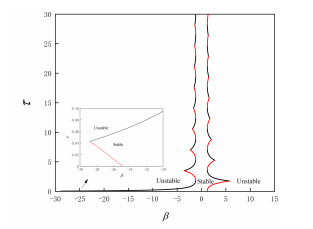
Coupled networks are common in diverse real-world systems and the dynamical properties are crucial for their function and application. This paper focuses on the behaviors of a network consisting of mutually coupled neural groups and time-delayed interactions. These interacting groups can include different sets of nodes and topological architecture, respectively. The local and global stability of the system are analyzed and the stable regions and bifurcation curves in parameter planes are obtained. Different patterns of bifurcated solutions arising from trivial and non-trivial equilibrium points are given, such as the coexistence of non-trivial equilibrium points and periodic responses and multiple coexisting periodic orbits. The bifurcation diagrams are shown and plenty of complex dynamic phenomena are observed, such as multi-period oscillations and multiple coexisting attractors.
Citation: Xiaochen Mao, Weijie Ding, Xiangyu Zhou, Song Wang, Xingyong Li. Complexity in time-delay networks of multiple interacting neural groups[J]. Electronic Research Archive, 2021, 29(5): 2973-2985. doi: 10.3934/era.2021022
[1] | Xiaochen Mao, Weijie Ding, Xiangyu Zhou, Song Wang, Xingyong Li . Complexity in time-delay networks of multiple interacting neural groups. Electronic Research Archive, 2021, 29(5): 2973-2985. doi: 10.3934/era.2021022 |
[2] | Weijie Ding, Xiaochen Mao, Lei Qiao, Mingjie Guan, Minqiang Shao . Delay-induced instability and oscillations in a multiplex neural system with Fitzhugh-Nagumo networks. Electronic Research Archive, 2022, 30(3): 1075-1086. doi: 10.3934/era.2022057 |
[3] | Jianmin Hou, Quansheng Liu, Hongwei Yang, Lixin Wang, Yuanhong Bi . Stability and bifurcation analyses of p53 gene regulatory network with time delay. Electronic Research Archive, 2022, 30(3): 850-873. doi: 10.3934/era.2022045 |
[4] | Fang Yan, Changyong Dai, Haihong Liu . Oscillatory dynamics of p53 pathway in etoposide sensitive and resistant cell lines. Electronic Research Archive, 2022, 30(6): 2075-2108. doi: 10.3934/era.2022105 |
[5] | Xin Du, Quansheng Liu, Yuanhong Bi . Bifurcation analysis of a two–dimensional p53 gene regulatory network without and with time delay. Electronic Research Archive, 2024, 32(1): 293-316. doi: 10.3934/era.2024014 |
[6] | Shuang Liu, Tianwei Xu, Qingyun Wang . Effect analysis of pinning and impulsive selection for finite-time synchronization of delayed complex-valued neural networks. Electronic Research Archive, 2025, 33(3): 1792-1811. doi: 10.3934/era.2025081 |
[7] | Xudong Hai, Chengxu Chen, Qingyun Wang, Xiaowei Ding, Zhaoying Ye, Yongguang Yu . Effect of time-varying delays' dynamic characteristics on the stability of Hopfield neural networks. Electronic Research Archive, 2025, 33(3): 1207-1230. doi: 10.3934/era.2025054 |
[8] | Xiaochun Gu, Fang Han, Zhijie Wang, Kaleem Kashif, Wenlian Lu . Enhancement of gamma oscillations in E/I neural networks by increase of difference between external inputs. Electronic Research Archive, 2021, 29(5): 3227-3241. doi: 10.3934/era.2021035 |
[9] | Xiaowen Zhang, Wufei Huang, Jiaxin Ma, Ruizhi Yang . Hopf bifurcation analysis in a delayed diffusive predator-prey system with nonlocal competition and schooling behavior. Electronic Research Archive, 2022, 30(7): 2510-2523. doi: 10.3934/era.2022128 |
[10] | Minglei Fang, Jinzhi Liu, Wei Wang . Finite-/fixed-time synchronization of leakage and discrete delayed Hopfield neural networks with diffusion effects. Electronic Research Archive, 2023, 31(7): 4088-4101. doi: 10.3934/era.2023208 |
Coupled networks are common in diverse real-world systems and the dynamical properties are crucial for their function and application. This paper focuses on the behaviors of a network consisting of mutually coupled neural groups and time-delayed interactions. These interacting groups can include different sets of nodes and topological architecture, respectively. The local and global stability of the system are analyzed and the stable regions and bifurcation curves in parameter planes are obtained. Different patterns of bifurcated solutions arising from trivial and non-trivial equilibrium points are given, such as the coexistence of non-trivial equilibrium points and periodic responses and multiple coexisting periodic orbits. The bifurcation diagrams are shown and plenty of complex dynamic phenomena are observed, such as multi-period oscillations and multiple coexisting attractors.
