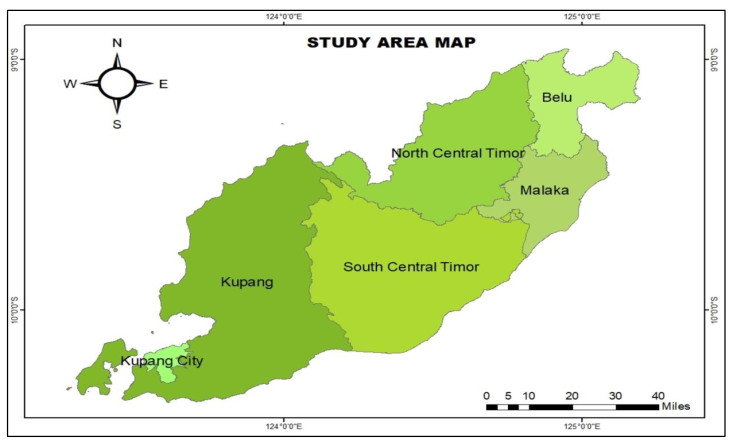
In recent decades, abnormal rainfall and temperature patterns have significantly impacted the environment and human life, particularly in East Nusa Tenggara. The region is known for its low rainfall and high temperatures, making it vulnerable to drought events, which have their own complexities due to being random and changing over time. This study aimed to analyze the trend of short-term meteorological drought intensity in Timor Island, East Nusa Tenggara. The analysis was carried out by utilizing the standardized precipitation evapotranspiration index (SPEI) for a 1-month period to characterize drought in intensity, duration, and severity. A power law process approach was used to model the intensity of the event, which is inversely proportional to the magnitude of the drought event. Intensity parameters of the power law process were estimated using the maximum likelihood estimation (MLE) method to predict an increase in the intensity of drought events in the future. The probability of drought was calculated using the non-homogeneous Poisson process. The analysis showed that "extremely dry" events in Timor Island are less frequent than "very dry" and "dry" events. The power law process model's estimated intensity parameter showed a beta value greater than 1, indicating an increase in future drought events. In the next 12 months, two months of drought are expected in each region of Timor Island, East Nusa Tenggara, with the following probabilities for each region: 0.264 for Kupang City, 0.25 for Kupang, 0.265 for South Central Timor, 0.269 for North Central Timor, 0.265 for Malaka, and 0.266 for Belu. This research provides important insights into drought dynamics in vulnerable regions such as East Nusa Tenggara and its potential impact on future mitigation and adaptation planning.
Citation: Nur Hikmah Auliana, Nurtiti Sunusi, Erna Tri Herdiani. Analysis of meteorological drought periods based on the Standardized Precipitation Evapotranspiration Index (SPEI) using the Power Law Process approach[J]. AIMS Environmental Science, 2024, 11(5): 682-702. doi: 10.3934/environsci.2024034
[1] | Kaoutar MOUNIR, Isabelle LA JEUNESSE, Haykel SELLAMI, Abdessalam ELKHANCHOUFI . Spatiotemporal analysis of drought occurrence in the Ouergha catchment, Morocco. AIMS Environmental Science, 2023, 10(3): 398-423. doi: 10.3934/environsci.2023023 |
[2] | Zinabu A. Alemu, Emmanuel C. Dioha, Michael O. Dioha . Hydro-meteorological drought in Addis Ababa: A characterization study. AIMS Environmental Science, 2021, 8(2): 148-168. doi: 10.3934/environsci.2021011 |
[3] | Carolyn Payus, Siti Irbah Anuar, Fuei Pien Chee, Muhammad Izzuddin Rumaling, Agoes Soegianto . 2019 Southeast Asia Transboundary Haze and its Influence on Particulate Matter Variations: A Case Study in Kota Kinabalu, Sabah. AIMS Environmental Science, 2023, 10(4): 547-558. doi: 10.3934/environsci.2023031 |
[4] | Joseph A. Kazery, Dayakar P. Nittala, H. Anwar Ahmad . Meteorological effects on trace element solubility in Mississippi coastal wetlands. AIMS Environmental Science, 2019, 6(1): 1-13. doi: 10.3934/environsci.2019.1.1 |
[5] | Suhail Mahmud, Md Al Masum Bhuiyan, Nusrat Sarmin, Sanjida Elahee . Study of wind speed and relative humidity using stochastic technique in a semi-arid climate region. AIMS Environmental Science, 2020, 7(2): 156-173. doi: 10.3934/environsci.2020010 |
[6] | Tuan Syaripah Najihah, Mohd Hafiz Ibrahim, Nurul Amalina Mohd Zain, Rosimah Nulit, Puteri Edaroyati Megat Wahab . Activity of the oil palm seedlings exposed to a different rate of potassium fertilizer under water stress condition. AIMS Environmental Science, 2020, 7(1): 46-68. doi: 10.3934/environsci.2020004 |
[7] | Abebe Kebede Habtegebreal, Abebaw Bizuneh Alemu, U. Jaya Prakash Raju . Examining the Role of Quasi-biennial Oscillation on Rainfall patterns over Upper Blue Nile Basin of Ethiopia. AIMS Environmental Science, 2021, 8(3): 190-203. doi: 10.3934/environsci.2021013 |
[8] | John R. Christy . Examination of Extreme Rainfall Events in Two Regions of the United States since the 19th Century. AIMS Environmental Science, 2019, 6(2): 109-126. doi: 10.3934/environsci.2019.2.109 |
[9] | Khaled Hazaymeh, Quazi K. Hassan . Remote sensing of agricultural drought monitoring: A state of art review. AIMS Environmental Science, 2016, 3(4): 604-630. doi: 10.3934/environsci.2016.4.604 |
[10] | Volodymyr Ivanov, Viktor Stabnikov, Chen Hong Guo, Olena Stabnikova, Zubair Ahmed, In S. Kim, and Eng-Ban Shuy . Wastewater engineering applications of BioIronTech process based on the biogeochemical cycle of iron bioreduction and (bio)oxidation. AIMS Environmental Science, 2014, 1(2): 53-66. doi: 10.3934/environsci.2014.2.53 |
In recent decades, abnormal rainfall and temperature patterns have significantly impacted the environment and human life, particularly in East Nusa Tenggara. The region is known for its low rainfall and high temperatures, making it vulnerable to drought events, which have their own complexities due to being random and changing over time. This study aimed to analyze the trend of short-term meteorological drought intensity in Timor Island, East Nusa Tenggara. The analysis was carried out by utilizing the standardized precipitation evapotranspiration index (SPEI) for a 1-month period to characterize drought in intensity, duration, and severity. A power law process approach was used to model the intensity of the event, which is inversely proportional to the magnitude of the drought event. Intensity parameters of the power law process were estimated using the maximum likelihood estimation (MLE) method to predict an increase in the intensity of drought events in the future. The probability of drought was calculated using the non-homogeneous Poisson process. The analysis showed that "extremely dry" events in Timor Island are less frequent than "very dry" and "dry" events. The power law process model's estimated intensity parameter showed a beta value greater than 1, indicating an increase in future drought events. In the next 12 months, two months of drought are expected in each region of Timor Island, East Nusa Tenggara, with the following probabilities for each region: 0.264 for Kupang City, 0.25 for Kupang, 0.265 for South Central Timor, 0.269 for North Central Timor, 0.265 for Malaka, and 0.266 for Belu. This research provides important insights into drought dynamics in vulnerable regions such as East Nusa Tenggara and its potential impact on future mitigation and adaptation planning.
The recent abnormal behavior of rainfall and air temperature has caused great impacts on the environment and human life. One of the most significant impacts is the unpredictable changes in seasonal patterns. In some areas, high rainfall will cause flooding; in others, low rainfall with high air temperatures will result in drought. Drought prediction is one of the biggest challenges for scientists and hydrologists, mainly due to its complex nature: these events are random and can fluctuate over time [1]. In predicting drought, the accuracy of rainfall data is crucial because rainfall is the main factor determining water availability in a region [2]. In addition, air temperature, which affects the rate of evaporation, also plays an important role in modeling and predicting drought phenomena [3,4,5].
Drought is one of the most devastating natural disasters, impacting water supply, agriculture, energy production, ecosystems, and society [6]. Drought has affected many parts of the world over the past few decades, such as in the Southeast United States [7], China [8,9], Brazil [10], and Pakistan [11]. Drought can be classified into four categories: Meteorological drought, which is the lack of rainfall to below-normal levels in a certain period of time, hydrological drought, the lack of water availability in and on the surface of the soil, agricultural drought, the reduced yield or agricultural production due to reduced water supply, and socioeconomic drought, related to demand and supply in a market for goods of economic value [12].
The measurement tool for drought is called the drought index, a single value that can describe the severity of drought. Meteorological drought indices that can be used to monitor drought conditions include the Palmer drought severity index (PDSI), which uses the water balance equation in the soil [13], and the standardized precipitation index (SPI) using the rainfall probability approach [14]. In recent years, new drought indices have been developed to improve the effectiveness of existing ones. One of these developments is the standardized precipitation evapotranspiration index (SPEI). The SPEI is a development of the SPI that only considers rainfall. SPEI adds potential evapotranspiration parameters in its calculation to describe drought better than relying on rainfall alone. This is a response to climate change and its effect on drought [15]. The time scales of SPEI calculation are the same as the SPI: The 1-month period is used for short-term drought recognition, the 3- and 6-month periods are used for seasonal drought recognition, the 12-month period is used for medium-term drought, and the 24- and 48-month periods are used for long-term drought assessment [14].
Drought monitoring using the 1-month SPEI drought index in Timor Island, East Nusa Tenggara, has shown that drought events in Kupang City spanned 94 months, with different intensity classifications: 63 months experienced "moderately dry" levels, 25 months had "severely dry" levels, and 6 months reached "extremely dry" levels. Meanwhile, in Kupang Regency, there were 93 months of drought, 62 months at the "moderately dry" level, 26 months at the "severely dry" level, and 5 months reaching the "extremely dry" level. South Central Timor Regency recorded 90 months of drought intensity, with 59 months at the "moderately dry" level, 25 months at the "severely dry" level, and 6 months reaching the "extremely dry" level. In North Central Timor Regency, 88 months of drought occurred, with 62 months at the "moderately dry" level, 20 months at the "severely dry" level, and 6 months at the "extremely dry" level. Malaka Regency recorded 95 months of drought, with 66 months at the "moderately dry" level, 25 months at the "severely dry" level, and 4 months at the "extremely dry" level. In Belu Regency, there were 87 months of drought, with 59 months at the "moderately dry" level, 23 months at the "severely dry" level, and 5 months at the "extremely dry" level.
