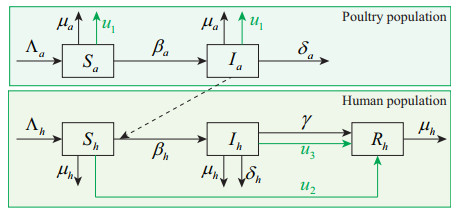
Avian influenza is an infectious viral disease caused by type A virus, which occurs frequently around the world and causes serious economic losses. Therefore, the adaptive control problem is explored in this paper for an avian influenza model in consideration of slaughtering to poultry, educational campaigns to the susceptible human and treatment to the infected human. First, by analyzing the transmission mechanism of avian influenza, a nonlinear adaptive control problem of avian influenza model is formulated, where some errors between model parameters and real values are allowed. Then, the parameters are estimated by constructing adaptive laws, which can be effectively used to design the applicative controllers to achieve the control goals. Besides, the stability of controlled model is analyzed with the aid of Lyapunov stability theory. Finally, numerical examples are proposed to verify the effectiveness and robustness of the designed controllers.
Citation: Ting Kang, Qimin Zhang, Qingyun Wang. Nonlinear adaptive control of avian influenza model with slaughter, educational campaigns and treatment[J]. Electronic Research Archive, 2023, 31(8): 4346-4361. doi: 10.3934/era.2023222
[1] | Qun Dai, Zeheng Wang . SIRV fractional epidemic model of influenza with vaccine game theory and stability analysis. Electronic Research Archive, 2024, 32(12): 6792-6821. doi: 10.3934/era.2024318 |
[2] |
Zuliang Lu, Fei Huang, Xiankui Wu, Lin Li, Shang Liu .
Convergence and quasi-optimality of |
[3] | Chao Ma, Hang Gao, Wei Wu . Adaptive learning nonsynchronous control of nonlinear hidden Markov jump systems with limited mode information. Electronic Research Archive, 2023, 31(11): 6746-6762. doi: 10.3934/era.2023340 |
[4] | Qianqian Zhang, Mingye Mu, Heyuan Ji, Qiushi Wang, Xingyu Wang . An adaptive type-2 fuzzy sliding mode tracking controller for a robotic manipulator. Electronic Research Archive, 2023, 31(7): 3791-3813. doi: 10.3934/era.2023193 |
[5] | Xinling Li, Xueli Qin, Zhiwei Wan, Weipeng Tai . Chaos synchronization of stochastic time-delay Lur'e systems: An asynchronous and adaptive event-triggered control approach. Electronic Research Archive, 2023, 31(9): 5589-5608. doi: 10.3934/era.2023284 |
[6] | Sida Lin, Lixia Meng, Jinlong Yuan, Changzhi Wu, An Li, Chongyang Liu, Jun Xie . Sequential adaptive switching time optimization technique for maximum hands-off control problems. Electronic Research Archive, 2024, 32(4): 2229-2250. doi: 10.3934/era.2024101 |
[7] | Huan Luo . Heterogeneous anti-synchronization of stochastic complex dynamical networks involving uncertain dynamics: an approach of the space-time discretizations. Electronic Research Archive, 2025, 33(2): 613-641. doi: 10.3934/era.2025029 |
[8] | Ziang Chen, Chunguang Dai, Lei Shi, Gaofang Chen, Peng Wu, Liping Wang . Reaction-diffusion model of HIV infection of two target cells under optimal control strategy. Electronic Research Archive, 2024, 32(6): 4129-4163. doi: 10.3934/era.2024186 |
[9] | Xiangwen Yin . Promoting peer learning in education: Exploring continuous action iterated dilemma and team leader rotation mechanism in peer-led instruction. Electronic Research Archive, 2023, 31(11): 6552-6563. doi: 10.3934/era.2023331 |
[10] | Liping Fan, Pengju Yang . Load forecasting of microgrid based on an adaptive cuckoo search optimization improved neural network. Electronic Research Archive, 2024, 32(11): 6364-6378. doi: 10.3934/era.2024296 |
Avian influenza is an infectious viral disease caused by type A virus, which occurs frequently around the world and causes serious economic losses. Therefore, the adaptive control problem is explored in this paper for an avian influenza model in consideration of slaughtering to poultry, educational campaigns to the susceptible human and treatment to the infected human. First, by analyzing the transmission mechanism of avian influenza, a nonlinear adaptive control problem of avian influenza model is formulated, where some errors between model parameters and real values are allowed. Then, the parameters are estimated by constructing adaptive laws, which can be effectively used to design the applicative controllers to achieve the control goals. Besides, the stability of controlled model is analyzed with the aid of Lyapunov stability theory. Finally, numerical examples are proposed to verify the effectiveness and robustness of the designed controllers.