The last few years have witnessed the rapid development of multiplex networks due to their important and extensive applications in many fields, such as brain, Internet, power grids, food webs, social networks, transport networks, etc[1,2,3,10,13,15]. Multiplex networks consist of interacting individual sub-networks with couplings between them. For example, in the brain, neurons are often lumped into connected groups and the interactions of them are crucial for the proper functioning of the brain[1]. Due to the finite propagation speeds of signals, time delays are inevitable phenomena in mulitplex networks[13,15]. For example, axonal conduction could result in time delays up to milliseconds for the singnal propagation between areas in the brain. As a matter of fact, neglecting time delays usually leads to false or even wrong results[6,9,14].
The analysis of the dynamics of multiplex networks has close relationships with their practical applications. In biological science, for example, the network of coupled cells (each cell is composed of a set of neurons) can exhibit the quadruped gaits of walk, trot, and pace. In particular, by adjusting the coupling strengths and time delays, the primary gaits can be produced from Hopf bifurcation. Experiments show that abnormal neural synchronous oscillations in interacting neural loops are closely related to some diseases, such as schizophrenia, epilepsy, autism, Parkinson's disease and Alzheimer's disease[3,4]. Chaotic motions have been shown in neural systems, which associate with cognition, learning, and retrieval. Multi-stability indicates the possibility to have several attractors for the same parameters when the initial conditions vary. It has also been observed in single neuron and neuronal populations. For instance, in cortical neurons, the transitions between spiking and bursting corresponded to a function of sleep and wakefulness[5].
In interacting networks, the dynamic processes happening in one network may vitally affect another network since a node in one network is likely part of another network[10]. The interplay of networks can lead to plenty of interesting dynamical features. For example, the couplings can provide a means for driving and modulating of sustainable oscillations. Actually, the behaviors of multiplex networks are often different from those observed in isolated network. Time delays, even very short in some cases, have significant influences on the properties of systems and cannot be simply neglected in the dynamical analysis. It is found that time delay can be used as a powerful tool to control various dynamical features, such as synchronization patterns and their transitions, quasi-periodic and chaotic motions, chimera states, et al[6,9,11,14]. For instance, in a two-ring network, the delayed interactions induce complicated waves and the appropriate time delay and transverse coupling can achieve the "backup and recovery" procedure[15]. Thus, the performances of multiplex networks are governed by the assumed dynamics of individual sub-network, their reciprocal interactions and time delays.
Multiplex structures are ubiquitous in biological and physiological systems, such as neural networks. In recent years, the dynamics of multiplex neural networks with time delays have drawn much attention. Nikitin et al.[12] investigated the spatio-temporal dynamics of a multiplex network with two different neuronal loops and delayed inter-layer couplings and revealed coexisting partial synchronization patterns of the two-layer network and presented effective control schemes. Sawicki et al.[13] studied the relay synchronization in a three-layer multiplex FitzHugh-Nagumo neural network with nonlocal coupling topologies within the layers and considered time delay in the interlayer coupling. For more related studies on the dynamics of multiplex neural networks with time delays, the reader is referred to the work in[3,8,15], and the references cited therein. However, few efforts are made on multiple coupled neural networks with time delays. In neural systems, many brain areas, consisting of neural assemblies, are coupled by physical or functional interactions among them. In functional brain networks, for instance, each link indicates a functional interaction between the activity (electrical, magnetic, or hemodynamic/metabolic) of two areas [1]. Particularly, some specific brain areas act as relays between other brain regions, playing important roles in brain functionality and dysfunctions, such as parahippocampal regions[13].
Motivated by the above discussions, the purpose of this paper is to study the dynamical behaviors of a multiplex network that consists of multiple Hopfield neural groups and time delays. Each sub-network can contain different sets of neurons and topological structures. The multiplex network is connected by the couplings between one neuron of each sub-network. The intra-layer time delays are neglected compared with the internal time scale of the neural system, while the inter-layer coupling time delay is larger and cannot be neglected. Then, different time delays are introduced into the couplings between networks.
