearing vibration signal contains noise elimination is better. It also shows that the less noise the signal contains after filtering, the better the filtering noise reduction effect.
S=(1−SE After filteringSEBefore filtering)×100% | (13) |
Data set and Failure location | Selected csv files | [hOptimal, fOptimal] | SEBefore filtering | SEAfter filtering | S |
Bearing2_1 inner ring |
EF: 418.csv; | [3.8464e-3, 1419.5204] | 3.0839 | 2.9927 | 2.96% |
MF: 451.csv; | [2.9960e-3, 2059.1770] | 2.9559 | 2.7446 | 7.15% | |
TF: 476.csv; | [6.1656e-4, 4058.7670] | 2.8998 | 2.3868 | 17.69% | |
Bearing2_2 outer ring | EF: 136.csv; | [1.7853e-3, 7621.4035] | 2.7099 | 2.0108 | 25.80% |
MF: 148.csv; | [7.3316e-4, 9719.7542] | 2.7018 | 2.0500 | 24.12% | |
TF: 156.csv; | [2.1630e-3, 12210.3952] | 3.1519 | 2.1851 | 30.67% | |
Bearing2_3 cage |
EF: 453.csv; | [2.3897e-3, 12174.4453] | 2.6797 | 2.4388 | 8.99% |
MF: 489.csv; | [1.1186e-3, 9726.5546] | 2.7697 | 2.4038 | 13.21% | |
TF: 517.csv; | [7.5635e-4, 3430.0532] | 2.8322 | 2.1330 | 24.69% |
From Table 3, it can be seen that whether it is an inner ring fault, outer ring fault or cage fault, the Shannon entropy of the signal after filtering is smaller than the Shannon entropy before filtering, that is to say, the filtered signal noise is reduced and the signal-to-noise ratio is increased. The Shannon entropy impairment value after filtering for all fault degrees of each type is greater than zero, i.e., it shows that this method has noise reduction filtering effect for all fault types of vibration signals and can effectively improve the signal-to-noise ratio of bearing vibration signals.
Observing S in Table 3, it is found that the impairment value of this method after filtering the data of various types of bearing failures in various periods varies from 2.96–30.67%. Among them, the vibration signal impairment value of bearing outer ring terminal failure (TF) is 30.67%, which indicates that this method has the best filtering effect on this signal and improves the signal-to-noise ratio of the signal the most, which can provide a high-quality data source for the subsequent feature extraction and fault diagnosis. The impairment value for the outer ring early failure signal is 25.80%, which is combined with the analysis of the results before and after filtering of the outer ring early failure signal in Section 4.1.1 to conclude that after filtering the signal compared with the original signal vibration shock components increased significantly and the vibration shock of the signal has a certain periodicity. The signal noise after filtering is significantly reduced compared to the original signal noise. The difference between the actual fault frequency and the theoretical fault frequency of the signal after filtering is significantly reduced. It can be shown that the Shannon entropy and Shannon entropy impairment value can have a certain assessment of the bearing signal filtering effect.
In addition, the Shannon entropy impairment value of the bearing outer ring fault signal after filtering in each period is greater than the maximum of other fault types, indicating that the filtering effect of the outer ring fault signal is the most obvious and the cage fault signal is the second. For the same fault type, the Shannon entropy reduction of terminal failure is greater than that of other periods, which is due to the fact that the vibration signal of terminal failure contains more noise. Also, the vibration amplitude of the bearing is larger, which is also in line with the actual working condition of the bearing, so the method in this paper is more effective for noise reduction filtering of strong noise signals.
In summary, the method in this paper has a certain effect on the rolling bearing fault vibration signal noise reduction filtering, which can effectively reduce the invalid signal and a large number of noise signals in the fault signal. It can effectively improve the signal-to-noise ratio of the signal and accurately obtain high-quality and reliable rolling bearing fault signals. For the next rolling bearing feature extraction, providing a high-quality signal source will improve the accuracy of fault identification and the reliability of life prediction.
In order to further validate the practical effectiveness of the present methodology, the proposed methodology will be validated again by utilizing the drive-end bearing fault data from the CWRU bearing dataset [44] at a sampling frequency of 12 kHz (fs = 12 kHz), using the fault data shown in Table 4. The rolling bearing type is 6205-2RS JEM SKF and its geometrical parameters are shown in Table 5.