The distribution of drought intensity on Timor Island, classified based on the 1-month SPEI drought level in each observation area, shows a very significant variation. In addition, there is a natural trend where more severe drought events, namely "extremely dry" and "very dry" events, tend to occur less frequently than less severe events, such as normal drought. In a stochastic process, this trend can be interpreted as a power law where the intensity of drought events decreases as their severity increases. The power law reflects that very severe drought events have a lower probability of occurrence than milder drought events. The large fluctuations in the tail of the power law distribution, which includes extreme but rare events, indicate that extreme events have a very low probability but can occur with very large intensity [16]. The power law process is one of the case models of the non-homogeneous Poisson process with the intensity function of the form (βγ)(tγ)(β−1) [17].
The non-homogeneous Poisson process is a commonly used model to model the number of events as a function of time [18]. Special case models of the non-homogeneous Poisson process have been widely used in various disciplines, including hydrometeorology, as shown in the study of Achcar et al. [19], where non-homogeneous Poisson process models, namely Weibull and Goel Okumoto with multiple variable points, were used to estimate the number of ozone levels exceeding the standard limit in Mexico City. Another study by Achar et al. [20] used the non-homogeneous Poisson process at the change point with the power law process model to analyze the drought period based on the SPI in Brazil. Ellahi et al. [21] used the non-homogeneous Poisson process model with a linear intensity function to assess the number of hydrological drought events using the SPI in Pakistan.
In addition, research on drought prediction using the SPEI index has also been carried out in many parts of the world. Ghasemi et al. [22] and Karbasi et al. [23] forecasted the SPEI 12 drought index in Iran; Dikshit et al. [24] predicted the size of drought using the SPEI on two different time scales (SPEI 1 and SPEI 3) in the New South Wales region, Australia; Affandy et al. [25] modeled and predicted meteorological drought measured by the SPEI with a time range of 1, 3, 6, and 12 months in Lamongan Regency, Indonesia.
The power law process can occur in various natural and artificial phenomena, covering several fields of science such as biology, economics, physics, chemistry, and computer science [26,27,28,29,30]. Statistical inference for the power law process is generally based on the maximum likelihood estimator (MLE) and its asymptotic properties. The MLE is used to find the parameter values in the power law process model that are most likely to yield the observed data [31]. A special characteristic of the power law process is the estimated value of the shape parameter (β), which can describe how an intensity can increase or decrease. If β>1, the intensity of an event will increase; if β<1, the intensity of an event will decrease; and if β=1, the power law process reduces to a homogeneous Poisson process [17]. Two categories of data cases can be applied for parameter estimation in the power law process model: the time interval between events and the number of events observed in the specified interval [32]. In this study, the estimation method is applied to the second category, where the number of drought events based on 1-month SPEI in Timor Island is considered a random variable with a predetermined observed time interval. The fit test results using the acute Cramér-von Mises test showed that the intensity of drought events based on the 1-month SPEI on Timor Island fits the power law process model. The parameter estimation of the power law process using MLE in each observation area shows (β)>1, with Kupang City having a value of 1.063; Kupang Regency 1.174; South Central Timor Regency 1.095; North Central Timor Regency 1.049; Malaka Regency 1.034, and Belu Regency 1.112. This indicates a possible increase in the intensity of drought events in these areas. Therefore, as a mitigation effort and early planning in the face of future drought events, this study aims to analyze short-term meteorological drought periods using the power law process to obtain an estimate of the duration of future drought events.
Figure 1 shows the area of study was conducted in the Timor Island region of East Nusa Tenggara (NTT), Indonesia, covering six regency/cities, namely Kupang City, Kupang Regency, South Central Timor Regency, North Central Timor Regency, Malaka Regency, and Belu Regency. Geographically, the six locations are located in the western part of the Timor Archipelago with coordinates of 9°14' N and 124°56' E. The data used are secondary in the form of monthly rainfall amounts and monthly average air temperatures obtained from NASA Power through the website https://power.larc.nasa.gov/data-access. Data were used as input data for the SPEI 1-month drought index calculation parameters These results were classified based on drought severity to obtain the intensity of drought events in each observation area. The intensity of drought events was measured based on the frequency of drought periods within a time span. The observation period was from January 1981 to December 2023, with a record length of 516 months.
The SPEI was designed to consider rainfall and potential evapotranspiration (PET) in determining drought. The SPEI drought index calculation is based on the deficit value between rainfall and PET [15]. PET can be calculated by the Thornthwaite method using average air temperature based on the following equation [33]:
PET=16K(10TI)m | (1) |
K is a correction factor based on the latitude position of the observation area, T is the monthly average air temperature (∘C), and I is the annual internal heat index obtained from the sum of i for 12 months in the following equation:
i=(T5)1,514andI=∑121i | (2) |
The m is a coefficient that is a variable tied to I with the equation given by:
m=6,75×10−7I3−7,71×10−5I2+1,792×10−2I+0.492 | (3) |
The deficit between rainfall and PET or climate water balance can be determined by the following equation:
Di=CHi−PETi | (4) |
Di is the value of climate water balance in month i, CHi is the amount of rainfall in month i, and PETi is PET in month i in mm. Next, the value of Di is standardized based on the probability density function of the log-logistic distribution with three parameters to capture the deficit value, since it is likely that the moisture deficit in arid and semi-arid areas may be negative. For the two-parameter distribution as used in SPI, the variable D has a lower limit of zero (0>D<∞), which means D can only take positive values, while for the three-parameter distribution used in SPEI, D can take values in the range (γ>D<∞), which means D can also take negative values [15]. The probability density function of the log-logistic distribution is given as:
f(D)=βα(D−γα)β−1[1+(D−γα)β]−2 | (5) |
The parameters α, β, γ in the log-logistic distribution are calculated using the L-moment procedure. L-moment calculation of Pearson Ⅲ distribution parameters can be obtained through the following equation [34]:
β=2W1−W06W1−W0−6W2 | (6) |
α=(W0−2W1)βГ(1+1β)Г(1−1β) | (7) |
γ=W0−αГ(1+1β)Г(1−1β) | (8) |
Г(β) is the gamma distribution function of β. W is the probability weighted moments (PWMs) obtained from the following equation:
Ws=1N∑Ni=1(1−Fi)sDi | (9) |
s is the number of PWMs, and Fi is a frequency estimator that can be calculated using the equation given by:
Fi=i−0,35N | (10) |
i is the range of observations arranged in ascending order and N is the number of data used. The probability function of the distribution D over various time scales can be calculated using the following equation:
F(D)=[1+(αD−γ)β]−1 | (11) |
Based on the probability function, the SPEI can be calculated using the following equation [35]:
SPEI=(t−c0+c1W+c2W21+d1W+d2W2+d3W3) | (12) |
W=√−2ln(P) for P ≤0.5 | (13) |
W=√−2ln(1−P) for P ≥0.5 | (14) |
P is the probability of exceeding the value of D, which is determined by the following equation:
P=1−F(x) | (15) |
The coefficient value of McKee is as follows:
c0=2.515517d1=1.432788c1=0.802853d2=0.189269c2=0.010328d3=0.001308 |
Drought occurs when SPEI reaches drought intensity with SPEI value ≤ –1. The classification of SPEI drought index values is based on Table 1 [36].
SPEI value | Classification |
≥2.00 | Extremely wet |
1.50–1.99 | Very wet |
1.00–1.49 | Moderately wet |
(–0.99)–0.99 | Normal |
(–1.00)–(–1.49) | Moderately dry |
(–1.50)–(–1.99) | Severely dry |
≤ –2.00 | Extremely dry |
The non-homogeneous Poisson process is a stochastic process used to count the number of events in a given time interval, where the rate of events is not constant but depends on time. A process counting {N(t),t≥0} is said [37] to be a non-homogeneous Poisson process with intensity function λ(t), t≥0, if:
a. N(0)=0,
b. {N(t),t≥0} has independent increment,
c. P{N(t+h)−N(t)=1}=λ(t)+o(h), and
d. P{N(t+h)−N(t)≥2}=o(h), where h > 0 and o(h) is a small number satisfying the condition limh→0o(h)h=0.
The expected value, also known as the cumulative function of the non-homogeneous Poisson process {N(t),t≥0} with intensity function λ(t) is defined as:
m(t)=∫t0λ(t)dt | (16) |
Based on Eq 16, the average estimate of N(t) is given by the equation:
ˆm(t)=E(N(t))=∫t0ˆλ(t)dt | (17) |
where E(.) is the expectation value. {N(t),t≥0}, modeled as a non-homogeneous Poisson process, is expressed as:
P(N(t)=n)=[∫t0λ(t)dt]n!exp−∫t0λ(t)dtn=0,1,2…n | (18) |
Based on Eq 16, for t,s>0,N(t+s)−N(t) has the following expected value function:
m(t+s)−m(t)=∫t+s0λ(t)dt | (19) |
Thus, if {N(t+s),t,s≥0}, based on Eq 18, it can be modeled as a non-homogeneous Poisson process as follows:
P(N(t+s)−N(t)=n)=(m(t+s)−m(t))nn!exp(−(m(t+s)−m(t)) | (20) |
A power law process is a special case of non-homogeneous Poisson process with intensity function given by [17]:
λ(t)=(βγ)(tγ)β−1,γ>0,β>1,t>0 | (21) |
Meanwhile, the expectation value based on Eq 16 is given by:
m(t)=(tγ)β,γ>0,β>1,t>0 | (22) |
The intensity function of the power law process can be used to estimate the event rate at a given time. This is because the shape parameter (β) can describe how an intensity can increase or decrease. If β > 1, the intensity of an event will increase: if β<1, the intensity of the event will decrease; and if β=1, the power law process reduces to a homogeneous Poisson process [17].
There are several goodness-of-fit test procedures that can be used to test the suitability of the power law process model, including Kuiper's V Test, Watson's U2 Test, Anderson-Darling A2 Test, Shapiro-Wilk Test, and Cramér-von Mises Test. The Cramér-von Mises test uses the following hypothesis:
H0: Event intensity fits the power law process model.
H1: Event intensity does not fit the power law process model.
The Cramér-von Mises test statistic is expressed based on the following equation:
C2R=112(n)+n∑i=1(¯R−2i−12n)2 | (23) |
¯R is the ratio power transformation given by Eq 29.
¯R=(tit)¯β | (24) |
¯β is the unbiased estimator given by Eq 27.
¯β=(n−2)∑ni=1ln(tti) | (25) |
The H0 decision is accepted if the calculated value of the C2R test statistic is smaller than the critical value for the Cramér-von Mises test, which means that the power law process model is appropriate. If the value of the C2R test statistic is greater than the critical value for the Cramér-von Mises test, then H0 is rejected, which means that the model is not suitable, and a more suitable model needs to be used [17].
