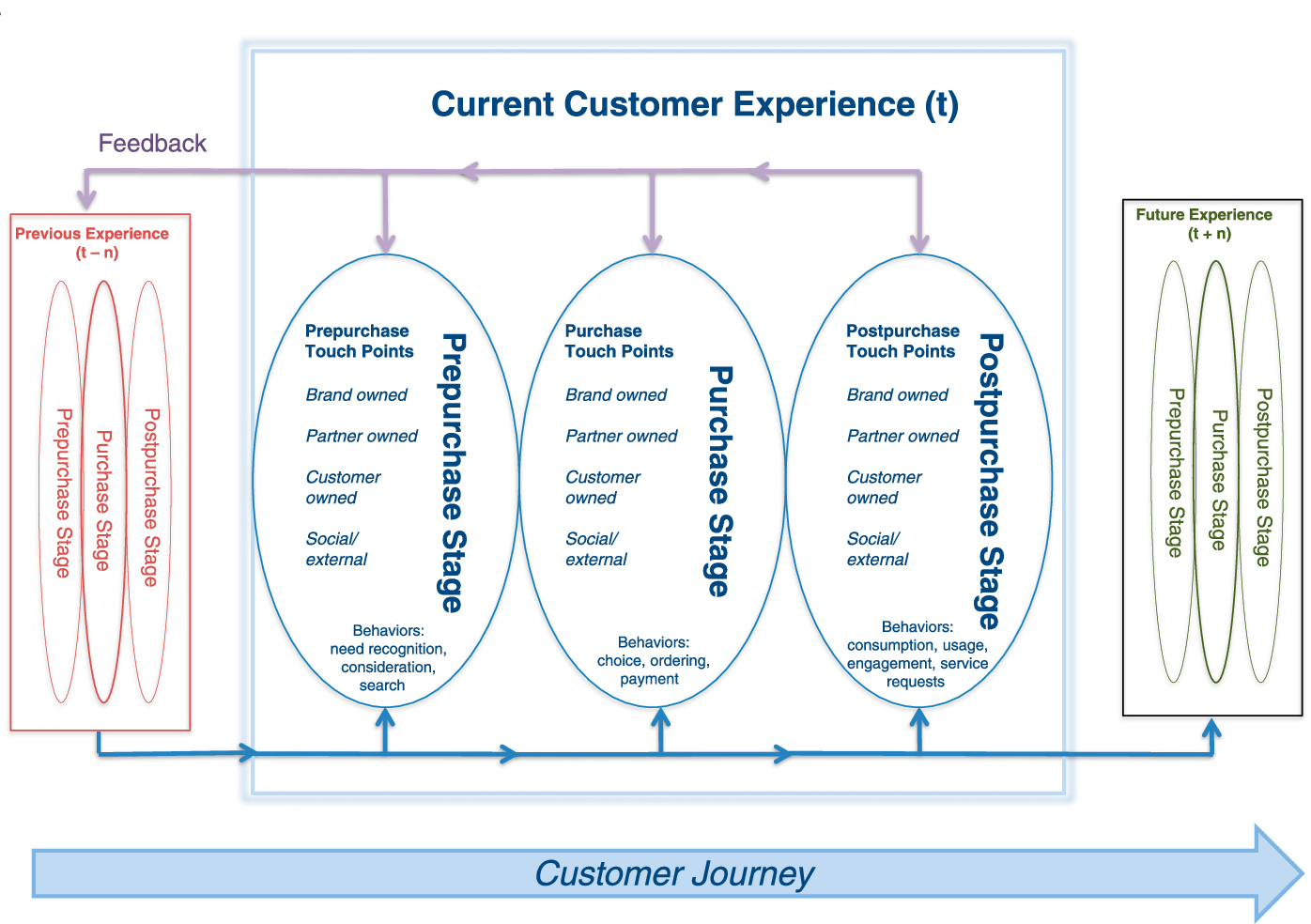
ResNet-based correlation models excel in age recognition algorithms, but specific age recognition research is currently limited and often plagued by substantial errors. We introduce an enhanced portrait age recognition algorithm based on ResNet, using CORAL (consistent rank logits) rank consistent ordered regression instead of traditional classification to predict precise ages. We further improve this approach by incorporating DCN (deformable convolution), resulting in the DCN-R model. DCN dynamically adjusts convolution kernels for diverse faces, improving accuracy and robustness. We tested DCN-R34 and DCN-R50 against the SOTA model, achieving better results with the same complexity. This reduces the computational load while maintaining or enhancing performance.
Citation: Ji Xi, Zhe Xu, Zihan Yan, Wenjie Liu, Yanting Liu. Portrait age recognition method based on improved ResNet and deformable convolution[J]. Electronic Research Archive, 2023, 31(11): 6585-6599. doi: 10.3934/era.2023333
[1] | Hui Jian, Min Gong, Meixia Cai . Global existence, blow-up and mass concentration for the inhomogeneous nonlinear Schrödinger equation with inverse-square potential. Electronic Research Archive, 2023, 31(12): 7427-7451. doi: 10.3934/era.2023375 |
[2] | Zhiyan Ding, Hichem Hajaiej . On a fractional Schrödinger equation in the presence of harmonic potential. Electronic Research Archive, 2021, 29(5): 3449-3469. doi: 10.3934/era.2021047 |
[3] | Guoliang Zhang, Shaoqin Zheng, Tao Xiong . A conservative semi-Lagrangian finite difference WENO scheme based on exponential integrator for one-dimensional scalar nonlinear hyperbolic equations. Electronic Research Archive, 2021, 29(1): 1819-1839. doi: 10.3934/era.2020093 |
[4] | Yunxia Niu, Chaoran Qi, Yao Zhang, Wahidullah Niazi . Numerical analysis and simulation of the compact difference scheme for the pseudo-parabolic Burgers' equation. Electronic Research Archive, 2025, 33(3): 1763-1791. doi: 10.3934/era.2025080 |
[5] | Chunye Gong, Mianfu She, Wanqiu Yuan, Dan Zhao . SAV Galerkin-Legendre spectral method for the nonlinear Schrödinger-Possion equations. Electronic Research Archive, 2022, 30(3): 943-960. doi: 10.3934/era.2022049 |
[6] | Huanhuan Li, Lei Kang, Meng Li, Xianbing Luo, Shuwen Xiang . Hamiltonian conserved Crank-Nicolson schemes for a semi-linear wave equation based on the exponential scalar auxiliary variables approach. Electronic Research Archive, 2024, 32(7): 4433-4453. doi: 10.3934/era.2024200 |
[7] | Hongquan Wang, Yancai Liu, Xiujun Cheng . An energy-preserving exponential scheme with scalar auxiliary variable approach for the nonlinear Dirac equations. Electronic Research Archive, 2025, 33(1): 263-276. doi: 10.3934/era.2025014 |
[8] | Xiaoguang Li . Normalized ground states for a doubly nonlinear Schrödinger equation on periodic metric graphs. Electronic Research Archive, 2024, 32(7): 4199-4217. doi: 10.3934/era.2024189 |
[9] | Xuefei He, Kun Wang, Liwei Xu . Efficient finite difference methods for the nonlinear Helmholtz equation in Kerr medium. Electronic Research Archive, 2020, 28(4): 1503-1528. doi: 10.3934/era.2020079 |
[10] | Riadh Chteoui, Abdulrahman F. Aljohani, Anouar Ben Mabrouk . Classification and simulation of chaotic behaviour of the solutions of a mixed nonlinear Schrödinger system. Electronic Research Archive, 2021, 29(4): 2561-2597. doi: 10.3934/era.2021002 |
ResNet-based correlation models excel in age recognition algorithms, but specific age recognition research is currently limited and often plagued by substantial errors. We introduce an enhanced portrait age recognition algorithm based on ResNet, using CORAL (consistent rank logits) rank consistent ordered regression instead of traditional classification to predict precise ages. We further improve this approach by incorporating DCN (deformable convolution), resulting in the DCN-R model. DCN dynamically adjusts convolution kernels for diverse faces, improving accuracy and robustness. We tested DCN-R34 and DCN-R50 against the SOTA model, achieving better results with the same complexity. This reduces the computational load while maintaining or enhancing performance.
In the financial sector, banks leverage branch mechanisms and utilize functional systems to open multiple channels. With the full support of various business departments, such as financial services, trust departments, wealth management departments, etc., banks facilitate the rapid development of related business groups. However, in recent years, the shift to e-commerce has transformed customer channels, replacing traditional methods like TV advertising with online channels such as paid media, social media, and proprietary platforms. This shift has made it challenging for financial holding companies to promptly grasp online touchpoints with customers, effectively enhance member services and customer experiences, and optimize resource allocation to manage online channels efficiently. Therefore, in the digital age, the financial industry's goal is to transition to artificial intelligence for online customer journey management (CJM) by understanding customer touchpoints. This transition has become a recent corporate objective for global financial holding companies, aiming to enhance experiences and improve conversion rates.
CJM empowers companies to understand customer online behaviors, streamline experiences, and optimize resource allocation across channels, aligning with both enterprise objectives and customer benefits (Grewala et al., 2020). To further advance this approach, our study integrates user behavior prediction and operational management strategies. Predicting user conversion values across channels enables channel operation managers to strategically allocate resources for improved operational outcomes. This fusion of deep learning prediction models with operational management reflects an emerging trend in modern management science, garnering attention from academia and industry alike (Kraus et al., 2020).
The literature review conducted by Lemon and Verhoef (2016) indicates a significant positive correlation between effectively analyzing customer journeys (purchase processes) and customer experience, conversion rates, loyalty, and overall business performance, especially in the e-commerce environment. Therefore, artificial intelligence plays a crucial role in customer journey management. In the application-oriented research of customer journey management, there are two categories of research topics. One focuses on evaluating customer purchase conversion or customer value, exploring how to assess the conversion value of customers or users through quantitative methods for effective customer relationship management. The other involves contact channel planning, using multivariate methods to maximize the performance of channels or customer touchpoints, increase customer satisfaction, plan experience patterns that meet specific customer needs, and effectively manage customer experiences.
Based on the research conclusions from these studies, it is apparent that for effective customer journey management, businesses should first understand the conversion value of customers. Subsequently, based on the different nature of channels, they should plan suitable contact channels to achieve optimal results in customer journeys. In other words, it is essential to accurately estimate the conversion performance of different-value customers in various channels before recommending different channels to the appropriate customers.
Our study presents a three-step analytical framework that leverages artificial intelligence and deep learning, building upon the insights of Lemon and Verhoef (2016) regarding multi-channel analysis within customer journeys. These steps aim to unveil user behavior patterns, formulate data-driven customer journey management strategies, and optimize financial resource allocation across diverse online channels for maximum benefits.
In summary, our study introduces an analytical framework that integrates customer journey management and revenue optimization. Rooted in the insights of Lemon and Verhoe (2016), we harness artificial intelligence and deep learning to enhance our understanding of customer behavior, formulate data-driven customer journey management strategies, and optimize resource allocation across various online channels. These findings provide valuable guidance to companies striving to allocate resources effectively and enhance customer experiences.
Compared to previous Customer Relationship Management (CRM) research, this study proposes a novel data methodology based on customer analysis and touchpoints in online channels, contributing to data-driven e-commerce models. By focusing on analyzing customer online channel data and online behavioral data within e-commerce systems, we introduce an analytical process called "integrated integer programming and deep learning methods". This method addresses real-world scenarios in digital marketing. Unlike traditional CRM analysis and multi-channel analysis, we incorporate an empirical model based on channel sequence prediction and optimization planning. We apply this model to solve common channel planning problems, including customer purchase predictions, channel selection recommendations, and guidelines for the digital marketing department. We systematically evaluate customer conversion value and optimize channel planning. These insights provide valuable practical references for data methodologies in research on data-driven business models in e-commerce.
Customer experience is a critical factor in a company's competitiveness (Edvardsson et al., 2005). With the rise of e-commerce, dynamic customer portraits have gained prominence, allowing real-time tracking of customer data during website browsing. Dynamic customer portraits enhance segmentation, targeting, and positioning (STP) strategies by enabling precise market segmentation based on customer behavior (Grewala et al., 2020).
Traditionally, customer satisfaction was assessed through post-experience questionnaires, limited to a single point in time. However, the measurement of customer online experiences has evolved to encompass the entire journey, CJM (Lemon and Verhoef, 2016). CJM is crucial for retailers and service providers to effectively understand and manage online customer experiences. This journey spans before, during, and after purchase, with multiple contact points at each stage (Lemon and Verhoef, 2016).
Companies must analyze these contact points across the customer journey, from initial bidding and search to post-purchase interactions, to comprehend customer preferences during different phases. The organizational structures of the future should incorporate a "journey product manager" responsible for identifying key channels, dynamically allocating resources, and generating profits within high-value customer journeys (Edelman et al., 2015). Lemon and Verhoef (2016) highlight three aspects of multi-channel analysis in customer journeys: (1) Customers exhibit varying channel preferences across purchase phases, allowing the identification of distinct multichannel segments based on consumer characteristics; (2) Channel choices in the purchase funnel are influenced by lock-in effects, channel inertia, and cross-channel synergies; (3) Channels offer different benefits and costs, making specific channels more suitable for particular purchase funnel stages. However, technological advancements are reducing these differences, as exemplified in Lemon and Verhoef's (2016) operational framework for customer journeys.