Avian influenza is a viral infectious disease induced by type A virus, which can be divided into the low pathogenic avian influenza and highly pathogenic avian influenza [1,2]. Both types of viruses commonly occur among wild birds around the world and can infect domestic poultry (such as turkeys, quails, chickens) and other bird species. To date, some avian influenza viruses, such as A (H5N1, H5N6, H7N9) [3,4,5,6] have been frequently reported to cross the species barrier, causing severe infections in humans and other mammals, and causing serious problems. For example, Hong Kong first reported 18 human cases (including 6 deaths) of avian influenza A (H5N1) in 1997, which attracted global attentions [7]. Since then, avian influenza viruses (such as H7N9, H5N6, etc.) have also been reported to pose a great potential threat to human. For example, H1N1 flu struck the United States and spread to 214 countries and territories, killing nearly 200,000 people in 2009; there are 132 cases of infected H7N9 virus were found, including 37 deaths in 2013; as of December 2019, the global cumulative number of cases of human infection with H5N1 avian influenza arrives 861, including 455 deaths [8,9,10]. Epidemiological investigations confirmed that the majority of people infected with H7N9 and H5N6 have had contact with infected poultry [11,12]. Avian influenza viruses, such as H7N9, H5N6 and H5N1, have not yet been found to be transmissible between humans, but sporadic outbreaks in human remain a enormous threat to world public health security. Live poultry markets and backyard poultry flocks are two main places of risk major for sporadic outbreaks of avian influenza in human [13,14]. It has also been noted that a mass of people infected with the avian influenza viruses has gradually declined after the closure of live poultry markets in many provinces [15]. Because of the frequent outbreaks of avian influenza worldwide, we need to have an in-depth understanding of the transmission dynamics of avian influenza and further propose the better control scheme to control its spread.
On the one hand, avian influenza has posed enormous economic pressures on society, mainly including opportunity loss, health-related expenditures and unemployment. On the other hand, the cost of implementing control measures, such as vaccination, treatment and educational campaigns, is also high. Hence, it is an important and meaningful problem to establish optimal control mechanisms of avian influenza with balance of costs and benefits from the epidemiological and social economic viewpoint. To address these issues, many researchers addressed the optimal control problems of avian influenza by introducing lots of control variables and applying the Pontryagin's maximum principle, and proposed the corresponding control strategies (both pharmaceutical and non-pharmaceutical) [8,16,17,18]. It should be pointed out that for optimal control, the control variables are obtained by setting the objective function. If the control objective is set as desired number of infected poultry or humans, the optimal control theory is invalid. Adaptive control, as one of the important control methods [19,20], allows us to preset the control objective concerning the number of infected poultry or humans [21,22]. Besides, different from the optimal control, where all the parameters are assumed to be completely known, the adaptive control can effectively estimate the system parameters through the designed adaptive laws and the control input is determined by the estimated parameters, which can overcome the problem of parameter uncertainties in the common transmission of avian influenza viruses. Since the theory of adaptive control was put forward in the field of engineering, its theoretical development is more and more perfect, and it can accurately deal with the control problems of nonlinear systems in engineering. Unfortunately, there are few studies on adaptive control strategies for biological systems [21,22,23], where the nonlinear adaptive control problems were studied for cancer chemotherapy, COVID-19, tuberculosis model, respectively. To our knowledge, for the adaptive control issue of avian influenza model, there is no relevant research results, which is the main motivation of this study.
Poultry is the main source responsible for the transmission of avian influenza virus to humans. Because of the difficulty in tracing and diagnosing infected poultry, control measures should be implemented for both susceptible and infected poultry populations. Especially, slaughter is a reactive control strategy aimed at eliminating infected poultry early in the outbreak, and the specific implementation policies of different regions should be different since slaughter is a high socio-economic losses control measure [24]. At the same time, the government departments will disseminate the avian influenza information through the mass media and internet in the early stage of the outbreak, so as to enhance the humans' public health awareness. Based on the avian influenza information disseminated by the government, some populations will change their behavior to reduce contact with the source of infection, which can reduce their risk of contracting avian influenza [25,26]. Moreover, timely treatment of infected humans during avian influenza outbreaks can effectively reduce mortality [27]. In conclusion, different control measures are of great significance in controlling the practical spread of avian influenza at different stages of avian influenza outbreaks, so it is necessary to explore the existed control effect of mixed control measures (educational campaigns to the susceptible human population, slaughtering to poultry and treatment to infected humans).
For all we know, there is no research result about the adaptive control strategies of avian influenza models. Hence, the main purpose of this paper is to design precise adaptive controllers and obtain the estimation values of unknown parameters according to the designed adaptive laws. The structure of this paper is arranged as follows: the avian influenza model is established and the adaptive control problem is formulated in Section 2. In Section 3, the stability of the closed-loop system under the adaptive laws and the designed controllers is analyzed. In Section 4, the effectiveness of the designed controllers is verified. Finally, a brief summary is presented in Section 5.
Because poultry is the most important infectious source to human, we only consider the transmission of avian influenza between poultry and human in this paper. The total poultry population Na(t) is divided into the susceptible poultry Sa(t) and the infected poultry Ia(t), and the total human population Nh(t) is classified into susceptible human Sh(t), infected human Ih(t) and Rh(t), where Rh represents human with certain immunity to avian influenza virus, which includes physiological immunity (due to infection and then recovery) and psychological immunity (due to changes in their own behavior under educational campaigns). The mathematical model is formulated as follows
{˙Sa(t)=Λa−βaSa(t)Ia(t)−(μa+u1(t))Sa(t),˙Ia(t)=βaSa(t)Ia(t)−(μa+δa+u1(t))Ia(t),˙Sh(t)=Λh−βhSh(t)Ia(t)−(μh+u2(t))Sh(t),˙Ih(t)=βhSh(t)Ia(t)−(μh+δh+γ+u3(t))Ih(t),˙Rh(t)=γIh(t)+u2(t)Sh(t)+u3(t)Ih(t)−μhRh(t), | (2.1) |
where all parameters are assumed non-negative and described as follows: Λa (Λh) stands for the new recruitment of poultry (human); βa and βh are transmission rates from infectious poultry to susceptible poultry and human; μa (μh) and δa (δh) are natural and disease-related death rates of poultry (human), respectively; γ is the recovery rate of the infectious human. Control variable u1(t) represents slaughter to susceptible and infected poultry, u2(t) represents educational campaigns to susceptible humans and u3(t) denotes treatment for infected humans. The control variables u1(t), u2(t) and u3(t) are defined to decrease the number of infected poultry and infected humans, respectively. We denote Sa(t),Ia(t),Sh(t),Ih(t),Rh(t) as Sa,Ia,Sh,Ih,Rh for convenience. In order to more clearly demonstrate the transmission process of avian influenza described in model (2.1), we also provide a schematic diagram as shown in Figure 1.