Suppose t1,t2,t3,…,tn are mutually independent random samples from a distribution with a joint probability density function f(t1,t2,t3,…,tn;β,γ) with n representing the number of events occurring until time ti for 0<t1<t2<t3<⋯<tn. If the joint likelihood function is expressed as a function of β and γ, then the likelihood function is denoted as L(t1,t2,t3,…,tn;β,γ). The likelihood function for the parameters β and γ is given as follows [17]:
L(t1,t2,t3,…,tn;β,γ)=(n∏i=1λ(ti;β,γ))exp(−∫tn0λ(ti;β,γ)dt) | (26) |
Based on Eq 21, the likelihood function with intensity function λ(ti;β,γ) in Eq 23 is:
L(ti;β,γ)=(n∏i=1(βγ)(tiγ)β−1)exp(−∫tn0(βγ)(tγ)β−1dt) | (27) |
Based on Eq 24, the logarithmic likelihood function L(t;β,γ)=ln(L(t;β,γ)) is:
L(t;β,γ)=nln(β)−nβln(γ)+(β−1)n∑i=1ln(ti)−(tγ)β | (28) |
Furthermore, Eq 25 is derived with respect to β and γ so that the maximum likelihood estimator is obtained as follows:
ˆβ=n∑ni=1ln(tti) | (29) |
ˆγ=tn1ˆβ | (30) |
The SPEI is a drought index used to analyze meteorological drought conditions by considering the standardization of rainfall deficits with potential evapotranspiration (PET) or climate water balance. In this study, the SPEI calculation time scale used a 1-month period, which is adjusted to the needs of researchers to evaluate drought in the short term. SPEI defines a drought event as occurring when the SPEI value is below or equal to the -1 threshold, and the drought event ends when the SPEI value returns to positive. The index classifies drought levels into three main categories: dry, very dry, and extremely dry. The classification of drought levels by SPEI is based on the SPI classification table as follows [14,15,36]:
1) Moderately dry: Occurs when the SPEI value is between –1 and –1.49. This indicates mild drought that may affect water availability.
2) Severely dry: Occurs when the SPEI value is between –1.5 and –1.99. This indicates a more serious drought that can significantly impact agriculture, clean water, and ecosystems.
3) Extremely dry: Occurs when the SPEI value is below –2. This category represents the worst drought index and can cause major losses to agriculture, water availability, and the environment.
The SPEI calculation process uses Eqs 1–15. A time series plot of the calculated values of the 1-month SPEI for each observation area on Timor Island is shown in Figure 2.
Figure 2 shows that SPEI values close to 0 in each observation area indicate near-normal conditions, while positive or negative values indicate above or below-normal conditions. There are many negative SPEI values lower than or equal to –1, indicating that there are frequent droughts at dry, very dry, and extremely dry levels in each observation area of Timor Island. This result aligns with the research of Kuswanto et al. [38], which shows that very dry events are more common in the eastern region of NTT; in this case, Timor Island is the eastern region of NTT.
Furthermore, SPEI values lower than or equal to –1 were characterized to obtain drought intensity, duration, and severity during the observation period [14]. The characterization of 1-month SPEI values in each region of Timor Island can be seen in Table 2.
Observation area | Extremely dry index | Longest drought duration | Intensity of drought months by drought level | ||||
Value | Month of incident | Moderately dry | Severely dry | Extremely dry | Total | ||
Kupang City | –2.47 | August 1988 | 4 months | 63 months | 25 months | 6 months | 94 months |
Kupang | –2.74 | April 2016 | 4 months | 62 months | 26 months | 5 months | 93 months |
South Central Timor | –3.86 | August 1998 | 4 months | 59 months | 25 months | 6 months | 90 months |
North Central Timor | –2.61 | August 1998 | 4 months | 62 months | 20 months | 6 months | 88 months |
Malaka | –2.82 | August 2010 | 5 months | 66 months | 25 months | 4 months | 95 months |
Belu | –3.07 | August 2010 | 4 months | 59 months | 23 months | 5 months | 87 months |
The worst SPEI indices, with values lower than or equal to –2 (extremely dry conditions), have occurred throughout Timor Island. On average, the most severe SPEI drought indices occurred in April, except in Kupang Regency. The most extreme short-term meteorological drought ever recorded on Timor Island occurred in South Central Timor Regency, with a drought index of –3.86 in August 1998.
The longest droughts were as follows: In Kupang City for 4 consecutive months from August to November 1988; in Kupang Regency for 4 consecutive months, occurring from June to September 1998; in South Central Timor Regency for 4 consecutive months from May to September 1998 and again from February to March 2018; in North Central Timor Regency for 4 consecutive months, also in two different periods, namely from June to September 1996 and June to September 1998; in Malaka Regency for 5 consecutive months, occurring from August to November 2020; and in Belu Regency for 4 consecutive months, occurring from November 1997 to February 1998 and again from June to September 1998. In addition, the intensity of drought events in each region varies greatly. The distribution of the intensity of drought events can be seen in Figure 3.
Figure 3 illustrates the pattern of variance in the intensity of drought events, which is that extremely dry events tend to occur less frequently than severely dry and moderately dry ones. This phenomenon demonstrates the complexity of drought as a natural phenomenon that involves factors such as time distribution, scale, and varying intensity of occurrence. An appropriate and effective method is needed to understand and describe drought dynamics.
In the analysis of short-term meteorological drought using the 1-month SPEI on Timor Island, the natural trend where more severe events tend to be less frequent than weaker events can be interpreted with a stochastic process model, the power law process. This process can explain how the event's intensity is inversely proportional to its magnitude [16,18]. The power law process helps researchers understand the pattern of drought intensity and can be used to predict the likelihood of future drought events. Therefore, to better understand the spatial distribution of SPEI values on Timor Island, the SPEI index was mapped every month in 2023, as shown in Figure 4.
The 2023 drought distribution map shows that all areas of Timor Island were affected by drought, as seen from the orange and red colors. In 2023, there were 3 months of drought in Kupang City, Kupang, South Central Timor, and Malaka, and 2 months in North Central Timor and Belu. In January, all observed areas experienced drought at a moderately dry level; in October, almost all observed areas experienced drought at a severely dry level, except for Malaka Regency, which experienced a moderately dry level. In November, a moderately dry drought occurred in Malaka Regency, while other regions had returned to normal. In December, an extremely dry drought occurred in South Central Timor Regency, and a severely dry drought was observed in Kupang City and Kupang Regency.
The presentation of the drought distribution map only for 2023 is based on the need to provide up-to-date information on drought conditions on Timor Island. Although the range of observations covers the years 1981–2023, 2023 was chosen due to the relevance of the current information desired in this study. By focusing on that year, a more in-depth understanding of the current spatial distribution of SPEI values in the Timor Island region can be obtained.
We tested the suitability of the power law process model using the Cramér-von Mises test [17]. The hypothesis used is as follows:
H0: The intensity of drought events based on the 1-month SPEI fits the power law process model.
H1: The intensity of drought events based on the 1-month SPEI does not fit the power law process model.
The Cramér-von Mises statistic (C2R) is obtained using Eqs 23–25. The results are presented in Table 3.
Observation area | C2R | Critical value | Decision |
Kupang City | 0.082 | 0.22 | H0 accepted |
Kupang | 0.062 | 0.22 | H0 accepted |
South Central Timor | 0.089 | 0.22 | H0 accepted |
North Central Timor | 0.171 | 0.22 | H0 accepted |
Malaka | 0.043 | 0.22 | H0 accepted |
Belu | 0.022 | 0.22 | H0 accepted |
Based on Table 3, the C2R value of all observation areas is below the critical value determined based on the frequency of drought events, and H0 is accepted. These results indicate that the intensity of drought events based on 1-month SPEI in each region of Timor Island fits the power law process model.
The estimated values of the shape (β) and scale (γ) parameters in the power law process intensity function were obtained using the MLE method based on Eqs 29 and 30 using the time truncated estimate of the power law process [17]. Data used are drought frequency, time of occurrence, and observation time span. The parameter estimation results are presented in Table 4.
Observation area | Parameter | |
ˆβ | ˆγ | |
Kupang City | 1.063 | 7.170 |
Kupang | 1.174 | 10.859 |
South Central Timor | 1.095 | 8.481 |
North Central Timor | 1.049 | 7.245 |
Malaka | 1.034 | 6.296 |
Belu | 1.112 | 9.282 |
Table 4 shows that the ˆβ parameter in each observation area was higher than 1. Based on the characteristics of the power law process, if the intensity function is greater than 1, the intensity of an event will increase [13]. Therefore, it can be concluded that the intensity of drought events in each region of Timor Island will increase, so it is necessary to estimate the frequency of future drought events.
Suppose the time value of drought occurrence in each observation area is ti<t2<⋯<tn<t so that N(t)={N,ti<t2<⋯<tn;(0,t]} with N expressing the frequency of months with drought occurrence in the time interval (0, t]. It is known that the total months observed from 1981–2023 are t = 516 months. The period of months to be estimated is the following 12 months, so if t + s, then 516 + 12 = 528, meaning that the last month of estimation is the 528th month. The initial time of estimation starts from the time after the last time of observation, so the estimation time interval becomes [517,528]. The number of months to be estimated is 12, so the possible value of n is n = 1, 2, 3, ..., 12. The interpretation of the observation time based on the range of 1981–2023 is as follows: The first month is January 2023, and the 516th month is December 2023. For the estimation months, the 517th month is January 2024, and the 528th month is December 2024.
The expected frequency m(t) of drought occurrence in the observation time [1,516] can be obtained by substituting the estimated values of parameters ˆβ and ˆγ in each region of Timor Island into Eq 22. A comparison between expected values and real monthly frequency values is presented in Figure 5.
Based on Figure 4, the expected value shows a very good level of agreement with the actual value. This indicates that the power law process model accurately predicts the number of months with drought events. Furthermore, the estimation of the expected value (m(t+s)) of drought frequency in the future 12 months or in observations [517,528] is presented in Table 5.
