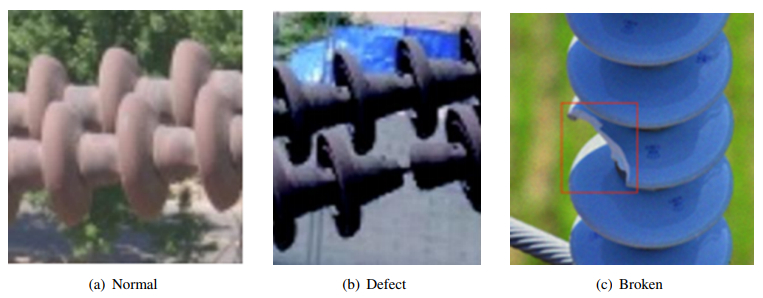
Insulators play a crucial role in transmission lines. Insulators exposed to natural environments are prone to various malfunctions. These faults will seriously affect the safety and stability of the power grid system operation, so intelligent detection of insulator defects has become increasingly important. This paper presents an insulator defect detection model based on the improved MViTv2-T (Multiscale Vision Transformers Version 2 Tiny). The new model utilizes the sore penalty mechanism (SPM) cluster non-maximum suppression (NMS) algorithm instead of the batched non-maximum suppression (NMS) algorithm from the original model. Additionally, it introduces the stage query recollection method, which integrates high-level and low-level module queries within each stage, along with various experimentation on integration functions between the two. The experimental results indicate that the improved MViTv2-T model attains an mAP (mean average precision)@0.5:0.95 of 76.1%, mAP@0.5 of 96.1%, and mAR@0.5 of 97.2% in insulator defect detection. Compared to the original model, there is a 1.8% increase in mAP@0.5:0.95 and a 17% decrease in the detection error rate at an Intersection over Union (IoU) threshold of 0.5. Furthermore, when compared to standard two-stage detection models and YOLO series models, the improved MViTv2-T model also exhibits distinct performance advantages.
Citation: Fuhong Meng, Guowu Yuan, Hao Zhou, Hao Wu, Yi Ma. Improved MViTv2-T model for insulator defect detection[J]. AIMS Electronics and Electrical Engineering, 2025, 9(1): 1-25. doi: 10.3934/electreng.2025001
[1] | Hakkee Jung . Analysis of drain induced barrier lowering for junctionless double gate MOSFET using ferroelectric negative capacitance effect. AIMS Electronics and Electrical Engineering, 2023, 7(1): 38-49. doi: 10.3934/electreng.2023003 |
[2] | Youness Chawki, Khalid Elasnaoui, Mohamed Ouhda . Classification and detection of Covid-19 based on X-Ray and CT images using deep learning and machine learning techniques: A bibliometric analysis. AIMS Electronics and Electrical Engineering, 2024, 8(1): 71-103. doi: 10.3934/electreng.2024004 |
[3] | Abdullah Yahya Abdullah Amer, Tamanna Siddiqu . A novel algorithm for sarcasm detection using supervised machine learning approach. AIMS Electronics and Electrical Engineering, 2022, 6(4): 345-369. doi: 10.3934/electreng.2022021 |
[4] | Martin Seilmayer, Varun Kumar Katepally . Thermal conductivity survey of different manufactured insulation systems of rectangular copper wires. AIMS Electronics and Electrical Engineering, 2018, 2(1): 27-36. doi: 10.3934/ElectrEng.2018.1.27 |
[5] | Sebin J Olickal, Renu Jose . LSTM projected layer neural network-based signal estimation and channel state estimator for OFDM wireless communication systems. AIMS Electronics and Electrical Engineering, 2023, 7(2): 187-195. doi: 10.3934/electreng.2023011 |
[6] | Abdul Yussif Seidu, Elvis Twumasi, Emmanuel Assuming Frimpong . Hybrid optimized artificial neural network using Latin hypercube sampling and Bayesian optimization for detection, classification and location of faults in transmission lines. AIMS Electronics and Electrical Engineering, 2024, 8(4): 508-541. doi: 10.3934/electreng.2024024 |
[7] | D Venkata Ratnam, K Nageswara Rao . Bi-LSTM based deep learning method for 5G signal detection and channel estimation. AIMS Electronics and Electrical Engineering, 2021, 5(4): 334-341. doi: 10.3934/electreng.2021017 |
[8] | M V Raghunathareddy, G Indumathi, K R Niranjan . Highly sensitive optical MEMS based photonic biosensor for colon tissue detection. AIMS Electronics and Electrical Engineering, 2022, 6(3): 285-295. doi: 10.3934/electreng.2022017 |
[9] | J. Rajeshwari, M. Sughasiny . Modified PNN classifier for diagnosing skin cancer severity condition using SMO optimization technique. AIMS Electronics and Electrical Engineering, 2023, 7(1): 75-99. doi: 10.3934/electreng.2023005 |
[10] | Ruyi Dong, Kai Yang, Tong Wang . Research on tracking strategy of manipulator based on fusion reward mechanism. AIMS Electronics and Electrical Engineering, 2025, 9(1): 99-117. doi: 10.3934/electreng.2025006 |