Fault width (mm) | Motor load (HP) | Shaft speed (rpm) | Inner ring | Outer ring |
0.007" | 1 | 1772 | IR007_1 | OR007@6_1 |
Bearing type | Inside diameter (DI/mm) | Outside diameter (DO/mm) | Ball diameter (d/mm) | Number of rolling elements (Z) | Angle of load from radial plane (a/rad) | Pitch diameter (D/mm) |
6205-2RS JEM SKF | 25 | 52 | 7.94 | 9 | 0 | 39.04 |
The rolling bearing failure data in Table 4 are used to re-validate the effectiveness of the noise reduction filtering of this paper's method. According to Eqs (5), (10) and (11), the theoretical fault frequency of the bearing, the search range of the improved Morlet wavelet width h and the search range of the center frequency f are calculated respectively, as shown in Table 6. The remaining marine predation algorithm related parameter settings are consistent with Section 4.1.1. According to the waveform matching optimization process of rolling bearing vibration signal based on improved Morlet wavelet, the noise reduction filtering operation is performed on the rolling bearing fault data in Table 4, and the filtering results are shown in Table 6 and Figure 7.
Fault type | Theoretical failure frequency (fz/Hz) | Waveform width (h/s) |
Center frequency (f/Hz) | [hOptimal, fOptimal] | SEBefore filtering | SEAfter filtering | S |
Inner ring | 159.91 | [8.3e-5, 1.25e-2] | [0, 6000] | [5.42e-3, 5875.72] | 2.63 | 1.65 | 37.26% |
Outer ring | 105.86 | [8.3e-5, 1.89e-2] | [0, 6000] | [3.83e-3, 2891.39] | 2.36 | 1.59 | 32.63% |
Observing Table 6, the inner ring signal Shannon entropy decreases from 2.63 to 1.65 with a Shannon entropy impairment value of 37.26% and the outer ring signal Shannon entropy decreases from 2.36 to 1.59 with a Shannon entropy impairment value of 32.63%. It shows that the signal noise is effectively reduced after filtering, which also reflects that the signal-to-noise ratio is enhanced, i.e., the invalid signal and noise signal are effectively filtered out, the distribution of the bearing fault signal becomes sparse and orderly and the fault characteristics are more obvious. Similarly, comparing the original signal and the signal after filtering in Figure 7, it can be clearly seen that the fault characteristics of the signal after filtering are prominent and the periodicity of the vibration impact signal is more obvious.
In order to verify that the after filtering signal is valid, the envelope analysis of the filtered signal is performed and the results are shown in Figures 8 and 9. The actual fault eigenfrequency fundamental frequencies of the inner and outer rings (159.6 Hz and 106.4 Hz respectively) are similar to the theoretical fault frequencies (159.91 Hz and 105.86 Hz respectively) and the fault eigenfrequency 2-fold frequencies (319.2 Hz and 212.4 Hz respectively) can also be clearly seen.
In summary, the results corroborate each other and jointly show that the method in this paper is effective for rolling bearing fault signal noise reduction filtering and also verifies the reliability of the proposed method in this paper.
For the problems of unclear physical significance of traditional Morlet wavelet waveform factors and complicated optimization process, this paper applied the improved Morlet wavelet to the noise reduction filtering of rolling bearing vibration signals and put forward a rolling bearing vibration signal noise reduction filtering method based on the improved Morlet wavelet. The improved Morlet wavelet utilizes the Gaussian waveform width to replace the traditional wavelet shape factor, and its physical meaning is clear, which is more conducive to determining the optimization range of the wavelet parameters. Optimization of the improved Morlet wavelet width and center frequency using the marine predation algorithm achieved the best match between the improved Morlet wavelet and rolling bearing fault signal waveforms. Then, completing the rolling bearing fault vibration signal in the invalid signal and noise signal effective filtering, highlighted the bearing fault impact characteristics, improved the signal-to-noise ratio and accurately obtained high-quality and reliable rolling bearing fault characteristics of the signal. In this paper, the proposed method was validated using two bearing datasets:
1) XJTU-SY rolling bearing accelerated life test dataset was used to experimentally verify the method of this paper. First, the outer ring early failure vibration data was taken as an example and the validity of the method of this paper was verified through the comparison of the bearing signal waveforms before and after filtering, as well as the analysis of the signal envelope after filtering. Second, experiments were carried out on the bearing vibration signal data of different fault types and different fault periods, and at the same time, the Shannon entropy impairment value was used as the evaluation index of the filtering noise reduction effect, and the results showed that the method in this paper has an effect on the rolling bearing fault vibration signal noise reduction filtering, which can effectively reduce the invalid signal and a large number of noise signals in the fault signal. It can effectively improve the signal-to-noise ratio of the signal. Among them, for the rolling bearing outer ring fault vibration signal, the filtering noise reduction effect is better than other types of faults, and at the same time, for various types of terminal failure vibration signals, the filtering noise reduction effect is better than that of early failure and mid-term failure.