Deep learning and artificial intelligence have introduced innovative methods to address these challenges (Klein et al., 2020; Strauss et al., 2018; Han et al., 2010; Chang, 2002; Dodin and Huang Chan, 1991). Artificial intelligence and deep learning methods provide solutions to dynamic sequence problems beyond traditional neural networks' capabilities. These techniques use iterative algorithms to find optimal solutions under resource and time constraints, offering a management strategy for optimizing users' online journeys and profits.
Our study introduces an optimal revenue analysis model addressing dynamic channel programming issues based on user behaviors in online channels. It optimizes resource allocation to maximize revenue while minimizing operating costs. This study integrates clustering, convolutional neural network (CNN), long short-term memory (LSTM), and channel planning into a unified model. Drawing inspiration from Lemon and Verhoef's (2016) concept of the customer journey, we develop models for user characteristic behavior analysis, user journey behavior prediction, and online channel planning. Combining CNN, used for image data processing, with LSTM, suitable for text sequence data, allows us to analyze user sequences on online channels. The CNN-LSTM model predicts user behaviors during their online journey, providing insights into how this journey influences subsequent conversions on the online platform. We further employ optimization analysis to create a model for efficient resource and budget allocation across different channels.
Both purchase conversion assessment and multichannel analysis are important topics in CRM research. The former focuses on empirical verification of customer consumption behavior through customer assessment methods. The latter involves channel analysis to meet the resource allocation and channel configuration needs of businesses. This study proposes an integrated optimization model primarily based on recommendation systems, aiming to construct a management system that satisfies multiple decision goals, including purchase conversion assessment and multichannel analysis models.
A comparison of the methods listed in Table 1 reveals several advantages of the integrated optimization model with a recommendation system, summarized as follows: (1) The integrated optimization model does not require many assumptions, such as avoiding autocorrelation in residual terms, avoiding multicollinearity issues among independent variables, and assuming data follows a normal distribution, and it does not need complex parameter estimation. It can handle both linear and nonlinear problems widely, demonstrating superior solving capabilities in constructing mathematical models for solving nonlinear problems. In evaluating methods without hard computational formulas, it relies on its rigorous mathematical framework to provide accurate results; (2) This study combines integer programming, enabling the model to obtain the optimal solution for multichannel planning within a limited computational time, successfully executing high-dimensional and complex constraints.
Type | Research Topic | Methodology | Advantages | Disadvantages | Purpose |
Customer | 1. Purchase Conversion Assessment 2. Consumer Behavior Analysis |
- RFM Model - NES Model |
Analyzing customer databases to establish models through multivariate relationships, understanding customer management approaches. | The constructed models are reusable and effectively integrate predictive variables with faster execution speed. Analysis results may be suboptimal if they violate normality or variable assumptions. The model is challenging to analyze dynamic customer data. | The main objective is to explore the correlation between customer data and order transactions, enabling marketing efforts to be more precise and aligned with customer patterns to achieve business goals. |
Channel | 1. Multichannel Analysis 2. Customer Journey Analysis |
- Channel Path Model - Advertisement Traffic Analysis Model - Multichannel Attribution Model |
Optimizes resource allocation for small-scale and distribution problems, providing models close to real scenarios with easily understandable analysis rules. | Lacks the ability to process large amounts of data, fault tolerance, associative learning, and has poor predictive capabilities. The model complexity may result in a large data space and extended processing time, limiting practical applications. | The primary goal is to use mathematical planning methods to evaluate channel or advertisement effectiveness, extracting high-performing channel allocation rules to develop advertisement budget allocation models. |
Recomme-ndation -System | 1. User Recommendations 2. Content Recommendations |
Machine Learning/Deep Learning | Predictive model with rigorous mathematical inference, massive parallel processing capabilities, fault tolerance, high associative capabilities, and noise filtering capabilities without requiring many assumptions. | Poor interpretability due to its black-box nature, and the integration of complex models may lead to long processing times during training. | The main focus is on finding the most accurate model, adjusting optimal parameters for continuous improvement of predictive patterns. |
Integration Model | This study proposes an optimized integration model, suitable for solving high-dimensional and complex constraint problems. It demonstrates practical feasibility for large-scale issues and dynamic problem-solving. | Appropriate model selection is crucial, and the model may face challenges in convergence. The solution obtained may be a local optimum or feasible solution, with no guarantee of being a global optimal solution. | The objective is to find an approximate solution within a limited computational time, serving as a viable decision-making approach. |
The customer journey perspective explains the entire process through which customers move from initial awareness of a brand, product, or service to the final purchase and interaction with the brand. In the field of data analysis, numerous studies have provided frameworks based on the stages of customer experience to assist businesses in understanding different phases of the customer journey and formulating corresponding marketing strategies. The classic purchase funnel theory describes the various stages consumers go through during the purchasing process, including awareness, interest, desire, action, and purchase. Simultaneously, the AIDA model emphasizes the psychological processes consumers undergo during the purchase journey, focusing on attention, interest, desire, and action. Following this, touchpoint management concentrates on the various interaction points between customers and the brand, such as websites, social media, and customer service. Optimizing these touchpoints enables businesses to enhance the overall customer experience. Furthermore, there is an emphasis on providing value throughout the entire customer journey, not just during the purchase phase. Understanding customer value and meeting their needs contributes to increased customer loyalty. Considering that modern consumers use multiple channels for information search and shopping, multichannel marketing emphasizes delivering a consistent brand experience across different platforms.
Drawing on the foundational research and the perspective of customer journey management, this study suggests that a customer journey management system equipped with channel planning and customer value analysis can effectively enable businesses to manage customer experiences. Advanced data analysis technologies incorporated into the system enhance big data analytics capabilities and operational efficiency. Therefore, this study proposes the research framework depicted in Figure 2.
In this study, the process of constructing the model was integrated into a research framework, as depicted in Figure 2. It consisted of three sequential steps. The first step focused on behavior clustering, where machine learning techniques (specifically the K-means model) were employed to analyze user behavior characteristics based on their past browsing behavior. This led to the segmentation of users into distinct groups. Subsequently, in the second step, multi-classification of sequential category data was addressed. In addition to user characteristics, this step considered users' channel paths. The deep learning model CNN-LSTM was utilized to process the sequential data of users' online channel interactions. This enabled the prediction of their future behaviors based on their journey and behavioral data, essentially addressing a multi-classification prediction problem. CNN-LSTM provided an effective framework for analyzing sequential category data and predicting user behavior. The third step involved the establishment of a mathematical model for profit calculation and the formulation of target formulas. This step was informed by interviews with senior company executives and a review of literature on customer journey and revenue optimization. The result was a constrained mathematical model for online channel planning, facilitating the analysis of optimal revenue allocation. The output from this model served as a reference for enterprise resource allocation decisions. The following sections provide a detailed analysis of the steps depicted in Figure 2.
The construction reasons for the three-step analytical framework in this case study are briefly described as follows:
Customer segmentation model: Implementation of this step involves cluster analysis of the existing customer database. Customer cluster analysis helps discover common patterns in user journeys. This information can be utilized to optimize user experiences, ensuring that users receive optimal support and guidance during interactions with products or services. This step is crucial for formulating more effective channel business strategies. By analyzing customer online channel behavior and generating key customer insights through the subsequent clustering process, this step ensures rapid acquisition of valuable customer insights. As a result, the challenging task of retrieving multi-channel user data is significantly simplified.
Evaluation of customer conversion value: When product managers engage with key customers, the system rapidly assesses the user's conversion value in real-time, including webpage clicks, purchase clicks, actual purchases, etc. By evaluating a series of target conversions, this step enables product managers to identify conversion values and allocate relevant resources. The availability of deep learning models accelerates the complexity of digital marketing, greatly contributing to detailed marketing planning.
Multi-Channel planning model: An innovative aspect of this step involves establishing a solid foundation in customer touchpoints and recommending frameworks using integer programming models. The optimization planning mechanism further strengthens this aspect. This step automatically elucidates the inherent relationship between customer conversion and channel planning. It assists product managers in accelerating various aspects of customer development, including customer value assessment, copywriting, and evaluating channel selection.
Step 1: Collect online data of users, including user ID, online channel data, data of users visiting web page, users' devices, and their transaction data. The collected data were then processed in two parts: (1) merging data and (2) data conversion. In terms of data merging, the previously collected user online data and transaction data were sorted and merged for subsequent model analysis. For data conversion, firstly, channel data was identified, separating original and yet different sources of website information into "channel", such as advertisement on Google, native advertisement, different website sources, cross-platform marketing, etc., in which these were calculated and converted into binary and sequential format.
Step 2: Each user (i) would undergo cluster analysis with a corresponding behavioral variable (Al) using the machine learning K-means model for user behavioral characteristics. By dividing users into multiple groups (Zik), the residual sum of squares was minimized and the most suitable number of suitable target groups (k) could be found, in order to understand the overalls of users, their online behaviors, product preference and the current value for the subsequent analysis of user's characteristic behavior, such that the customer portraits at different stages of purchase process could be painted, providing as the pre-screening process of the user's journey prediction model.
Step 3: This step was divided into two parts. The first part was to process the sequential data of the online channel of users through CNN-LSTM model of deep learning, so as to obtain the subsequent behaviors of users during their journey, such as predicting if they will click a button (AC3), click a purchase button (AC2), or actually purchase a product (AC1). The results were then compared with other machine learning classification methods, such as the LSTM model, random forest (RF) model, and artificial neural network (ANN) model. The second part involved the prediction of user's target conversion value in a channel by decision tree approach, such as transaction revenue (Rij), conversion value of clicking the purchase button (CLij), and conversion value of clicking a button (Sij). Through decision tree classification rules, companies were able to identify attribute properties and input the predicted value into the subsequent optimization model.
Step 4: Based on the analysis results, literature review and in-depth interviews, a mathematical model for online channel planning was constructed, with the integer programming method and heuristic binary algorithm, to conduct channel planning analysis of optimal returns, in which the mathematical model (Figure 4) was then converted into an adaptive function for the heuristic algorithm to solve the problem of iterative optimization.
Step 5: The analysis results of the three models were interpreted and compared to verify the four suggested propositions, as a recommended management implication.
The appendices (Appendix A and Appendix B) provide a detailed analysis flow chart for the first four steps.
Based on online behavior prediction, this study would provide as a reference for companies to make decision on resource allocation problems, which could be described and defined as follows: users would enter the company websites through different channels to take a series of actions, and according to Lemon & Verhoef (2016), who stated, "during the purchase cycle, we will regard customer experience as the "journey" of companies and customers across multiple contact points". Lemon & Verhoef (2016) conceptualized the customer journey as a dynamic process and also iterative, continuing from before purchase (including search phase) to after purchase (as shown in Figure 1). Therefore, we defined user as i, and there were n number of users. User of i would enter on the channel at the t(th) click, yielding a total of t clicks, where the user showed three types of actions AC1、AC2、AC3, respectively indicated purchasing, clicking the purchase button, and clicking the button. These mark the user's dynamic journey. The data recorded the user's i clicks on the channel and the behavior for entering the channel. And each record represented the sequential data of clicking the channel, as each click was the point of contact during the customer's journey online. If the time interval between each click of the user exceeded a certain standard, such actions would be regarded as separate behavior. For example, if you click on the same day but once in the morning and once in the evening, they would be defined as two clicks. In addition, due to the multiple sources of the original data, to improve the model efficiency, the sources were classified into m types of channels. Therefore, each user would have a set of click data, and a r-number of records in this data could be used to predict the user's r+1(th) action; AC1, AC2, AC3, As well as predicting the user's i value of conversion at the r+1(th) action at the j(th) channel. Once user j finalized a transaction, the converted value was output with the transaction amount r+1; and if the user clicked on the purchase button, the converted value was output as CLij. If the user i simply clicked, the converted value was output as Sij, where the target value of entering the website through certain channel from conversion of clicking the purchase button and click a button was defined by the company with mathematical symbols or variables, as summarized in Table 2.
Symbol | Definition |
i | The 1st, 2nd, …………n(th) user |
j | The 1st, 2nd, …………m(th) channel |
t | The 1st, 2nd …………r(th) click |
k | The 1st, 2nd …………k(th) cluster |
AC1 | AC1 is 1 or 0, indicating if the user has or has not purchased |
AC2 | AC2 is 1 or 0, indicating if the user has or has not clicked the purchase button |
AC3 | AC3 is 1 or 0, indicating if the user has or has not clicked |
Rij | The conversion value of the ith user when purchasing on the jth channel |
CLij | The conversion value of the ith user when clicking the purchase button on the jth channel |
Sij | The conversion value of the ith user then clicking button on the jth channel |
Al | The behavioral variable of the 1st, 2nd … to the lth user |
Zik | The value of Zik is 0 or 1 and represents the ith user of cluster k |
Nk | The number of users with specific behaviors, who have also been contacted and contracted with a purchase. |
C1j | The unit cost required to complete a transaction by the ith user, converted on the jth channel |
C2j | The unit cost required for clicking the purchase button by the ith user, converted on the jth channel |
C3j | The unit cost required for clicking a button by the ith user, converted on the jth channel |
Di | The number of days the ith user is contacted by the selected channel |
di | The maximum number of days the ith user is contacted by the selected channel |
E | The current estimated expense for acquiring customer |
Xij | The value of Xij is 0 or 1, to determine if the ith user would select the jth channel. This is a decision variable. |
Y | Total target value of conversion |
The purpose of using clusters in this study was to treat users with similar past behaviors as the same cluster, to significantly reduce the difference in projection points of similar users that their characteristic behaviors could be analyzed to paint the portraits of them, including aspects of users at different stages of purchase, their preferences and channel utilization. These would eventually serve as the pre-screening of user journey prediction model. According to Lemon and Peter (2016), customers showed different preferences and channel usage at different purchase stages, and we must identify user characteristics to subdivide different specific multiple channels. However, the subdivision of clusters and the number of clusters must be determined according to the data characteristics. Generally speaking, data sets with many user characteristic variables or data sets with a large number of users are usually subdivided into more clusters. However, for data sets with a large number of users or data sets with few user characteristic variables, more clusters would typically yield a poorer outcome.