Months | Kupang City | Kupang | South Central Timor | Nort Central Timor | Malaka | Belu |
517 (Jan 2024) | 94.207 | 93.244 | 90.195 | 87.957 | 95.393 | 87.374 |
518 (Feb 2024) | 94.400 | 93.456 | 90.386 | 88.136 | 95.584 | 87.562 |
519 (Mar 2024) | 94.594 | 93.668 | 90.577 | 88.314 | 95.775 | 87.750 |
520 (Apr 2024) | 94.788 | 93.879 | 90.768 | 88.493 | 95.965 | 87.938 |
521 (May 2024) | 94.981 | 94.091 | 90.959 | 88.671 | 96.156 | 88.126 |
522 (Jun 2024) | 95.175 | 94.304 | 91.150 | 88.850 | 96.347 | 88.315 |
523 (Jul 2024) | 95.369 | 94.516 | 91.342 | 89.028 | 96.538 | 88.503 |
524 (Aug 2024) | 95.563 | 94.728 | 91.533 | 89.207 | 96.729 | 88.691 |
525 (Sep 2024 | 95.756 | 94.940 | 91.724 | 89.385 | 96.920 | 88.879 |
526 (Oct 2024) | 95.950 | 95.152 | 91.916 | 89.564 | 97.111 | 89.067 |
527 (Nov 2024) | 96.144 | 95.365 | 92.107 | 89.743 | 97.301 | 89.256 |
528 (Dec 2024) | 96.338 | 95.577 | 92.299 | 89.921 | 97.492 | 89.444 |
The predicted results show that, in the next 12 months, the drought frequency in each region of Timor Island will increase by 2 months from the initial observation. For example, in Kupang City, the drought frequency in the 516-month observation is 94 months, and in the 528-month observation is 96 months. The same is true for every other observation area, where in the next 12 months, the frequency of drought events will increase by 2 months. Therefore, it is imperative to make early preparations and implement effective mitigation strategies to reduce the possible impacts of more frequent droughts in the future.
Using the homogeneous Poisson process, the probability value of the expected future frequency of drought can be obtained by substituting the expected value m(t+s) into the non-homogeneous Poisson process based on Eq 20, where m(t) is the expected value at the last time of observation. As mentioned, the power law process is a special case of the non-expected frequency of drought occurrence in the future, as presented in Tables 6–11. For example, if the expected frequency of drought in the next 12 months is 2 months, the probability values based on Tables 6–11 for each region of Timor Island are 0.264 for Kupang City, 0.254 for Kupang, 0.265 for South Central Timor, 0.269 for North Central Timor, 0.266 for Malaka, and 0.267 for Belu. In addition, the probability value of the expected frequency is 1, 3, and 12 months. For example, in Table 7, it is shown that the probability of drought frequency being 3 months in the future 12 months in Kupang City is 0.205. Meanwhile, in Kupang Regency, that probability value is 0.216. This interpretation also applies to each of the following estimates.
Months | n | |||||||||||
1 | 2 | 3 | 4 | 5 | 6 | 7 | 8 | 9 | 10 | 11 | 12 | |
517 (Jan 2024) | 0.160 | |||||||||||
518 (Feb 2024) | 0.263 | 0.051 | ||||||||||
519 (Mar 2024) | 0.325 | 0.094 | 0.018 | |||||||||
520 (Apr 2024) | 0.357 | 0.138 | 0.036 | 0.007 | ||||||||
521 (May 2024) | 0.368 | 0.178 | 0.057 | 0.014 | 0.003 | |||||||
522 (Jun 2024) | 0.364 | 0.211 | 0.082 | 0.024 | 0.006 | 0.001 | ||||||
523 (Jul 2024) | 0.349 | 0.237 | 0.107 | 0.036 | 0.010 | 0.002 | 0.000 | |||||
524 (Aug 2024) | 0.329 | 0.255 | 0.132 | 0.051 | 0.016 | 0.004 | 0.001 | 0.000 | ||||
525 (Sep 2024 | 0.305 | 0.266 | 0.154 | 0.067 | 0.023 | 0.007 | 0.002 | 0.000 | 0.000 | |||
526 (Oct 2024) | 0.279 | 0.270 | 0.175 | 0.085 | 0.033 | 0.011 | 0.003 | 0.001 | 0.000 | 0.000 | ||
527 (Nov 2024) | 0.253 | 0.270 | 0.191 | 0.102 | 0.043 | 0.015 | 0.005 | 0.001 | 0.000 | 0.000 | 0.000 | |
528 (Dec 2024) | 0.227 | 0.264 | 0.205 | 0.119 | 0.055 | 0.021 | 0.007 | 0.002 | 0.001 | 0.000 | 0.000 | 0.000 |
Months | n | |||||||||||
1 | 2 | 3 | 4 | 5 | 6 | 7 | 8 | 9 | 10 | 11 | 12 | |
517 (Jan 2024) | 0.172 | |||||||||||
518 (Feb 2024) | 0.277 | 0.059 | ||||||||||
519 (Mar 2024) | 0.337 | 0.107 | 0.023 | |||||||||
520 (Apr 2024) | 0.363 | 0.154 | 0.043 | 0.009 | ||||||||
521 (May 2024) | 0.367 | 0.194 | 0.069 | 0.018 | 0.004 | |||||||
522 (Jun 2024) | 0.357 | 0.227 | 0.096 | 0.031 | 0.008 | 0.002 | ||||||
523 (Jul 2024) | 0.336 | 0.250 | 0.123 | 0.046 | 0.014 | 0.003 | 0.001 | |||||
524 (Aug 2024) | 0.311 | 0.264 | 0.149 | 0.063 | 0.021 | 0.006 | 0.001 | 0.000 | ||||
525 (Sep 2024 | 0.283 | 0.270 | 0.172 | 0.082 | 0.031 | 0.010 | 0.003 | 0.001 | 0.000 | |||
526 (Oct 2024) | 0.254 | 0.270 | 0.191 | 0.101 | 0.043 | 0.015 | 0.005 | 0.001 | 0.000 | 0.000 | ||
527 (Nov 2024) | 0.226 | 0.264 | 0.205 | 0.120 | 0.056 | 0.022 | 0.007 | 0.002 | 0.001 | 0.000 | 0.000 | |
528 (Dec 2024) | 0.200 | 0.254 | 0.216 | 0.137 | 0.070 | 0.030 | 0.011 | 0.003 | 0.001 | 0.000 | 0.000 | 0.000 |
Months | n | |||||||||||
1 | 2 | 3 | 4 | 5 | 6 | 7 | 8 | 9 | 10 | 11 | 12 | |
517 (Jan 2024) | 0.158 | |||||||||||
518 (Feb 2024) | 0.261 | 0.050 | ||||||||||
519 (Mar 2024) | 0.323 | 0.093 | 0.018 | |||||||||
520 (Apr 2024) | 0.356 | 0.136 | 0.035 | 0.007 | ||||||||
521 (May 2024) | 0.367 | 0.175 | 0.056 | 0.013 | 0.003 | |||||||
522 (Jun 2024) | 0.364 | 0.209 | 0.080 | 0.023 | 0.005 | 0.001 | ||||||
523 (Jul 2024) | 0.351 | 0.235 | 0.105 | 0.035 | 0.009 | 0.002 | 0.000 | |||||
524 (Aug 2024) | 0.331 | 0.253 | 0.129 | 0.049 | 0.015 | 0.004 | 0.001 | 0.000 | ||||
525 (Sep 2024 | 0.308 | 0.265 | 0.152 | 0.065 | 0.022 | 0.006 | 0.002 | 0.000 | 0.000 | |||
526 (Oct 2024) | 0.283 | 0.270 | 0.172 | 0.082 | 0.031 | 0.010 | 0.003 | 0.001 | 0.000 | 0.000 | ||
527 (Nov 2024) | 0.257 | 0.270 | 0.189 | 0.100 | 0.042 | 0.015 | 0.004 | 0.001 | 0.000 | 0.000 | 0.000 | |
528 (Dec 2024) | 0.231 | 0.265 | 0.203 | 0.116 | 0.053 | 0.020 | 0.007 | 0.002 | 0.000 | 0.000 | 0.000 | 0.000 |
Months | n | |||||||||||
1 | 2 | 3 | 4 | 5 | 6 | 7 | 8 | 9 | 10 | 11 | 12 | |
517 (Jan 2024) | 0.149 | |||||||||||
518 (Feb 2024) | 0.250 | 0.045 | ||||||||||
519 (Mar 2024) | 0.313 | 0.084 | 0.015 | |||||||||
520 (Apr 2024) | 0.350 | 0.125 | 0.030 | 0.005 | ||||||||
521 (May 2024) | 0.366 | 0.163 | 0.049 | 0.011 | 0.002 | |||||||
522 (Jun 2024) | 0.367 | 0.197 | 0.070 | 0.019 | 0.004 | 0.001 | ||||||
523 (Jul 2024) | 0.358 | 0.224 | 0.093 | 0.029 | 0.007 | 0.002 | 0.000 | |||||
524 (Aug 2024) | 0.342 | 0.244 | 0.116 | 0.042 | 0.012 | 0.003 | 0.001 | 0.000 | ||||
525 (Sep 2024 | 0.322 | 0.259 | 0.139 | 0.056 | 0.018 | 0.005 | 0.001 | 0.000 | 0.000 | |||
526 (Oct 2024) | 0.299 | 0.267 | 0.159 | 0.071 | 0.025 | 0.008 | 0.002 | 0.000 | 0.000 | 0.000 | ||
527 (Nov 2024) | 0.276 | 0.271 | 0.177 | 0.087 | 0.034 | 0.011 | 0.003 | 0.001 | 0.000 | 0.000 | 0.000 | |
528 (Dec 2024) | 0.251 | 0.269 | 0.192 | 0.103 | 0.044 | 0.016 | 0.005 | 0.001 | 0.000 | 0.000 | 0.000 | 0.000 |
Months | n | |||||||||||
1 | 2 | 3 | 4 | 5 | 6 | 7 | 8 | 9 | 10 | 11 | 12 | |
517 (Jan 2024) | 0.158 | |||||||||||
518 (Feb 2024) | 0.261 | 0.050 | ||||||||||
519 (Mar 2024) | 0.323 | 0.092 | 0.018 | |||||||||
520 (Apr 2024) | 0.356 | 0.136 | 0.035 | 0.007 | ||||||||
521 (May 2024) | 0.367 | 0.175 | 0.056 | 0.013 | 0.003 | |||||||
522 (Jun 2024) | 0.364 | 0.209 | 0.080 | 0.023 | 0.005 | 0.001 | ||||||
523 (Jul 2024) | 0.351 | 0.235 | 0.104 | 0.035 | 0.009 | 0.002 | 0.000 | |||||
524 (Aug 2024) | 0.332 | 0.253 | 0.129 | 0.049 | 0.015 | 0.004 | 0.001 | 0.000 | ||||
525 (Sep 2024 | 0.308 | 0.265 | 0.152 | 0.065 | 0.022 | 0.006 | 0.002 | 0.000 | 0.000 | |||
526 (Oct 2024) | 0.283 | 0.270 | 0.172 | 0.082 | 0.031 | 0.010 | 0.003 | 0.001 | 0.000 | 0.000 | ||
527 (Nov 2024) | 0.257 | 0.270 | 0.189 | 0.099 | 0.042 | 0.015 | 0.004 | 0.001 | 0.000 | 0.000 | 0.000 | |
528 (Dec 2024) | 0.232 | 0.266 | 0.203 | 0.116 | 0.053 | 0.020 | 0.007 | 0.002 | 0.000 | 0.000 | 0.000 | 0.000 |
Months | n | |||||||||||
1 | 2 | 3 | 4 | 5 | 6 | 7 | 8 | 9 | 10 | 11 | 12 | |
517 (Jan 2024) | 0.156 | |||||||||||
518 (Feb 2024) | 0.258 | 0.049 | ||||||||||
519 (Mar 2024) | 0.321 | 0.090 | 0.017 | |||||||||
520 (Apr 2024) | 0.354 | 0.133 | 0.033 | 0.006 | ||||||||
521 (May 2024) | 0.367 | 0.173 | 0.054 | 0.013 | 0.002 | |||||||
522 (Jun 2024) | 0.365 | 0.206 | 0.077 | 0.022 | 0.005 | 0.001 | ||||||
523 (Jul 2024) | 0.353 | 0.232 | 0.102 | 0.034 | 0.009 | 0.002 | 0.000 | |||||
524 (Aug 2024) | 0.334 | 0.251 | 0.126 | 0.047 | 0.014 | 0.004 | 0.001 | 0.000 | ||||
525 (Sep 2024 | 0.311 | 0.264 | 0.149 | 0.063 | 0.021 | 0.006 | 0.001 | 0.000 | ||||
526 (Oct 2024) | 0.287 | 0.270 | 0.169 | 0.080 | 0.030 | 0.009 | 0.003 | 0.001 | 0.000 | |||
527 (Nov 2024) | 0.261 | 0.270 | 0.186 | 0.096 | 0.040 | 0.014 | 0.004 | 0.001 | 0.000 | 0.000 | ||
528 (Dec 2024) | 0.236 | 0.267 | 0.201 | 0.113 | 0.051 | 0.019 | 0.006 | 0.002 | 0.000 | 0.000 | 0.000 | 0.000 |
Note that a limitation of this study lies in the estimation of the power law process model, where the researchers stipulated that the value of the shape parameter (β) should be greater than 1 to indicate an increase in event intensity, as described in Rigdon and Basu’s study [17]. In addition, it should be noted that other modeling approaches or the use of different drought index datasets can be considered. For example, Ghasemi et al. used a Gaussian process regression model to forecast the SPEI drought index [22]; other drought indices, such as the Palmer drought severity index (PDSI) or the Z-Index, may also be used. However, it is important to highlight that future research could focus on modeling and comparing different results. Furthermore, the model used to analyze the SPEI drought index in Timor Island has a wide potential application in cases where extreme events are rare compared to common weaker events, such as in the study of earthquakes, extreme weather, temperature changes, and others.