The rest of this paper is organized as follows. In Section 2, the model of the network is presented and the stability and bifurcations of the network are analyzed. Case studies of illustrative examples are given to support the theoretical results and abundant neural activities are shown in Section 3. Finally, conclusions are drawn in Section 4.
The multiplex network consists of multiple interacting sub-networks with delayed couplings, which can be described by the following delay differential equations
{.up(t)=−up(t)+N1∑q=1bpqf(uq),1≤p≤N1−1.up(t)=−up(t)+N1∑q=1bpqf(uq)+cmg(uNm(t−τm)),p=N1.up(t)=−up(t)+N2∑q=N1+1bpqf(uq),N1+1≤p≤N2−1.up(t)=−up(t)+N2∑q=N1+1bpqf(uq)+c1g(uN1(t−τ1)),p=N2.......up(t)=−up(t)+Nm∑q=Nm−1+1bpqf(uq),Nm−1+1≤p≤Nm−1.up(t)=−up(t)+Nm∑q=Nm−1+1bpqf(uq)+cm−1g(uNm−1(t−τm−1)),p=Nm | (1) |
where
.u(t)=−u(t)+Au(t)+β1L1u(t−τ1)+β2L2u(t−τ2)+⋯+βmLmu(t−τm) | (2) |
where
The characteristic matrix of the system is
M(λ,τ1,τ2,…,τm)=[λI1−A10⋯0−Cme−λτm−C1e−λτ1λI2−A2⋯00⋮⋮⋱⋮⋮00⋯λIm−1−Am−1000⋯−Cm−1e−λτm−1λIm−Am] |
where
Δ(λ,τ)=m∏k=1|(λ+1)Ik−Ak|−βe−λτm∏k=1|(λ+1)˜Ik−˜Ak|=m∏k=1nk∏h=1(λ−sk,h)−βe−λτm∏k=1nk−1∏l=1(λ−˜sk,l)=m∏k=1Gk(λ)−βe−λτm∏k=1Hk(λ)=G(λ)−βe−λτH(λ)=0 | (3) |
where
Δ(λ,0)=G(λ)−βH(λ)=∏(λ−sk,h)−β∏(λ−˜sk,l)=λn+g1λn−1+g2λn−2+⋯+(gm−β)λn−m+⋯ |
+(gn−1−βhn−m−1)λ+gn−βhn−m=λn+e1λn−1+e2λn−2+⋯+en−1λ+en | (4) |
where
Δ(iω,τ)=m∏k=1Gk(iω)−βe−iωτm∏k=1Hk(iω)=GR(ω)+iGI(ω)−β[HR(ω)+iHI(ω)][cos(ωτ)−isin(ωτ)]=0 | (5) |
where
Based on the above results, the stability of the trivial equilibrium of the network can be obtained by the characteristic values
Let
Let
Δ(λ,τ)=[G1(λ)−β1e−λτsH1(λ)][G1(λ)+β1e−λτsH1(λ)][G21(λ)+β21e−2λτsH21(λ)]=Δ1(λ,τ)Δ2(λ,τ)Δ3(λ,τ)Δ4(λ,τ)=0 |
where
As shown in Figure 2, there exists only delay-independent stability region, where the trivial equilibrium is always locally stabile for all time delays. In particular, one can check that the distribution of the characteristic roots of the four-coupled network is the same as the two-coupled model consisting of a pair of bidirectional rings. Then, the grey region shown in Figure 2 is also the stable area of the two-coupled ring neural networks.
In neural systems, due to the input-output relation of neurons, the activation functions
where
(1) Let
(2)
Figure 5(a) shows that two non-trivial equilibria (0, 0, 0, 0.553, -0.553, 0, 0, 0, 0) and (0, 0, 0, -0.553, 0.553, 0, 0, 0, 0) come into being when
(3)
Figure 11 depicts the detailed bifurcation diagrams on the Poincaré section as the function of the coupling time delays under different initial conditions. The points on Poincaré section depend on the behaviors of the system. If the final motion of the system is periodic, there is only one point in the Poincaré section. For a period-n motion, n points will appear in the section, but the numbers of points become infinite for non-periodic motions such as chaotic responses. The Poincaré sections are defined by
In biological and physiological systems, multiplex networks often represent neurons in different areas of the brain. In this paper, the stability, bifurcations and complicated oscillations of a multiplex neural network with multiple Hopfield-type neural sub-networks and delayed couplings are studied. Each sub-network contains different sets of nodes and topologies. Time delays are considered in the couplings between sub-networks. The delay-independent and delay-dependent stability of the trivial equilibrium of the multiplex network are discussed by decomposing and analyzing the associated characteristic equation. It is shown that the stability of the network is determined by the root distributions of the characteristic equations of the isolated sub-networks and the product of coupling strengths and the sum of time delays. The regions indicating the stability and instability of the network in the parameter planes are shown. Different types of bifurcations are analyzed, such as steady state bifurcation, Hopf bifurcation and the interaction of them. The coexistence of two non-trivial equilibrium points and periodic oscillations and three coexisting periodic oscillations are obtained. By taking the coupling time delay as parameter, the bifurcation diagrams on Poincaré section are given and interesting dynamical phenomena are shown, such as the coexistence of different patterns of multi-period orbits and strange attractors. The revealed results in this paper can provide promising information for understanding the mechanisms of rhythms, complicated firing patterns, and multi-stability in large-scare complex neural systems.