Insulators play a crucial role in transmission lines. Insulators exposed to natural environments are prone to various malfunctions. These faults will seriously affect the safety and stability of the power grid system operation, so intelligent detection of insulator defects has become increasingly important. This paper presents an insulator defect detection model based on the improved MViTv2-T (Multiscale Vision Transformers Version 2 Tiny). The new model utilizes the sore penalty mechanism (SPM) cluster non-maximum suppression (NMS) algorithm instead of the batched non-maximum suppression (NMS) algorithm from the original model. Additionally, it introduces the stage query recollection method, which integrates high-level and low-level module queries within each stage, along with various experimentation on integration functions between the two. The experimental results indicate that the improved MViTv2-T model attains an mAP (mean average precision)@0.5:0.95 of 76.1%, mAP@0.5 of 96.1%, and mAR@0.5 of 97.2% in insulator defect detection. Compared to the original model, there is a 1.8% increase in mAP@0.5:0.95 and a 17% decrease in the detection error rate at an Intersection over Union (IoU) threshold of 0.5. Furthermore, when compared to standard two-stage detection models and YOLO series models, the improved MViTv2-T model also exhibits distinct performance advantages.
With the rapid industrial development in China, the demand for electricity has steadily increased, leading to the expansion of various power facilities such as transmission lines. Insulators play critical roles in these lines, providing essential electrical insulation and mechanical support functions. Exposed to the natural environment, insulators are prone to damage like defects and brokenness caused by diverse and harsh weather conditions. These issues can significantly compromise the safety and stability of the power grid system, emphasizing the vital need for regular insulator inspections[1]. Manual inspection remains the primary maintenance method, with power companies relying heavily on manual checks and maintenance of crucial transmission line components, including insulators. However, the continuous expansion of the power grid, the proliferation of long-distance transmission lines, and the placement of lines in remote mountainous areas have posed numerous challenges for manual inspections, marked by high costs and operational complexities.
In recent years, the proliferation of artificial intelligence technology has led to the widespread application of defect detection methods based on deep learning across various engineering domains, significantly impacting the field [2,3]. Numerous researchers have proposed deep learning-based insulator defect detection methods. [4] introduced the bidirectional feature pyramid network (BiFPN) module into YOLOv5, and then the BiFPN module and SimAM were combined, achieving higher detection accuracy while maintaining a high detection speed. [5] merged YOLOv3 with SRCNN. Experimental findings indicate that this approach boosts detection accuracy by 1% to 3% compared to Faster R-CNN and SSD, offering improved speed and nearly achieving real-time performance. However, challenges persist in detecting small targets. [6] proposed an improved YOLOv8n-based insulator defect detection model, introducing a triplet attention module. They put forward a lighter SC-Detect to replace the original SC-Detect and reconstructed the neck structure using GSConv-based Slim-neck, reducing the model's parameters and computational load to meet the requirements of high accuracy and real-time performance. [7] presented a multi-scale insulator defect detection approach, which is introduced by utilizing the detection transformer (DETR). The approach includes a multi-scale backbone, a self-attention upsampling (SAU) module, and the insulator defect (IDIoU) loss function, resulting in exceptional performance in detecting small defects. [8] introduced a dense connection architecture incorporating multi-scale features, an adaptive weight transfer module operating at multiple scales, and a multi-branch detection unit. This architecture successfully enables the accurate identification and precise localization of insulator defects, outperforming the comparison algorithm in terms of both accuracy and speed. [9] presented an insulator defect detection framework in an unsupervised image reconstruction manner. Collecting and using the catenary insulator defect (CID) dataset, they achieved high accuracy without manual annotations. [10] presented an enhanced Faster R-CNN algorithm that employs the ResNeSt network as its backbone. This improved model integrates the regional proposal network (RPN) within the ResNeSt network to boost the extraction of defect features, leading to a heightened detection accuracy of 98.38% for insulator defects. [11] proposed a detection method based on a microwave technique and an automatic detection system to detect the internal defects of composite insulators, performing efficiently, while being labor-saving and robust. [12] proposed a coordinate attention mechanism (CAM) and feature channel shuffle operation (CSO) YOLO (CACS-YOLO). Using synthetic weather algorithms for data enhancement and introducing the CAM and CSO in the YOLOv8m model, they improved the detection precision and reduced the parameters of the model.