2) The methodology proposed in this paper was again validated using the Case Western Reserve University bearing dataset of drive-end bearing inner and outer ring failure data. The results of the experiment showed that the signal noise is effectively reduced after filtering and the signal-to-noise ratio is enhanced. That is, invalid signals and noise signals are effectively filtered out, the distribution of bearing fault signals becomes sparse and orderly, the fault characteristics become more obvious and the periodicity of vibration impact signals becomes more obvious.
In conclusion, the method of this paper is effective, reliable and practical for noise reduction filtering of rolling bearing fault vibration signals.
The method of this paper can be used in the future to process vibration signals of mechanical equipment faults in rolling bearings and gears. It can be applied in the fields of bearing health monitoring, fault diagnosis, predictive maintenance, etc., providing strong support for improving equipment reliability and safety.
Research on this topic will continue in the future. Subsequent research will further introduce other types of signal-to-noise evaluation indexes, combined with Shannon entropy, to realize the optimization of improved Morlet wavelet parameters under the combination of multiple evaluation indexes of signal-to-noise ratio and provide the best filtering and noise reduction filtering technical means for rolling bearing signal preprocessing, feature extraction and accurate identification of fault states.
The authors declare they have not used Artificial Intelligence (AI) tools in the creation of this article.
The research for this paper was funded by the following program grants: project supported by the Key Project of Scientific Research of Anhui Provincial Education Department (No. 2022AH050995; No. 2022AH050975); supported by Anhui Province Intelligent Mine Technology and Equipment Engineering Laboratory Open Fund (No. AIMTEEL202201); Project of Science and Technology Bureau of Wuhu City (No. KZ32022014); Open Fund of Anhui Key Laboratory of Electric Transmission and Control (No. DQKJ202208); Research Project of Anhui Polytechnic University (No. Xjky2022007); Research Start-up Fund Project of Anhui Polytechnic University (No. 2022YQQ004); Applied Basic Research Project of Wuhu City (No. 2022jc20); and 2023 National Innovative Training Program for Undergraduates Project (No. 202310363011).
The authors declare there is no conflict of interest.
[1] |
M. Cerrada, R. V. Sanchez, C. Li, F. Pacheco, D. Cabrera, J. V. de Oliveira, et al., A review on data-driven fault severity assessment in rolling bearings, Mech. Syst. Signal Process., 99 (2018), 169–196. https://doi.org/10.1016/j.ymssp.2017.06.012 doi: 10.1016/j.ymssp.2017.06.012
![]() |
[2] |
M. Xia, T. Li, L. Xu, L. Liu, C. W. de Silva, Fault diagnosis for rotating machinery using multiple sensors and convolutional neural networks, IEEE/ASME Trans. Mechatron., 23 (2017), 101–110. https://doi.org/10.1109/TMECH.2017.2728371 doi: 10.1109/TMECH.2017.2728371
![]() |
[3] |
M. Liang, K. Zhou, Probabilistic bearing fault diagnosis using Gaussian process with tailored feature extraction, Int. J. Adv. Manuf. Technol., 119 (2022), 2059–2076. https://doi.org/10.1007/s00170-021-08392-6 doi: 10.1007/s00170-021-08392-6