The authors thank the anonymous reviewers for their helpful comments and suggestions that have helped to improve the presentation.
[1] |
F. Battiston, V. Nicosia, M. Chavez and V. Latora, Multilayer motif analysis of brain networks, Chaos, 27 (2017), 047404, 8 pp. doi: 10.1063/1.4979282
![]() |
[2] |
The structure and dynamics of multilayer networks. Phys. Rep. (2014) 544: 1-122. ![]() |
[3] |
Delayed coupling between two neural network loops. SIAM J. Appl. Math. (2004) 65: 316-335. ![]() |
[4] | Improving control effects of absence seizures using single-pulse alternately resetting stimulation (SARS) of corticothalamic circuit. Appl. Math. Mech. (Eng. Edit.) (2020) 41: 1287-1302. |
[5] | F. Frohlich and M. Bazhenov, Coexistence of tonic firing and bursting in cortical neurons, Phys. Rev. E, 74 (2006), 031922. |
[6] | Robust synchronization of bursting Hodgkin-Huxley neuronal systems coupled by delayed chemical synapses. Int. J. Nonlinear Mech. (2015) 70: 105-111. |
[7] | Neurons with graded response have collective computational properties like those of two-state neurons. Proc. Nati. Acad. Sci. USA (1984) 81: 3088-3092. |
[8] |
Periodic oscillations arising and death in delay-coupled neural loops. Internat. J. Bifur. Chaos Appl. Sci. Engrg. (2007) 17: 4015-4032. ![]() |
[9] |
H. Y. Hu and Z. H. Wang, Dynamics of Controlled Mechanical Systems with Delayed Feedback, Springer-Verlag, Heidelberg, 2002. doi: 10.1007/978-3-662-05030-9
![]() |
[10] | S. Majhi, M. Perc and D. Ghosh, Chimera states in uncoupled neurons induced by a multilayer structure, Sci. Rep., 6 (2016), 39033. |
[11] |
X. Mao, X. Li, W. Ding, S. Wang, X. Zhou and L. Qiao, Dynamics of a multiplex neural network with delayed couplings, Appl. Math. Mech. (Eng. Edit.), (2021). doi: 10.1007/s10483-021-2709-6
![]() |
[12] |
D. Nikitin, I. Omelchenko, A. Zakharova, M. Avetyan, A. L. Fradkov and E. Schöll, Complex partial synchronization patterns in networks of delay-coupled neurons, Philos. Trans. Roy. Soc. A, 377 (2019), 20180128, 19 pp. doi: 10.1098/rsta.2018.0128
![]() |
[13] | J. Sawicki, I. Omelchenko, A. Zakharova and E. Schoell, Delay controls chimera relay synchronization in multiplex networks, Phys. Rev. E, 98 (2018), 062224. |
[14] |
Z. Wang, S. Liang, C. A. Molnar, T. Insperger and G. Stepan, Parametric continuation algorithm for time-delay systems and bifurcation caused by multiple characteristic roots, Nonlinear Dynam., (2020). doi: 10.1007/s11071-020-05799-w
![]() |
[15] |
Inter-layer synchronization of periodic solutions in two coupled rings with time delay. Physica D (2019) 396: 1-11. ![]() |
1. | Moutian Liu, Lixia Duan, In-phase and anti-phase spikes synchronization within mixed Bursters of the pre-Bözinger complex, 2022, 30, 2688-1594, 961, 10.3934/era.2022050 | |
2. | Keiji Konishi, Koki Yoshida, Yoshiki Sugitani, Naoyuki Hara, Delay-induced amplitude death in multiplex oscillator network with frequency-mismatched layers, 2024, 109, 2470-0045, 10.1103/PhysRevE.109.014220 |