The clustering selection was determined according to the characteristics of user data and analysis mode. One of the most common approaches is K-means clustering, and is defined as follows: Assuming a data set D:{x1,x2……,xn} of a total number of data n, where k represents the number of groups for user classification, that C:{C1,C2……,Ck} is the centerpoint of the population with k≤n, the k-means algorithm starts by randomly finding k points in the data set as initial center-point(s) and calculates the Euclidean distance from each point to the center of k points(s), designated as dis(xj,Cj)=√∑mj=1|xj−Cj|. Then, the point of the smallest relative distance would be located near the current center point to continue calculating the Euclidean distance until the center point does not change, which means that this center point is the best solution for the group; hence, the completion of clustering.
K-means clustering method needs to define the initial clustering number, and thus, Elbow method and iterative training optimization were first used to find the most suitable target clustering number. By calculating the square after the distance residual, the group number with the minimum sum of the square could be obtained. Through RFM (recency, frequency, monetary) value of users, frequency of channel contact, content information and devices used, user characteristics could be cluster analyzed. According to user's i behavioral variable A, there were l types of behavioral data and based on the A behavioral data of users i, each user i could be categorized to their respective cluster Zik, yielding a total of k groups.
In this study, the model in the second step was the online user journey prediction model. First, the data of users in multiple channels were organized and the order in which users selected channels was defined by each visit to a web page through the channel as a working process, and in sequence, the process and the interaction with the website would be recorded. According to the literature on the attribution model, the channel that the user contacts online and the corresponding behavior will affect the subsequent conversion. Therefore, in this step, the model hopes to combine the concept of customer journey with the prediction model to dynamically predict the subsequent behavior of users and simulate the target value that can be converted under the channel, which can be used as the simulation value of subsequent resource allocation, and to clarify the classification rules through the decision tree method to assist in solving attribution problems in marketing. At this step, two results were to be predicted, namely, the user behavior in the channel and the conversion value under the channel. Since the training materials were sequential in time and categorized, users' online information on certain channels were temporal in properties. In this study, CNN and LSTM, which have excellent dynamic processing capability in terms of time, would be used as the primary analysis models, and were more capable of multivariate classification prediction on users' online data than other more conventional machine learning methods, such as single long short-term memory neural network, random forest, artificial neural network, etc., when compared.
The schematic diagram of CNN-LSTM for user input data is shown in Figure 3:
The defined channel number in combination with the converted binary coding of channel vector, which portrayed the channel click profile of the user, would be sorted in the order of click date, and further supported with predictive variables such as "browsing behavior", "device used", "browser used", "user's RFM", "channel used", and "user luster", while the information browsed by the user, namely, commodity information, home page information, activity information, member information and content information, would be further classified, as the number of browsing on each information was also calculated. Then, the user's behavior at the r+1(th) set of action would be predicted according to accumulative understanding of the behavior from the first to the r(th) act, and respectively so on for the r+2(th) set of action, as predicted from the second to the r+1(th) act, in order to produce a portrait of corresponding r∗(Al+2) pixel size. Then, such picture was input into the first convolution layer, which used 32 convolution kernels of a size of 3*3, with ReLU function as the activation function of this layer, and each convolution layer was matched with a 2*2 pooling layer using the maximum pooling method. Then, the reduced-dimensional picture was input into the second convolution layer, in which 64 convolution kernels of a size of 3*3 are used, where ReLU function was the activation function of this layer, before the reduced-dimensional picture was input into the pooling layer of a size of 2*2 for dimension reduction. After that, once flattened, 25% of nodes and neurons were randomly severed through the dropout layer to prevent over-fitting. Then, it was sent to LSTM layer with 128 output quantity, and it was sent to flatten layer to make the multi-dimensional input one-dimensional, and the full connection layer with softmax function for activation was used as output to classify the result. Adadelta was the optimizer and category cross entropy was used for accuracy and error calculation. Training is carried out through the CNN-LSTM model, verification is carried out with test data, and results are evaluated with multiple classification indexes. The framework of the CNN-LSTM model is shown in Figure 4.
Linear Programming is a fundamental method for solving optimization problems and stands as a significant scientific breakthrough from the mid-20th century. Optimization, in this context, pertains to the allocation and distribution of data resources under constraints, employing rigorous mathematical models or structured computer data planning to achieve the overarching goal of maximizing or minimizing outcomes. When both the mathematical objective function and the model's constraint equations are first-order equations, the approach employed to solve them is termed linear programming. In cases where solving the problem necessitates that variables within the model be integer values, it is referred to as mixed integer linear programming (MILP) or integer programming (IP). In this study, we tackled the channel planning problem by formulating it into an IP mathematical model for resolution. The way to implement integer programming for channel planning can be summarized as follows:
Step 1. Confirmation of system parameters
The parameter issues of channel planning problem for researchers in this study could be categorized in three parts: channel attribute, parameter of user characteristic attribute and target conversion value.
Step 2. Determine the decision variable
The decision variable is the presentable solution to the programming problem. The decision variable in this model is whether the channel is in contact with the user, so this study used a binary integer to represent the planning state Xij, and if its solution was 1, it confirmed the contact with the user and in contrast, if 0 for no contact.
Step 3. Determine the constraint
Determine the resource constraints in the problem and the conditions that the decision variable must meet, and then convert these constraints into linear equations dominated by decision variables. Its linear equations include inequalities or equations. These linear equations are called restrictive equations.
Step 4. Determine the objective function.
Determine the objective of the optimization model. The objective function of this study was to maximize the value of target transformation (Y), and the user behavior was predicted to three actions (AC1,AC2,AC3), so it was converted to a multi-objective equation problem for solving. The objective function and restriction conditions are presented in the form of linear functions of decision variables.
To validate our proposed three-step analysis model, we conducted empirical analysis using online user data provided by a financial organization in Taiwan. This organization implements tracking codes via the Google Tag Manager tool on their website's front page and employs Google Analytics to monitor users' website browsing activities. The data collection period spanned from March 2020 to November 2020, encompassing a dataset of 180,760 user records. The dataset primarily consisted of two types of data: user online data and user transaction data, with each user identified by a unique ID. After merging these two data sources based on user ID, we excluded variables that were not defined by the organization and selected variables of numerical significance, resulting in a set of 34 behavioral variables. Additionally, adhering to Taiwan's Personal Data Protection Law, even though the user IDs were anonymized, our study refrained from utilizing any personal information about these users, including age, location, interests, gender, etc. The combined user data predominantly included information related to user online channels, webpage interactions, transaction histories, and device specifications.
The comparison of literature regarding the variables used in this study is presented in Table 3, while the original data can be found in Tables 4 and 5. Additionally, detailed information about user variables is provided in Table 6.
Variables | Reference |
RFM | Birant (2011). |
Purchase stage | Sun et al. (2022) |
Online advertising forms | De Haan et al. (2016) |
Purchase funnel metrics | De Haan et al. (2016) |
Web behavior | Huang et al. (2007) |
Customer Attribution | He et al. (2014) |
UserID | Source/Medium | Channel | Landing Page Path | Action | Target Conversion |
1587443807754.o1ejysi7 | google/cpc | Paid Search | Product page | Click | 949 |
1587443807754.o1ejysi7 | fuboneip/banner | Display | Event page | Click the purchase button | 3985 |
1587443807754.o1ejysi7 | shop.hsbc.com.tw/referral | Referral | Event page | Purchasing | 10000 |
UserID | Sex | Recency | Frequency | Monetary | Customer Value |
1587443807754.o1ejysi7 | 1 | 43439 | 1 | 779 | 47 |
1584696454073.yx1ajfnt | 1 | 43419 | 1 | 615 | 36 |
1584684955336.odv2og9 | 1 | 43209 | 1 | 303 | 32 |
Feature | Description |
UserID | Unique User ID in the company, used to track the user across multiple sessions using Company Account |
Date | The date of the session formatted as YYYYMMDD. |
Channel | The source of referrals. For manual campaign tracking, it is the value of the utm_source campaign tracking parameter. Defined by the management of the company. |
Device Category | The category of the device: mobile, tablet, or desktop |
Browser | The raw name of the browser |
Recency | How recently a customer has made a purchase. In this research, the higher the score, the longer the interval |
Frequency | How often a customer makes a purchase |
Monetary | How much money a customer spends on purchases |
Revenue | The total sale revenue (excluding shipping and tax) of the transaction. |
Campaign Content | For manual campaign tracking, it is the value of the utm_content campaign tracking parameter. Defined by the management of the company. |
Page Content | A page on the website specified by path and/or query parameters. Use this with hostname to get the page's full URL. The display content is defined by the management of the company. |
User Type | Either New Visitor or Returning Visitor, indicating if the users are new or returning. |
Session Duration | The length of a session measured in seconds and reported in second increments. |
Sessions | The total number of sessions. |
Pages | The average number of pages viewed during a session, including repeated views of a single page. |
Action Stage | The action stage at the end of each session. This is the target feature for this use case. |
This section introduces the definition of online channel. According to the company's channel strategy provided by senior management, there are 7 different types of channel: (1) paid search volume, where company purchases the layout page provided by the search engine that the website is placed in a conspicuous place on the search results page during a specific search; (2) display advertisement, to be placed on a third-party website, differing from the text-linked advertisement on Google, in which its content combines texts, logo, images, etc., for it not only includes the message the brand wants to convey, but also includes the brand trademark and image of the enterprise as a whole; (3) natural search of keyword, where user searches for specific keyword to return with websites to be clicked as such; The similarity and difference between natural search of keyword and paid search of category 4 is that both types are clicked in the search results, but only when the content clicked by the user is paid advertisement by the company that it is classified as category 4, or else, it is classified as category 3; (4) paid search volume, where company purchases the layout page provided by the search engine that the website is placed in a conspicuous place on the search results page during a specific search; (5) self-own media, including mailboxes and text messages, to be clicked through links in e-mail or from mobile phone text messages, as well as social media, Facebook, Twitter or Instagram managed by companies; (6) cross-sales, referring to displaying and selling to existing customer another product B when the user is browsing for product A on the official website, which is the behavior of cross-sales or alliance marketing, which establishes a partnership between enterprises and bloggers or individuals, allowing cooperative websites or blogs to help advertise or market the product, share profit, and achieve the purpose of commodity sales or list collection, similar to today's "sponsored marketing"; (7) direct visitors, who are already very familiar with the website and typically the behavior of members to reach the website by setting the website as my favorite or directly entering the website address without searching or passing through any links; (8) others, when company employs many strategies and methods for advertising, where it involves Google Analytics in analyzing data sources and classifying those channels that cannot be classified, but this category would not be used in this study in order to reduce errors.
In this section, we define the indicators of the evaluation model to evaluate the prediction efficiency of the classification model to build the best prediction model. The method of model training was iterative learning with input of training data, and to train all possible classification rules for prediction by using the mathematical equation set by the classification algorithm. We evaluated the classification accuracy of the model by comparing the actual tag of the test data with the predicted tag of the test data, which would serve as the basis for evaluating the model performance.
Taking the binary classification model as an example, purchase and click are the two possible outcomes. If both the prediction and the actual action are purchase, it is defined as true positive, and if the actual action is purchase while the predicted action is clicking the purchase or any buttons, it is defined as false negative. When the actual action is to click the purchase or any buttons and the predicted action is to purchase, it is defined as false positive. When both the predicted and actual actions are not part of purchase, it is defined as true negative. However, this study yielded three types of output result: purchase, click on the purchase button and click on a button. Therefore, the classification results of the model could be clearly evaluated through the ternary confusion matrix, and the accuracy, F-1 score, and Cohen's kappa coefficiency of the model were also extended to be calculated. As seen, this study used four classification evaluation methods, namely, ternary confusion matrix, F-1 score, accuracy, and Cohen's kappa coefficiency, to comprehensively examine the prediction performance of multivariate classification method.
The classification evaluation formula is calculated as follows:
(1) Accuracy
Accuracy=TP+TNTP+TN+FP+FN | (1) |
TP (true positive), TN (true negative), FP (false positive), FN (false negative)
(2) Precision
Precision=TPTP+FP | (2) |
(3) Recall
Recall=TPTP+FN | (3) |
(4) F-1 score
F1score=2×Precision×RecallPrecision+Recall | (4) |
(5) Cohen's kappa coefficient
Kappa=(p0−pc)(1−pc) | (5) |
(ⅰ) p0 is addition of diagonal elements of the percentage confusion matrix
(ⅱ) pc is sum of each category (the sum of the actual category ⅰ multiplied by the sum of the predicted category ⅰ).
(ⅲ) Kappa is a number between -1 and 1. The larger the value, the better the classification effect is.
Pearson's correlation was used to test the correlation and significance, and the correlation variables between channel use and user behavior were identified to clarify the relationship between user characteristics and channel behavior, and further verify proposition 1 of this study.
The following results are presented in a matrix manner, and the analysis results are shown in Table 7.