Several challenges arise when detecting crucial components in transmission lines like insulators, including substantial scale variations in detection targets involving numerous small and medium-sized objects[13,14], intricate backgrounds, and occlusion occurrences[15,16]. To address these challenges while catering to practical engineering needs, it is crucial to strike a balance between detection precision and speed. Consequently, the current research focuses on creating a lightweight, precise, and robust defect detection model[17,18].
In this paper, we propose a defect detection model for insulator defect detection according to the requirements of intelligent inspection of power grids. Unlike other research that widely applies the YOLO model in the field of insulator defect detection, we innovatively introduce the MViTv2 model based on transformers. Unlike other research that tends to add complex modules to improve performance, we attempt to introduce no additional modules or increase complex computational processes, focusing instead on fully integrating and utilizing the features learned by each layer of the model. In this way, we achieve higher detection accuracy without introducing additional parameters and significantly slowing the detection speed. Our model's metrics meet the engineering application standards and can be applied to the front-end acquisition equipment for power grid inspection and monitoring.
The insulator defect dataset in this paper mainly comes from the State Grid Corporation of China and Yunnan Limited Company of China Southern Power Grid. All insulator images are sourced from real insulators on China's power grid transmission lines, and collected through fixed or drone photography. Due to the difficulty of collection, the sample size is limited. The State Grid Corporation of China provides the normal type and the defect type insulator samples, with 600 and 248 images, respectively; Yunnan Limited Company of China Southern Power Grid provides the broken type and the defect type insulator samples, with 232 and 134 images each. Therefore, there are 600 images for normal insulators, 382 for defect insulators, and 232 for broken insulators, totaling 1214 images.
The insulator is composed of insulator units and metal connectors. Insulators with all intact insulator units are the normal type insulators; insulators with one or more broken insulator units are the broken type insulators, which can still perform their normal functions but need to be replaced; insulators with one or more missing insulator units are the defect type insulators, which have completely lost their normal functions and need to be replaced urgently. Figure 1 shows the three categories of labeled targets.
This paper visualizes statistics on the dataset, as shown in Figure 2. Figure 2(a) displays the number of samples for each category, Figure 2(b) presents the width and height of detected targets relative to the image, and Figure 2(c) shows the statistics of all image sizes.
From Figure 2(a), it can be seen that in the insulator defect dataset, the majority of detected targets are normal insulators, while the number of broken and defect insulator targets is relatively small, indicating the presence of a sample imbalance issue in the dataset.
Observing Figure 2(b), it is evident that over half of the detection targets in the insulator defect dataset cover a small area of the whole image, while some targets occupy a larger area, highlighting noticeable variations in target sizes. This underscores the necessity for the model to possess robust multi-scale detection capabilities.
Based on Figure 2(c), it is clear that more than half of the images in the insulator defect dataset have dimensions below 2000 x 2000. However, a notable fraction of images exhibit larger dimensions, indicating an uneven distribution of image sizes within the dataset. During model training, images are usually resized, which can result in the loss of features in oversized images.
All images contain one or more detection targets, typically against backgrounds of hills, land, sky, forests, and grasslands, which are varied and complex. The dataset also includes cases of target occlusion, adding to the difficulty of detection.