The reasons why we set u1(t),u2(t) and u3(t) as control variables are summarized as follows:
(i) For human, the infected poultry is the most important spreading source of avian influenza, and in practice, the susceptible and infected poultry may not be effectively distinguished. Thus, in order to quickly eliminate the spreading source, the slaughter to both susceptible and infected poultry is a necessary and effective strategy, which can be described by the control variable u1(t).
(ii) When avian influenza spreads in poultry, it is necessary to warn people not to contact infected poultry or contaminated poultry markets through educational campaigns to prevent the spread of avian influenza among human. Therefore, the control variable u2(t) is introduced into (2.1).
(iii) Once diagnosed with avian influenza, patients are hospitalized. The specific treatment method, either single or combination treatment, should be applied by the severity of the patient's conditions. Furthermore, the dosage of medicine and length of hospitalization also vary with the severity of the patient's conditions. Thus, the treatment measure is a necessary strategy for the infected humans, which is described by control variable u3(t).
The control objective of this paper is to make the number of susceptible and infected poultry (humans) achieve the preset goals with appropriate speed by adopting control measures u1(t),u2(t) and u3(t).
In this section, we will design the schemes of control variables u1(t),u2(t) and u3(t) to achieve the control objective, and analyze the stability of model (2.1) under the designed control schemes.
Compared with the classical optimal control, it is allowable to adaptive control that we can adjust the values of system states to set the control variables u1(t), u2(t) and u3(t), and the purpose of which is to gradually reduce the number of susceptible poultry, susceptible humans and infected humans to reach the desired values. Additionally, the uncertainty of system parameters is inevitable for the practical avian influenza model. Thus, in order to indicate the robust of the presented control schemes, we also take some difference of parameters into account and analyze the stability of controlled system.
To give the final control schemes of u1(t),u2(t) and u3(t), we first formulate the control inputs in avian influenza model (2.1) as follows
u1(t)=−˙NaNa+ΛaNa−μaSaNa−(μa+δa)IaNa, | (3.1) |
u2(t)=−˙ShSh+ΛhSh−βhIa−μh, | (3.2) |
u3(t)=−˙IhIh+βhShIaIh−(μh+δh+γ). | (3.3) |
Since the terms ˙Na,˙Sh and ˙Ih in (3.1)–(3.3) cannot be directly obtained, we now replace them by x1, x2 and x3, respectively, and the forms of x1, x2 and x3 will be specified later. Therefore, by rearranging (3.1)–(3.3) in the linearly parameterized forms, we can get
u1(t)=ΨT1(x1,Sa,Ia)θ1,u2(t)=ΨT2(x2,Sh,Ia)θ2,u3(t)=ΨT3(x3,Sh,Ia,Ih)θ3, |
where vectors Ψ1(x1,Sa,Ia), Ψ2(x2,Sh,Ia) and Ψ3(x3,Sh,Ia,Ih) consist of known and measurable functions, and vectors θi(i=1,2,3) include the unknown parameters in model (2.1) and can be estimated by the adaptive laws. Based on (3.1)–(3.3), Ψi and θi(i=1,2,3) can be expressed as follows
Ψ1(x1,Sa,Ia)=[−x1Na,1Na,−SaNa,−IaNa]T, | (3.4) |
Ψ2(x2,Sh,Ia)=[−x2Sh,1Sh,−Ia,−1]T, | (3.5) |
Ψ3(x3,Sh,Ia,Ih)=[−x3Ih,ShIaIh,−1]T, | (3.6) |
θ1=[1,Λa,μa,μa+δa]T, | (3.7) |
θ2=[1,Λh,βh,μh]T, | (3.8) |
θ3=[1,βh,μh+δh+γ]T. | (3.9) |
Now, the variables x1, x2 and x3 are defined as
x1=˙Nad−α1˜Na,x2=˙Shd−α2˜Sh,x3=˙Ihd−α3˜Ih, |
where αi>0(i=1,2,3) are the designed parameters, Nad,Shd and Ihd are the desired values of Na,Sh and Ih, respectively, ˜Na=Na−Nad, ˜Sh=Sh−Shd and ˜Ih=Ih−Ihd are defined as the tracking errors related to the desired values Nad,Shd and Ihd, respectively.
Remark 1. The optimal control [16] obtains the values of control variables by setting objective function, which cannot preset the number of infected poultry or human as control objective, while for the adaptive control, the values of Na,Sh,Ih can converge to the desired values Nad,Shd,Ihd, respectively. Thus, we can set the values of Nad,Shd,Ihd such that Na,Sh,Ih achieve the given number of infected poultry or human.