[1] | [2] | [3] | [4] | [5] | [6] | [7] | [8] | [9] | [10] | [11] | …. | [34] | |
Recency[1] | 1 | ||||||||||||
Frequency[2] | 0.04∗∗∗ | 1 | |||||||||||
Monetary[3] | 0.01 | 0.14∗∗∗ | 1 | ||||||||||
Enterprise Homepage channel[4] | 0.02. | 0.06∗∗∗ | 0.05∗∗∗ | 1 | |||||||||
External-advertising channel [5] | −0.001 | 0.00 | 0.04 | 0.26∗∗∗ | 1 | ||||||||
Organic-search channel [6] | 0.001 | 0.01 | 0.01 | 0.40 | 0.10∗∗∗ | 1 | |||||||
Keyword-advertising channel[7] | 0.011 | 0.33 | 0.03 | 0.21 | 0.25 | 0.28∗∗∗ | 1 | ||||||
Own-media channel [8] | 0.011 | 0.05 | 0.02 | 0.40 | 0.12 | 0.19 | 0.11∗∗∗ | 1 | |||||
Cross-cooperation channel [9] | −0.003 | 0.01 | 0.00 | 0.45 | 0.05 | 0.80 | 0.17 | 0.48∗∗∗ | 1 | ||||
Direct landing page channel [10] | 0.02. | 0.08 | 0.00 | 0.44 | 0.02 | 0.09 | 0.07 | 0.60 | 0.41∗∗∗ | 1 | |||
Homepage[11] | 0.03∗∗∗ | 0.14 | 0.06 | 0.69 | 0.32 | 0.63 | 0.40 | 0.53 | 0.65 | 0.50∗∗∗ | 1 | ||
Product Information[12] | 0.02.. | 0.09 | 0.04 | 0.72 | 0.44 | 0.53 | 0.33 | 0.62 | 0.62 | 0.42 | 0.83∗∗∗ | 1 | |
Campaign Information[13] | 0.008 | 0.02 | 0.06 | 0.67 | 0.48 | 0.60 | 0.36 | 0.54 | 0.57 | 0.20 | 0.73 | 0.84 | 1 |
Situational Information[14] | 0.015 | 0.05 | 0.02 | 0.54 | 0.19 | 0.59 | 0.20 | 0.70 | 0.78 | 0.56 | 0.69 | 0.73 | 0.61 |
Member Services Information [15] | 0.02∗. | 0.08 | 0.03 | 0.74 | 0.37 | 0.57 | 0.35 | 0.50 | 0.62 | 0.50 | 0.77 | 0.83 | 0.67 |
Pages[16] | 0.02. | 0.07 | 0.05 | 0.75 | 0.45 | 0.63 | 0.37 | 0.64 | 0.69 | 0.42 | 0.86 | 0.97 | 0.92 |
Sessions[17] | 0.01 | 0.06 | 0.03 | 0.75 | 0.20 | 0.77 | 0.29 | 0.62 | 0.90 | 0.54 | 0.83 | 0.81 | 0.76 |
Session Duration[18] | 0.02 | 0.15 | 0.07 | 0.56 | 0.21 | 0.75 | 0.35 | 0.33 | 0.76 | 0.36 | 0.74 | 0.67 | 0.63 |
NewVisitor [19] | 0.02 | 0.22 | 0.00 | 0.31 | 0.27 | 0.59 | 0.49 | 0.08 | 0.56 | 0.22 | 0.58 | 0.54 | 0.44 |
Returning Visitor[20] | 0.04 | 0.25 | 0.09 | 0.68 | 0.31 | 0.60 | 0.47 | 0.51 | 0.63 | 0.49 | 0.87 | 0.82 | 0.70 |
Desktop [21] | 0.04 | 0.12 | 0.04 | 0.69 | 0.28 | 0.62 | 0.41 | 0.53 | 0.66 | 0.49 | 0.86 | 0.82 | 0.71 |
Mobile [22] | 0.03 | 0.24 | 0.05 | 0.50 | 0.20 | 0.53 | 0.45 | 0.40 | 0.67 | 0.54 | 0.69 | 0.63 | 0.49 |
Tablet [23] | 0.02 | 0.02 | 0.00 | 0.24 | 0.64 | 0.02 | 0.24 | 0.00 | 0.00 | 0.00 | 0.17 | 0.31 | 0.37 |
Android Webview[24] | −0.01 | 0.04 | 0.04 | −0.01 | 0.01 | −0.01 | 0.05 | 0.00 | 0.00 | 0.02 | 0.01 | 0.01 | 0.00 |
Opera[25] | 0.01 | 0.03 | 0.00 | 0.00 | 0.01 | 0.00 | 0.02 | 0.00 | 0.00 | 0.02 | 0.01 | 0.01 | 0.00 |
Samsung Internet[26] | 0.01 | 0.04 | 0.00 | 0.20 | 0.00 | 0.03 | 0.13 | 0.00 | 0.01 | 0.23 | 0.17 | 0.07 | 0.00 |
Chorme[27] | 0.04 | 0.12 | 0.04 | 0.69 | 0.28 | 0.62 | 0.41 | 0.53 | 0.66 | 0.49 | 0.86 | 0.82 | 0.71 |
Safari[28] | 0.02 | 0.19 | 0.04 | 0.48 | 0.17 | 0.55 | 0.33 | 0.42 | 0.71 | 0.52 | 0.64 | 0.63 | 0.49 |
Edge[29] | −0.01 | 0.02 | 0.03 | 0.00 | 0.31 | 0.00 | 0.03 | 0.00 | 0.00 | 0.01 | 0.07 | 0.05 | 0.04 |
Firefox[30] | 0.01 | 0.03 | 0.01 | 0.26 | 0.67 | 0.02 | 0.25 | 0.00 | 0.00 | 0.00 | 0.18 | 0.33 | 0.39 |
Internet Explorer[31] | 0.02 | 0.05 | 0.07 | 0.67 | 0.00 | 0.07 | 0.01 | 0.58 | 0.32 | 0.54 | 0.44 | 0.44 | 0.35 |
Clicking[32] | −0.11 | −0.17 | −0.01 | 0.19 | 0.14 | 0.60 | 0.19 | 0.04 | 0.63 | 0.03 | 0.40 | 0.27 | 0.36 |
Clicking "buy now"[33] | 0.10 | 0.00 | 0.06 | 0.70 | 0.29 | 0.33 | 0.29 | 0.60 | 0.36 | 0.56 | 0.74 | 0.80 | 0.62 |
Purchasing[34] | 0.03 | 0.86 | 0.09 | 0.06 | −0.01 | 0.01 | 0.34 | 0.04 | 0.01 | 0.08 | 0.14 | 0.09 | 0.02 |
From Table 3, our analysis reveals that approximately 80% of the variable relationships exhibited statistical significance, while the remaining 20% did not. Notably, the most prominent significant relationships were observed among users' RFM metrics, as well as their channel utilization and browsing behavior. These variables demonstrated predominantly positive correlations. Conversely, the interval time between online sessions and users' transaction frequency exhibited a negative correlation with their click activity. This can be attributed to users with frequent transactions being well-acquainted with the website's purchase process, resulting in a higher propensity for direct purchases rather than extensive clicking. Based on these findings, it is recommended that businesses proactively engage users through channels such as self-owned media, as this was also found to be significantly associated with transaction frequency. However, variables showing a negative correlation included RFM metrics related to device and channel utilization by users, as well as RFM metrics related to browser and channel usage by users. This suggests that the devices used by users were less influenced by the browsers and channels employed. Consequently, browsers and devices used by users may not be suitable as input variables for cluster analysis.
This section was based on the enterprise's member management strategy and subdivided the user characteristics and usage channels according to Lemon and Verhoef's (2016) proposition. By using K-means cluster technique, the behavior data of users would be analyzed, as their transaction data and online behavioral data would be subdivided accordingly to the characteristics to distinguish cluster users with different purchase stages and different online behaviors. Based on the results of the correlation analysis in the previous section, we input variables that showed significant differences in channel usage and behavior and performed K-means analysis to find the most suitable target cluster number k = 7 by calculating the residual sum of squares of errors for different cluster numbers. For the detailed process, please refer to Appendix A.
The user cluster results are shown in Table 8.
Cluster 1 | Cluster 2 | Cluster 3 | Cluster 4 | Cluster 5 | Cluster 6 | Cluster 7 | |
Recency | 33.28 | 1.31 | 52.01 | 27.76 | 32.87 | 40.14 | 24.25 |
Frequency | 0.64 | 0.70 | 1.27 | 0.83 | 0.72 | 0.87 | 0.94 |
Monetary | 947.36 | 2532.60 | 3158.19 | 6799.02 | 9334.24 | 1777.86 | 4946.58 |
Enterprise homepage channel | 1.08 | 1.55 | 3.49 | 3.09 | 3.19 | 1.09 | 1.27 |
External-advertising channel | 0.00 | 0.18 | 0.13 | 0.15 | 0.27 | 0.01 | 0.02 |
Organic-search channel | 1.48 | 1.54 | 2.48 | 2.60 | 2.87 | 1.32 | 1.41 |
Keyword-advertising channel | 4.61 | 4.61 | 8.14 | 5.78 | 2.99 | 5.18 | 5.93 |
Own-media channel | 0.25 | 0.67 | 1.19 | 0.76 | 0.74 | 0.58 | 1.26 |
Cross-cooperation channel | 0.20 | 0.28 | 1.52 | 1.69 | 0.26 | 0.09 | 0.23 |
Direct landing page | 1.14 | 1.20 | 2.52 | 0.73 | 1.34 | 1.78 | 1.52 |
Homepage | 0.22 | 0.04 | 0.41 | 0.22 | 0.24 | 0.21 | 0.14 |
Product information | 0.76 | 0.17 | 1.22 | 0.96 | 0.81 | 0.92 | 1.03 |
Campaign information | 0.01 | 0.01 | 0.16 | 0.14 | 0.12 | 0.03 | 0.06 |
Situational Information | 0.00 | 0.00 | 0.07 | 0.03 | 0.01 | 0.02 | 0.03 |
Member Services information | 0.05 | 0.04 | 0.41 | 0.26 | 0.17 | 0.13 | 0.20 |
Pages | 1.04 | 0.26 | 2.27 | 1.61 | 1.36 | 1.32 | 1.48 |
Sessions | 1.05 | 1.01 | 2.33 | 1.73 | 1.42 | 1.27 | 1.38 |
Session Duration | 964 | 954 | 761 | 817 | 867 | 825 | 795 |
The cluster analysis yielded these key findings and recommendations:
High-Value Clusters: Clusters 3 and 5 stand out as the most valuable in the RFM model. Cluster 3 shows frequent transactions and short intervals, while Cluster 5 demonstrates substantial transaction amounts.
Regular Purchasers: Cluster 3 consists of loyal, regular purchasers who engage extensively with the company's website. They use various channels, such as the homepage, keyword ads, and self-owned media. To engage them effectively, consider proactive communication via email or SMS and regularly showcasing activity information.
High-Spenders: Cluster 5 comprises users with high spending patterns who prefer external advertising and self-owned media for product information. Focus on direct product promotions through external ads and encourage users to land on product introduction pages.
Potential Users: Cluster 4 represents potential users with moderate transaction activity but higher-than-average expenditure. They show interest in campaign and member service information. Utilize cross-cooperation channels and organic searches to foster customer loyalty.
Lapsed Users: Cluster 2 consists of lapsed users with low transaction and website visit rates. Concentrate efforts on external advertising and self-owned media to re-engage them. Tailored content and promotions are crucial to retaining their interest.
These findings provide actionable insights into effectively managing different user segments based on their behavior and preferences.
The second step of user journey prediction was divided into two parts. First, the 7 channels used by the users of input variables were sorted according to the contact order, and there were r = 8 sets of sequence data for each user to predict the user's r+1 = 9th action to be either purchase or clicking (AC1, AC3). This problem was considered as the binary classification prediction issue. By the accuracy of model classification, it was confirmed that LSTM model of deep learning for processing user sequences was better than other non-sequence models in prediction performance, as proposition 2 was verified: a dependency relationship between user's channel sequence data. Then, the mode was changed to predict the behavior of three types of users (AC1, AC2, AC3) in terms of user journey sequence, and user behaviors and cluster tag variables were additionally added. Such problem was a multi-classification prediction issue. Through the accuracy of the multivariate classification model, the best multivariate classification prediction method was selected, to verify if the integrated deep learning method through stacking was more accurate than individual deep learning; hence, the best user journey prediction model. In this section, we used multivariate confusion matrix, F-1 score, accuracy, and Cohen's kappa coefficiency for evaluation and comparison, to establish the best prediction model of user journey. The binary classification comparison included the LSTM model, RF model, and ANN model. The multivariate classification comparison included the CNN-LSTM model, LSTM model, CNN model, and RNN model. The data were randomly divided into 80% training data and 20% test data, and the classification model was trained with the training data. The test data were used to verify its accuracy. The results of binary classification were listed in Table 9 and the multivariate classification results were seen in Table 6. For detailed process of the binary classification, please refer to Appendix B.
