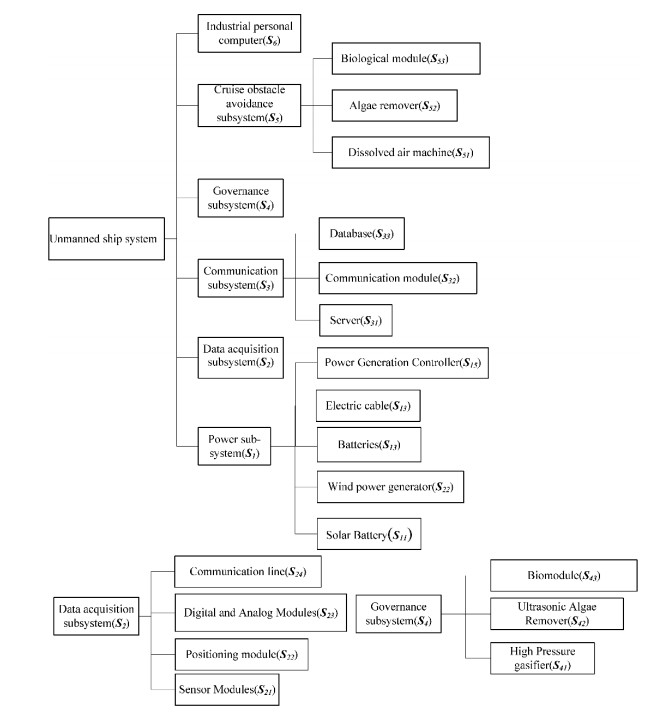
With the development of unmanned ships, their use in production is becoming more and more common. However, the unmanned ship work cycle is long and the work environment is complex, and it is still very difficult to calculate the phased mission reliability without unmanned ship. We analyze the unmanned ship phased mission reliability based on the binary decision diagram. Moreover, redundancy is used as the unmanned ship reliability optimization scheme. Considering the resource limitation, and the capacity of unmanned ship, the redundancy allocation scheme of unmanned ship is established. The redundancy allocation scheme is solved by marginal optimization algorithm. Finally, a case study is established to analyze the effectiveness and practicality of the proposed method.
Citation: Xu Zhan, Yang Yong, Wang Xiao. Phased mission reliability analysis of unmanned ship systems[J]. Electronic Research Archive, 2023, 31(10): 6425-6444. doi: 10.3934/era.2023325
[1] | Ran Yan, Ying Yang, Yuquan Du . Stochastic optimization model for ship inspection planning under uncertainty in maritime transportation. Electronic Research Archive, 2023, 31(1): 103-122. doi: 10.3934/era.2023006 |
[2] | Yiwei Wu, Yadan Huang, H Wang, Lu Zhen . Nonlinear programming for fleet deployment, voyage planning and speed optimization in sustainable liner shipping. Electronic Research Archive, 2023, 31(1): 147-168. doi: 10.3934/era.2023008 |
[3] | Xu Xin, Xiaoli Wang, Tao Zhang, Haichao Chen, Qian Guo, Shaorui Zhou . Liner alliance shipping network design model with shippers' choice inertia and empty container relocation. Electronic Research Archive, 2023, 31(9): 5509-5540. doi: 10.3934/era.2023280 |
[4] | Liling Huang, Yong Tan, Jinzhu Ye, Xu Guan . Coordinated location-allocation of cruise ship emergency supplies under public health emergencies. Electronic Research Archive, 2023, 31(4): 1804-1821. doi: 10.3934/era.2023093 |
[5] | Shuaian Wang . Shipping Information Modeling (SIM): bridging the gap between academia and industry. Electronic Research Archive, 2022, 30(10): 3632-3634. doi: 10.3934/era.2022185 |
[6] | Simon Tian, Xinyi Zhu . Data analytics in transport: Does Simpson's paradox exist in rule of ship selection for port state control?. Electronic Research Archive, 2023, 31(1): 251-272. doi: 10.3934/era.2023013 |
[7] | Hongyan Dui, Yong Yang, Xiao Wang . Reliability analysis and recovery measure of an urban water network. Electronic Research Archive, 2023, 31(11): 6725-6745. doi: 10.3934/era.2023339 |
[8] | Nishui Cai, Guofeng He . Multi-cloud resource scheduling intelligent system with endogenous security. Electronic Research Archive, 2024, 32(2): 1380-1405. doi: 10.3934/era.2024064 |
[9] | Hongyan Dui, Huiting Xu, Haohao Zhou . Reliability analysis and resilience measure of complex systems in shock events. Electronic Research Archive, 2023, 31(11): 6657-6672. doi: 10.3934/era.2023336 |
[10] | Ran Yan, Shuaian Wang . Ship detention prediction using anomaly detection in port state control: model and explanation. Electronic Research Archive, 2022, 30(10): 3679-3691. doi: 10.3934/era.2022188 |
With the development of unmanned ships, their use in production is becoming more and more common. However, the unmanned ship work cycle is long and the work environment is complex, and it is still very difficult to calculate the phased mission reliability without unmanned ship. We analyze the unmanned ship phased mission reliability based on the binary decision diagram. Moreover, redundancy is used as the unmanned ship reliability optimization scheme. Considering the resource limitation, and the capacity of unmanned ship, the redundancy allocation scheme of unmanned ship is established. The redundancy allocation scheme is solved by marginal optimization algorithm. Finally, a case study is established to analyze the effectiveness and practicality of the proposed method.
With the development of unmanned equipment, more and more unmanned equipment is put into daily life. What is studied in this paper is an environmental protection type unmanned ship. The unmanned ship can collect data, environmental monitoring and environmental management of the ocean and river. Since the work of the unmanned ship is divided into several stages, there are many components involved in each stage of the work, and the marine environment is complex. Such factors have an important impact on the completion of the work of the unmanned ship. Therefore, a reasonable analysis of the mission reliability of the unmanned ship is the key to assessing the working ability of the unmanned ship. The unmanned ship has a long mission cycle, and the staff cannot maintain it during the execution of the mission. Therefore, improving the mission reliability of the unmanned ship is the key to guaranteeing the unmanned ship's successful completion of the mission. Under the limitation of resources, it is crucial to determine the optimal reliability optimization scheme for unmanned ships.