![]() |
[4] |
W. Yang, R. Court, Experimental study on the optimum time for conducting bearing maintenance, Measurement, 46 (2013), 2781–2791. https://doi.org/10.1016/j.measurement.2013.04.016 doi: 10.1016/j.measurement.2013.04.016
![]() |
[5] |
C. Mongia, D. Goyal, S. Sehgal, Vibration response-based condition monitoring and fault diagnosis of rotary machinery, Mater. Today Proc., 50 (2022), 679–683. https://doi.org/10.1016/j.matpr.2021.04.395 doi: 10.1016/j.matpr.2021.04.395
![]() |
[6] |
W. Ahmad, S. A. Khan, J. M. Kim, A hybrid prognostics technique for rolling element bearings using adaptive predictive models, IEEE Trans. Ind. Electron., 65 (2017), 1577–1584. https://doi.org/10.1109/TIE.2017.2733487 doi: 10.1109/TIE.2017.2733487
![]() |
[7] |
M. A. Ugwiri, M. Carratú, V. Paciello, C. Liguori, Benefits of enhanced techniques combining negentropy, spectral correlation and kurtogram for bearing fault diagnosis, Measurement, 185 (2021), 110013. https://doi.org/10.1016/j.measurement.2021.110013 doi: 10.1016/j.measurement.2021.110013
![]() |
[8] |
S. Gawde, S. Patil, S. Kumar, P. Kamat, K. Kotecha, A. Abraham, Multi-fault diagnosis of Industrial Rotating Machines using Data-driven approach: a review of two decades of research, Eng. Appl. Artif. Intell., 123 (2023), 106139. https://doi.org/10.1016/j.engappai.2023.106139 doi: 10.1016/j.engappai.2023.106139
![]() |
[9] |
Y. Xu, Z. Li, S. Wang, W. Li, T. Sarkodie-Gyan, S. Feng, A hybrid deep-learning model for fault diagnosis of rolling bearings, Measurement, 169 (2021), 108502. https://doi.org/10.1016/j.measurement.2020.108502 doi: 10.1016/j.measurement.2020.108502
![]() |
[10] |
H. Shao, H. Jiang, Y. Lin, X. Li, A novel method for intelligent fault diagnosis of rolling bearings using ensemble deep auto-encoders, Mech. Syst. Signal Process., 102 (2018), 278–297. https://doi.org/10.1016/j.ymssp.2017.09.026 doi: 10.1016/j.ymssp.2017.09.026
![]() |
[11] |
L. Wen, X. Li, L. Gao, Y. Zhang, A new convolutional neural network-based data-driven fault diagnosis method, IEEE Trans. Ind. Electron., 65 (2018), 5990–5998. https://doi.org/10.1109/TIE.2017.2774777 doi: 10.1109/TIE.2017.2774777
![]() |
[12] |
M. Gan, C. Wang, C. Zhu, Construction of hierarchical diagnosis network based on deep learning and its application in the fault pattern recognition of rolling element bearings, Mech. Syst. Signal Process., 72–73 (2016), 92–104. https://doi.org/10.1016/j.ymssp.2015.11.014 doi: 10.1016/j.ymssp.2015.11.014
![]() |
[13] |
X. F. Xu, S. T. Hu, P. M. Shi, H. S. Shao, R. X. Li, Z. Li, Natural phase space reconstruction-based broad learning system for short-term wind speed prediction: case studies of an offshore wind farm, Energy, 262 (2023), 125342. https://doi.org/10.1016/j.energy.2022.125342 doi: 10.1016/j.energy.2022.125342
![]() |
[14] |
X. F. Xu, S. T. Hu, H. S. Shao, P. M. Shi, R. X. Li, D. G. Li, A spatio-temporal forecasting model using optimally weighted graph convolutional network and gated recurrent unit for wind speed of different sites distributed in an offshore wind farm, Energy, 284 (2023), 128565. https://doi.org/10.1016/j.energy.2023.128565 doi: 10.1016/j.energy.2023.128565