Models | F-score | Accuracy | Cohen's |
LSTM | 0.7915 | 0.7987 | 0.5830 |
ANN | 0.7405 | 0.7531 | 0.4802 |
RFs | 0.7694 | 0.7771 | 0.5387 |
Table 10 presents the results of binary classifier comparisons, focusing on predicting user purchases (AC1,AC3) based on user channel sequences. The objective is to validate Proposition 2. The evaluation employs four performance metrics: ternary confusion matrix, F-1 score, accuracy, and Cohen's kappa coefficient. The findings demonstrate that deep learning models, particularly LSTM, outperform traditional machine learning models, highlighting a significant dependency between user channel sequences and subsequent transactions. Moving to Table 11, it expands the analysis to multivariate classifiers predicting three user behaviors (AC1, AC2, AC3) based on channel sequences, user contact information, and user clusters. The aim is to explore the potential of stacking CNN and LSTM models and compare their accuracy with single deep learning methods, ultimately constructing an optimal user journey prediction model and data structure for multi-classification (as shown in Figure 6). Table 10 clearly demonstrates that the stacked deep learning model surpasses single deep learning models in accuracy. All four evaluation metrics confirm that the CNN-LSTM model outperforms other methods, with the performance of single CNN models notably inferior. While CNNs are traditionally used for image classification and lack the order dependence inherent to recurrent neural networks (RNNs) like LSTM, their advantage in forecasting time series lies in dilated convolutions. Dilated convolutions enable filters to compute dilations between cells, effectively capturing differential observations in time series data. Consequently, the integration of LSTM and CNN layers enables LSTM to account for sequential dependencies while benefiting from the informative aspects of CNN layers through dilated convolutions. This study enhances LSTM's classification accuracy by incorporating CNN characteristics, particularly in processing user journey sequence data. By stacking CNN and LSTM models and applying them to user online data, the CNN-LSTM model demonstrates superior predictive accuracy.
Models | F-score | Accuracy | Cohen's |
CNN-LSTM | 0.5618 | 0.5737 | 0.2232 |
LSTM | 0.5374 | 0.5504 | 0.1979 |
ANN | 0.4075 | 0.5019 | 0.1112 |
RFs | 0.5007 | 0.5265 | 0.1371 |
Planning method | Channel No. | 1 | 2 | 3 | 4 | 5 | 6 | 7 | Total | Cost | Revenue(R) |
Manual planning | (Zi5) | 9 | 2 | 5 | 2 | 5 | 1 | 1 | 25 | 9830 | 17418 |
(Zi3) | 7 | 0 | 0 | 12 | 6 | 0 | 0 | 25 | |||
(Zi4) | 15 | 0 | 3 | 6 | 0 | 1 | 0 | 25 | |||
(Zi2) | 17 | 0 | 3 | 3 | 1 | 0 | 1 | 25 | |||
Total | 48 | 2 | 11 | 23 | 12 | 2 | 2 | 100 | |||
Goal 1 IP-model planning | (Zi5) | 5 | 0 | 1 | 1 | 4 | 0 | 14 | 25 | 4110 | 26778 |
(Zi3) | 4 | 0 | 1 | 0 | 1 | 3 | 16 | 25 | |||
(Zi4) | 0 | 1 | 1 | 0 | 9 | 2 | 12 | 25 | |||
(Zi2) | 0 | 3 | 0 | 0 | 1 | 6 | 15 | 25 | |||
Total | 9 | 4 | 3 | 1 | 15 | 11 | 57 | 100 | |||
Planning method | Channel No. | 1 | 2 | 3 | 4 | 5 | 6 | 7 | Total | Cost | Value(Y) |
Goal 2 IP-model planning | (Zi5) | 5 | 0 | 1 | 1 | 4 | 0 | 14 | 25 | 7408 | 35559 |
(Zi3) | 6 | 0 | 0 | 0 | 1 | 3 | 15 | 25 | |||
(Zi4) | 2 | 10 | 0 | 0 | 1 | 0 | 12 | 25 | |||
(Zi2) | 0 | 3 | 0 | 0 | 1 | 6 | 15 | 25 | |||
Total | 13 | 13 | 1 | 1 | 7 | 9 | 56 | 100 |
Looking back on the problem description in Chapter 3, what we finally need to solve is how to allocate the optimal resources of the channel through the three-step model to assist the top managers of the enterprise to plan the strategy to pursue the maximum conversion revenue (Y). In this section, the current results and models were further integrated and analyzed. By clustering the results of the first step, the users' cluster attribution was analyzed (Zik). CNN-LSTM of the second step predicted the subsequent behavior of users under the customer's journey sequence (i.e., to purchase (AC1), to click the purchase button (AC2), or to click (AC3)) and combined the target conversion values (Rij,CLij,Sij), as predicted by the decision tree in the previous section with the actual planning channel data during the period provided by the enterprise, including current estimated acquisition cost (E), cost-per-acquisition/conversion (C1j,C2j), cost-per-click (C3j), and the number of day of contact through channel (Di). The current estimated customer acquisition cost (E) is the budget compiled by the enterprise in the previous year, so it is usually a fixed value. However, when an enterprise signs a contract with an advertiser, cost-per-acquisition/conversion (C1j,C2j) and cost-per-click (C3j) do not fluctuate significantly and thus, the price range can be set based on the contract by the mathematical model. Based on the above, the integrated model was used to solve the channel planning problem in description by integer programming. For mathematical symbols, variable definitions, and mathematical models, please refer to Figure 3 and Figure 4 in Chapter 3 of this study, respectively. The target conversion value (Y) of manual planning under different strategies and restrictions during the period were compared to verify proposition 3. However, we verified proposition 3 with several examples of companies, that even under different goals, users and the final converted channels would yield different matching results. Through interviews with senior managers, we have summarized two main strategies of enterprises at present, namely, revenue-oriented strategy for value conversion and flow-oriented strategy for target conversion (via click). However, we set up the model according to two companies' objectives and analyzed the channel planning results. 100 users of high-consumption cluster, regular repurchase cluster, potential cluster, loss cluster (Zi5, Zi3, Zi4, Zi2), and 7 (j=7) channels were respectively selected by random stratified sampling as input data for model analysis and verification.
Table 11 shows the results of manual planning and two types of company target integer programming model. Manual planning included the results of the enterprise's current planning and conversion. Target 1: the company pursues a larger revenue (R); Target 2: The enterprise is not geared to promoting purchase. The target will consider three types of action: to purchase (AC1), to click the purchase button (AC2), or to click a button (AC3). the company pursues a larger goal conversion (Y). The results of Table 6 showed the revenue of manual planning (R) at 17418, while the characteristics of users were shown as the results of previous clusters, (shown in Table 3), and most clusters used familiar channels for final conversion. However, in the case of using the same channels and users, re-planning of 7 different channels to contact different users using and implementing the IP model of the target 1, as shown in Table 12, its main channel cost should be concentrated in the primary high-consumption group (Zi5) and the regular repurchase group (Zi3), in order for the final channel combination to be concentrated in the conversion channels commonly used by these high-value groups; thus, verifying Lemon and Verhoef's (2016) proposition 3: the benefits converted by the purchase channel were more useful than the channels converted by other behaviors. The best results obtained from the model of target 1 showed that the revenue of (R) was greatly improved compared with the manual planning, from 17418 to 26778, increasing 54% in revenue and reducing 58% in cost. In other words, under the same restrictions, the proposed IP model could produce better resource allocation combination than the manual planning by enterprises. However, the main difference between the IP model of target 2 and the IP model of target 1 was in the comprehensive consideration of multi-objective formula and the setting of the upper limit of the number of shared populations. When the IP model of target 2 was implemented, in order to pursue the maximum target conversion value, the high consumption group (Zi5) and the regular repurchase group (Zi3) would be arranged to be contacted by the appropriate channels. Then, since the model of target 2 took into account the implemented multi-objective formula (∑ni∑mjRijXij+∑ni∑mjCLijXij+∑ni∑mjSijXij), it would be matched with the high-conversion value group after completing matching with the high-value group. In other words, IP model of target 2 would prioritize users and channels that led to more "purchase" action in order to maximize the target conversion value. The combination distribution in the final Table 11 verifies our hypothesis. Channel 7 was the most commonly used, followed by 1 and 2. After completing the matching of high-value groups, the model would begin to match the exposure/inducing channel with the cluster of potential users Zi4 in order to obtain the maximum target conversion value. Restrictions on the number of focus groups can assist companies in membership control (∑niZikXij≤Nk).
Enterprise online channel/Target customer cluster | This channel is the main customer touch point in the target market | This channel is not the main customer touch point in the target market |
The online channels of the enterprise result in higher conversion values for purchases among the targeted cluster customers | Continuing to optimize the channel planning while exercising caution against over-reliance on online channels to prevent customer fatigue at this touchpoint and potential challenges such as fierce competition in advertising bids. | Conduct a cost assessment to determine the feasibility of increasing channel resources for customer engagement. This channel could serve as a valuable avenue for interacting with customers and might represent a blue ocean market in the competitive landscape of advertising bids. |
The online channels of the enterprise result in lower conversion values for purchases among the targeted cluster customers | Adjust and optimize the content and service experience of the channel to assess if there is a potential for improving the channel conversion rate. | Realign the target customer clusters and channel selection, continuously adjusting communication content and service experience design. |
We've developed a systematic program for predicting users' journey behavior, optimizing channel resource allocation, and maximizing conversion profit. Our objective is not to provide perfect predictions due to external uncertainties but to assist decision-makers in formulating effective channel planning and business strategies. The program integrates various research in marketing and operations management and comprises six key steps:
Market definition and segmentation: Identify major users and segment the market based on user characteristics, focusing on online behavior, transaction data, RFM values, and contact information.
User journey prediction: Predict future user behaviors (AC1, AC2, AC3) based on past user journeys. Utilize sequence processing methods like LSTM models, which outperform standalone deep learning models, to understand cross-channel user behavior.
Customer switching value estimation: Quantitatively evaluate and establish decision rules for target simulations. Use decision tree frameworks to predict switching values of users under various channel and cluster combinations. Determine which clusters to target and which channels to use based on business objectives.
Optimal channel planning analysis: Allocate channel resources optimally, considering differences in channel revenue and costs at different purchase stages. Pursue maximum conversion revenue by following a three-step model.
Scheme analysis: Develop multiple possible schemes based on enterprise goals and resources. Create mathematical models to simulate future market scenarios, leveraging estimated values from the three-step model. Iterate and refine schemes to reach a feasible plan.
Continuous monitoring and adjustment: Continuously monitor future events, update information, and make necessary adjustments to strategic actions based on evolving circumstances.
This process enables decision-makers to predict user behavior and optimize channel planning while ensuring dynamic adaptability. We illustrate its application by grouping new users based on behavioral patterns and using the CNN-LSTM model to predict their actions. This approach assists managers in dynamically understanding and managing user actions, tailoring strategies to different user groups. Practical implementations help companies make informed decisions, formulate standards, and allocate budgets for various online channels, enhancing their decision-making processes.
Upon delineating the decision-making process, our focus shifts towards deriving management implications through the aforementioned methodology. The extensive examination of customer experience by Lemon and Verhoef (2016) underscores the imperative for businesses to consistently reassess and optimize customer experiences. This involves progressing towards a more diverse and systematic service encounter. Customer journey management, emphasizing touchpoints spanning the pre-purchase, purchase, and post-purchase stages, assumes a pivotal role. It encompasses any channel through which customers engage with a company's products or services, fostering a holistic service experience. Subsequently, tailored solutions can be crafted to yield high-quality customer experience effects.
This study aims to elucidate the online channel preferences of customers in the financial industry through meticulous data analysis. It seeks to discern key customer characteristics based on online insurance data and evaluate the alignment of the case company's channel planning strategy with the behavioral patterns of target customers. The objective is to formulate a 2X2 channel planning matrix applicable to financial and insurance companies. This knowledge is anticipated to be invaluable for applying service experience theory and implementing practical customer journey strategies, as illustrated in the subsequent table:
This study endeavors to practically apply the methodology and multi-channel planning model in customer management within the financial insurance industry. To that end, an integrated three-stage analytical framework recommending channel planning has been developed by incorporating machine learning, deep technology, and integer programming. This model considers the conversion goals of online customers and automatically suggests channel planning information aligned with customer behavior.
In our research, we constructed an artificial intelligence architecture based on concepts from the current literature regarding customer journey and customer experience. We explored four different deep learning techniques, integrated them with integer programming, and conducted optimization tests. Through meticulous selection of the best-performing predictive models, our integrated model showcases exceptional predictive capabilities and robustness compared to other benchmark models. It presents a novel integrated approach for multi-channel analysis and customer relationship management modeling, establishing our model as a benchmark in the literature.
Notably, there is limited prior research applying such technology to revenue optimization through user journey prediction. We demonstrated how CNN-LSTM can predict user behavior and used decision trees to allocate resources effectively, maximizing revenue in channel planning through integer programming optimization.
The dynamics in our model are implicit and rooted in the analysis of user behaviors in online channels:
User behavior dynamics: The customer segmentation model considers the dynamic characteristics of user behavior in online channels. Through a thorough examination of user interactions, our model inherently captures the dynamic patterns and trends exhibited by users over time.
Real-Time assessment: The evaluation of customer conversion value model includes a real-time assessment of user conversion value, encompassing webpage clicks, purchase clicks, and actual purchases. This real-time evaluation acknowledges the dynamic nature of user engagement and conversions, providing a more nuanced understanding of how user behaviors evolve over time.
Optimized channel planning: The utilization of deep learning and integer programming models enhances the adaptability of our analysis to the continually changing digital environment. Deep learning allows the model to learn and adapt to evolving user behaviors, accurately predicting customer conversion value and ensuring that the channel planning model can respond in real-time to dynamic patterns in online channels.
In conclusion, our study provides a practical guide to integrating deep learning prediction with business operations management. We addressed complex business problems through mathematical modeling and demonstrated the effectiveness of our approach using empirical data. Our work contributes to the understanding of user behavior prediction, channel management, and resource allocation for online platforms.