The reliability of staged mission systems has been studied by many scholars. Liu et al. [1] organized the past studies on unmanned ship failures and provided the idea of unmanned ship reliability analysis. Gao et al. [2] established a dynamic fault tree model of unmanned ship. The system mission reliability was solved by dynamic Bayesian network. Li et al. [3] proposed the idea of modularization to simplify the calculation of system mission reliability. Xing et al. [4] proposed a method to analyze the reliability by transforming the DFT model of a system into a graph of binary decisions. It simplifies the computation of complex system reliability. Xing and Levitin [5] proposed a method based on binary decision diagrams for solving the task reliability of a system under the influence of faults. Tang et al. [6] proposed a method for solving the phased task reliability of a system under multiple failure modes using phase-equivalent binary decision diagrams. Wang et al. [7] proposed a method to analyze the phase dependency problem using decision tree and solve the system reliability by Bayesian network. Wu et al. [8] proposed a method to calculate the system reliability in the presence of phase conflict. Li et al. [9] proposed a method to compute the mission reliability of a system in the presence of cold spares using a generalized generating function. Cheng et al. [10] proposed a method to analyze the reliability of a system considering k-out-of-n (G) under multiple failure modes. Li et al. [11] proposed a mission reliability assessment model considering system backup and redundancy. Wang et al. [12] proposed a method for assessing the mission reliability of a system considering phase combining as well as component redundancy. It provides reliability analysis ideas for analyzing systems with redundancy. Zhou et al. [13] propose a minimum path method to assess the task reliability of the system. Xu et al. [14] propose a modular approach to chunk the system and design for redundancy. Liu et al. [15] propose a method to analyze the reliability of a system considering the arrival time of external shocks. Anwa et al. [16] proposed a data-driven approach to analyze the task reliability of a system.
In the study of reliability optimization, Liu et al. [17] proposed a system reliability optimization scheme under cost constraints as well as reliability importance as an indicator. Zhang et al. [18] proposed a method to transform a complex system into a graph and use an algorithm of factorization theorem to solve the reliability redundancy of the system. Li et al. [19] proposed a method based on particle swarm algorithm to optimize the reliability of the system. Xu et al. [20] proposed a redundancy design approach to optimize the reliability of the system to improve the reliability of the system. Li et al. [21] proposed a hybrid redundancy approach for reliability optimization. It is optimized for systems with backup tasks and rescue tasks. Yeh et al. [22] proposed a novel generalized algorithm to improve the redundancy-based system reliability optimization scheme. Nourelfath et al. [23] proposed a redundancy design scheme with system availability as an indicator. Zhang et al. [24] proposed a reliability optimization design scheme considering the degradation of spare parts performance. Wang et al [25] proposed a system reliability optimization method for periodic maintenance of redundant components considering the performance degradation of spare components. Atashgar and Abdollahzadeh [26] proposed a redundancy-based reliability optimization method with cost and loss as objective values. Golmohammadi and Ardakan [27] proposed a hybrid strategy considering redundancy as well as preventive maintenance for optimizing system reliability. Feng et al. [28] proposed a system redundancy design method of importance combined with a marginal optimization algorithm to optimize the system reliability. Dui et al. [29] proposed a system reliability optimization design method considering the conversion time of spare parts. Yan et al. [30] propose a stochastic optimization model for optimal design of system reliability. Chang et al. [31] proposed a system reliability optimization scheme considering cost and time. Ren et al. [32] proposed an improved particle swarm algorithm for reliability optimization of staged task systems.
Then, the current research does not examine the unmanned ship phased mission system in detail. Unmanned ships have numerous components, some of which are involved in multiple systems. These have not been mentioned in previous studies. In addition, information errors can have an impact on unmanned ship missions. Considering the impact of information errors on the reliability of unmanned ship missions, we analyze the unmanned ship phased mission system in detail. The divide-and-conquer idea is used to categorize the unmanned ship mission reliability under information error. Then, the reliability of unmanned ship staged mission system is optimized based on marginal optimization algorithm under the limitation of resources.
The remainder of this paper is structured as follows. In Section 2, the failure causes of the unmanned ship staged mission system are analyzed. Section 3, the unmanned ship staged mission reliability is analyzed using BDD. Section 4, optimizes the unmanned ship reliability based on the marginal optimization algorithm. Section 5, a case study is built to verify the practicality of the method. Section 6, summarizes the paper.
The unmanned ship chosen to be analyzed in this paper is some kind of environmentally friendly unmanned ship. The unmanned ship carries a variety of sensors can monitor the data in the river, the ocean. Through the communication equipment carried by the hull of the data will be transmitted to the shore in real time for analysis. The unmanned ship not only has the ability of environmental monitoring but also has the ability of environmental management. The water quality treatment device can realize the function of environmental management ability.
The unmanned ship can be divided into five subsystems. They are power subsystem, data acquisition subsystem, communication subsystem, governance subsystem and cruise obstacle avoidance subsystem. The specific composition of the subsystems is shown in Figure 1.The working process of an unmanned ship is shown as follows.
(ⅰ) The power subsystem provides kinetic energy for the unmanned ship to ensure that other subsystems in the unmanned ship complete their work successfully. Before the unmanned ship starts working, the power subsystem is turned on to provide energy for the unmanned ship. After the mission starts, the cruise obstacle avoidance subsystem starts to work. In the process of unmanned ship traveling, due to the complexity of the water situation, there may be large floating objects, or seaweed and touching the reef and so on. The unmanned ship should have certain obstacle avoidance ability. Water quality monitoring and management cycle is long, the unmanned ship should be in accordance with the fixed trajectory of the operation. Therefore, the unmanned ship needs to have the ability to cruise. Therefore, after the unmanned ship task starts, the cruise obstacle avoidance sub-system needs to start working.
(ⅱ) After the start of the task, the shore staff communicates information with the unmanned ship through the base station carried by the ship, and then controls the ship. After the ship arrives at the designated area, it carries out water quality management or monitoring of the target sea area through the management subsystem.
(ⅲ) After the completion of the current water quality management, the power subsystem works, and through the communication subsystem, the shore staff controls the unmanned ship and completes the return voyage of the unmanned ship. In the return process, the cruise obstacle avoidance system plays an important role to protect the unmanned ship navigation safety.
The specific composition of the unmanned ship system subsystem is shown below.
1) The power subsystem (S1) has five parts, namely, the three power sources of solar cell (S11), wind generator (S12) and storage battery (S13) as well as the cable (S14) and power generation controller (S15). Among them, the storage battery is a supplement to the two power generation methods of solar energy and wind power.