Considering the dataset characteristics and practical requirements, this paper utilizes Facebook's multiscale vision transformers (MViTv2) model as the original model and opts for its tiny version model with fewer parameters and quicker detection speed. Subsequently, the model will be improved to better align with the insulator defect dataset and enhance defect detection performance.
In practical engineering applications, the detection model needs to be integrated into the cameras for real-time detection in crucial areas (substations and abnormal monitoring areas); in non-crucial areas, the cameras take scheduled photos and transmit them to the data center for detection. Thus, the detection model should have faster speed and higher accuracy under the same configuration. The MViTv2 model is a deep learning model derived from ViT (vision transformer). Its accuracy and speed are aligned with the demands of practical engineering applications. In this paper, we choose the MViTv2-T model as the original model. Based on the characteristics of our application, we replace the NMS algorithm and propose the stage query recollection method.
The MViTv1[19] model was proposed by Facebook in 2021 to address the issues of large parameters and computational complexity in ViTs by introducing attention pooling, which reduces the number of parameters and computational burden. The MViTv2[20] model, improved and released by Facebook in 2022. Figure 3 shows the self-attention mechanism of the MViTv1 model and the MViTv2 model for comparison.
The main improvement of MViTv2 is the relative position embedding and residual pooling connection, as shown in the red part of Figure 3(b). MViTv1's modeling between two tokens only depends on their absolute positions in the image, even if their relative positions remain unchanged, this ignores the fundamental principle of shift-invariance in vision. To address this, MViTv2 uses relative position embedding, which only depends on the relative location distance between tokens. From MViTv1, it is evident that pooling attention significantly reduces computational complexity and memory requirements, and enhances the network's feature extraction capability. To better train the pooling attention blocks, MViTv2 employs a residual pooling connection, which increases information flow.
The formula for the self-attention mechanism calculation in the MViTv2 model is
Attn(Q,K,V)=softmax(QKT√dk)V+Q | (1) |
where, Q, K, and V are the query, key, and value matrices obtained through Linearq,k,v, and dk is the dimension of the key matrix.
The MViTv2-T model is divided into three parts: feature extraction module (Backbone with FPN), RPN, and ROI. The structure of the MViTv2-T model is shown in Figure 4.
The backbone of the MViTv2-T model comprises ten interconnected MViT blocks organized into 4 stages. The structure of the backbone is depicted in Figure 5.
The MViTv2-T model is a two-stage detection model that uses the batched NMS algorithm implemented in Torchvision to suppress the candidate boxes obtained by RPN. The principle of the traditional NMS algorithm is to perform NMS on all bounding boxes (BBox) together. The difference of the batched NMS algorithm lies in performing NMS only on the BBoxes within each category, while the calculation principle is the same. Therefore, this algorithm suffers the same drawback as the traditional NMS algorithm: even if the number of candidate boxes before and after NMS is restricted by hyperparameters, there are still too many redundant candidate boxes, further affecting the subsequent detection performance. To avoid this situation and balance accuracy and speed, this paper considers using the SPM cluster NMS[21] algorithm to replace the batched NMS algorithm. The pseudocode for the SPM Cluster NMS algorithm is shown below:
Algorithm 1 SPM Cluster NMS Algorithm | |
Input: | |
boxes (Tensor[N, 4]) | ▹ Bounding boxes |
scores (Tensor[N, 1]) | ▹ Scores for each box |
NMS_threshold (float) | ▹ IoU threshold for NMS |
Output: | |
boxes_kept (Tensor[M, 4]) | ▹ Filtered bounding boxes |
scores_kept (Tensor[M, 1]) | ▹ Filtered scores |
1: scores, idx ← sort(scores, descending = True) | ▹ Sort scores and get indices |
2: boxes ← boxes[idx] | ▹ Rearrange boxes based on sorted indices |
3: iou ← box_iou(boxes, boxes) | ▹ Compute IoU matrix |
4: C ← triu(iou, diagonal = 1) | ▹ Upper triangular IoU matrix |
5: Initialize i←0 | |
6: while i<200 do | |
7: A ← C | ▹ Store current IoU matrix |
8: maxA ← max(A, dim = 0)[0] | ▹ Find column maximum values |
9: E ← (maxA < NMS_threshold) | ▹ Create exclusion mask |
10: C ← iou ⋅ E | ▹ Element-wise multiplication with IoU |
11: if A.equal(C) then | |
12: break | ▹ Exit loop if stable |
13: end if | |
14: i←i+1 | |
15: end while | |
16: scores ← prod(exp(−C2/0.2), 0) ⋅ (scores.squeeze(1)) | ▹ Penalty on scores |
17: keep ← scores > 0.01 | ▹ Apply score thresholding |
18 return boxes[keep], scores[keep] | ▹ Return filtered boxes and scores |
This NMS algorithm introduces the score penalty mechanism (SPM), and its formula is
Scoresj=Scoresj∏ie−C2ij0.2 | (2) |
where, Scores is a tensor of size N representing the scores of N candidate boxes in the preliminary detection of RPN, and C is the intermediate matrix in the NMS calculation process.