Considering uncertainty of parameters in the avian influenza model, the schemes of control variables can be designed as follows
ˆu1(t)=−˙Nad−α1˜NaNa+ˆΛaNa−ˆμaSaNa−(ˆμa+ˆδa)IaNa=ΨT1(x1,Sa,Ia)ˆθ1, | (3.10) |
ˆu2(t)=−˙Shd−α2˜ShSh+ˆΛhSh−ˆβhIa−ˆμh=ΨT2(x2,Sh,Ia)ˆθ2, | (3.11) |
ˆu3(t)=−˙Ihd−α3˜IhIh+ˆβhShIaIh−(ˆμh+ˆδh+ˆγ)=ΨT3(x3,Sh,Ia,Ih)ˆθ3, | (3.12) |
where ˆθi(i=1,2,3) are the estimations of vectors θi with the following forms
ˆθ1=[1,ˆΛa,ˆμa,ˆμa+ˆδa]T, | (3.13) |
ˆθ2=[1,ˆΛh,ˆβh,ˆμh]T, | (3.14) |
ˆθ3=[1,ˆβh,ˆμh+ˆδh+ˆγ]T. | (3.15) |
From (3.10)–(3.12), we see that if Na,Sh or Ih tends to zero, the values of ˆu1(t),ˆu2(t) or ˆu3(t) may tend to infinity. Thus, we modify them as follows
ˆu1(t)={Ψ1(x1,Sa,Ia)ˆθ1,Na≥κ1,0,Na<κ1,ˆu2(t)={Ψ2(x2,Sh,Ia)ˆθ2,Sh≥κ2,0,Sh<κ2,ˆu3(t)={Ψ3(x3,Sh,Ia,Ih)ˆθ3,Ih≥κ3,0,Ih<κ3, |
where κ1,κ2 and κ3 are small positive constants. For poultry, if Na tends to zero, then almost all of poultry has been slaughtered, which means that the control measure u1 can be canceled. Thus, the modification for ˆu1(t) is reasonable. Similarly, the rationality of modifications for ˆu2(t) and ˆu3(t) can be analyzed. Since these modifications do not influence on the stability of controlled system, we also adopt (3.10)–(3.12) during stability analysis. The adaptive laws of parameter vectors ˆθi(i=1,2,3) are designed as follows
˙ˆθ1=˜NaNaΓT1Ψ1, | (3.16) |
˙ˆθ2=˜ShShΓT2Ψ2, | (3.17) |
˙ˆθ3=˜IhIhΓT3Ψ3, | (3.18) |
where positive definite matrices Γi(i=1,2,3) denote adaptive gain matrices. From the adaptive laws (3.16)–(3.18), we can obtain the estimations of vectors ˆθ1,ˆθ2 and ˆθ3, and thus the control inputs ˆu1(t),ˆu2(t) and ˆu3(t) can be calculated, which will be used to control the model (2.1) such that the state variables Na,Sh and Ih converge to Nad,Shd and Ihd, respectively.
In this section, we will investigate the stability of the presented closed-loop system under the designed adaptive control strategy by constructing the appropriate Lyapunov function.
Theorem 1. For the avian influenza model (2.1), the designed control schemes (3.10)–(3.12) and parameter adaptive laws (3.16)–(3.18) can ensure that:
(i) All variables in the presented closed-loop system are bounded;
(ii) Na=Sa+Ia,Sh and Ih asymptotically converge to Nad,Shd and Ihd.
Proof. Consider the following Lyapunov function candidate:
V=12[˜N2a+˜S2h+˜I2h+˜θT1Γ−11˜θ1+˜θT2Γ−12˜θ2+˜θT3Γ−13˜θ3], |
where ˜Na=Na−Nad,˜Sh=Sh−Shd,˜Ih=Ih−Ihd,˜θi=ˆθi−θi(i=1,2,3). Since θi are constants, we have ˙˜θi=˙ˆθ1(i=1,2,3). Therefore, the derivative of V is calculated as
˙V=˜Na(˙Na−˙Nad)+˜Sh(˙Sh−˙Shd)+˜Ih(˙Ih−˙Ihd)+˙ˆθT1Γ−11˜θ1+˙ˆθT2Γ−12˜θ2+˙ˆθT3Γ−13˜θ3=˜Na[Λa−μaSa−(μa+δa)Ia−u1Na]−˙Nad˜Na+˜Sh[Λh−βhShIa−μhSh−u2Sh]−˙Shd˜Sh+˜Ih[βhShIa−(μh+δh+γ)Ih−u3Ih]−˙Ihd˜Ih+˙ˆθT1Γ−11˜θ1+˙ˆθT2Γ−12˜θ2+˙ˆθT3Γ−13˜θ3. | (3.19) |
Substituting (3.10)–(3.12) into (3.19) and rearranging its terms, one has
˙V=−˜Na(ˆΛa−Λa)+Sa˜Na(ˆμa−μa)+Ia˜Na(ˆμa+ˆδa−(μa+δa))−α1˜N2a−˜Sh(ˆΛh−Λh)+IaSh˜Sh(ˆβh−βh)+Sh˜Sh(ˆμh−μh)−α2˜S2h−ShIa˜Ih(ˆβh−βh)+Ih˜Ih((ˆμh+ˆδh+ˆγ)−(μh+δh+γ))−α3˜I2h+˙ˆθT1Γ−11˜θ1+˙ˆθT2Γ−12˜θ2+˙ˆθT3Γ−13˜θ3. |
It then follows from ˜θi=ˆθi−θi(i=1,2,3) and (3.4)–(3.9), (3.13)–(3.15) that