2) The data acquisition subsystem (S2) consists of a sensor module (S21), a positioning module (S22), a digital and analog module (S23), a communication cable (S24) and an industrial controller.
3) The communication subsystem (S3) is composed of an onshore server (S31), a communication module (S32) and a database (S33). Among them, the onshore server is responsible for establishing the communication link with the unmanned ship.
4) The governance subsystem (S4) has a high-pressure dissolving gas machine (S41), an ultrasonic algae remover (S42) and a biological module (S43). Among them, the industrial control computer is deployed in the governance system for the operation of the water quality treatment device.
5) The cruise obstacle avoidance sub-system (S5) is composed of a sensor module (S51), a positioning system (S52), an industrial control machine and a paddle machine (S53). In this system, the sensor module, the localization system and the industrial control machine are all designed to regulate and control the operation of the paddle machine.
In each of the above systems, the industrial control machine serves the role of data transmission, data collection and storage and gives commands. The convenience of analysis should be the industrial control machine (ICM) as a separate independent system, does not belong to the above systems, then define the industrial control machine as S6.
In the process of the unmanned ship's mission, each stage needs to call the system. In this section, the reliability of each stage is analyzed separately to get the FT of the stage. Before analyzing the reliability of the unmanned ship stage mission, it is necessary to analyze the failure of each subsystem. By analyzing the faults of each subsystem, the subsystems can be regarded as a module and the state explosion problem can be solved. In Figure 2 we analyze the failure of each subsystem and establish the FT model of the subsystem.
Subsystem S2 is mainly responsible for collecting information about the target sea area and transmitting it back to S6 for data analysis. When S21 and S23 fail, it will lead to wrong information collected or no information collected. The failure will trigger the failure of the industrial control machine, which will lead to the failure of the industrial control machine to make decisions, which in turn will cause the failure of the whole mission. The failure principle of the FDEP in subsystem S5 is the same as in S2.
In the first stage, the power subsystem (S1) and the cruise obstacle avoidance subsystem (S5) need to be involved in the work to guarantee that the unmanned ship navigates according to a reasonable trajectory and avoids obstacles.
In the second stage, the data acquisition stage. It is necessary for the unmanned ship to arrive at the designated stage and call the sensor system to collect the water information of the target water area. The onshore server accesses the communication subsystem to get the water quality data.
In the third phase, the governance phase. The unmanned ship, powered by the power subsystem, establishes contact with the shore server through the communication subsystem. The shore staff gives instructions to the unmanned ship, and the instruction information is sent from the industrial control machine to the governance subsystem to complete the water quality governance work.
In the fourth stage, the return stage. Same as the ferrying stage, the power subsystem and the cruise obstacle avoidance subsystem work at the same time and return smoothly.
The components that participate in the various phases of the unmanned ship mission are shown in Figure 3.
The unmanned ship is susceptible to extreme natural weather, such as thunder and lightning, wind and waves, etc., during its mission. With high humidity in the ocean, the components of the unmanned ship are susceptible to corrosion and accelerated aging. Therefore, the components of the unmanned ship are easy to be affected by the natural environment and fail during the execution of the mission. However, the failure of some components will have a bad impact on the execution of the whole task, forming a large area paralyzed by the components. For example, the industrial control machine in the unmanned ship is to give commands to other components inside the ship, or to carry out information feedback. If the ICM delivers wrong instructions, it may cause the mission of the unmanned ship to fail or even be damaged. There are two aspects to the transmission of incorrect commands by the ICM. On the one hand, it is due to the destruction of the ICM itself, which is unable to establish a connection with other components, or the transmission of information is incomplete. On the other hand, it is due to the fact that the other components pass the wrong information.
In this paper, we propose a reliability analysis method for phased tasks of unmanned ships, which consists of five steps.
Step 1: Analyze the cause of information error. First, there are two types of sources of error information analyzed or transmitted for the ICM, which have been briefly explained above. For the second source of error information, in the S2 subsystem it is due to the failure of S21 and S23 themselves, resulting in the formation of erroneous information in the process of information acquisition and information analysis. However, for the error messages analyzed or delivered by the ICM, it does not necessarily lead to the failure of the mission of the unmanned ship. For example, in the S2 subsystem due to S21 and S23 collecting the wrong information, but because the target area, i.e., the information of the area to be governed, has been detected several times. Then, the information collected by S21 and S23, which merely serves as a supplement to the information from previous detections, will not have a practical impact on the mission.
Step 2: For the effect of uncertain information error probability on unmanned ship mission reliability mentioned above, SEA algorithm is adopted to analyze unmanned ship PM reliability.
R(t)=RE(t)⋅E(t)+RU(t)⋅U(t) | (1) |
In Eq (1), R(t) denotes the reliability of the unmanned ship PM. RE(t) denotes the reliability of the unmanned ship under the condition that an information error occurs. e(t) denotes the probability that an information error occurs. Conversely, RU(t) denotes the reliability of the unmanned ship under the condition that no information error occurs. u(t) denotes the probability that the information error does not occur.
In the above, this paper mentions that due to the component under the trigger event, it is information gathering for the target sea area. So when the triggering event is generated, it does not necessarily lead to error message delivery. Based on the above, for the PMS reliability calculation of unmanned ships, it needs to be divided into three cases. That is, error information is generated but does not affect, error information is generated and delivered and no error information is generated.
Case 1: Error information is generated but does not affect system safety. In the Unmanned Ship FT model can be understood as the failure of the trigger component of the I & C, which collects and delivers an error message or does not collect and deliver the message, but the event does not affect the decision of the I & C. In this case the reliability of the unmanned ship system is Eq (2).
R1(t)=REU(t)⋅EU(t)⋅E(t) | (2) |
In Eq (2) R1(t) denotes the system reliability in Case 1. EU(t)denotes the probability that the error message is generated but does not affect the system safety.
Case 2: Error messages are generated and affect the system security. For this case, it can be understood that the target sea area in which the unmanned ship is traveling is an unfamiliar area, and there is no information about the area in the I & C. Therefore, the information delivered by the triggering event is critical information, and in this case, the reliability of the unmanned ship is 0. That is, the error message will lead to the failure of the unmanned ship mission.
Case 3: The error message is not generated, i.e., the component under the triggering event has not failed. Then in this case the reliability of the unmanned ship system is Eq (3).
R3(t)=RU(t)⋅U(t) | (3) |
Step 3: System reliability analysis without considering information errors. Without considering information errors, only the reliability of the system under each stage is analyzed. First, the DFT of the unmanned ship system needs to be transformed into a BDD model.