![]() |
[15] |
L. J. Zhang, J. W. Xu, J. H. Yang, D. B. Yang, D. D. Wang, Multiscale morphology analysis and its application of fault diagnosis, Mech. Syst. Signal Process., 22 (2008), 597–610. https://doi.org/10.1016/j.ymssp.2007.09.010 doi: 10.1016/j.ymssp.2007.09.010
![]() |
[16] |
Z. Li, S. Cai, X. Li, S. Shao, X. Y. Yang, Fault diagnosis of Rolling Bearing for Motor Based on LSTM-EEMD and Genetic Optimization, J. Phys.: Conf. Ser., 2549 (2023), 012025. https://doi.org/10.1088/1742-6596/2549/1/012025 doi: 10.1088/1742-6596/2549/1/012025
![]() |
[17] |
K. Zhou, J. Tang, A wavelet neural network informed by time-domain signal preprocessing for bearing remaining useful life prediction, Appl. Math. Modell., 122 (2023), 220–241. https://doi.org/10.1016/j.apm.2023.05.042 doi: 10.1016/j.apm.2023.05.042
![]() |
[18] |
Q. Miao, C. Tang, W. Liang, M. Pecht, Health assessment of cooling fan bearings using wavelet-based filtering, Sensors, 13 (2013), 274–291. https://doi.org/10.3390/s130100274 doi: 10.3390/s130100274
![]() |
[19] |
K. Belaid, A. Miloudi, H. Bournine, The processing of resonances excited by gear faults using continuous wavelet transform with adaptive complex Morlet wavelet and sparsity measurement, Measurement, 180 (2021), 109576. https://doi.org/10.1016/j.measurement.2021.109576 doi: 10.1016/j.measurement.2021.109576
![]() |
[20] |
P. Liang, W. Wang, X. Yuan, S. Liu, L. Zhang, Y. Cheng, Intelligent fault diagnosis of rolling bearing based on wavelet transform and improved ResNet under noisy labels and environment, Eng. Appl. Artif. Intell., 115 (2022), 105269. https://doi.org/10.1016/j.engappai.2022.105269 doi: 10.1016/j.engappai.2022.105269
![]() |
[21] |
J. Ma, H. Li, Y. Chen, J. Wang, Z. Zou, Application of VMD and dynamic wavelet noise reduction techniques in rolling bearing fault diagnosis, J. Phys.: Conf. Ser., 2528 (2023), 012048. https://doi.org/10.1088/1742-6596/2528/1/012048 doi: 10.1088/1742-6596/2528/1/012048
![]() |
[22] |
G. Naima, H. A. Elias, S. Salah, An improved fast kurtogram based on an optimal wavelet coefficient for wind turbine gear fault detection, J. Electr. Eng. Technol., 17 (2022), 1335–1346. https://doi.org/10.1007/s42835-021-00937-9 doi: 10.1007/s42835-021-00937-9
![]() |
[23] |
L. Liang, G. H. Xu, C. G. Hou, Continuous wavelet transform denoising method based on singular value decomposition, J. Xi'an Jiaotong Univ., 38 (2004), 904–908. https://doi.org/10.3321/j.issn:0253-987X.2004.09.006 doi: 10.3321/j.issn:0253-987X.2004.09.006
![]() |
[24] |
J. Lin, L. S. Qu, Feature extraction based on Morlet wavelet and its application form echanical fault diagnosis, J. Sound Vib., 234 (2000), 135–148. https://doi.org/10.1006/jsvi.2000.2864 doi: 10.1006/jsvi.2000.2864
![]() |
[25] |
W. Zhang, M. P. Jia, L. Zhu, An adaptive Morlet wavelet filter method and its application in detecting early fault feature of ball bearings (in Chinese), J. Southeast Univ. (Nat. Sci. Ed.), 46 (2016), 457–463. https://doi.org/10.3969/j.issn.1001-0505.2016.03.001 doi: 10.3969/j.issn.1001-0505.2016.03.001
![]() |
[26] |