The IoU matrix represents the relevance between boxes. In Algorithm 1, the IoU matrix is an upper triangular matrix calculated after score-descending sorting Boxes and Scores. When i<j, the IoUij is meaningful and represents the similarity between Boxi and Boxj, with Scorei≥Scorej. The larger the IoUij, the higher the similarity between the two boxes, and the greater the likelihood of retaining only one. The iteratively derived C matrix also possesses this characteristic. The SPM penalizes the score of the box by quantifying the likelihood, and due to the characteristics of the upper triangular matrix, Scorej is only penalized by those boxes with higher scores than Scorej. For the calculation process of the SPM, refer to the example in Figure 6.
In Figure 6, C02=0.13 implies that the similarity between Box0 and Box2 is 0.13, which indicates a high probability of retaining both boxes simultaneously. Therefore, by calculating, the similarity of 0.13 is transformed into a penalty ratio of 0.92, signifying that Box0 exerts a minimal penalty on Box2. Similarly, the penalty ratio 0.93 indicates the penalty exerted by Box1 on Box2. When these values are multiplied column-wise, the penalty ratio 0.86 represents the combined penalty of Box0 and Box1 on Box2, where both Score0 and Score1 are greater than Score2.
Because most insulators are located in the wild with complex and changing backgrounds, and are constrained by shooting angles leading to common occlusion phenomena, some missed and false detections occur during detection. To address missed detections and false detections caused by complex backgrounds and occlusions, this paper proposes integrating the query of high-level modules and the query of low-level modules in each stage, fully utilizing contextual information to enhance the saliency of detection targets, helping the model better locate features and extract features, known as stage query recollection. This method can fully integrate and utilize contextual image information and semantic information, enhancing the saliency of detection targets in situations like complex backgrounds and occlusions. The stage query recollection method is shown in Figure 7.
The formula for the self-attention mechanism after the stage query recollection method is
Attn{Q,K,V}=softmax(fn(Qhigh,Qlow)KT√dk)V+Qlow | (3) |
where Qhigh represents the query of the MViT Block with high-level, Qlow represents the query of the MViT Block with low-level, and fn is the function that integrates the Qhigh and the Qlow.
Initially, we present three basic integration functions fn to integrate two queries, each with progressively increasing parameters from left to right, as depicted in Figure 8.
The Conv block consists of Conv2d, GELU, and BatchNorm, while the inner block consists of 2 conv block, and Conv2d, as shown in Figure 9(a) and in Figure 9(b), respectively.
In Figure 9(b), the inner block imitates the CNN and transformer fusion module in the experimental part of TransXNet[22], where the inner block equals max(16,C/Ratio), and Ratio is a manually set hyperparameter, while Figure 9(a) represents the basic module simplified from inner block.
The three basic fn functions in Figure 8 are
f1(Qhigh,Qlow)=Qhigh+Qlowf2(Qhigh,Qlow)=Conv(Concat(Qhigh,Qlow))f3(Qhioh,Qlow)=Inner(Concat(Qhioh,Qlow),Ratio) | (4) |
where Concat is the concatenation of matrices along the channel dimension, and Conv and Inner represent the Conv block and the inner block, respectively.