In this paper, the DFT model of unmanned ship is plotted. In order to facilitate the calculation of the reliability of the unmanned ship, the FT model is transformed into a BDD model.
In Figure 4, Sji indicates that the S_i system is involved in the unmanned ship mission at stage j. The figure represents the BDD of the unmanned ship at each stage. The figure represents the BDD of the unmanned ship at each stage. throughout the mission, there is no indication of which subsystems are involved as the mission progresses. It is clear from Figure 5 that the involvement of the subsystems changes as the mission progresses. Therefore, the disjoint paths to system success under each phase, if shown in Eq (4).
{ϕ1=S11S15ϕ2=S21S22S23S15ϕ3=S31S22S23S34S15ϕ4=S41S22S23S34S45 | (4) |
∅i denotes the disjointed successful routes in phase i. Success of the task in phase i+1 presupposes success of the task in phase i. Subsystems involved in the task in both phases i and i+1 need to ensure that the working state remains normal in phase i + 1. Components that are only involved in phase i only need to ensure that their working state is normal within phase i. Components within the subsystem do not work when they are not involved in the current phase of the task. The time of each stage during the execution of the task by the unmanned ship is T=[T1,T2,T3,T4], and the total period of the task is the time T. The reliability of the system, without considering the information error, is Eq (5).
R(t)={RS1(t)RS5(t)0≤t≤T1RS1(t)RS2(t−T1)RS3(t−T1)RS5(T1)T1≤t≤T1+T2RS1(t)RS2(T2)RS3(T2)RS4[t−(T1+T2)]RS5(T1)T1+T2≤t≤T−T4RS1(t)RS2(T2)RS3(t−T1)RS4(T2+T3)RS5[T1+(t−T+T4)]T−T4≤t≤T | (5) |
Step 4: Through the analysis above, the calculation of system reliability in this paper is divided into two cases, i.e., only Cases 1 and 3 are considered. the method of calculating the reliability of each stage of the system in terms of modularization has been analyzed in Step 3, and the reliability of each subsystem will be analyzed in this step.
Case 1: Under the condition of occurrence of information error, it specifically affects S2 subsystem and S5 subsystem. In this case, although an information error occurs, i.e., the trigger event fails, it does not cause the task to fail. That is, the triggering event had no effect on the execution of the task. Therefore, in the reliability analysis of S2 and S5, the triggering event should be canceled from the DFT and S6 should be merged into the OR gate with other events. To facilitate the calculation, the fault trees of the S2 and S5 systems are transformed into a BDD structure, as shown in Figure 5.
According to the BDD shown, there is one and only one path for the system to succeed, and then the S2 and S5 system reliability will be follows.
R1S2(t)=RS6(t)RS22(t)RS24(t) | (6) |
R1S5(t)=RS6(t)RS52(t)RS53(t) | (7) |
Case 3: No information error has occurred. In this case, the reliability calculation of the S2 subsystem and the S5 subsystem needs to consider the triggering event. That is, the FDEP gates are converted to OR gates and incorporated into the DFT. The fault tree in the DFT obtained after the transformation in Case 3 is the subsystem DFT model under the condition that no information error occurs. Same as above, the DFT of subsystem S2 with subsystem S5 is converted to BDD, where the FDEP gates are converted to OR gates as shown in Figure 6.
According to the BDD shown in Case 3, the reliability of S2 subsystem and S5 subsystem is shown in Eqs (8) and (9).
R3S2(t)=RS6(t)RS21(t)RS22(t)RS23(t)RS24(t) | (8) |
R3S5(t)=RS6(t)RS51(t)RS52(t)RS53(t) | (9) |
In Cases 1 and 3, we discuss the reliability of two subsystems under information errors, but the other subsystems are not analyzed. The other subsystems are not affected by information errors. Then, for the other subsystems, the reliability does not change regardless of the case. As above, the DFT of each other subsystem is transformed into BDD as shown in Figures 7 and 8.
Figure 7 shows the BDD diagram of S1 subsystem and S3 subsystem. Based on the above analysis easy to obtain the reliability of the unmanned ship S1 and S3 subsystems are follows.
RS1=[1−(1−RS11(t))(1−RS12(t))(1−RS13(t))]R14(t)R15(t) | (10) |
RS3(t)=RS31(t)RS32(t)RS33(t) | (11) |
In the S4 subsystem, component S6 is a triggering event in the FDEP gate, and the error message delivered by S6 triggers other components to work incorrectly. In the case described above, the error message delivered by S6 has been analyzed in Case 1. Therefore, in the reliability analysis of the S4 subsystem, only the mechanical failure of S6 is considered. In the same way as analyzed above, this FDEP gate is converted to an OR gate and a BDD model is built as shown in Figure 8.
According to the BDD model, the reliability of the S4 subsystem is calculated as Eq (12).
RS4(t)=RS6(t)RS41(t)RS42(t)RS43(t) | (12) |
Step 5: Comprehensive analysis of system reliability In Steps 3 and 4, we analyze the system reliability under modularization and the reliability of each subsystem respectively. For the system reliability of each stage, the case of information error needs to be considered. Therefore, the system reliability under the action of both Cases 1 and 3 should be considered together. Due to the existence of the above cases, the subsystems S2 and S5 are now specifically analyzed. Taking S2 as an example, S21 and S22 will trigger information errors, and the reliability of S2 is analyzed by combining Eqs (2) and (3), as shown in Eq (13).
RS2(t)=R1S2(T2)⋅ES21(t)ES23(t)⋅EUS21(t)EUS23(t)+R3S2(t) | (13) |
ESij represents the probability of component propagating error information, i.e., the failure probability of the component. EUSij represents the conditional probability under the condition that the component fails but has no effect on the system safety. In Eq (13), since no error message propagation occurs, R3Sij in Case 3 is the system reliability under the condition of no error message. Therefore R3(t) is calculated without considering the probability U(t) that no error message has occurred, and Eq (13) can be obtained from the full probability Equation.
Similarly, the reliability of subsystem S5, as shown in Eq (14).
RS5(t)=R1S5(T2)⋅ES51(t)⋅EUS51(t)+R3S5(t) | (14) |
The other subsystems, are not affected by information errors, so the calculation of unmanned ship reliability can be derived by combining Eq (14).