˙V=−α1˜N2a−α2˜S2h−α3˜I2h−Na˜NaΨT1˜θ1−Sh˜ShΨT2˜θ2−Ih˜IhΨT3˜θ3+˙ˆθT1Γ−11˜θ1+˙ˆθT2Γ−12˜θ2+˙ˆθT3Γ−13˜θ3=−α1˜N2a−α2˜S2h−α3˜I2h+(˙ˆθT1Γ−11−Na˜NaΨT1)˜θ1+(˙ˆθT2Γ−12−Sh˜ShΨT2)˜θ2+(˙ˆθT3Γ−13−Ih˜IhΨT3)˜θ3. | (3.20) |
Substituting (3.16)–(3.18) into (3.20), the time derivative of the presented Lyapunov function is calculated as
˙V=−α1˜N2a−α2˜S2h−α3˜I2h≤0. | (3.21) |
From the inequality (Eq 3.21), we have V(Υ(t))≤V(Υ(0)) with Υ=(˜Na,˜Sh,˜Ia,˜θT1,˜θT2,˜θT3)T, which implies the boundedness of ˜Na,˜Sh,˜Ia,˜θ1,˜θ2 and ˜θ3. Since θ1,θ2 and θ3 are constant vectors, ˆθ1,ˆθ2 and ˆθ3 are bounded. Besides, it follows from the boundedness of Nad,Shd and Ihd that Na,Sh and Ih are bounded. From (2.1), Sa(t),Ia(t),Sh(t),Ih(t) and Rh(t) are non-negative for any non-negative initial values Sa(0),Ia(0),Sh(0),Ih(0) and Rh(0). Thus, Sa and Ia are bounded. In addition, from the last three equations of (2.1), we have Sh+Ih+Rh≤Λhμh, so Rh is also bounded. Hence, all variables in the closed-loop system are bounded.
Now, we show the asymptotic convergence of Na,Sh and Ih. The time derivative of ˙V can be calculated as
¨V=−2α1˜Na˙˜Na−2α2˜Sh˙˜Sh−2α3˜Ih˙˜Ih. | (3.22) |
Since Sa(t),Ia(t),Sh(t),Ih(t) and Rh(t) are non-negative and bounded, we obtain from (2.1) that ˙Na,˙Sh and ˙Ih are bounded, which, together with ˜Na=Na−Nad, ˜Sh=Sh−Shd and ˜Ih=Ih−Ihd, imply the boundedness of ˙˜Na,˙˜Sh and ˙˜Ih. Thus, from (3.22), we know that ¨V is bounded, which means that ˙V is uniformly continuous. By feat of the Barbalat's lemma [28], we conclude that the tracking errors ˜Na,˜Sh and ˜Ih will converge to zero when t→∞, i.e., Na→Nad, Sh→Shd and Ih→Iad. This completes the proof.
This section is devoted to illustrating the effectiveness of the designed controllers. The parameter values of model (2.1) are presented in Table 1, and the initial value is (Sa(0),Ia(0),Sh(0),Ih(0),Rh(0))=(3,363,000,100,52,000,10,1). The designed parameters are chosen as: κ1=κ2=κ3=1, α1=310,α2=23.5,α3=20 and
Γ1=[10.20.30.10.320.40.10.30.220.20.50.10.22],Γ2=[20.30.40.20.140.20.30.10.320.10.30.30.44],Γ3=[10.40.20.130.40.10.22]. |
Parameter | Value | Source of data |
Λa | 1000/245 day−1 | [8,29] |
βa | 8.1×10−6 day−1 | Assumed |
μa | 1/245 day−1 | [8,29] |
δa | 6/400 day−1 | Assumed |
Λh | 2000/36,500 day−1 | [8,29] |
βh | 2×10−9 day−1 | [8,29] |
μh | 5.48×10−5 day−1 | [8,30] |
δh | 0.001 day−1 | [8,30] |
γ | 0.1 day−1 | [8,30] |
The minimum eigenvalues of Γ1,Γ2 and Γ3 are 0.8760,1.7992 and 0.9693, respectively. Thus, Γ1,Γ2 and Γ3 are positive definite matrices, which satisfy the condition of Theorem 3.1. The desired values are selected as
Nad=a1+(Sa(0)+Ia(0)−a1)e−a2t,Shd=b1+(Sh(0)−b1)e−b2t,Ihd=rβh∫tt−τS(ω)I(ω)dω+Ih(0)e−0.5t, |
where a1=1500,a2=0.6, b1=25,000,b2=0.01, τ=5,r=0.6.
Remark 2. The forms of desired values Nad, Shd and Ihd are selected based on the practical requirements. In fact, for Nad (Shd) (they have similar forms):
(i) a1 (b1) represents the finally desired value of total poultry population Na (susceptible human Sh);
(ii) since the slaughtering to poultry and educational campaigns to human can not work immediately, we introduce the terms e−a2t and e−b2t to describe the process of the number of poultry and susceptible human falling from the initial value to the finally desired values, where a2 and b2 denote the descending rates.