The analysis of short-term meteorological drought events using SPEI for a 1-month period on Timor Island shows that extremely dry events are less frequent than very dry and dry events. The results of the power law process parameter estimation show a β>1 value in all regions of Timor Island, specifically 1.063 for Kupang City, 1.174 for Kupang Regency, 1.095 for South Central Timor Regency, 1.049 for North Central Timor Regency, 1.034 for Malaka Regency, and 1.112 for Belu Regency. This indicates an increase in drought events in the future. In the next 12 months, the estimated duration of short-term meteorological droughts in all regions is 2 months, with the following probability values: 0.264 for Kupang City, 0.25 for Kupang, 0.265 for South Central Timor, 0.269 for North Central Timor, 0.265 for Malaka, and 0.266 for Belu.
The authors declare they have not used Artificial Intelligence (AI) tools in the creation of this article.
The authors are grateful for financial support from the Directorate General of Higher Education, Ministry of Education and Culture, Research and Technology of Indonesia.
The authors stated that there is no conflict of interest for the study.
[1] |
Hao Z, Singh VP, Xia Y (2018) Seasonal drought prediction: Advances, challenges, and future prospects. Rev Geophys 56: 108–141. https://doi.org/10.1002/2016RG000549 doi: 10.1002/2016RG000549
![]() |
[2] |
Sunusi N (2023) Bias of automatic weather parameter measurement in monsoon area, a case study in Makassar Coast. AIMS Environ Sci 10: 1–15. https://doi.org/10.3934/environsci.2023001 doi: 10.3934/environsci.2023001
![]() |
[3] |
Supari, Tangang F, Juneng L, et al. (2017) Observed changes in extreme temperature and precipitation over Indonesia. Int J Climatol 37: 1979–1997. https://doi.org/10.1002/joc.4829 doi: 10.1002/joc.4829
![]() |
[4] |
Easterling DR, Wallis TW, Lawrimore JH, et al. (2007) Effects of temperature and precipitation trends on US drought. Geophys Res Lett 34. https://doi.org/10.1029/2007GL031541 doi: 10.1029/2007GL031541
![]() |
[5] |
Yang M, Mou Y, Meng Y, et al. (2020) Modeling the effects of precipitation and temperature patterns on agricultural drought in China from 1949 to 2015. Sci Total Environ 711. https://doi.org/10.1016/j.scitotenv.2019.135139 doi: 10.1016/j.scitotenv.2019.135139
![]() |
[6] |
Adedeji O, Olusola A, James G, et al. (2020) Early warning systems development for agricultural drought assessment in Nigeria. Environ Monit Assess 192: 1–21. https://doi.org/10.1007/s10661-020-08730-3 doi: 10.1007/s10661-020-08730-3
![]() |
[7] |
Nguyen H, Thompson A, Costello C (2023) Impacts of historical droughts on maize and soybean production in the southeastern United States. Agr Water Manage 281: 1–12. https://doi.org/10.1016/j.agwat.2023.108237 doi: 10.1016/j.agwat.2023.108237
![]() |
[8] |
Cai S, Zuo D, Wang H, et al. (2023) Assessment of agricultural drought based on multi-source remote sensing data in a major grain producing area of Northwest China. Agr Water Manage 278: 1–17. https://doi.org/10.1016/j.agwat.2023.108142 doi: 10.1016/j.agwat.2023.108142
![]() |
[9] |
Yang B, Cui Q, Meng Y, et al. (2023) Combined multivariate drought index for drought assessment in China from 2003 to 2020. Agr Water Manage 281: 1–16. https://doi.org/10.1016/j.agwat.2023.108241 doi: 10.1016/j.agwat.2023.108241
![]() |
[10] |
Domingues LM, da Rocha HR (2022) Serial droughts and loss of hydrologic resilience in a subtropical basin: The case of water inflow into the Cantareira reservoir system in Brazil during 2013–2021. J Hydrol-Reg Stud 44: 1–18. https://doi.org/10.1016/j.ejrh.2022.101235 doi: 10.1016/j.ejrh.2022.101235
![]() |
[11] |
Ashraf M, Ullah K, Adnan S (2022) Satellite based impact assessment of temperature and rainfall variability on drought indices in Southern Pakistan. Int J Appl Earth Obs 108: 1–20. https://doi.org/10.1016/j.jag.2022.102726 doi: 10.1016/j.jag.2022.102726
![]() |
[12] |
Wilhite DA, Glantz MH (1985) Understanding: the drought phenomenon: The role of definitions. Water Int 10: 111–120. https://doi.org/10.1080/02508068508686328 doi: 10.1080/02508068508686328
![]() |
[13] | Palmer WC (1965) Meteorological drought. Washington DC: US Weather Bureau. |
[14] | McKee TB, Doesken NJ, Kleist J (1993) The relationship of drought frequency and duration to time scales. 8th Conference on Applied Climatology, Anaheim, 179–184. |
[15] |
Serrano SMV, Beguería S, Moreno JIL (2010) A multiscalar drought index sensitive to global warming: The standardized precipitation evapotranspiration index. J Climate 23: 1696–1718. https://doi.org/10.1175/2009JCLI2909.1 doi: 10.1175/2009JCLI2909.1
![]() |
[16] |
Clauset A, Shalizi CR, Newman ME (2009) Power-law distributions in empirical data. SIAM Rev 51: 661–703. https://doi.org/10.1137/070710111 doi: 10.1137/070710111
![]() |
[17] |
Rigdon SE, Basu AP (1989) The power law process: A model for the reliability of repairable systems. J Qual Technol 21: 251–260. https://doi.org/10.1080/00224065.1989.11979183 doi: 10.1080/00224065.1989.11979183
![]() |
[18] |
Chehade A, Shi Z, Krivtsov V (2020) Power-law nonhomogeneous Poisson process with a mixture of latent common shape parameters. Reliab Eng Syst Safe 3: 1–9. https://doi.org/10.1016/j.ress.2020.107097 doi: 10.1016/j.ress.2020.107097
![]() |
[19] |
Achcar JA, Rodrigues ER, Tzintzun G (2011) Using non-homogeneous Poisson models with multiple change-points to estimate the number of ozone exceedances in Mexico City. Environmetrics 22: 1–12. https://doi.org/10.1002/env.1029 doi: 10.1002/env.1029
![]() |
[20] |
Achcar JA, Barros EAC, Souza RMD (2016) Use of non-homogeneous Poisson process (NHPP) in presence of change-points to analyze drought periods: a case study in Brazil. Environ Ecol Stat 23: 405–419. https://doi.org/10.1007/s10651-016-0345-z doi: 10.1007/s10651-016-0345-z
![]() |
[21] |
Ellahi A, Hussain I, Hashmi MZ, et al. (2021) Agricultural drought periods analysis by using nonhomogeneous Poisson models and regionalization of appropriate model parameters. Tellus A 73: 1–16. http://dx.doi.org/10.1080/16000870.2021.1948241 doi: 10.1080/16000870.2021.1948241
![]() |
[22] |
Ghasemi P, Karbasi M, Nouri AZ, et al. (2021) Application of Gaussian process regression to forecast multi-step ahead SPEI drought index. Alex Eng J 60: 5375–5392. https://doi.org/10.1016/j.aej.2021.04.022 doi: 10.1016/j.aej.2021.04.022
![]() |
[23] |
Karbasi M, Karbasi M, Jamei M, et al. (2022) Development of a new wavelet-based hybrid model to forecast multi-scalar SPEI drought index (Case study: Zanjan city, Iran). Theor Appl Climatol 147: 499–522. https://doi.org/10.1007/s00704-021-03825-4 doi: 10.1007/s00704-021-03825-4