In the process of accomplishing the phased mission, the unmanned ship has a long sailing time and a long sailing area. If the unmanned ship component fails, the shore staff can't repair it in time, which will lead to the failure of the unmanned ship mission. The marine environment is complex and changeable, extreme weather is frequent and the humid environment will aggravate the degradation of unmanned ship components. In response to the above situation, preventive maintenance should be carried out on the unmanned ship system before the unmanned ship carries out its mission. In order to improve the ability of unmanned ships to resist natural disasters. In this paper, a redundancy-based unmanned ship system reliability optimization scheme is proposed.
The redundant components of an unmanned ship component are cold storage before the component fails, i.e., the storage phase does not affect the life of the redundant components. Because the relationship between the redundant component and the component should be cold storage gate, according to the conversion relationship of cold storage gate, the reliability of the component after adding redundancy is Eq (15).
RReSij=1−(1−RSij)(1−RSij)m | (15) |
In Eq (15) m is the number of redundant components. The increase in redundancy of components increases the reliability of the system consisting of the component and its redundant components. For the system, the reliability is also increased. Therefore, the method of adding redundant components is practical. When the component fails, the redundant component continues to work. However, with limited resources, it is necessary to maximize the system reliability and minimize the cost as much as possible. At the same time, the number of redundant components for a given component is limited by the space available in the cabin of the unmanned ship. For the whole system, the total number of components should be increased within a certain range, not exceeding the maximum carrying capacity of the unmanned ship.
Based on the above analysis, the determination of the optimal redundant components is an integer programming problem. As shown in Eq (16).
maxR(t){∑ni=1vij∗Q(Sij)≤V)Q(Sij)≤DSijQ(Sij)≥0Q(Sij)∈Z | (16) |
where n is the number of components within the unmanned ship system. vij is the cost of the redundant components of component Sij. Q(Sij) is the number of redundant components of the unmanned ship component Sij. DSij is the maximum number of redundant components that the component Sij can so afford. This variable is limited by the internal space and load capacity of the unmanned ship.
The marginal optimization algorithm is proposed to solve this optimization problem. The marginal optimization algorithm has the characteristics of fast solution speed and good convergence effect. The marginal cost for a component at moment t has Eq (17).
CERSij=R(S11,...,Sij+1,...,S53)−R(S11,...,Sij,...,S53)Vfuture−Vnow | (17) |
Equation (17) represents the ratio of the increase in the overall reliability of the system with the addition of a redundant component to the increase in the cost of the component. Vfuture cannot exceed the constraint of the total cost. Vnow denotes the cost of the system at the moment t before the redundant component is added to the system.
The above marginal cost gives the ratio of the increase in reliability to the cost when adding a component. When this value is larger, it is more favorable for component Sij to add a redundant component. Solving the above optimization problem, the optimal redundancy scheme can be determined using Eq (18), and incorporating the relevant constraints.
In this section, the reliability analysis of the unmanned ship will be performed and optimized based on the model presented in this paper. The system components of an unmanned ship have been analyzed in Section 2.1. An unmanned ship consists of 6 subsystems with 19 components.
In this section, it is assumed that the failure probability of the unmanned ship components unmanned ship components obeys a Weibull distribution, W(t,θ,γ). Therefore, the reliability of each component is R(t)=exp[−(tθ)γ−1] and the failure rate is λ(t)=γθ(tθ)γ−2. The parameters of each component of the unmanned ship and are shown in Table 1.
Serial number | Coding | θ | γ | Serial number | Coding | θ | γ |
1 | S11 | 3247 | 1.65 | 11 | S32 | 2199 | 1.75 |
2 | S12 | 2574 | 1.76 | 12 | S33 | 2199 | 1.75 |
3 | S13 | 2416 | 2.21 | 13 | S41 | 1478 | 1.78 |
4 | S14 | 2150 | 1.96 | 14 | S42 | 1478 | 1.78 |
5 | S15 | 3589 | 2.14 | 15 | S43 | 3247 | 1.65 |
6 | S21 | 3589 | 2.14 | 16 | S51 | 2574 | 1.76 |
7 | S22 | 3165 | 1.54 | 17 | S52 | 2416 | 2.21 |
8 | S23 | 3165 | 1.54 | 18 | S53 | 2150 | 1.96 |
9 | S24 | 2550 | 1.48 | 19 | S6 | 3589 | 2.14 |
10 | S31 | 2550 | 1.48 | \ | \ | \ | \ |
The reliability calculation of the system in Case 3 does not need to consider the effect of information errors. It can be calculated directly according to BDD. The conditional probability of information error needs to be calculated under Case 1, and the specific subsystems to be analyzed are S2 and S5. The information error is due to the failure of the relevant component. In this case, the conditional probability that the system did not fail follows an exponential distribution with a failure rate of λ. The conditional probability EUSij(t) of each component with failure rate λ, is shown in Table 2.
Number | 1 | 2 |
Coding | S21 | S23 |
Failure rate | λ=1.5e−6 | λ=2e−6 |
The time required for the four phases of the unmanned ship is T=[150,400,500,150]. The above information, along with the analysis of the mission reliability of the unmanned ship in Section 3, leads to Figure 9.
Figure 9 shows the unmanned ship mission reliability versus time for stages 1 through 4, respectively.
In phases 2 and 3, the unmanned ship works for a longer period of time and all the subsystems are involved in both phases. Since the unmanned ship subsystems are all in parallel in that phase, i.e., one subsystem fails and the mission fails. So the unmanned ship mission reliability in stages 2 and 3 decreases more compared to the other two stages. As can be seen in Figure 9, all components of the unmanned ship are in perfect condition in stage 1. So the decrease in reliability is not significant in this stage. At stage 4, the unmanned ship components are in a reduced state after a long period of operation. Thus, at the end of stage 4, the reliability of the unmanned ship system is poor. Such a trend is consistent with common sense and the assumptions made, as shown in Figure 10.
As can be seen in Figure 10, the curve of the mission reliability of the unmanned ship under the full cycle has three inflection points. These three inflection points are the intersection of two phases. The downward trend of the reliability of the unmanned ship changes after moving from the current phase to the next phase and is due to the change in the components involved in the job. From Figure 10 it can be seen that the unmanned ship has a work cycle of 1200 units of time, and there are many components involved, with a total of 19 components involved. So the reliability of finalizing the task is only 0.2878. It can be seen from Figures 9 and 10 that reliability optimization of the unmanned ship is important to improve the probability of the unmanned ship completing the mission.