In addition, r and τ denote the treatment efficiency and treatment duration for infected human (Ihd), respectively. Meanwhile, the number of infected humans needing treatment is related to the number of infected people from t−τ to t and the treatment efficiency, so the form of Ihd can be obtained. It is worth noting that the above mentioned parameters a1,a2,b1,b2,τ and r can be adjusted according to the cost of epidemic prevention and control.
Example 1. The effects of different control combination.
In order to reveal the effect of each control variable, consider the following eight control combinations: Case A1, u1=u2=u3=0; Case A2, u1≠0,u2=u3=0; Case A3, u2≠0,u1=u3=0; Case A4, u1=u2=0,u3≠0; Case A5, u1,u2≠0,u3=0; Case A6, u1,u3≠0,u2=0; Case A7, u1=0,u2,u3≠0; Case A8, u1,u2,u3≠0. Here, ui≠0 (i=1,2 or 3) denotes that the corresponding control measure is adopted instead of ui(t)≠0 for all t≥0. The simulation results are shown in Figures 2–9.
Figures 2 and 3 present the trajectories of Sa(t) and Ia(t) under different control combinations, from which we see that once the control measure u1(t) is adopted (i.e., Cases A2, A5, A6 and A8), the infected poultry Ia(t) will decrease significantly. Since the control measures u2(t) and u3(t) only act on the human population, they cannot influence the poultry population. From Figure 4, we can see the effect of control measure u2(t). In Cases A1, A2, A4 and A6, u2(t) is in absence, so the trajectories of susceptible human Sh(t) drop slowly, while in other cases, the trajectories of Sh(t) drop rapidly, since some people move into Rh through educational campaigns. Figure 5 gives the trajectories of Ih(t), which shows the effect of each control combination more clearly. When all of control measures are in absence (i.e., Case A1), the peak value of infected human Ih(t) is more than 150, while the peak value of Ia(t) is not more than 20 when all control measures are adopted (i.e., Case A8), which shows that the adopted control measures have a significant impact on the spread of the avian influenza. Besides, in Figure 5, the peak values of Ih(t) in Case A2 (A5, A6, A8), where u1(t)≠0, are less than that in Case A1 (A3, A4, A7). This, together with Figure 3, indicate that slaughter (i.e., the measure u1(t)) to poultry population can suppress the outbreak of avian influenza in both poultry and human populations, which is because poultry population is the critical source of avian influenza virus. Figure 6 gives the trajectories of Rh(t), which also shows that the control measures can raise the individual number of Rh(t). From (3.10)–(3.12), we know that the values of u1(t),u2(t) and u3(t) may be negative, which is unrealistic. Thus, when ui(t)(i=1,2,3) are negative, we take their values as zero, and the trajectories of them are shown in Figures 7–9.
Remark 3. As stated in Section 2, u1(t) represents slaughter rate to susceptible and infected poultry. Therefore, the value of u1(t) can be determined according to the slaughtering range, for example, u1(t)=0.05 means the epidemic can be controlled if 5% of the total number of poultry are slaughtered, which is exactly the total number of poultry in the farm where the first case occurred. u2(t) indicates the frequency of educational campaigns to susceptible humans through television, internet, loudspeakers and other medias. Thus, if we take the frequency of epidemic information broadcast by the loudspeaker (from 6 am to 10 pm (960 minutes)) as the values of u2(t), for example, u2(t)=0.008 indicates that the message is broadcasted every 8 minutes. The values of u3(t) depend on the treatment plan the doctor takes. For example, u3(t)=1 indicates that the effective rate of treatment is 100%, which is because doctors take antiviral and traditional Chinese medicine treatment in order to control the disease in a short time in the early stage of treatment (1–3 days). u3(t)=0.8 means that the effective rate of traditional Chinese medicine treatment is 80%, which can be determined by the way of taking Chinese medicine, for example, from one dose a day to one dose every two days to one dose every three days.
Example 2. The effects of uncertainty parameters.
All the parameters in Example 1 are assumed to be completely known, which are used to determine the initial values of adaptive laws of parameter vectors in (3.16)–(3.18). However, in practice, there are some errors between the obtained parameters and true ones. Therefore, we consider the tracking performance when the parameters have some deviations, which is reflected by the initial value of the adaptive laws, namely, the initial value is equal to the true value of the parameter plus a certain deviation. Let ˆθi(0)=(1+η)θi(i=1,2,3) and consider the following four cases: Case B1, η=−0.3; Case B2, η=0.0 (i.e., No deviation); Case B3, η=0.3; Case B4, η=0.6. The tracking errors in each case are shown in Figures 10–12, from which we see that the tracking error in Case B2 is closest to 0, because the true values of θi(i=1,2,3) are used. Although there exist some errors in Cases B1, B3 and B4, compared with the population size of poultry and human, the errors are very small, which are tolerable. This also indicates the robustness of the proposed controllers.
On account of slaughtering to poultry, educational campaigns to susceptible human and treatment to the infected human, we investigate the adaptive control problem of avian influenza model in this paper. First, the designed controllers can achieve the preset control goal and ensure the stability of the achieved closed-loop system. Then, the effectiveness and robustness of the designed controllers are verified by several numerical examples. The numerical results indicate that comprehensive control strategies are essential to quickly and effectively curb the spreading of avian influenza in both human and poultry population, and the slaughter to poultry population is critical to eliminate the source of avian influenza virus. At present, there are few research achievements on adaptive control of biological models, so this paper only studies adaptive control of ordinary differential avian influenza model. However, many other factors (such as time delay, random environmental noise, etc.) also affect the spread of avian influenza, so how to investigate the adaptive control of delayed avian influenza model and stochastic avian influenza model will be carried put in the future research perspectives.