The three basic fn functions proposed above assume equal importance between Qhigh and Qlow: the proportion between the two is 1:1, meaning the importance of the two is equal. Furthermore, introducing additional blocks into the model increases the number of parameters. To decrease the additional number of parameters, the hypothesis is made that perhaps the importance of integrating the query of high-level and low-level modules is not equal. Hence, this paper introduces an additional hyperparameter representing the proportion between Qhigh and Qlow, as illustrated in Figure 10.
The three fn functions in Figure 10 are
f4(Qhigh,Qlow)=W0×Qhigh+W1×Qlowf5(Qhigh,Qlow)=Conv(Concat(W0×Qhigh,W1×Qlow))f6(Qhigh,Qlow)=Inner(Concat(W0×Qhigh,W1×Qlow),Ratio) | (5) |
where, W0 represents the ratio of Qhigh, and W1 represents the ratio of Qlow.
Due to the insufficient sample images and the sample imbalance, it is necessary to augment the data. The data augmentation in this paper and its examples are shown in Figure 11.
After data augmentation, the numbers of the training set, validation set, and test set are 1800,600, and 600, respectively, with an equal number of images for each of the three categories in each subset.
This paper visualizes statistics on the dataset after data augmentation, as shown in Figure 12. Figure 12(a) displays the number of various detection targets after data augmentation, Figure 12(b) presents the width and height of detected targets relative to the image after data augmentation, and Figure 12(c) shows the statistics of all image sizes after data augmentation.
The operating system used in the experiment is Windows 11, and the GPU selected is an Nvidia GeForce RTX 4090. The deep learning framework utilized is Detectron2. During training, multi-scale training is employed (scaling the shorter edge to the range [480,800] and ensuring the longer edge is under 1333). The optimization is performed using the AdamW optimizer (with β1 = 0.9, β2 = 0.999, the initial learning rate of 1.6×10−4, Batch size of 4, and weight decay of 0.1). The loss functions used during training are the smoothing L1 loss and the cross-entropy Loss, respectively. The total training duration is 150 epochs. The drop path rate is configured as 0.1. We employed automatic mixed precision in PyTorch for training, utilizing the pretrained Mask RCNN-MViTv2-T model trained on Imagenet-1k.
This paper utilizes some of the evaluation metrics from COCO 2017 for experimental assessment, including average precision (AP), AP50, AP75, AR50, and FPS. To align with commonly used metrics, this paper conducted conversions. AP corresponds to mAP@0.5:0.95, which involves computing mAP across IoU thresholds from 0.5 to 0.95 in intervals of 0.05, then averaging the results. AP50 and AP75 represent mAP@0.5 and mAP@0.75, respectively, indicating average precision at IoU thresholds of 0.5 and 0.75. AR50 is equivalent to mAR@0.5, denoting the ratio of correct predictions to all positive predictions at an IoU threshold 0.5. FPS stands for frames per second, signifying the detection model's processing speed in images per second. In this research, uniform evaluation metrics in the table are employed, namely mAP@0.5:0.95, mAP@0.5, mAP@0.75, mAR@0.5, and FPS.
The formulas for calculating precision(P), recall(R), mean average precision(mAP), average recall(AR), and mean average recall(mAR) are
P=TPTP+FPR=TPTP+FN | (6) |
where TP indicates a positive sample correctly predicted as positive, FP indicates a negative sample incorrectly predicted as positive, and FN denotes a positive sample incorrectly predicted as negative, representing a missed detection.
The formulas for mean average precision(mAP), average recall(AR), and mean average recall(mAR) are as follows:
AP=∫10p(r)drmAP=K∑i=1APiKAR=2∫10.5recall(o)domAR=K∑i=1ARiK | (7) |
where K represents the total number of categories, and APi and ARi represent the AP and AR of category i, respectively.
To mitigate the problem of excessive redundant candidate boxes generated during the NMS stage by the detection model, this paper explores the substitution of the Batched NMS algorithm. The experimental investigation includes the evaluation of various improved NMS algorithms such as soft NMS[23], DIoU NMS[24], fast NMS[25], cluster NMS, and SPM cluster NMS.