This study has certain limitations. First, our experimental data is limited to the insurance sector within the financial industry. Therefore, it is important to recognize that the best predictive models proposed in our study may not be universally applicable across different financial sectors, path classifications, or experimental scenarios. To address this limitation, we use different data sizes for model validation and develop a generic online path classification for the insurance industry to compare path planning between different clusters. This strategy draws inspiration from methodologies highlighted in the academic literature and aims to enhance the robustness of our findings, particularly considering the constraints imposed by changes in industry, customer profiles, and pipeline categories.
The author affirms that no artificial intelligence (AI) tools are used in the creation of this work.
The author declares no conflicts of interest in this paper.
[1] |
Z. Huang, J. Zhang, H. Shan, When age-invariant face recognition meets face age synthesis: A multi-task learning framework and a new benchmark, IEEE Trans. Pattern Anal. Mach. Intell., 45 (2023), 7917–7932. https://doi.org/10.1109/TPAMI.2022.3217882 doi: 10.1109/TPAMI.2022.3217882
![]() |
[2] | A. M. Abu Nada, E. Alajrami, A. A. Al-Saqqa, S. Abu-Naser, Age and gender prediction and validation through single user images using CNN, Int. J. Acad. Eng. Res., 4 (2020), 21–24. |
[3] | I. Rafique, A. Hamid, S. Naseer, M. Asad, M. Awais, T. Yasir, Age and gender prediction using deep convolutional neural networks, in 2019 International Conference on Innovative Computing (ICIC), 2019, 1–6. https://doi.org/10.1109/ICIC48496.2019.8966704. |
[4] |
A. Othmani, A. R. Taleb, H. Abdelkawy, A. Hadid, Age estimation from faces using deep learning: A comparative analysis, Comput. Vision Image Understanding, 196 (2020). https://doi.org/10.1016/j.cviu.2020.102961 doi: 10.1016/j.cviu.2020.102961
![]() |
[5] |
N. Sharma, R. Sharma, N. Jindal, Face-based age and gender estimation using improved convolutional neural network approach, Wireless Pers. Commun., 124 (2022), 3035–3054. https://doi.org/10.1007/s11277-022-09501-8 doi: 10.1007/s11277-022-09501-8
![]() |
[6] |
A. Sakata, N. Takemura, Y. Yagi, Gait-based age estimation using multi-stage convolutional neural network, IPSJ Trans. Comput. Vision Appl., 4 (2019), 1–10. https://doi.org/10.1186/s41074-019-0054-2 doi: 10.1186/s41074-019-0054-2
![]() |
[7] |
C. Y. Hsu, L. E. Lin, C. H. Lin, Age and gender recognition with random occluded data augmentation on facial images, Multimedia Tools Appl., 80 (2021), 11631–11653. https://doi.org/10.1007/s11042-020-10141-y doi: 10.1007/s11042-020-10141-y
![]() |
[8] | B. B. Mamatkulovich, H. A. Alijon o'g'li, Facial image-based gender and age estimation, Eurasian Sci. Her., 18 (2023), 47–50. |
[9] | L. Li, H. T. Lin, Ordinal regression by extended binary classification, Adv. Neural Inf. Process. Syst., 19 (2006). |
[10] |
W. Cao, V. Mirjalili, S. Raschka, Rank consistent ordinal regression for neural networks with application to age estimation, Pattern Recognit. Lett., 140 (2020), 325–331. https://doi.org/10.1016/j.patrec.2020.11.008 doi: 10.1016/j.patrec.2020.11.008
![]() |
[11] | J. Paplham, V. Franc, Unraveling the age estimation puzzle: Comparative analysis of deep learning approaches for facial age estimation, arXiv preprint, (2023), arXiv: 2307.04570. https://doi.org/10.48550/arXiv.2307.04570 |
[12] | J. Dai, H. Qi, Y. Xiong, Y. Li, G. Zhang, H. Hu, et al., Deformable convolutional networks, in Proceedings of the IEEE International Conference on Computer Vision, (2017), 764–773. |
[13] | Z. Niu, M. Zhou, L. Wang, X. Gao, G. Hua, Ordinal regression with multiple output CNN for age estimation, in Proceedings of the IEEE conference on computer Vision and Pattern Recognition, (2016), 4920–4928. |
Type | Research Topic | Methodology | Advantages | Disadvantages | Purpose |
Customer | 1. Purchase Conversion Assessment 2. Consumer Behavior Analysis |
- RFM Model - NES Model |
Analyzing customer databases to establish models through multivariate relationships, understanding customer management approaches. | The constructed models are reusable and effectively integrate predictive variables with faster execution speed. Analysis results may be suboptimal if they violate normality or variable assumptions. The model is challenging to analyze dynamic customer data. | The main objective is to explore the correlation between customer data and order transactions, enabling marketing efforts to be more precise and aligned with customer patterns to achieve business goals. |
Channel | 1. Multichannel Analysis 2. Customer Journey Analysis |
- Channel Path Model - Advertisement Traffic Analysis Model - Multichannel Attribution Model |
Optimizes resource allocation for small-scale and distribution problems, providing models close to real scenarios with easily understandable analysis rules. | Lacks the ability to process large amounts of data, fault tolerance, associative learning, and has poor predictive capabilities. The model complexity may result in a large data space and extended processing time, limiting practical applications. | The primary goal is to use mathematical planning methods to evaluate channel or advertisement effectiveness, extracting high-performing channel allocation rules to develop advertisement budget allocation models. |
Recomme-ndation -System | 1. User Recommendations 2. Content Recommendations |
Machine Learning/Deep Learning | Predictive model with rigorous mathematical inference, massive parallel processing capabilities, fault tolerance, high associative capabilities, and noise filtering capabilities without requiring many assumptions. | Poor interpretability due to its black-box nature, and the integration of complex models may lead to long processing times during training. | The main focus is on finding the most accurate model, adjusting optimal parameters for continuous improvement of predictive patterns. |
Integration Model | This study proposes an optimized integration model, suitable for solving high-dimensional and complex constraint problems. It demonstrates practical feasibility for large-scale issues and dynamic problem-solving. | Appropriate model selection is crucial, and the model may face challenges in convergence. The solution obtained may be a local optimum or feasible solution, with no guarantee of being a global optimal solution. | The objective is to find an approximate solution within a limited computational time, serving as a viable decision-making approach. |
Symbol | Definition |
i | The 1st, 2nd, …………n(th) user |
j | The 1st, 2nd, …………m(th) channel |
t | The 1st, 2nd …………r(th) click |
k | The 1st, 2nd …………k(th) cluster |
AC1 | AC1 is 1 or 0, indicating if the user has or has not purchased |
AC2 | AC2 is 1 or 0, indicating if the user has or has not clicked the purchase button |
AC3 | AC3 is 1 or 0, indicating if the user has or has not clicked |
Rij | The conversion value of the ith user when purchasing on the jth channel |
CLij | The conversion value of the ith user when clicking the purchase button on the jth channel |
Sij | The conversion value of the ith user then clicking button on the jth channel |
Al | The behavioral variable of the 1st, 2nd … to the lth user |
Zik | The value of Zik is 0 or 1 and represents the ith user of cluster k |
Nk | The number of users with specific behaviors, who have also been contacted and contracted with a purchase. |
C1j | The unit cost required to complete a transaction by the ith user, converted on the jth channel |
C2j | The unit cost required for clicking the purchase button by the ith user, converted on the jth channel |
C3j | The unit cost required for clicking a button by the ith user, converted on the jth channel |
Di | The number of days the ith user is contacted by the selected channel |
di | The maximum number of days the ith user is contacted by the selected channel |
E | The current estimated expense for acquiring customer |
Xij | The value of Xij is 0 or 1, to determine if the ith user would select the jth channel. This is a decision variable. |
Y | Total target value of conversion |
Variables | Reference |
RFM | Birant (2011). |
Purchase stage | Sun et al. (2022) |
Online advertising forms | De Haan et al. (2016) |
Purchase funnel metrics | De Haan et al. (2016) |
Web behavior | Huang et al. (2007) |
Customer Attribution | He et al. (2014) |
UserID | Source/Medium | Channel | Landing Page Path | Action | Target Conversion |
1587443807754.o1ejysi7 | google/cpc | Paid Search | Product page | Click | 949 |
1587443807754.o1ejysi7 | fuboneip/banner | Display | Event page | Click the purchase button | 3985 |
1587443807754.o1ejysi7 | shop.hsbc.com.tw/referral | Referral | Event page | Purchasing | 10000 |
UserID | Sex | Recency | Frequency | Monetary | Customer Value |
1587443807754.o1ejysi7 | 1 | 43439 | 1 | 779 | 47 |
1584696454073.yx1ajfnt | 1 | 43419 | 1 | 615 | 36 |
1584684955336.odv2og9 | 1 | 43209 | 1 | 303 | 32 |
Feature | Description |
UserID | Unique User ID in the company, used to track the user across multiple sessions using Company Account |
Date | The date of the session formatted as YYYYMMDD. |
Channel | The source of referrals. For manual campaign tracking, it is the value of the utm_source campaign tracking parameter. Defined by the management of the company. |
Device Category | The category of the device: mobile, tablet, or desktop |
Browser | The raw name of the browser |
Recency | How recently a customer has made a purchase. In this research, the higher the score, the longer the interval |
Frequency | How often a customer makes a purchase |
Monetary | How much money a customer spends on purchases |
Revenue | The total sale revenue (excluding shipping and tax) of the transaction. |
Campaign Content | For manual campaign tracking, it is the value of the utm_content campaign tracking parameter. Defined by the management of the company. |
Page Content | A page on the website specified by path and/or query parameters. Use this with hostname to get the page's full URL. The display content is defined by the management of the company. |
User Type | Either New Visitor or Returning Visitor, indicating if the users are new or returning. |
Session Duration | The length of a session measured in seconds and reported in second increments. |
Sessions | The total number of sessions. |
Pages | The average number of pages viewed during a session, including repeated views of a single page. |
Action Stage | The action stage at the end of each session. This is the target feature for this use case. |
[1] | [2] | [3] | [4] | [5] | [6] | [7] | [8] | [9] | [10] | [11] | …. | [34] | |