The authors declare they have not used Artificial Intelligence (AI) tools in the creation of this article.
This work was supported by the Natural Science Foundation of China (12201330 and 12161068).
The authors declare there is no conflicts of interest.
[1] | Centers for Disease Control and Prevention, Seasonal Influenza (flu), Technical report, 2012. Available from: https://www.cdc.gov/flu/. |
[2] | Public Health Agency of Canada, Human Health Issues Related to Avian Influenza in Canada, Technical report, 2006. Available from: https://www.canada.ca/en/public-health/services/reports-publications/human-health-issues-related-avian-influenza.html. |
[3] |
P. Chen, J. Xie, Q. Lin, L. Zhao, Y. Zhang, H. Chen, et al., A study of the relationship between human infection with avian influenza a (H5N6) and environmental avian influenza viruses in Fujian, China, BMC Infect. Dis., 19 (2019), 762. https://doi.org/10.1186/s12879-019-4145-6 doi: 10.1186/s12879-019-4145-6
![]() |
[4] |
H. Gao, H. Lu, B. Cao, B. Du, H. Shang, J. Gan, et al., Clinical findings in 111 cases of influenza A (H7N9) virus infection, N. Engl. J. Med., 368 (2013), 2277–2285. https://doi.org/10.1056/NEJMoa1305584 doi: 10.1056/NEJMoa1305584
![]() |
[5] |
R. Gao, B. Cao, Y. Hu, Z. Feng, D. Wang, W. Hu, et al., Human infection with a novel avian-origin influenza A (H7N9) virus, N. Engl. J. Med., 368 (2013), 1888–1897. https://doi.org/ 10.1056/NEJMoa1304459 doi: 10.1056/NEJMoa1304459
![]() |
[6] |
D. Liu, W. Shi, Y. Shi, D. Wang, H. Xiao, W. Li, et al., Origin and diversity of novel avian influenza A H7N9 viruses causing human infection: phylogenetic, structural, and coalescent analyses, Lancet, 381 (2013), 1926–1932. https://doi.org/10.1016/S0140-6736(13)60938-1 doi: 10.1016/S0140-6736(13)60938-1
![]() |
[7] |
E. Claas, A. Osterhaus, R. Van-Beek, J. Jong, G. F. Rimmelzwaan, D. A. Senne, et al., Human influenza A H5N1 virus related to a highly pathogenic avian influenza virus, Lancet, 351 (1998), 472–477. https://doi.org/10.1016/S0140-6736(97)11212-0 doi: 10.1016/S0140-6736(97)11212-0
![]() |
[8] |
T. Kang, Q. Zhang, L. Rong, A delayed avian influenza model with avian slaughter: stability analysis and optimal control, Physica A, 529 (2019), 121544. https://doi.org/10.1016/j.physa.2019.121544 doi: 10.1016/j.physa.2019.121544
![]() |
[9] |
K. A. Pawelek, A. Oeldorf-Hirsch, L. Rong, Modeling the impact of twitter on influenza epidemics, Math. Biosci. Eng., 11 (2014), 1337–1356. https://doi.org/10.3934/mbe.2014.11.1337 doi: 10.3934/mbe.2014.11.1337
![]() |
[10] | World Health Organization, Human infection with avian influenza A (H7N9) virus–update, 2014. Available from: https://www.who.int/emergencies/disease-outbreak-news/item/2014_09_04_avian_influenza-en. |
[11] |
L. Chen, X. Lin, W. Guo, J. Tian, W. Wang, X. Ying, et al., Diversity and evolution of avian influenza viruses in live poultry markets, free-range poultry and wild wetland birds in China, J. Gen. Virol., 97 (2016), 844–854. https://doi.org/10.1099/jgv.0.000399 doi: 10.1099/jgv.0.000399
![]() |
[12] |
K. Shimizu, L. Wulandari, E. D. Poetranto, R. A. Setyoningrum, R. Yudhawati, A. Sholikhah, et al., Seroevidence for a high prevalence of subclinical infection with avian influenza A(H5N1) virus among workers in a livepoultry market in Indonesia, J. Infect. Dis., 214 (2016), 1929–1936. https://doi.org/10.1093/infdis/jiw478 doi: 10.1093/infdis/jiw478
![]() |
[13] |
C. Bao, L. Cui, M. Zhou, L. Hong, G. F. Gao, H. Wang, Live-animal markets and influenza A (H7N9) virus infection, N. Engl. J. Med., 368 (2013), 2337–2339. https://doi.org/10.1056/NEJMc1306100 doi: 10.1056/NEJMc1306100
![]() |
[14] |
Y. Xiao, X. Sun, S. Tang, J. Wu, Transmission potential of the novel avian influenza A(H7N9) infection in mainland China, J. Theor. Biol., 352 (2014), 1–5. https://doi.org/10.1016/j.jtbi.2014.02.038 doi: 10.1016/j.jtbi.2014.02.038
![]() |
[15] |