![]() |
[24] |
Dikshit A, Pradhan B, Huete A (2021) An improved SPEI drought forecasting approach using the long short-term memory neural network. J Environ Manage 283: 1–12. https://doi.org/10.1016/j.jenvman.2021.111979 doi: 10.1016/j.jenvman.2021.111979
![]() |
[25] |
Affandy NA, Anwar N, Maulana MA, et al. (2023) Forecasting meteorological drought through SPEI with SARIMA model, In AIP Conference Proceedings, 2846. http://dx.doi.org/10.1063/5.0154230 doi: 10.1063/5.0154230
![]() |
[26] |
Marquet PA, Quiñones RA, Abades S, et al. (2005) Scaling and power-laws in ecological systems. J Exp Biol 208: 1749–1769. http://dx.doi.org/10.1242/jeb.01588 doi: 10.1242/jeb.01588
![]() |
[27] |
Lyth DH, Stewart ED (1992) The curvature perturbation in power law (e.g., extended) inflation. Phys Lett B 274: 168–172. https://doi.org/10.1016/0370-2693(92)90518-9 doi: 10.1016/0370-2693(92)90518-9
![]() |
[28] |
Li X, Guo F, Liu YH (2021) The acceleration of charged particles and formation of power-law energy spectra in nonrelativistic magnetic reconnection. Phys Plasmas 28: 1–38. https://doi.org/10.48550/arXiv.2104.10732 doi: 10.48550/arXiv.2104.10732
![]() |
[29] |
Bu T, Towsley D (2002) On distinguishing between Internet power law topology generators, In Proceedings. Twenty-first annual joint conference of the ieee computer and communications societies, 2: 638–647. https://doi.org/10.1109/INFCOM.2002.1019309 doi: 10.1109/INFCOM.2002.1019309
![]() |
[30] |
Andreatta D, Lustres JLP, Kovalenko SA, et al. (2005) Power-law solvation dynamics in DNA over six decades in time. J Am Chem Soc 127: 7270–7271. https://doi.org/10.1021/ja044177v doi: 10.1021/ja044177v
![]() |
[31] | Zhao M, Xie M (1996) On maximum likelihood estimation for a general non-homogeneous Poisson process. Scand J Stat 23: 597–607. |
[32] |
Turk LA (2014) Testing the performance of the Power Law Process model considering the use of Regression estimation approach. Int J Softw Eng Appl 5: 35–46. http://dx.doi.org/10.5121/ijsea.2014.5503 doi: 10.5121/ijsea.2014.5503
![]() |
[33] |
Thornthwaite CW (1948) An approach toward a rational classification of climate. Geogr Rev 38: 55–94. http://dx.doi.org/10.2307/210739 doi: 10.2307/210739
![]() |
[34] |
Singh VP, Guo H, Yu FX (1993) Parameter estimation for 3-parameter log-logistic distribution (LLD3) by Pome. Stoch Hydrol Hydraulics 7: 163–177. https://doi.org/10.1007/BF01585596 doi: 10.1007/BF01585596
![]() |
[35] | Abramowitz M, Stegun IA (1964) Handbook of mathematical functions with formulas, graphs, and mathematical tables, US Government Printing Office, 55. |
[36] | Svoboda M, Hayes M, Wood D (2012) Standardized precipitation index: User guide. |
[37] | Ross SM (2014) Introduction to probability models, 4 Eds., Berkeley: Academic Press, 1–647. |
[38] |
Kuswanto H, Puspa AW, Ahmad IS, et al. (2021) Drought analysis in East Nusa Tenggara (Indonesia) using regional frequency analysis. The Scientific World J 2021: 1–10. http://dx.doi.org/10.1155/2021/6626102 doi: 10.1155/2021/6626102
![]() |
1. | Nurtiti Sunusi, Nur Hikmah Auliana, Assessing SPI and SPEI for Drought Forecasting through the Power Law Process: A Case Study in South Sulawesi, Indonesia, 2025, 22150161, 103235, 10.1016/j.mex.2025.103235 |
SPEI value | Classification |
≥2.00 | Extremely wet |
1.50–1.99 | Very wet |
1.00–1.49 | Moderately wet |
(–0.99)–0.99 | Normal |
(–1.00)–(–1.49) | Moderately dry |
(–1.50)–(–1.99) | Severely dry |
≤ –2.00 | Extremely dry |
Observation area | Extremely dry index | Longest drought duration | Intensity of drought months by drought level | ||||
Value | Month of incident | Moderately dry | Severely dry | Extremely dry | Total | ||
Kupang City | –2.47 | August 1988 | 4 months | 63 months | 25 months | 6 months | 94 months |
Kupang | –2.74 | April 2016 | 4 months | 62 months | 26 months | 5 months | 93 months |
South Central Timor | –3.86 | August 1998 | 4 months | 59 months | 25 months | 6 months | 90 months |
North Central Timor | –2.61 | August 1998 | 4 months | 62 months | 20 months | 6 months | 88 months |
Malaka | –2.82 | August 2010 | 5 months | 66 months | 25 months | 4 months | 95 months |
Belu | –3.07 | August 2010 | 4 months | 59 months | 23 months | 5 months | 87 months |
Observation area | C2R | Critical value | Decision |
Kupang City | 0.082 | 0.22 | H0 accepted |
Kupang | 0.062 | 0.22 | H0 accepted |
South Central Timor | 0.089 | 0.22 | H0 accepted |
North Central Timor | 0.171 | 0.22 | H0 accepted |
Malaka | 0.043 | 0.22 | H0 accepted |
Belu | 0.022 | 0.22 | H0 accepted |
Observation area | Parameter | |
ˆβ | ˆγ | |
Kupang City | 1.063 | 7.170 |
Kupang | 1.174 | 10.859 |
South Central Timor | 1.095 | 8.481 |
North Central Timor | 1.049 | 7.245 |
Malaka | 1.034 | 6.296 |
Belu | 1.112 | 9.282 |
Months | Kupang City | Kupang | South Central Timor | Nort Central Timor | Malaka | Belu |
517 (Jan 2024) | 94.207 | 93.244 | 90.195 | 87.957 | 95.393 | 87.374 |
518 (Feb 2024) | 94.400 | 93.456 | 90.386 | 88.136 | 95.584 | 87.562 |
519 (Mar 2024) | 94.594 | 93.668 | 90.577 | 88.314 | 95.775 | 87.750 |
520 (Apr 2024) | 94.788 | 93.879 | 90.768 | 88.493 | 95.965 | 87.938 |
521 (May 2024) | 94.981 | 94.091 | 90.959 | 88.671 | 96.156 | 88.126 |
522 (Jun 2024) | 95.175 | 94.304 | 91.150 | 88.850 | 96.347 | 88.315 |
523 (Jul 2024) | 95.369 | 94.516 | 91.342 | 89.028 | 96.538 | 88.503 |
524 (Aug 2024) | 95.563 | 94.728 | 91.533 | 89.207 | 96.729 | 88.691 |
525 (Sep 2024 | 95.756 | 94.940 | 91.724 | 89.385 | 96.920 | 88.879 |
526 (Oct 2024) | 95.950 | 95.152 | 91.916 | 89.564 | 97.111 | 89.067 |
527 (Nov 2024) | 96.144 | 95.365 | 92.107 | 89.743 | 97.301 | 89.256 |
528 (Dec 2024) | 96.338 | 95.577 | 92.299 | 89.921 | 97.492 | 89.444 |
Months | n | |||||||||||
1 | 2 | 3 | 4 | 5 | 6 | 7 | 8 | 9 | 10 | 11 | 12 | |
517 (Jan 2024) | 0.160 | |||||||||||
518 (Feb 2024) | 0.263 | 0.051 | ||||||||||
519 (Mar 2024) | 0.325 | 0.094 | 0.018 | |||||||||
520 (Apr 2024) | 0.357 | 0.138 | 0.036 | 0.007 | ||||||||
521 (May 2024) | 0.368 | 0.178 | 0.057 | 0.014 | 0.003 | |||||||
522 (Jun 2024) | 0.364 | 0.211 | 0.082 | 0.024 | 0.006 | 0.001 | ||||||
523 (Jul 2024) | 0.349 | 0.237 | 0.107 | 0.036 | 0.010 | 0.002 | 0.000 | |||||
524 (Aug 2024) | 0.329 | 0.255 | 0.132 | 0.051 | 0.016 | 0.004 | 0.001 | 0.000 | ||||
525 (Sep 2024 | 0.305 | 0.266 | 0.154 | 0.067 | 0.023 | 0.007 | 0.002 | 0.000 | 0.000 | |||
526 (Oct 2024) | 0.279 | 0.270 | 0.175 | 0.085 | 0.033 | 0.011 | 0.003 | 0.001 | 0.000 | 0.000 | ||
527 (Nov 2024) | 0.253 | 0.270 | 0.191 | 0.102 | 0.043 | 0.015 | 0.005 | 0.001 | 0.000 | 0.000 | 0.000 | |