Recency[1] | 1 | ||||||||||||
Frequency[2] | 0.04∗∗∗ | 1 | |||||||||||
Monetary[3] | 0.01 | 0.14∗∗∗ | 1 | ||||||||||
Enterprise Homepage channel[4] | 0.02. | 0.06∗∗∗ | 0.05∗∗∗ | 1 | |||||||||
External-advertising channel [5] | −0.001 | 0.00 | 0.04 | 0.26∗∗∗ | 1 | ||||||||
Organic-search channel [6] | 0.001 | 0.01 | 0.01 | 0.40 | 0.10∗∗∗ | 1 | |||||||
Keyword-advertising channel[7] | 0.011 | 0.33 | 0.03 | 0.21 | 0.25 | 0.28∗∗∗ | 1 | ||||||
Own-media channel [8] | 0.011 | 0.05 | 0.02 | 0.40 | 0.12 | 0.19 | 0.11∗∗∗ | 1 | |||||
Cross-cooperation channel [9] | −0.003 | 0.01 | 0.00 | 0.45 | 0.05 | 0.80 | 0.17 | 0.48∗∗∗ | 1 | ||||
Direct landing page channel [10] | 0.02. | 0.08 | 0.00 | 0.44 | 0.02 | 0.09 | 0.07 | 0.60 | 0.41∗∗∗ | 1 | |||
Homepage[11] | 0.03∗∗∗ | 0.14 | 0.06 | 0.69 | 0.32 | 0.63 | 0.40 | 0.53 | 0.65 | 0.50∗∗∗ | 1 | ||
Product Information[12] | 0.02.. | 0.09 | 0.04 | 0.72 | 0.44 | 0.53 | 0.33 | 0.62 | 0.62 | 0.42 | 0.83∗∗∗ | 1 | |
Campaign Information[13] | 0.008 | 0.02 | 0.06 | 0.67 | 0.48 | 0.60 | 0.36 | 0.54 | 0.57 | 0.20 | 0.73 | 0.84 | 1 |
Situational Information[14] | 0.015 | 0.05 | 0.02 | 0.54 | 0.19 | 0.59 | 0.20 | 0.70 | 0.78 | 0.56 | 0.69 | 0.73 | 0.61 |
Member Services Information [15] | 0.02∗. | 0.08 | 0.03 | 0.74 | 0.37 | 0.57 | 0.35 | 0.50 | 0.62 | 0.50 | 0.77 | 0.83 | 0.67 |
Pages[16] | 0.02. | 0.07 | 0.05 | 0.75 | 0.45 | 0.63 | 0.37 | 0.64 | 0.69 | 0.42 | 0.86 | 0.97 | 0.92 |
Sessions[17] | 0.01 | 0.06 | 0.03 | 0.75 | 0.20 | 0.77 | 0.29 | 0.62 | 0.90 | 0.54 | 0.83 | 0.81 | 0.76 |
Session Duration[18] | 0.02 | 0.15 | 0.07 | 0.56 | 0.21 | 0.75 | 0.35 | 0.33 | 0.76 | 0.36 | 0.74 | 0.67 | 0.63 |
NewVisitor [19] | 0.02 | 0.22 | 0.00 | 0.31 | 0.27 | 0.59 | 0.49 | 0.08 | 0.56 | 0.22 | 0.58 | 0.54 | 0.44 |
Returning Visitor[20] | 0.04 | 0.25 | 0.09 | 0.68 | 0.31 | 0.60 | 0.47 | 0.51 | 0.63 | 0.49 | 0.87 | 0.82 | 0.70 |
Desktop [21] | 0.04 | 0.12 | 0.04 | 0.69 | 0.28 | 0.62 | 0.41 | 0.53 | 0.66 | 0.49 | 0.86 | 0.82 | 0.71 |
Mobile [22] | 0.03 | 0.24 | 0.05 | 0.50 | 0.20 | 0.53 | 0.45 | 0.40 | 0.67 | 0.54 | 0.69 | 0.63 | 0.49 |
Tablet [23] | 0.02 | 0.02 | 0.00 | 0.24 | 0.64 | 0.02 | 0.24 | 0.00 | 0.00 | 0.00 | 0.17 | 0.31 | 0.37 |
Android Webview[24] | −0.01 | 0.04 | 0.04 | −0.01 | 0.01 | −0.01 | 0.05 | 0.00 | 0.00 | 0.02 | 0.01 | 0.01 | 0.00 |
Opera[25] | 0.01 | 0.03 | 0.00 | 0.00 | 0.01 | 0.00 | 0.02 | 0.00 | 0.00 | 0.02 | 0.01 | 0.01 | 0.00 |
Samsung Internet[26] | 0.01 | 0.04 | 0.00 | 0.20 | 0.00 | 0.03 | 0.13 | 0.00 | 0.01 | 0.23 | 0.17 | 0.07 | 0.00 |
Chorme[27] | 0.04 | 0.12 | 0.04 | 0.69 | 0.28 | 0.62 | 0.41 | 0.53 | 0.66 | 0.49 | 0.86 | 0.82 | 0.71 |
Safari[28] | 0.02 | 0.19 | 0.04 | 0.48 | 0.17 | 0.55 | 0.33 | 0.42 | 0.71 | 0.52 | 0.64 | 0.63 | 0.49 |
Edge[29] | −0.01 | 0.02 | 0.03 | 0.00 | 0.31 | 0.00 | 0.03 | 0.00 | 0.00 | 0.01 | 0.07 | 0.05 | 0.04 |
Firefox[30] | 0.01 | 0.03 | 0.01 | 0.26 | 0.67 | 0.02 | 0.25 | 0.00 | 0.00 | 0.00 | 0.18 | 0.33 | 0.39 |
Internet Explorer[31] | 0.02 | 0.05 | 0.07 | 0.67 | 0.00 | 0.07 | 0.01 | 0.58 | 0.32 | 0.54 | 0.44 | 0.44 | 0.35 |
Clicking[32] | −0.11 | −0.17 | −0.01 | 0.19 | 0.14 | 0.60 | 0.19 | 0.04 | 0.63 | 0.03 | 0.40 | 0.27 | 0.36 |
Clicking "buy now"[33] | 0.10 | 0.00 | 0.06 | 0.70 | 0.29 | 0.33 | 0.29 | 0.60 | 0.36 | 0.56 | 0.74 | 0.80 | 0.62 |
Purchasing[34] | 0.03 | 0.86 | 0.09 | 0.06 | −0.01 | 0.01 | 0.34 | 0.04 | 0.01 | 0.08 | 0.14 | 0.09 | 0.02 |
Cluster 1 | Cluster 2 | Cluster 3 | Cluster 4 | Cluster 5 | Cluster 6 | Cluster 7 | |
Recency | 33.28 | 1.31 | 52.01 | 27.76 | 32.87 | 40.14 | 24.25 |
Frequency | 0.64 | 0.70 | 1.27 | 0.83 | 0.72 | 0.87 | 0.94 |
Monetary | 947.36 | 2532.60 | 3158.19 | 6799.02 | 9334.24 | 1777.86 | 4946.58 |
Enterprise homepage channel | 1.08 | 1.55 | 3.49 | 3.09 | 3.19 | 1.09 | 1.27 |
External-advertising channel | 0.00 | 0.18 | 0.13 | 0.15 | 0.27 | 0.01 | 0.02 |
Organic-search channel | 1.48 | 1.54 | 2.48 | 2.60 | 2.87 | 1.32 | 1.41 |
Keyword-advertising channel | 4.61 | 4.61 | 8.14 | 5.78 | 2.99 | 5.18 | 5.93 |
Own-media channel | 0.25 | 0.67 | 1.19 | 0.76 | 0.74 | 0.58 | 1.26 |
Cross-cooperation channel | 0.20 | 0.28 | 1.52 | 1.69 | 0.26 | 0.09 | 0.23 |
Direct landing page | 1.14 | 1.20 | 2.52 | 0.73 | 1.34 | 1.78 | 1.52 |
Homepage | 0.22 | 0.04 | 0.41 | 0.22 | 0.24 | 0.21 | 0.14 |
Product information | 0.76 | 0.17 | 1.22 | 0.96 | 0.81 | 0.92 | 1.03 |
Campaign information | 0.01 | 0.01 | 0.16 | 0.14 | 0.12 | 0.03 | 0.06 |
Situational Information | 0.00 | 0.00 | 0.07 | 0.03 | 0.01 | 0.02 | 0.03 |
Member Services information | 0.05 | 0.04 | 0.41 | 0.26 | 0.17 | 0.13 | 0.20 |
Pages | 1.04 | 0.26 | 2.27 | 1.61 | 1.36 | 1.32 | 1.48 |
Sessions | 1.05 | 1.01 | 2.33 | 1.73 | 1.42 | 1.27 | 1.38 |
Session Duration | 964 | 954 | 761 | 817 | 867 | 825 | 795 |
Models | F-score | Accuracy | Cohen's |
LSTM | 0.7915 | 0.7987 | 0.5830 |
ANN | 0.7405 | 0.7531 | 0.4802 |
RFs | 0.7694 | 0.7771 | 0.5387 |
Models | F-score | Accuracy | Cohen's |
CNN-LSTM | 0.5618 | 0.5737 | 0.2232 |
LSTM | 0.5374 | 0.5504 | 0.1979 |
ANN | 0.4075 | 0.5019 | 0.1112 |
RFs | 0.5007 | 0.5265 | 0.1371 |
Planning method | Channel No. | 1 | 2 | 3 | 4 | 5 | 6 | 7 | Total | Cost | Revenue(R) |
Manual planning | (Zi5) | 9 | 2 | 5 | 2 | 5 | 1 | 1 | 25 | 9830 | 17418 |
(Zi3) | 7 | 0 | 0 | 12 | 6 | 0 | 0 | 25 | |||
(Zi4) | 15 | 0 | 3 | 6 | 0 | 1 | 0 | 25 | |||
(Zi2) | 17 | 0 | 3 | 3 | 1 | 0 | 1 | 25 | |||
Total | 48 | 2 | 11 | 23 | 12 | 2 | 2 | 100 | |||
Goal 1 IP-model planning | (Zi5) | 5 | 0 | 1 | 1 | 4 | 0 | 14 | 25 | 4110 | 26778 |
(Zi3) | 4 | 0 | 1 | 0 | 1 | 3 | 16 | 25 | |||
(Zi4) | 0 | 1 | 1 | 0 | 9 | 2 | 12 | 25 | |||
(Zi2) | 0 | 3 | 0 | 0 | 1 | 6 | 15 | 25 | |||
Total | 9 | 4 | 3 | 1 | 15 | 11 | 57 | 100 | |||
Planning method | Channel No. | 1 | 2 | 3 | 4 | 5 | 6 | 7 | Total | Cost | Value(Y) |
Goal 2 IP-model planning | (Zi5) | 5 | 0 | 1 | 1 | 4 | 0 | 14 | 25 | 7408 | 35559 |
(Zi3) | 6 | 0 | 0 | 0 | 1 | 3 | 15 | 25 | |||
(Zi4) | 2 | 10 | 0 | 0 | 1 | 0 | 12 | 25 | |||
(Zi2) | 0 | 3 | 0 | 0 | 1 | 6 | 15 | 25 | |||
Total | 13 | 13 | 1 | 1 | 7 | 9 | 56 | 100 |
Enterprise online channel/Target customer cluster | This channel is the main customer touch point in the target market | This channel is not the main customer touch point in the target market |
The online channels of the enterprise result in higher conversion values for purchases among the targeted cluster customers | Continuing to optimize the channel planning while exercising caution against over-reliance on online channels to prevent customer fatigue at this touchpoint and potential challenges such as fierce competition in advertising bids. | Conduct a cost assessment to determine the feasibility of increasing channel resources for customer engagement. This channel could serve as a valuable avenue for interacting with customers and might represent a blue ocean market in the competitive landscape of advertising bids. |
The online channels of the enterprise result in lower conversion values for purchases among the targeted cluster customers | Adjust and optimize the content and service experience of the channel to assess if there is a potential for improving the channel conversion rate. | Realign the target customer clusters and channel selection, continuously adjusting communication content and service experience design. |
Type | Research Topic | Methodology | Advantages | Disadvantages | Purpose |
Customer | 1. Purchase Conversion Assessment 2. Consumer Behavior Analysis |
- RFM Model - NES Model |
Analyzing customer databases to establish models through multivariate relationships, understanding customer management approaches. | The constructed models are reusable and effectively integrate predictive variables with faster execution speed. Analysis results may be suboptimal if they violate normality or variable assumptions. The model is challenging to analyze dynamic customer data. | The main objective is to explore the correlation between customer data and order transactions, enabling marketing efforts to be more precise and aligned with customer patterns to achieve business goals. |
Channel | 1. Multichannel Analysis 2. Customer Journey Analysis |
- Channel Path Model - Advertisement Traffic Analysis Model - Multichannel Attribution Model |
Optimizes resource allocation for small-scale and distribution problems, providing models close to real scenarios with easily understandable analysis rules. | Lacks the ability to process large amounts of data, fault tolerance, associative learning, and has poor predictive capabilities. The model complexity may result in a large data space and extended processing time, limiting practical applications. | The primary goal is to use mathematical planning methods to evaluate channel or advertisement effectiveness, extracting high-performing channel allocation rules to develop advertisement budget allocation models. |
Recomme-ndation -System | 1. User Recommendations 2. Content Recommendations |
Machine Learning/Deep Learning | Predictive model with rigorous mathematical inference, massive parallel processing capabilities, fault tolerance, high associative capabilities, and noise filtering capabilities without requiring many assumptions. | Poor interpretability due to its black-box nature, and the integration of complex models may lead to long processing times during training. | The main focus is on finding the most accurate model, adjusting optimal parameters for continuous improvement of predictive patterns. |
Integration Model | This study proposes an optimized integration model, suitable for solving high-dimensional and complex constraint problems. It demonstrates practical feasibility for large-scale issues and dynamic problem-solving. | Appropriate model selection is crucial, and the model may face challenges in convergence. The solution obtained may be a local optimum or feasible solution, with no guarantee of being a global optimal solution. | The objective is to find an approximate solution within a limited computational time, serving as a viable decision-making approach. |
Symbol | Definition |
i | The 1st, 2nd, …………n(th) user |
j | The 1st, 2nd, …………m(th) channel |
t | The 1st, 2nd …………r(th) click |
k | The 1st, 2nd …………k(th) cluster |
AC1 | AC1 is 1 or 0, indicating if the user has or has not purchased |
AC2 | AC2 is 1 or 0, indicating if the user has or has not clicked the purchase button |
AC3 | AC3 is 1 or 0, indicating if the user has or has not clicked |
Rij | The conversion value of the ith user when purchasing on the jth channel |
CLij | The conversion value of the ith user when clicking the purchase button on the jth channel |
Sij | The conversion value of the ith user then clicking button on the jth channel |