P. W. Tse, D. Wang, The automatic selection of an optimal wavelet filter and its enhancement by the new Sparsogram for bearing fault detection: part 2 of the two related manuscripts that have a joint title as "two automatic vibration-based fault diagnostic methods using the novel sparsity measurement-Parts 1 and 2", Mech. Syst. Signal Process., 40 (2013), 520–544. https://doi.org/10.1016/j.ymssp.2013.05.018 doi: 10.1016/j.ymssp.2013.05.018
![]() |
[27] |
Y. Jiang, B. Tang, Y. Qin, W. Liu, Feature extraction method of wind turbine based on adaptive Morlet wavelet and SVD, Renewable Energy, 36 (2011), 2146–2153. https://doi.org/10.1016/j.renene.2011.01.009 doi: 10.1016/j.renene.2011.01.009
![]() |
[28] |
M. Behzad, A. Kiakojouri, H. A. Arghand, A. Davoodabadi, Inaccessible rolling bearing diagnosis using a novel criterion for Morlet wavelet optimization, J. Vib. Control, 28 (2022), 1239–1250. https://doi.org/10.1177/1077546321989503 doi: 10.1177/1077546321989503
![]() |
[29] |
X. Gu, S. Yang, Y. Liu, F. Deng, B. Ren, Compound faults detection of the rolling element bearing based on the optimal complex Morlet wavelet filter, Proc. Inst. Mech. Eng., Part C: J. Mech. Eng. Sci., 232 (2018), 1786–1801. https://doi.org/10.1177/0954406217710673 doi: 10.1177/0954406217710673
![]() |
[30] |
Y. Zhang, B. P. Tang, Z. R. Liu, R. X. Chen, An adaptive demodulation approach for bearing fault detection based on adaptive wavelet filtering and spectral subtraction, Meas. Sci. Technol., 27 (2015), 025001. https://doi.org/10.1088/0957-0233/27/2/025001 doi: 10.1088/0957-0233/27/2/025001
![]() |
[31] |
W. Su, F. Wang, H. Zhu, Z. Zhang, Z. Guo, Rolling element bearing faults diagnosis based on optimal Morlet wavelet filter and autocorrelation enhancement, Mech. Syst. Signal Process., 24 (2010), 1458–1472. https://doi.org/10.1016/j.ymssp.2009.11.011 doi: 10.1016/j.ymssp.2009.11.011
![]() |
[32] |
X. Han, J. Xu, S. Song, J. Zhou, Crack fault diagnosis of vibration exciter rolling bearing based on genetic algorithm–optimized Morlet wavelet filter and empirical mode decomposition, Int. J. Distrib. Sens. Netw., 18 (2022). https://doi.org/10.1177/15501329221114566 doi: 10.1177/15501329221114566
![]() |
[33] |
M. X. Cohen, A better way to define and describe Morlet wavelets for time-frequency analysis, Neuroimage, 199 (2019), 81–86. https://doi.org/10.1016/j.neuroimage.2019.05.048 doi: 10.1016/j.neuroimage.2019.05.048
![]() |
[34] |
A. Dey, S. Bhattacharyya, S. Dey, D. Konar, J. Platos, V. Snasel, et al., A review of quantum-inspired metaheuristic algorithms for automatic clustering, Mathematics, 11 (2023), 2018. https://doi.org/10.3390/math11092018 doi: 10.3390/math11092018
![]() |
[35] |
A. Faramarzi, M. Heidarinejad, S. Mirjalili, A. H. Gandomi, Marine Predators Algorithm: a nature-inspired metaheuristic, Expert Syst. Appl., 152 (2020), 113377. https://doi.org/10.1016/j.eswa.2020.113377 doi: 10.1016/j.eswa.2020.113377
![]() |
[36] | S. Devendiran, K. Manivannan, Vibration based condition monitoring and fault diagnosis technologies for bearing and gear components a review, Int. J. Appl. Eng. Res., 11 (2016), 3966–3975. |
[37] |
N. G. Nikolaou, I. A. Antoniadis, Demodulation of vibration signals generated by defects in rolling element bearings using complex shifted Morlet wavelets, Mech. Syst. Signal Process., 16 (2002), 677–694. https://doi.org/10.1006/mssp.2001.1459 doi: 10.1006/mssp.2001.1459