X. Wang, W. Cheng, Z. Yu, S. Liu, H. Mao, E. Chen, Risk factors for avian influenza virus in backyard poultry flocks and environments in Zhejiang province, China: a cross-sectional study, Infect. Dis. Poverty, 7 (2018), 65. https://doi.org/10.1186/s40249-018-0445-0 doi: 10.1186/s40249-018-0445-0
![]() |
[16] |
E. Jung, S. Iwami, Y. Takeuchi, T. Jo, Optimal control strategy for prevention of avian influenza pandemic, J. Theor. Biol., 260 (2009), 220–229. https://doi.org/10.1016/j.jtbi.2009.05.031 doi: 10.1016/j.jtbi.2009.05.031
![]() |
[17] |
T. Kang, Q. Zhang, H. Wang, Optimal control of an avian influenza model with multiple time delays in state and control variables, Discrete Contin. Dyn. Syst. - Ser. B, 26 (2021), 4147–4171. https://doi.org/10.3934/dcdsb.2020278 doi: 10.3934/dcdsb.2020278
![]() |
[18] |
S. Sharma, A. Mondal, A. Pal, G. P. Samanta, Stability analysis and optimal control of avian influenza virus A with time delays, Int. J. Dyn. Control, 6 (2018), 1351–1366. https://doi.org/10.1007/s40435-017-0379-6 doi: 10.1007/s40435-017-0379-6
![]() |
[19] |
B. Cao, X. Nie, Event-triggered adaptive neural networks control for fractional-order nonstrict-feedback nonlinear systems with unmodeled dynamics and input saturation, Neural Networks, 142 (2021), 288–302. https://doi.org/10.1016/j.neunet.2021.05.014 doi: 10.1016/j.neunet.2021.05.014
![]() |
[20] |
B. Cao, X. Nie, Z. Wu, C. Xue, J. Cao, Adaptive neural network control for nonstrict-feedback uncertain nonlinear systems with input delay and asymmetric time-varying state constraints, J. Franklin Inst., 358 (2021), 7073–7095. https://doi.org/10.1016/j.jfranklin.2021.07.020 doi: 10.1016/j.jfranklin.2021.07.020
![]() |
[21] |
B. Cao, T. Kang, Nonlinear adaptive control of COVID-19 with media campaigns and treatment, Biochem. Biophys. Res. Commun., 555 (2021), 202–209. https://doi.org/10.1016/j.bbrc.2020.12.105 doi: 10.1016/j.bbrc.2020.12.105
![]() |
[22] |
H. Moradi, M. Sharifi, G. Vossoughi, Adaptive robust control of cancer chemotherapy in the presence of parametric uncertainties: a comparison between three hypotheses, Comput. Biol. Med., 56 (2015), 145–157. https://doi.org/10.1016/j.compbiomed.2014.11.002 doi: 10.1016/j.compbiomed.2014.11.002
![]() |
[23] |
M. H. Nematollahi, R. Vatankhah, M. Sharifi, Nonlinear adaptive control of tuberculosis with consideration of the risk of endogenous reactivation and exogenous reinfection, J. Theor. Biol., 486 (2020), 110081. https://doi.org/10.1016/j.jtbi.2019.110081 doi: 10.1016/j.jtbi.2019.110081
![]() |
[24] |
Y. Hayama, Y. Kimura, T. Yamamoto, S. Kobayashi, T, Tsutsui, Potential risk associated with animal culling and disposal during the foot-and-mouth disease epidemic in Japan in 2010, Res. Vet. Sci., 102 (2015), 228–230. https://doi.org/10.1016/j.rvsc.2015.08.017 doi: 10.1016/j.rvsc.2015.08.017
![]() |
[25] |
B. Barnes, A. Scott, M. Hernandez-Jover, J. A. Toribio, B. Moloney, K. Glass, Modelling high pathogenic avian influenza outbreaks in the commercial poultry industry, Theor. Popul Biol., 126 (2019), 59–71. https://doi.org/10.1016/j.tpb.2019.02.004 doi: 10.1016/j.tpb.2019.02.004
![]() |
[26] |
A. Wang, Y. Xiao, A Filippov system describing media effects on the spread of infectious diseases, Nonlinear Anal. Hybrid Syst., 11 (2014), 84–97. https://doi.org/10.1016/j.nahs.2013.06.005 doi: 10.1016/j.nahs.2013.06.005
![]() |
[27] |
X. Zhang, X. Liu, Backward bifurcation of an epidemic model with saturated treatment function, J. Math. Anal. Appl., 348 (2008), 433–443. https://doi.org/10.1016/j.jmaa.2008.07.042 doi: 10.1016/j.jmaa.2008.07.042
![]() |
[28] | J. J. E. Slotine, W. Li, Applied Nonlinear Control, Prentice Hall, Englewood Cliffs, New Jersey, 1991. |
[29] | F. Chen, J. Cui, Cross-species epidemic dynamic model of influenza, in 2016 9th International Congress on Image and Signal Processing, BioMedical Engineering and Informatics (CISP-BMEI), (2016), 1567–1572. https://doi.org/10.1109/CISP-BMEI.2016.7852965 |
[30] |
S. Liu, S. Ruan, X. Zhang, Nonlinear dynamics of avian influenza epidemic models, Math. Biosci., 283 (2017), 118–135. https://doi.org/10.1016/j.mbs.2016.11.014 doi: 10.1016/j.mbs.2016.11.014
![]() |