The unmanned ship operates for a long time, and if some of the components fail, the shore staff cannot repair them in time. By adding redundancy, after a component fails, a new component participates in the work and the shore staff may not repair it.
For the optimization of the mission reliability of the unmanned ship, the cost of the components of the unmanned ship, as well as the capacity limitations of each component, are specified in this section, as shown in Table 3.
Number | Code | Capacity | Cost | Number | Code | Capacity | Cost |
1 | S11 | 15 | 1963 | 11 | S32 | 22 | 902 |
2 | S12 | 15 | 1562 | 12 | S33 | 16 | 962 |
3 | S13 | 15 | 2932 | 13 | S41 | 18 | 1679 |
4 | S14 | 16 | 1332 | 14 | S42 | 15 | 544 |
5 | S15 | 17 | 1654 | 15 | S43 | 22 | 1846 |
6 | S21 | 18 | 796 | 16 | S51 | 16 | 902 |
7 | S22 | 22 | 544 | 17 | S52 | 18 | 544 |
8 | S23 | 15 | 2489 | 18 | S53 | 15 | 1846 |
9 | S24 | 16 | 544 | 19 | S6 | 15 | 902 |
10 | S31 | 18 | 1846 |
The marginal optimization algorithm requires initial feasible solutions for the components of the unmanned ship. Based on the feasible solutions, the redundant components of the unmanned ship are optimized. The initial component feasible solution of the unmanned ship is set as [10,10,10,1,1,1,1,1,1,3,2,1,1,1,1,1,1,2,2,11]. The above initial feasible solution is the total number of a component and its redundant components. For this optimization problem, three optimization schemes are set up in this paper. We can get the different optimization schemes, as shown in Table 4.
Optimization method | Method of this paper | Regardless of cost | Stochastic |
Cost | 23,513 | 26,760 | 44,038 |
Initial reliability | 0.3284 | 0.3284 | 0.3284 |
Optimized result | 0.9288 | 0.9314 | 0.3391 |
The optimization scheme in Table 4 shows the optimization results when the number of redundant components is increased to 20. From Table 4, it is easy to see that the marginal optimization algorithm has the largest improvement in system reliability if cost is not considered. However, the cost is higher than the scheme proposed in this paper. From the above analysis, it is easy to see that the optimization scheme proposed in this paper is highly feasible and practical.
In order to continue to explore, the relationship between the optimization scheme described in this paper and the number of redundant components of the unmanned ship. According to the optimization process, the relationship between the number of redundant components of the unmanned ship and the reliability of the system can be obtained. Figure 11 shows the reliability curve during the optimization process.
From Figure 11, it is easy to see that the curve of the system's task reliability gradually slows down as the number of redundant components increases. This indicates that the optimization scheme proposed in this paper is more effective for a smaller number of components.
We evaluate the system's staged mission reliability based on the unmanned ship's operating characteristics, which are categorized into three scenarios. The marginal cost is used to determine the allocation scheme of components. The marginal cost integrates the impact of components on system reliability and the cost of components. In addition, the optimal redundancy allocation scheme is determined using the model of this paper based on the cost as well as the capacity constraints of this paper. Three different allocation schemes are compared to verify the effectiveness and practicality of the model proposed in this paper.
The process of unmanned ships performing missions often presents the characteristics of formation and clustering. Therefore, the mission reliability analysis of unmanned ship clusters is our future research direction. The research interests include the topology of unmanned ships, the reliability of communication links of unmanned ships and so on.
The authors declare they have not used Artificial Intelligence (AI) tools in the creation of this article.
The authors gratefully acknowledge the financial support for this research from the National Natural Science Foundation of China (No. 62173335).
The authors declare there is no conflict of interest.
[1] |
F. Liu, H. Tang, Y. Qin, C. Duan, J. Luo, H. Pu, Review on fault diagnosis of unmanned underwater vehicles, Ocean Eng., 243 (2022), 110290. https://doi.org/10.1016/j.oceaneng.2021.110290 doi: 10.1016/j.oceaneng.2021.110290
![]() |
[2] |
C. Gao, Y. Guo, M. Zhong, X. Liang, H. Wang, H. Yi, Reliability analysis based on dynamic Bayesian networks: A case study of an unmanned surface vessel, Ocean Eng., 240 (2021), 109970. https://doi.org/10.1016/j.oceaneng.2021.109970 doi: 10.1016/j.oceaneng.2021.109970
![]() |
[3] |
X. Li, Y. Li, H. Huang, E. Zio, Reliability assessment of phased-mission systems under random shocks, Reliab. Eng. Syst. Saf., 180 (2018), 352–361. https://doi.org/10.1016/j.ress.2018.08.002 doi: 10.1016/j.ress.2018.08.002
![]() |
[4] |
L. Xing, M. Tannous, V. M. Vokkarane, H. Wang, J. Guo, Reliability modeling of mesh storage area networks for Internet of Things, Reliab. Eng. Syst. Saf., 4 (2017), 2047–2057. https://doi.org/10.1109/JIOT.2017.2749375 doi: 10.1109/JIOT.2017.2749375