Al | The behavioral variable of the 1st, 2nd … to the lth user |
Zik | The value of Zik is 0 or 1 and represents the ith user of cluster k |
Nk | The number of users with specific behaviors, who have also been contacted and contracted with a purchase. |
C1j | The unit cost required to complete a transaction by the ith user, converted on the jth channel |
C2j | The unit cost required for clicking the purchase button by the ith user, converted on the jth channel |
C3j | The unit cost required for clicking a button by the ith user, converted on the jth channel |
Di | The number of days the ith user is contacted by the selected channel |
di | The maximum number of days the ith user is contacted by the selected channel |
E | The current estimated expense for acquiring customer |
Xij | The value of Xij is 0 or 1, to determine if the ith user would select the jth channel. This is a decision variable. |
Y | Total target value of conversion |
Variables | Reference |
RFM | Birant (2011). |
Purchase stage | Sun et al. (2022) |
Online advertising forms | De Haan et al. (2016) |
Purchase funnel metrics | De Haan et al. (2016) |
Web behavior | Huang et al. (2007) |
Customer Attribution | He et al. (2014) |
UserID | Source/Medium | Channel | Landing Page Path | Action | Target Conversion |
1587443807754.o1ejysi7 | google/cpc | Paid Search | Product page | Click | 949 |
1587443807754.o1ejysi7 | fuboneip/banner | Display | Event page | Click the purchase button | 3985 |
1587443807754.o1ejysi7 | shop.hsbc.com.tw/referral | Referral | Event page | Purchasing | 10000 |
UserID | Sex | Recency | Frequency | Monetary | Customer Value |
1587443807754.o1ejysi7 | 1 | 43439 | 1 | 779 | 47 |
1584696454073.yx1ajfnt | 1 | 43419 | 1 | 615 | 36 |
1584684955336.odv2og9 | 1 | 43209 | 1 | 303 | 32 |
Feature | Description |
UserID | Unique User ID in the company, used to track the user across multiple sessions using Company Account |
Date | The date of the session formatted as YYYYMMDD. |
Channel | The source of referrals. For manual campaign tracking, it is the value of the utm_source campaign tracking parameter. Defined by the management of the company. |
Device Category | The category of the device: mobile, tablet, or desktop |
Browser | The raw name of the browser |
Recency | How recently a customer has made a purchase. In this research, the higher the score, the longer the interval |
Frequency | How often a customer makes a purchase |
Monetary | How much money a customer spends on purchases |
Revenue | The total sale revenue (excluding shipping and tax) of the transaction. |
Campaign Content | For manual campaign tracking, it is the value of the utm_content campaign tracking parameter. Defined by the management of the company. |
Page Content | A page on the website specified by path and/or query parameters. Use this with hostname to get the page's full URL. The display content is defined by the management of the company. |
User Type | Either New Visitor or Returning Visitor, indicating if the users are new or returning. |
Session Duration | The length of a session measured in seconds and reported in second increments. |
Sessions | The total number of sessions. |
Pages | The average number of pages viewed during a session, including repeated views of a single page. |
Action Stage | The action stage at the end of each session. This is the target feature for this use case. |
[1] | [2] | [3] | [4] | [5] | [6] | [7] | [8] | [9] | [10] | [11] | …. | [34] | |
Recency[1] | 1 | ||||||||||||
Frequency[2] | 0.04∗∗∗ | 1 | |||||||||||
Monetary[3] | 0.01 | 0.14∗∗∗ | 1 | ||||||||||
Enterprise Homepage channel[4] | 0.02. | 0.06∗∗∗ | 0.05∗∗∗ | 1 | |||||||||
External-advertising channel [5] | −0.001 | 0.00 | 0.04 | 0.26∗∗∗ | 1 | ||||||||
Organic-search channel [6] | 0.001 | 0.01 | 0.01 | 0.40 | 0.10∗∗∗ | 1 | |||||||
Keyword-advertising channel[7] | 0.011 | 0.33 | 0.03 | 0.21 | 0.25 | 0.28∗∗∗ | 1 | ||||||
Own-media channel [8] | 0.011 | 0.05 | 0.02 | 0.40 | 0.12 | 0.19 | 0.11∗∗∗ | 1 | |||||
Cross-cooperation channel [9] | −0.003 | 0.01 | 0.00 | 0.45 | 0.05 | 0.80 | 0.17 | 0.48∗∗∗ | 1 | ||||
Direct landing page channel [10] | 0.02. | 0.08 | 0.00 | 0.44 | 0.02 | 0.09 | 0.07 | 0.60 | 0.41∗∗∗ | 1 | |||
Homepage[11] | 0.03∗∗∗ | 0.14 | 0.06 | 0.69 | 0.32 | 0.63 | 0.40 | 0.53 | 0.65 | 0.50∗∗∗ | 1 | ||
Product Information[12] | 0.02.. | 0.09 | 0.04 | 0.72 | 0.44 | 0.53 | 0.33 | 0.62 | 0.62 | 0.42 | 0.83∗∗∗ | 1 | |
Campaign Information[13] | 0.008 | 0.02 | 0.06 | 0.67 | 0.48 | 0.60 | 0.36 | 0.54 | 0.57 | 0.20 | 0.73 | 0.84 | 1 |
Situational Information[14] | 0.015 | 0.05 | 0.02 | 0.54 | 0.19 | 0.59 | 0.20 | 0.70 | 0.78 | 0.56 | 0.69 | 0.73 | 0.61 |
Member Services Information [15] | 0.02∗. | 0.08 | 0.03 | 0.74 | 0.37 | 0.57 | 0.35 | 0.50 | 0.62 | 0.50 | 0.77 | 0.83 | 0.67 |
Pages[16] | 0.02. | 0.07 | 0.05 | 0.75 | 0.45 | 0.63 | 0.37 | 0.64 | 0.69 | 0.42 | 0.86 | 0.97 | 0.92 |
Sessions[17] | 0.01 | 0.06 | 0.03 | 0.75 | 0.20 | 0.77 | 0.29 | 0.62 | 0.90 | 0.54 | 0.83 | 0.81 | 0.76 |
Session Duration[18] | 0.02 | 0.15 | 0.07 | 0.56 | 0.21 | 0.75 | 0.35 | 0.33 | 0.76 | 0.36 | 0.74 | 0.67 | 0.63 |
NewVisitor [19] | 0.02 | 0.22 | 0.00 | 0.31 | 0.27 | 0.59 | 0.49 | 0.08 | 0.56 | 0.22 | 0.58 | 0.54 | 0.44 |
Returning Visitor[20] | 0.04 | 0.25 | 0.09 | 0.68 | 0.31 | 0.60 | 0.47 | 0.51 | 0.63 | 0.49 | 0.87 | 0.82 | 0.70 |
Desktop [21] | 0.04 | 0.12 | 0.04 | 0.69 | 0.28 | 0.62 | 0.41 | 0.53 | 0.66 | 0.49 | 0.86 | 0.82 | 0.71 |
Mobile [22] | 0.03 | 0.24 | 0.05 | 0.50 | 0.20 | 0.53 | 0.45 | 0.40 | 0.67 | 0.54 | 0.69 | 0.63 | 0.49 |
Tablet [23] | 0.02 | 0.02 | 0.00 | 0.24 | 0.64 | 0.02 | 0.24 | 0.00 | 0.00 | 0.00 | 0.17 | 0.31 | 0.37 |
Android Webview[24] | −0.01 | 0.04 | 0.04 | −0.01 | 0.01 | −0.01 | 0.05 | 0.00 | 0.00 | 0.02 | 0.01 | 0.01 | 0.00 |
Opera[25] | 0.01 | 0.03 | 0.00 | 0.00 | 0.01 | 0.00 | 0.02 | 0.00 | 0.00 | 0.02 | 0.01 | 0.01 | 0.00 |
Samsung Internet[26] | 0.01 | 0.04 | 0.00 | 0.20 | 0.00 | 0.03 | 0.13 | 0.00 | 0.01 | 0.23 | 0.17 | 0.07 | 0.00 |
Chorme[27] | 0.04 | 0.12 | 0.04 | 0.69 | 0.28 | 0.62 | 0.41 | 0.53 | 0.66 | 0.49 | 0.86 | 0.82 | 0.71 |
Safari[28] | 0.02 | 0.19 | 0.04 | 0.48 | 0.17 | 0.55 | 0.33 | 0.42 | 0.71 | 0.52 | 0.64 | 0.63 | 0.49 |
Edge[29] | −0.01 | 0.02 | 0.03 | 0.00 | 0.31 | 0.00 | 0.03 | 0.00 | 0.00 | 0.01 | 0.07 | 0.05 | 0.04 |
Firefox[30] | 0.01 | 0.03 | 0.01 | 0.26 | 0.67 | 0.02 | 0.25 | 0.00 | 0.00 | 0.00 | 0.18 | 0.33 | 0.39 |
Internet Explorer[31] | 0.02 | 0.05 | 0.07 | 0.67 | 0.00 | 0.07 | 0.01 | 0.58 | 0.32 | 0.54 | 0.44 | 0.44 | 0.35 |
Clicking[32] | −0.11 | −0.17 | −0.01 | 0.19 | 0.14 | 0.60 | 0.19 | 0.04 | 0.63 | 0.03 | 0.40 | 0.27 | 0.36 |
Clicking "buy now"[33] | 0.10 | 0.00 | 0.06 | 0.70 | 0.29 | 0.33 | 0.29 | 0.60 | 0.36 | 0.56 | 0.74 | 0.80 | 0.62 |
Purchasing[34] | 0.03 | 0.86 | 0.09 | 0.06 | −0.01 | 0.01 | 0.34 | 0.04 | 0.01 | 0.08 | 0.14 | 0.09 | 0.02 |
Cluster 1 | Cluster 2 | Cluster 3 | Cluster 4 | Cluster 5 | Cluster 6 | Cluster 7 | |
Recency | 33.28 | 1.31 | 52.01 | 27.76 | 32.87 | 40.14 | 24.25 |
Frequency | 0.64 | 0.70 | 1.27 | 0.83 | 0.72 | 0.87 | 0.94 |
Monetary | 947.36 | 2532.60 | 3158.19 | 6799.02 | 9334.24 | 1777.86 | 4946.58 |
Enterprise homepage channel | 1.08 | 1.55 | 3.49 | 3.09 | 3.19 | 1.09 | 1.27 |
External-advertising channel | 0.00 | 0.18 | 0.13 | 0.15 | 0.27 | 0.01 | 0.02 |
Organic-search channel | 1.48 | 1.54 | 2.48 | 2.60 | 2.87 | 1.32 | 1.41 |
Keyword-advertising channel | 4.61 | 4.61 | 8.14 | 5.78 | 2.99 | 5.18 | 5.93 |
Own-media channel | 0.25 | 0.67 | 1.19 | 0.76 | 0.74 | 0.58 | 1.26 |
Cross-cooperation channel | 0.20 | 0.28 | 1.52 | 1.69 | 0.26 | 0.09 | 0.23 |
Direct landing page | 1.14 | 1.20 | 2.52 | 0.73 | 1.34 | 1.78 | 1.52 |
Homepage | 0.22 | 0.04 | 0.41 | 0.22 | 0.24 | 0.21 | 0.14 |
Product information | 0.76 | 0.17 | 1.22 | 0.96 | 0.81 | 0.92 | 1.03 |
Campaign information | 0.01 | 0.01 | 0.16 | 0.14 | 0.12 | 0.03 | 0.06 |
Situational Information | 0.00 | 0.00 | 0.07 | 0.03 | 0.01 | 0.02 | 0.03 |
Member Services information | 0.05 | 0.04 | 0.41 | 0.26 | 0.17 | 0.13 | 0.20 |
Pages | 1.04 | 0.26 | 2.27 | 1.61 | 1.36 | 1.32 | 1.48 |
Sessions | 1.05 | 1.01 | 2.33 | 1.73 | 1.42 | 1.27 | 1.38 |
Session Duration | 964 | 954 | 761 | 817 | 867 | 825 | 795 |
Models | F-score | Accuracy | Cohen's |
LSTM | 0.7915 | 0.7987 | 0.5830 |
ANN | 0.7405 | 0.7531 | 0.4802 |
RFs | 0.7694 | 0.7771 | 0.5387 |
Models | F-score | Accuracy | Cohen's |
CNN-LSTM | 0.5618 | 0.5737 | 0.2232 |
LSTM | 0.5374 | 0.5504 | 0.1979 |
ANN | 0.4075 | 0.5019 | 0.1112 |
RFs | 0.5007 | 0.5265 | 0.1371 |
Planning method | Channel No. | 1 | 2 | 3 | 4 | 5 | 6 | 7 | Total | Cost | Revenue(R) |
Manual planning | (Zi5) | 9 | 2 | 5 | 2 | 5 | 1 | 1 | 25 | 9830 | 17418 |
(Zi3) | 7 | 0 | 0 | 12 | 6 | 0 | 0 | 25 | |||
(Zi4) | 15 | 0 | 3 | 6 | 0 | 1 | 0 | 25 | |||
(Zi2) | 17 | 0 | 3 | 3 | 1 | 0 | 1 | 25 | |||
Total | 48 | 2 | 11 | 23 | 12 | 2 | 2 | 100 | |||
Goal 1 IP-model planning | (Zi5) | 5 | 0 | 1 | 1 | 4 | 0 | 14 | 25 | 4110 | 26778 |
(Zi3) | 4 | 0 | 1 | 0 | 1 | 3 | 16 | 25 | |||
(Zi4) | 0 | 1 | 1 | 0 | 9 | 2 | 12 | 25 | |||
(Zi2) | 0 | 3 | 0 | 0 | 1 | 6 | 15 | 25 | |||
Total | 9 | 4 | 3 | 1 | 15 | 11 | 57 | 100 | |||
Planning method | Channel No. | 1 | 2 | 3 | 4 | 5 | 6 | 7 | Total | Cost | Value(Y) |
Goal 2 IP-model planning | (Zi5) | 5 | 0 | 1 | 1 | 4 | 0 | 14 | 25 | 7408 | 35559 |
(Zi3) | 6 | 0 | 0 | 0 | 1 | 3 | 15 | 25 | |||
(Zi4) | 2 | 10 | 0 | 0 | 1 | 0 | 12 | 25 | |||
(Zi2) | 0 | 3 | 0 | 0 | 1 | 6 | 15 | 25 | |||
Total | 13 | 13 | 1 | 1 | 7 | 9 | 56 | 100 |
Enterprise online channel/Target customer cluster | This channel is the main customer touch point in the target market | This channel is not the main customer touch point in the target market |
The online channels of the enterprise result in higher conversion values for purchases among the targeted cluster customers | Continuing to optimize the channel planning while exercising caution against over-reliance on online channels to prevent customer fatigue at this touchpoint and potential challenges such as fierce competition in advertising bids. | Conduct a cost assessment to determine the feasibility of increasing channel resources for customer engagement. This channel could serve as a valuable avenue for interacting with customers and might represent a blue ocean market in the competitive landscape of advertising bids. |
The online channels of the enterprise result in lower conversion values for purchases among the targeted cluster customers | Adjust and optimize the content and service experience of the channel to assess if there is a potential for improving the channel conversion rate. | Realign the target customer clusters and channel selection, continuously adjusting communication content and service experience design. |