528 (Dec 2024) | 0.227 | 0.264 | 0.205 | 0.119 | 0.055 | 0.021 | 0.007 | 0.002 | 0.001 | 0.000 | 0.000 | 0.000 |
Months | n | |||||||||||
1 | 2 | 3 | 4 | 5 | 6 | 7 | 8 | 9 | 10 | 11 | 12 | |
517 (Jan 2024) | 0.172 | |||||||||||
518 (Feb 2024) | 0.277 | 0.059 | ||||||||||
519 (Mar 2024) | 0.337 | 0.107 | 0.023 | |||||||||
520 (Apr 2024) | 0.363 | 0.154 | 0.043 | 0.009 | ||||||||
521 (May 2024) | 0.367 | 0.194 | 0.069 | 0.018 | 0.004 | |||||||
522 (Jun 2024) | 0.357 | 0.227 | 0.096 | 0.031 | 0.008 | 0.002 | ||||||
523 (Jul 2024) | 0.336 | 0.250 | 0.123 | 0.046 | 0.014 | 0.003 | 0.001 | |||||
524 (Aug 2024) | 0.311 | 0.264 | 0.149 | 0.063 | 0.021 | 0.006 | 0.001 | 0.000 | ||||
525 (Sep 2024 | 0.283 | 0.270 | 0.172 | 0.082 | 0.031 | 0.010 | 0.003 | 0.001 | 0.000 | |||
526 (Oct 2024) | 0.254 | 0.270 | 0.191 | 0.101 | 0.043 | 0.015 | 0.005 | 0.001 | 0.000 | 0.000 | ||
527 (Nov 2024) | 0.226 | 0.264 | 0.205 | 0.120 | 0.056 | 0.022 | 0.007 | 0.002 | 0.001 | 0.000 | 0.000 | |
528 (Dec 2024) | 0.200 | 0.254 | 0.216 | 0.137 | 0.070 | 0.030 | 0.011 | 0.003 | 0.001 | 0.000 | 0.000 | 0.000 |
Months | n | |||||||||||
1 | 2 | 3 | 4 | 5 | 6 | 7 | 8 | 9 | 10 | 11 | 12 | |
517 (Jan 2024) | 0.158 | |||||||||||
518 (Feb 2024) | 0.261 | 0.050 | ||||||||||
519 (Mar 2024) | 0.323 | 0.093 | 0.018 | |||||||||
520 (Apr 2024) | 0.356 | 0.136 | 0.035 | 0.007 | ||||||||
521 (May 2024) | 0.367 | 0.175 | 0.056 | 0.013 | 0.003 | |||||||
522 (Jun 2024) | 0.364 | 0.209 | 0.080 | 0.023 | 0.005 | 0.001 | ||||||
523 (Jul 2024) | 0.351 | 0.235 | 0.105 | 0.035 | 0.009 | 0.002 | 0.000 | |||||
524 (Aug 2024) | 0.331 | 0.253 | 0.129 | 0.049 | 0.015 | 0.004 | 0.001 | 0.000 | ||||
525 (Sep 2024 | 0.308 | 0.265 | 0.152 | 0.065 | 0.022 | 0.006 | 0.002 | 0.000 | 0.000 | |||
526 (Oct 2024) | 0.283 | 0.270 | 0.172 | 0.082 | 0.031 | 0.010 | 0.003 | 0.001 | 0.000 | 0.000 | ||
527 (Nov 2024) | 0.257 | 0.270 | 0.189 | 0.100 | 0.042 | 0.015 | 0.004 | 0.001 | 0.000 | 0.000 | 0.000 | |
528 (Dec 2024) | 0.231 | 0.265 | 0.203 | 0.116 | 0.053 | 0.020 | 0.007 | 0.002 | 0.000 | 0.000 | 0.000 | 0.000 |
Months | n | |||||||||||
1 | 2 | 3 | 4 | 5 | 6 | 7 | 8 | 9 | 10 | 11 | 12 | |
517 (Jan 2024) | 0.149 | |||||||||||
518 (Feb 2024) | 0.250 | 0.045 | ||||||||||
519 (Mar 2024) | 0.313 | 0.084 | 0.015 | |||||||||
520 (Apr 2024) | 0.350 | 0.125 | 0.030 | 0.005 | ||||||||
521 (May 2024) | 0.366 | 0.163 | 0.049 | 0.011 | 0.002 | |||||||
522 (Jun 2024) | 0.367 | 0.197 | 0.070 | 0.019 | 0.004 | 0.001 | ||||||
523 (Jul 2024) | 0.358 | 0.224 | 0.093 | 0.029 | 0.007 | 0.002 | 0.000 | |||||
524 (Aug 2024) | 0.342 | 0.244 | 0.116 | 0.042 | 0.012 | 0.003 | 0.001 | 0.000 | ||||
525 (Sep 2024 | 0.322 | 0.259 | 0.139 | 0.056 | 0.018 | 0.005 | 0.001 | 0.000 | 0.000 | |||
526 (Oct 2024) | 0.299 | 0.267 | 0.159 | 0.071 | 0.025 | 0.008 | 0.002 | 0.000 | 0.000 | 0.000 | ||
527 (Nov 2024) | 0.276 | 0.271 | 0.177 | 0.087 | 0.034 | 0.011 | 0.003 | 0.001 | 0.000 | 0.000 | 0.000 | |
528 (Dec 2024) | 0.251 | 0.269 | 0.192 | 0.103 | 0.044 | 0.016 | 0.005 | 0.001 | 0.000 | 0.000 | 0.000 | 0.000 |
Months | n | |||||||||||
1 | 2 | 3 | 4 | 5 | 6 | 7 | 8 | 9 | 10 | 11 | 12 | |
517 (Jan 2024) | 0.158 | |||||||||||
518 (Feb 2024) | 0.261 | 0.050 | ||||||||||
519 (Mar 2024) | 0.323 | 0.092 | 0.018 | |||||||||
520 (Apr 2024) | 0.356 | 0.136 | 0.035 | 0.007 | ||||||||
521 (May 2024) | 0.367 | 0.175 | 0.056 | 0.013 | 0.003 | |||||||
522 (Jun 2024) | 0.364 | 0.209 | 0.080 | 0.023 | 0.005 | 0.001 | ||||||
523 (Jul 2024) | 0.351 | 0.235 | 0.104 | 0.035 | 0.009 | 0.002 | 0.000 | |||||
524 (Aug 2024) | 0.332 | 0.253 | 0.129 | 0.049 | 0.015 | 0.004 | 0.001 | 0.000 | ||||
525 (Sep 2024 | 0.308 | 0.265 | 0.152 | 0.065 | 0.022 | 0.006 | 0.002 | 0.000 | 0.000 | |||
526 (Oct 2024) | 0.283 | 0.270 | 0.172 | 0.082 | 0.031 | 0.010 | 0.003 | 0.001 | 0.000 | 0.000 | ||
527 (Nov 2024) | 0.257 | 0.270 | 0.189 | 0.099 | 0.042 | 0.015 | 0.004 | 0.001 | 0.000 | 0.000 | 0.000 | |
528 (Dec 2024) | 0.232 | 0.266 | 0.203 | 0.116 | 0.053 | 0.020 | 0.007 | 0.002 | 0.000 | 0.000 | 0.000 | 0.000 |
Months | n | |||||||||||
1 | 2 | 3 | 4 | 5 | 6 | 7 | 8 | 9 | 10 | 11 | 12 | |
517 (Jan 2024) | 0.156 | |||||||||||
518 (Feb 2024) | 0.258 | 0.049 | ||||||||||
519 (Mar 2024) | 0.321 | 0.090 | 0.017 | |||||||||
520 (Apr 2024) | 0.354 | 0.133 | 0.033 | 0.006 | ||||||||
521 (May 2024) | 0.367 | 0.173 | 0.054 | 0.013 | 0.002 | |||||||
522 (Jun 2024) | 0.365 | 0.206 | 0.077 | 0.022 | 0.005 | 0.001 | ||||||
523 (Jul 2024) | 0.353 | 0.232 | 0.102 | 0.034 | 0.009 | 0.002 | 0.000 | |||||
524 (Aug 2024) | 0.334 | 0.251 | 0.126 | 0.047 | 0.014 | 0.004 | 0.001 | 0.000 | ||||
525 (Sep 2024 | 0.311 | 0.264 | 0.149 | 0.063 | 0.021 | 0.006 | 0.001 | 0.000 | ||||
526 (Oct 2024) | 0.287 | 0.270 | 0.169 | 0.080 | 0.030 | 0.009 | 0.003 | 0.001 | 0.000 | |||
527 (Nov 2024) | 0.261 | 0.270 | 0.186 | 0.096 | 0.040 | 0.014 | 0.004 | 0.001 | 0.000 | 0.000 | ||
528 (Dec 2024) | 0.236 | 0.267 | 0.201 | 0.113 | 0.051 | 0.019 | 0.006 | 0.002 | 0.000 | 0.000 | 0.000 | 0.000 |
SPEI value | Classification |
≥2.00 | Extremely wet |
1.50–1.99 | Very wet |
1.00–1.49 | Moderately wet |
(–0.99)–0.99 | Normal |
(–1.00)–(–1.49) | Moderately dry |
(–1.50)–(–1.99) | Severely dry |
≤ –2.00 | Extremely dry |
Observation area | Extremely dry index | Longest drought duration | Intensity of drought months by drought level | ||||
Value | Month of incident | Moderately dry | Severely dry | Extremely dry | Total | ||
Kupang City | –2.47 | August 1988 | 4 months | 63 months | 25 months | 6 months | 94 months |
Kupang | –2.74 | April 2016 | 4 months | 62 months | 26 months | 5 months | 93 months |
South Central Timor | –3.86 | August 1998 | 4 months | 59 months | 25 months | 6 months | 90 months |
North Central Timor | –2.61 | August 1998 | 4 months | 62 months | 20 months | 6 months | 88 months |
Malaka | –2.82 | August 2010 | 5 months | 66 months | 25 months | 4 months | 95 months |
Belu | –3.07 | August 2010 | 4 months | 59 months | 23 months | 5 months | 87 months |
Observation area | C2R | Critical value | Decision |
Kupang City | 0.082 | 0.22 | H0 accepted |
Kupang | 0.062 | 0.22 | H0 accepted |
South Central Timor | 0.089 | 0.22 | H0 accepted |
North Central Timor | 0.171 | 0.22 | H0 accepted |
Malaka | 0.043 | 0.22 | H0 accepted |
Belu | 0.022 | 0.22 | H0 accepted |
Observation area | Parameter | |
ˆβ | ˆγ | |
Kupang City | 1.063 | 7.170 |
Kupang | 1.174 | 10.859 |
South Central Timor | 1.095 | 8.481 |
North Central Timor | 1.049 | 7.245 |
Malaka | 1.034 | 6.296 |
Belu | 1.112 | 9.282 |
Months | Kupang City | Kupang | South Central Timor | Nort Central Timor | Malaka | Belu |
517 (Jan 2024) | 94.207 | 93.244 | 90.195 | 87.957 | 95.393 | 87.374 |