![]() |
[38] |
P. K. Kankar, S. C. Sharma, S. P. Harsha, Rolling element bearing fault diagnosis using wavelet transform, Neurocomputing, 74 (2011), 1638–1645. https://doi.org/10.1016/j.neucom.2011.01.021 doi: 10.1016/j.neucom.2011.01.021
![]() |
[39] |
R. Dubey, V. Rajpoot, A. Chaturvedi, A. Dixit, S. Maheshwari, Ball-bearing fault classification using comparative analysis of wavelet coefficient based on entropy measurement, IETE J. Res., 25 (2022). https://doi.org/10.1080/03772063.2022.2142685 doi: 10.1080/03772063.2022.2142685
![]() |
[40] |
S. Dong, X. Xu, R. Chen, Application of fuzzy C-means method and classification model of optimized K-nearest neighbor for fault diagnosis of bearing, J. Braz. Soc. Mech. Sci. Eng., 38 (2016), 2255–2263. https://doi.org/10.1007/s40430-015-0455-9 doi: 10.1007/s40430-015-0455-9
![]() |
[41] |
B. Wang, Y. Lei, N. Li, N. Li, A hybrid prognostics approach for estimating remaining useful life of rolling element bearings, IEEE Trans. Reliab., 69 (2018), 401–412. https://doi.org/10.1109/TR.2018.2882682 doi: 10.1109/TR.2018.2882682
![]() |
[42] |
T. H. Loutas, D. Roulias, G. Georgoulas, Remaining useful life estimation in rolling bearings utilizing data-driven probabilistic e-support vectors regression, IEEE Trans. Reliab., 62 (2013), 821–832. https://doi.org/10.1109/TR.2013.2285318 doi: 10.1109/TR.2013.2285318
![]() |
[43] |
X. F. Xu, B. Li, Z. J. Qiao, P. M. Shi, H. S. Shao, R. X. Li, Caputo-Fabrizio fractional order derivative stochastic resonance enhanced by ADOF and its application in fault diagnosis of wind turbine drivetrain, Renewable Energy, 219 (2023), 119398. https://doi.org/10.1016/j.renene.2023.119398 doi: 10.1016/j.renene.2023.119398
![]() |
[44] |
W. A. Smith, R. B. Randall, Rolling element bearing diagnostics using the Case Western Reserve University data: a benchmark study, Mech. Syst. Signal Process., 64–65 (2015), 100–131. https://doi.org/10.1016/j.ymssp.2015.04.021 doi: 10.1016/j.ymssp.2015.04.021
![]() |
Bearing type | Inside diameter (DI/mm) | Outside diameter (DO/mm) | Ball diameter (d/mm) | Number of rolling elements (Z) | Angle of load from radial plane (a/rad) |
Pitch diameter (D/mm) |
LDK UER204 Ball bearing |
29.3 | 39.8 | 7.92 | 8 | 0 | 34.55 |
Experimental condition | Data set | Sample size | Failure location | Selected csv files | Theoretical failure frequency (fz/Hz) |
Rotation speed: 37.5 Hz Radial force: 11 KN |
Bearing2_1 | 491 | Inner ring | EF: 418.csv; MF: 451.csv; TF: 476.csv; |
184.39 |
Bearing2_2 | 161 | Outer ring | EF: 136.csv; MF: 148.csv; TF: 156.csv; |
115.61 | |
Bearing2_3 | 533 | Cage | EF: 453.csv; MF: 489.csv; TF: 517.csv; |
14.45 |
Data set and Failure location | Selected csv files | [hOptimal, fOptimal] | SEBefore filtering | SEAfter filtering | S |
Bearing2_1 inner ring |
EF: 418.csv; | [3.8464e-3, 1419.5204] | 3.0839 | 2.9927 | 2.96% |
MF: 451.csv; | [2.9960e-3, 2059.1770] | 2.9559 | 2.7446 | 7.15% | |
TF: 476.csv; | [6.1656e-4, 4058.7670] | 2.8998 | 2.3868 | 17.69% | |
Bearing2_2 outer ring | EF: 136.csv; | [1.7853e-3, 7621.4035] | 2.7099 | 2.0108 | 25.80% |
MF: 148.csv; | [7.3316e-4, 9719.7542] | 2.7018 | 2.0500 | 24.12% | |