![]() |
[5] |
L. Xing, G. Levitin, BDD-based reliability evaluation of phased-mission systems with internal/external common-cause failures, Reliab. Eng. Syst. Saf., 112 (2013), 145–153. https://doi.org/10.1016/j.ress.2012.12.003 doi: 10.1016/j.ress.2012.12.003
![]() |
[6] |
M. Tang, T. Xiahou, Y. Liu, Mission performance analysis of phased-mission systems with cross-phase competing failures, Reliab. Eng. Syst. Saf., 234 (2023), 109174. https://doi.org/10.1016/j.ress.2023.109174 doi: 10.1016/j.ress.2023.109174
![]() |
[7] |
Z. Wang, S. Zeng, J. Guo, H. Che, A Bayesian network for reliability assessment of man-machine phased-mission system considering the phase dependencies of human cognitive error, Reliab. Eng. Syst. Saf., 207 (2021), 107385. https://doi.org/10.1016/j.ress.2020.107385 doi: 10.1016/j.ress.2020.107385
![]() |
[8] |
X. Wu, H. Yu, N. Balakrishnan, Modular model and algebraic phase algorithm for reliability modelling and evaluation of phased-mission systems with conflicting phase redundancy, Reliab. Eng. Syst. Saf., 227 (2022), 108735. https://doi.org/10.1016/j.ress.2022.108735 doi: 10.1016/j.ress.2022.108735
![]() |
[9] |
J. Li, Y. Lu, X. Liu, X. Jiang, Reliability analysis of cold-standby phased-mission system based on GO-FLOW methodology and the universal generating function, Reliab. Eng. Syst. Saf., 233 (2023), 109125. https://doi.org/10.1016/j.ress.2023.109125 doi: 10.1016/j.ress.2023.109125
![]() |
[10] |
C. Cheng, J. Yang, L. Li, Reliability evaluation of a k-out-of-n (G)-subsystem based multi-state phased mission system with common bus performance sharing subjected to common cause failures, Reliab. Eng. Syst. Saf., 216 (2021), 108003. https://doi.org/10.1016/j.ress.2021.108003 doi: 10.1016/j.ress.2021.108003
![]() |
[11] |
X. Li, X. Xiong, J. Guo, H. Huang, X. Li, Reliability assessment of non-repairable multi-state phased mission systems with backup missions, Reliab. Eng. Syst. Saf., 233 (2022), 108462. https://doi.org/10.1016/j.ress.2022.108462 doi: 10.1016/j.ress.2022.108462
![]() |
[12] |
C. Wang, L. Xing, J. Yu, Q. Guan, C. Yang, M. Yu, Phase reduction for efficient reliability analysis of dynamic k-out-of-n phased mission systems, Reliab. Eng. Syst. Saf., 237 (2023) 109349. https://doi.org/10.1016/j.ress.2023.109349 doi: 10.1016/j.ress.2023.109349
![]() |
[13] |
X. Zhou, G. Bai, J. Tao, B. Xu, An improved method to search all minimal paths in networks, IEEE Trans. Reliab., 2023 (2023). https://doi.org/10.1109/TR.2023.3234055 doi: 10.1109/TR.2023.3234055
![]() |
[14] |
B. Xu, G. Bai, T. Liu, Y. Fang, Y. A. Zhang, J. Tao, An improved swarm model with informed agents to prevent swarm-splitting, Chaos Solitons Fractals, 169 (2023), 113296. https://doi.org/10.1016/j.chaos.2023.113296 doi: 10.1016/j.chaos.2023.113296
![]() |
[15] |
T. Liu, G. Bai, J. Tao, Y. A. Zhang, Y. Fang, B. Xu, Modeling and evaluation method for resilience analysis of multi-state networks, Reliab. Eng. Syst. Saf., 226 (2022), 108663. https://doi.org/10.1016/j.ress.2022.108663 doi: 10.1016/j.ress.2022.108663
![]() |
[16] |
S. Anwar, S. Lone, A. Khan, S. Almutlak, Stress-strength reliability estimation for the inverted exponentiated Rayleigh distribution under unified progressive hybrid censoring with application, Electron. Res. Arch., 31 (2023), 4011–4033. https://doi.org/10.3934/era.2023204 doi: 10.3934/era.2023204
![]() |
[17] |
M. Liu, D. Wang, S. Si, Mixed reliability importance-based solving algorithm design for the cost-constrained reliability optimization model, Reliab. Eng. Syst. Saf., 237 (2023), 109363. https://doi.org/10.1016/j.ress.2023.109363 doi: 10.1016/j.ress.2023.109363
![]() |
[18] |
Z. Zhang, L. Yang, Y. Xu, R. Zhu, Y. Cao, A novel reliability redundancy allocation problem eqtion for complex systems, Reliab. Eng. Syst. Saf., 239 (2023), 109471. https://doi.org/10.1016/j.ress.2023.109471 doi: 10.1016/j.ress.2023.109471
![]() |
[19] |
S. Li, X. Chi, B. Yu, An improved particle swarm optimization algorithm for the reliability-redundancy allocation problem with global reliability, Reliab. Eng. Syst. Saf., 225 (2022), 108604. https://doi.org/10.1016/j.ress.2022.108604 doi: 10.1016/j.ress.2022.108604
![]() |
[20] |
D. Xu, Y. Tian, J. Shi, D. Wang, M. Zhang, H. Li, Reliability analysis and optimal redundancy for a satellite power supply system based on a new dynamic k-out-of-n: G model, Reliab. Eng. Syst. Saf., 236 (2023), 109317. https://doi.org/10.1016/j.ress.2023.109317 doi: 10.1016/j.ress.2023.109317
![]() |
[21] |
X. Y. Li, X. Li, C. Li, X. Xiong, H. Huang, Reliability analysis and optimization of multi-phased spaceflight with backup missions and mixed redundancy strategy, Reliab. Eng. Syst. Saf., 237 (2023), 109373. https://doi.org/10.1016/j.ress.2023.109373 doi: 10.1016/j.ress.2023.109373
![]() |
[22] |
C. W. Yeh, W. Zhu, S. Y. Tan, G. Wang, Y. Yeh, Novel general active reliability redundancy allocation problems and algorithm, Reliab. Eng. Syst. Saf., 218 (2022), 108167. https://doi.org/10.1016/j.ress.2022.108843 doi: 10.1016/j.ress.2022.108843
![]() |
[23] |