518 (Feb 2024) | 94.400 | 93.456 | 90.386 | 88.136 | 95.584 | 87.562 |
519 (Mar 2024) | 94.594 | 93.668 | 90.577 | 88.314 | 95.775 | 87.750 |
520 (Apr 2024) | 94.788 | 93.879 | 90.768 | 88.493 | 95.965 | 87.938 |
521 (May 2024) | 94.981 | 94.091 | 90.959 | 88.671 | 96.156 | 88.126 |
522 (Jun 2024) | 95.175 | 94.304 | 91.150 | 88.850 | 96.347 | 88.315 |
523 (Jul 2024) | 95.369 | 94.516 | 91.342 | 89.028 | 96.538 | 88.503 |
524 (Aug 2024) | 95.563 | 94.728 | 91.533 | 89.207 | 96.729 | 88.691 |
525 (Sep 2024 | 95.756 | 94.940 | 91.724 | 89.385 | 96.920 | 88.879 |
526 (Oct 2024) | 95.950 | 95.152 | 91.916 | 89.564 | 97.111 | 89.067 |
527 (Nov 2024) | 96.144 | 95.365 | 92.107 | 89.743 | 97.301 | 89.256 |
528 (Dec 2024) | 96.338 | 95.577 | 92.299 | 89.921 | 97.492 | 89.444 |
Months | n | |||||||||||
1 | 2 | 3 | 4 | 5 | 6 | 7 | 8 | 9 | 10 | 11 | 12 | |
517 (Jan 2024) | 0.160 | |||||||||||
518 (Feb 2024) | 0.263 | 0.051 | ||||||||||
519 (Mar 2024) | 0.325 | 0.094 | 0.018 | |||||||||
520 (Apr 2024) | 0.357 | 0.138 | 0.036 | 0.007 | ||||||||
521 (May 2024) | 0.368 | 0.178 | 0.057 | 0.014 | 0.003 | |||||||
522 (Jun 2024) | 0.364 | 0.211 | 0.082 | 0.024 | 0.006 | 0.001 | ||||||
523 (Jul 2024) | 0.349 | 0.237 | 0.107 | 0.036 | 0.010 | 0.002 | 0.000 | |||||
524 (Aug 2024) | 0.329 | 0.255 | 0.132 | 0.051 | 0.016 | 0.004 | 0.001 | 0.000 | ||||
525 (Sep 2024 | 0.305 | 0.266 | 0.154 | 0.067 | 0.023 | 0.007 | 0.002 | 0.000 | 0.000 | |||
526 (Oct 2024) | 0.279 | 0.270 | 0.175 | 0.085 | 0.033 | 0.011 | 0.003 | 0.001 | 0.000 | 0.000 | ||
527 (Nov 2024) | 0.253 | 0.270 | 0.191 | 0.102 | 0.043 | 0.015 | 0.005 | 0.001 | 0.000 | 0.000 | 0.000 | |
528 (Dec 2024) | 0.227 | 0.264 | 0.205 | 0.119 | 0.055 | 0.021 | 0.007 | 0.002 | 0.001 | 0.000 | 0.000 | 0.000 |
Months | n | |||||||||||
1 | 2 | 3 | 4 | 5 | 6 | 7 | 8 | 9 | 10 | 11 | 12 | |
517 (Jan 2024) | 0.172 | |||||||||||
518 (Feb 2024) | 0.277 | 0.059 | ||||||||||
519 (Mar 2024) | 0.337 | 0.107 | 0.023 | |||||||||
520 (Apr 2024) | 0.363 | 0.154 | 0.043 | 0.009 | ||||||||
521 (May 2024) | 0.367 | 0.194 | 0.069 | 0.018 | 0.004 | |||||||
522 (Jun 2024) | 0.357 | 0.227 | 0.096 | 0.031 | 0.008 | 0.002 | ||||||
523 (Jul 2024) | 0.336 | 0.250 | 0.123 | 0.046 | 0.014 | 0.003 | 0.001 | |||||
524 (Aug 2024) | 0.311 | 0.264 | 0.149 | 0.063 | 0.021 | 0.006 | 0.001 | 0.000 | ||||
525 (Sep 2024 | 0.283 | 0.270 | 0.172 | 0.082 | 0.031 | 0.010 | 0.003 | 0.001 | 0.000 | |||
526 (Oct 2024) | 0.254 | 0.270 | 0.191 | 0.101 | 0.043 | 0.015 | 0.005 | 0.001 | 0.000 | 0.000 | ||
527 (Nov 2024) | 0.226 | 0.264 | 0.205 | 0.120 | 0.056 | 0.022 | 0.007 | 0.002 | 0.001 | 0.000 | 0.000 | |
528 (Dec 2024) | 0.200 | 0.254 | 0.216 | 0.137 | 0.070 | 0.030 | 0.011 | 0.003 | 0.001 | 0.000 | 0.000 | 0.000 |
Months | n | |||||||||||
1 | 2 | 3 | 4 | 5 | 6 | 7 | 8 | 9 | 10 | 11 | 12 | |
517 (Jan 2024) | 0.158 | |||||||||||
518 (Feb 2024) | 0.261 | 0.050 | ||||||||||
519 (Mar 2024) | 0.323 | 0.093 | 0.018 | |||||||||
520 (Apr 2024) | 0.356 | 0.136 | 0.035 | 0.007 | ||||||||
521 (May 2024) | 0.367 | 0.175 | 0.056 | 0.013 | 0.003 | |||||||
522 (Jun 2024) | 0.364 | 0.209 | 0.080 | 0.023 | 0.005 | 0.001 | ||||||
523 (Jul 2024) | 0.351 | 0.235 | 0.105 | 0.035 | 0.009 | 0.002 | 0.000 | |||||
524 (Aug 2024) | 0.331 | 0.253 | 0.129 | 0.049 | 0.015 | 0.004 | 0.001 | 0.000 | ||||
525 (Sep 2024 | 0.308 | 0.265 | 0.152 | 0.065 | 0.022 | 0.006 | 0.002 | 0.000 | 0.000 | |||
526 (Oct 2024) | 0.283 | 0.270 | 0.172 | 0.082 | 0.031 | 0.010 | 0.003 | 0.001 | 0.000 | 0.000 | ||
527 (Nov 2024) | 0.257 | 0.270 | 0.189 | 0.100 | 0.042 | 0.015 | 0.004 | 0.001 | 0.000 | 0.000 | 0.000 | |
528 (Dec 2024) | 0.231 | 0.265 | 0.203 | 0.116 | 0.053 | 0.020 | 0.007 | 0.002 | 0.000 | 0.000 | 0.000 | 0.000 |
Months | n | |||||||||||
1 | 2 | 3 | 4 | 5 | 6 | 7 | 8 | 9 | 10 | 11 | 12 | |
517 (Jan 2024) | 0.149 | |||||||||||
518 (Feb 2024) | 0.250 | 0.045 | ||||||||||
519 (Mar 2024) | 0.313 | 0.084 | 0.015 | |||||||||
520 (Apr 2024) | 0.350 | 0.125 | 0.030 | 0.005 | ||||||||
521 (May 2024) | 0.366 | 0.163 | 0.049 | 0.011 | 0.002 | |||||||
522 (Jun 2024) | 0.367 | 0.197 | 0.070 | 0.019 | 0.004 | 0.001 | ||||||
523 (Jul 2024) | 0.358 | 0.224 | 0.093 | 0.029 | 0.007 | 0.002 | 0.000 | |||||
524 (Aug 2024) | 0.342 | 0.244 | 0.116 | 0.042 | 0.012 | 0.003 | 0.001 | 0.000 | ||||
525 (Sep 2024 | 0.322 | 0.259 | 0.139 | 0.056 | 0.018 | 0.005 | 0.001 | 0.000 | 0.000 | |||
526 (Oct 2024) | 0.299 | 0.267 | 0.159 | 0.071 | 0.025 | 0.008 | 0.002 | 0.000 | 0.000 | 0.000 | ||
527 (Nov 2024) | 0.276 | 0.271 | 0.177 | 0.087 | 0.034 | 0.011 | 0.003 | 0.001 | 0.000 | 0.000 | 0.000 | |
528 (Dec 2024) | 0.251 | 0.269 | 0.192 | 0.103 | 0.044 | 0.016 | 0.005 | 0.001 | 0.000 | 0.000 | 0.000 | 0.000 |
Months | n | |||||||||||
1 | 2 | 3 | 4 | 5 | 6 | 7 | 8 | 9 | 10 | 11 | 12 | |
517 (Jan 2024) | 0.158 | |||||||||||
518 (Feb 2024) | 0.261 | 0.050 | ||||||||||
519 (Mar 2024) | 0.323 | 0.092 | 0.018 | |||||||||
520 (Apr 2024) | 0.356 | 0.136 | 0.035 | 0.007 | ||||||||
521 (May 2024) | 0.367 | 0.175 | 0.056 | 0.013 | 0.003 | |||||||
522 (Jun 2024) | 0.364 | 0.209 | 0.080 | 0.023 | 0.005 | 0.001 | ||||||
523 (Jul 2024) | 0.351 | 0.235 | 0.104 | 0.035 | 0.009 | 0.002 | 0.000 | |||||
524 (Aug 2024) | 0.332 | 0.253 | 0.129 | 0.049 | 0.015 | 0.004 | 0.001 | 0.000 | ||||
525 (Sep 2024 | 0.308 | 0.265 | 0.152 | 0.065 | 0.022 | 0.006 | 0.002 | 0.000 | 0.000 | |||
526 (Oct 2024) | 0.283 | 0.270 | 0.172 | 0.082 | 0.031 | 0.010 | 0.003 | 0.001 | 0.000 | 0.000 | ||
527 (Nov 2024) | 0.257 | 0.270 | 0.189 | 0.099 | 0.042 | 0.015 | 0.004 | 0.001 | 0.000 | 0.000 | 0.000 | |
528 (Dec 2024) | 0.232 | 0.266 | 0.203 | 0.116 | 0.053 | 0.020 | 0.007 | 0.002 | 0.000 | 0.000 | 0.000 | 0.000 |
Months | n | |||||||||||
1 | 2 | 3 | 4 | 5 | 6 | 7 | 8 | 9 | 10 | 11 | 12 | |
517 (Jan 2024) | 0.156 | |||||||||||
518 (Feb 2024) | 0.258 | 0.049 | ||||||||||
519 (Mar 2024) | 0.321 | 0.090 | 0.017 | |||||||||
520 (Apr 2024) | 0.354 | 0.133 | 0.033 | 0.006 | ||||||||
521 (May 2024) | 0.367 | 0.173 | 0.054 | 0.013 | 0.002 | |||||||
522 (Jun 2024) | 0.365 | 0.206 | 0.077 | 0.022 | 0.005 | 0.001 | ||||||
523 (Jul 2024) | 0.353 | 0.232 | 0.102 | 0.034 | 0.009 | 0.002 | 0.000 | |||||
524 (Aug 2024) | 0.334 | 0.251 | 0.126 | 0.047 | 0.014 | 0.004 | 0.001 | 0.000 | ||||
525 (Sep 2024 | 0.311 | 0.264 | 0.149 | 0.063 | 0.021 | 0.006 | 0.001 | 0.000 | ||||
526 (Oct 2024) | 0.287 | 0.270 | 0.169 | 0.080 | 0.030 | 0.009 | 0.003 | 0.001 | 0.000 | |||
527 (Nov 2024) | 0.261 | 0.270 | 0.186 | 0.096 | 0.040 | 0.014 | 0.004 | 0.001 | 0.000 | 0.000 | ||
528 (Dec 2024) | 0.236 | 0.267 | 0.201 | 0.113 | 0.051 | 0.019 | 0.006 | 0.002 | 0.000 | 0.000 | 0.000 | 0.000 |