TF: 156.csv; | [2.1630e-3, 12210.3952] | 3.1519 | 2.1851 | 30.67% | |
Bearing2_3 cage |
EF: 453.csv; | [2.3897e-3, 12174.4453] | 2.6797 | 2.4388 | 8.99% |
MF: 489.csv; | [1.1186e-3, 9726.5546] | 2.7697 | 2.4038 | 13.21% | |
TF: 517.csv; | [7.5635e-4, 3430.0532] | 2.8322 | 2.1330 | 24.69% |
Fault width (mm) | Motor load (HP) | Shaft speed (rpm) | Inner ring | Outer ring |
0.007" | 1 | 1772 | IR007_1 | OR007@6_1 |
Bearing type | Inside diameter (DI/mm) | Outside diameter (DO/mm) | Ball diameter (d/mm) | Number of rolling elements (Z) | Angle of load from radial plane (a/rad) | Pitch diameter (D/mm) |
6205-2RS JEM SKF | 25 | 52 | 7.94 | 9 | 0 | 39.04 |
Fault type | Theoretical failure frequency (fz/Hz) | Waveform width (h/s) |
Center frequency (f/Hz) | [hOptimal, fOptimal] | SEBefore filtering | SEAfter filtering | S |
Inner ring | 159.91 | [8.3e-5, 1.25e-2] | [0, 6000] | [5.42e-3, 5875.72] | 2.63 | 1.65 | 37.26% |
Outer ring | 105.86 | [8.3e-5, 1.89e-2] | [0, 6000] | [3.83e-3, 2891.39] | 2.36 | 1.59 | 32.63% |
Bearing type | Inside diameter (DI/mm) | Outside diameter (DO/mm) | Ball diameter (d/mm) | Number of rolling elements (Z) | Angle of load from radial plane (a/rad) |
Pitch diameter (D/mm) |
LDK UER204 Ball bearing |
29.3 | 39.8 | 7.92 | 8 | 0 | 34.55 |
Experimental condition | Data set | Sample size | Failure location | Selected csv files | Theoretical failure frequency (fz/Hz) |
Rotation speed: 37.5 Hz Radial force: 11 KN |
Bearing2_1 | 491 | Inner ring | EF: 418.csv; MF: 451.csv; TF: 476.csv; |
184.39 |
Bearing2_2 | 161 | Outer ring | EF: 136.csv; MF: 148.csv; TF: 156.csv; |
115.61 | |
Bearing2_3 | 533 | Cage | EF: 453.csv; MF: 489.csv; TF: 517.csv; |
14.45 |
Data set and Failure location | Selected csv files | [hOptimal, fOptimal] | SEBefore filtering | SEAfter filtering | S |
Bearing2_1 inner ring |
EF: 418.csv; | [3.8464e-3, 1419.5204] | 3.0839 | 2.9927 | 2.96% |
MF: 451.csv; | [2.9960e-3, 2059.1770] | 2.9559 | 2.7446 | 7.15% | |
TF: 476.csv; | [6.1656e-4, 4058.7670] | 2.8998 | 2.3868 | 17.69% | |
Bearing2_2 outer ring | EF: 136.csv; | [1.7853e-3, 7621.4035] | 2.7099 | 2.0108 | 25.80% |
MF: 148.csv; | [7.3316e-4, 9719.7542] | 2.7018 | 2.0500 | 24.12% | |
TF: 156.csv; | [2.1630e-3, 12210.3952] | 3.1519 | 2.1851 | 30.67% | |
Bearing2_3 cage |
EF: 453.csv; | [2.3897e-3, 12174.4453] | 2.6797 | 2.4388 | 8.99% |
MF: 489.csv; | [1.1186e-3, 9726.5546] | 2.7697 | 2.4038 | 13.21% | |
TF: 517.csv; | [7.5635e-4, 3430.0532] | 2.8322 | 2.1330 | 24.69% |
Fault width (mm) | Motor load (HP) | Shaft speed (rpm) | Inner ring | Outer ring |
0.007" | 1 | 1772 | IR007_1 | OR007@6_1 |
Bearing type | Inside diameter (DI/mm) | Outside diameter (DO/mm) | Ball diameter (d/mm) | Number of rolling elements (Z) | Angle of load from radial plane (a/rad) | Pitch diameter (D/mm) |
6205-2RS JEM SKF | 25 | 52 | 7.94 | 9 | 0 | 39.04 |
Fault type | Theoretical failure frequency (fz/Hz) | Waveform width (h/s) |
Center frequency (f/Hz) | [hOptimal, fOptimal] | SEBefore filtering | SEAfter filtering | S |
Inner ring | 159.91 | [8.3e-5, 1.25e-2] | [0, 6000] | [5.42e-3, 5875.72] | 2.63 | 1.65 | 37.26% |
Outer ring | 105.86 | [8.3e-5, 1.89e-2] | [0, 6000] | [3.83e-3, 2891.39] | 2.36 | 1.59 | 32.63% |