M. Nourelfath, E. Châtelet, N. Nahas, Joint redundancy and imperfect preventive maintenance optimization for series-parallel multi-state degraded systems, Reliab. Eng. Syst. Saf., 103 (2012), 51–60. https://doi.org/10.1016/j.ress.2012.03.004 doi: 10.1016/j.ress.2012.03.004
![]() |
[24] |
J. Zhang, D. Du, X. Si, C. Hu, H. Zhang, Joint optimization of preventive maintenance and inventory management for standby systems with hybrid-deteriorating spare parts, Reliab. Eng. Syst. Saf., 214 (2021), 107686. https://doi.org/10.1016/j.ress.2021.107686 doi: 10.1016/j.ress.2021.107686
![]() |
[25] |
W. Wang, Z. Wu, J. Xiong, Y. Xu, Redundancy optimization of cold-standby systems under periodic inspection and maintenance, Reliab. Eng. Syst. Saf., 180 (2018), 394–402. https://doi.org/10.1016/j.ress.2018.08.004 doi: 10.1016/j.ress.2018.08.004
![]() |
[26] | K. Atashgar, H. Abdollahzadeh, Reliability optimization of wind farms considering redundancy and opportunistic maintenance strategy, 112 (2016), 445–458. https://doi.org/10.1016/j.enconman.2016.01.027 |
[27] |
E. Golmohammadi, M. A. Ardakan, Reliability optimization problem with the mixed strategy, degrading components, and a periodic inspection and maintenance policy, Reliab. Eng. Syst. Saf., 223 (2022), 108500. https://doi.org/10.1016/j.ress.2022.108500 doi: 10.1016/j.ress.2022.108500
![]() |
[28] |
Q. Feng, M. Liu, H. Dui, Y. Ren, B. Sun, D. Yang, et al., Importance measure-based phased mission reliability and UAV number optimization for swarm, Reliab. Eng. Syst. Saf., 223 (2022), 108478. https://doi.org/10.1016/j.ress.2022.108478 doi: 10.1016/j.ress.2022.108478
![]() |
[29] |
H. Dui, X. Yang, M. Liu, Importance measure-based maintenance analysis and spare parts storage configuration in two-echelon maintenance and supply support system, Int. J. Prod. Res., 2022 (2022). https://doi.org/10.1080/00207543.2022.2142312 doi: 10.1080/00207543.2022.2142312
![]() |
[30] |
R. Yan, Y. Yang, Y. Du, Stochastic optimization model for ship inspection planning under uncertainty in maritime transportation. Electron. Res. Arch., 31 (2023), 103–122. https://doi.org/10.3934/era.2023006 doi: 10.3934/era.2023006
![]() |
[31] |
J. Chang, X. Yin, C. Ma, D. Zhao, Y. Sun, Estimation of the time cost with pinning control for stochastic complex networks, Electron. Res. Arch., 30 (2022), 3509–3526. https://doi.org/10.3934/era.2022179 doi: 10.3934/era.2022179
![]() |
[32] |
J. Ren, S. Qu, L. Wang, L. Ma, T. Lu, Aircraft scheduling optimization model for on-ramp of corridors-in-the-sky, Electron. Res. Arch., 31 (2023), 3625–3648. https://doi.org/10.3934/era.2023184 doi: 10.3934/era.2023184
![]() |
Serial number | Coding | θ | γ | Serial number | Coding | θ | γ |
1 | S11 | 3247 | 1.65 | 11 | S32 | 2199 | 1.75 |
2 | S12 | 2574 | 1.76 | 12 | S33 | 2199 | 1.75 |
3 | S13 | 2416 | 2.21 | 13 | S41 | 1478 | 1.78 |
4 | S14 | 2150 | 1.96 | 14 | S42 | 1478 | 1.78 |
5 | S15 | 3589 | 2.14 | 15 | S43 | 3247 | 1.65 |
6 | S21 | 3589 | 2.14 | 16 | S51 | 2574 | 1.76 |
7 | S22 | 3165 | 1.54 | 17 | S52 | 2416 | 2.21 |
8 | S23 | 3165 | 1.54 | 18 | S53 | 2150 | 1.96 |
9 | S24 | 2550 | 1.48 | 19 | S6 | 3589 | 2.14 |
10 | S31 | 2550 | 1.48 | \ | \ | \ | \ |
Number | 1 | 2 |
Coding | S21 | S23 |
Failure rate | λ=1.5e−6 | λ=2e−6 |
Number | Code | Capacity | Cost | Number | Code | Capacity | Cost |
1 | S11 | 15 | 1963 | 11 | S32 | 22 | 902 |
2 | S12 | 15 | 1562 | 12 | S33 | 16 | 962 |
3 | S13 | 15 | 2932 | 13 | S41 | 18 | 1679 |
4 | S14 | 16 | 1332 | 14 | S42 | 15 | 544 |
5 | S15 | 17 | 1654 | 15 | S43 | 22 | 1846 |
6 | S21 | 18 | 796 | 16 | S51 | 16 | 902 |
7 | S22 | 22 | 544 | 17 | S52 | 18 | 544 |
8 | S23 | 15 | 2489 | 18 | S53 | 15 | 1846 |
9 | S24 | 16 | 544 | 19 | S6 | 15 | 902 |
10 | S31 | 18 | 1846 |
Optimization method | Method of this paper | Regardless of cost | Stochastic |
Cost | 23,513 | 26,760 | 44,038 |
Initial reliability | 0.3284 | 0.3284 | 0.3284 |
Optimized result | 0.9288 | 0.9314 | 0.3391 |
Serial number | Coding | θ | γ | Serial number | Coding | θ | γ |
1 | S11 | 3247 | 1.65 | 11 | S32 | 2199 | 1.75 |
2 | S12 | 2574 | 1.76 | 12 | S33 | 2199 | 1.75 |
3 | S13 | 2416 | 2.21 | 13 | S41 | 1478 | 1.78 |
4 | S14 | 2150 | 1.96 | 14 | S42 | 1478 | 1.78 |
5 | S15 | 3589 | 2.14 | 15 | S43 | 3247 | 1.65 |
6 | S21 | 3589 | 2.14 | 16 | S51 | 2574 | 1.76 |
7 | S22 | 3165 | 1.54 | 17 | S52 | 2416 | 2.21 |
8 | S23 | 3165 | 1.54 | 18 | S53 | 2150 | 1.96 |
9 | S24 | 2550 | 1.48 | 19 | S6 | 3589 | 2.14 |
10 | S31 | 2550 | 1.48 | \ | \ | \ | \ |
Number | 1 | 2 |
Coding | S21 | S23 |
Failure rate | λ=1.5e−6 | λ=2e−6 |
Number | Code | Capacity | Cost | Number | Code | Capacity | Cost |
1 | S11 | 15 | 1963 | 11 | S32 | 22 | 902 |
2 | S12 | 15 | 1562 | 12 | S33 | 16 | 962 |
3 | S13 | 15 | 2932 | 13 | S41 | 18 | 1679 |
4 | S14 | 16 | 1332 | 14 | S42 | 15 | 544 |
5 | S15 | 17 | 1654 | 15 | S43 | 22 | 1846 |
6 | S21 | 18 | 796 | 16 | S51 | 16 | 902 |
7 | S22 | 22 | 544 | 17 | S52 | 18 | 544 |
8 | S23 | 15 | 2489 | 18 | S53 | 15 | 1846 |
9 | S24 | 16 | 544 | 19 | S6 | 15 | 902 |
10 | S31 | 18 | 1846 |
Optimization method | Method of this paper | Regardless of cost | Stochastic |
Cost | 23,513 | 26,760 | 44,038 |
Initial reliability | 0.3284 | 0.3284 | 0.3284 |
Optimized result | 0.9288 | 0.9314 | 0.3391 |