The batched NMS algorithm is retained for model training, following the procedures outlined in the soft NMS paper. However, different improved NMS algorithms are employed for testing and comparative analysis during the evaluation of the test model's detection performance. Table 1 presents comparative experiments of each improved NMS algorithm.
Method | mAP @0.5:0.95/% |
mAP@0.5/% | mAP@0.75/% | mAR@0.5/% | FPS |
Original model | 74.3 | 95.3 | 87.3 | 95.8 | 22.2 |
Soft NMS | 75.3 | 95.4 | 87.6 | 96.1 | 0.5 |
DIoU NMS | 74.5 | 95.4 | 87.9 | 96.1 | 0.8 |
Fast NMS | 67.9 | 88.2 | 79.7 | 89.3 | 20.6 |
Cluster NMS | 74.7 | 95.4 | 87.1 | 96.2 | 17.2 |
SPM Cluster NMS | 75.4 | 95.4 | 87.4 | 96.2 | 17.1 |
From Table 1, it can be illustrated that the soft NMS algorithm exhibits high detection accuracy; however, its sequential structure underutilizes the GPU's high-speed computing capabilities, resulting in a noticeable drop in detection speed. The DIoU NMS algorithm also shows slow detection speed without improving detection performance. In contrast, the fast NMS, cluster NMS, and SPM cluster NMS algorithms demonstrate similar detection speeds. Specifically, the fast NMS algorithm achieves low detection accuracy, the cluster NMS algorithm only marginally enhances detection accuracy, while the SPM cluster NMS algorithm matches the soft NMS algorithm's performance with a moderate FPS reduction. Hence, this indicates the suitability of the SPM cluster NMS algorithm for the insulator defect dataset analyzed in this paper. Consequently, the SPM cluster NMS algorithm is selected as the replacement for the traditional NMS algorithm in this research.
After replacing the traditional NMS algorithm with the SPM cluster NMS algorithm, this paper visualizes the detection boxes selected by RPN, as shown in Figure 13. The left image in Figure 13 is the RPN detection image using the batched NMS algorithm, while the right image is the RPN detection image using the SPM cluster NMS algorithm, with green boxes visualizing the candidate boxes.
This paper proposes implementing the Stage Query Recollection method and explores different integration functions to tackle the challenges posed by missed detections and false alarms resulting from complex backgrounds and occlusions.
This paper first conducts experimental comparisons of three basic integration functions, with Table 2 comparing experimental results.
method | hyperparameter | mAP @0.5:0.95/% |
mAP@0.5/% | mAP@0.75/% | mAR@0.5/% | FPS | #Params |
Original model | - | 74.3 | 95.3 | 87.3 | 95.8 | 22.2 | 41.0M |
f1 | - | 75.2 | 95.7 | 87.3 | 95.3 | 22.6 | 41.0M |
f2 | - | 74.0 | 95.3 | 86.9 | 96.0 | 15.7 | 41.1M |
f3 | Ratio=0.5 | 75.3 | 96.0 | 87.7 | 95.3 | 21.4 | 41.6M |
Ratio=1 | 75.6 | 96.4 | 87.0 | 97.0 | 21.7 | 41.3M | |
Ratio=4 | 75.0 | 95.6 | 86.9 | 96.4 | 21.9 | 41.1M |
From Table 2, it can be seen that after the introduction of stage query recollection, using f1 as the integration function, i.e., directly adding the high-level module Query with the low-level module Query, the experimental results show that while maintaining closeness to the original detection model in terms of FPS, there is an improvement of 0.9% and 0.4% in mAP@0.5:0.95 and mAP@0.5, respectively, without introducing additional parameters. Using f2 as the integration function, i.e., a Conv block, the experimental results show that after introducing an additional parameter load of 0.1M, almost all indicators exhibited varying degrees of decline. Using f3 as the integration function, i.e., the inner block with the hyperparameter ratio set to 1, the experimental results show that its overall performance is optimal, with around a 1% improvement in metrics such as mAP@0.5:0.95, mAP@0.5, and mAR@0.5. However, this integration function introduces an additional parameter load of 0.3 M.
This paper then introduces two hyperparameters based on the above three basic integration functions and conducts experiments.
Table 3 shows comparative experiments of three integration functions after the inclusion of hyperparameters.
method | hyperparameter | mAP @0.5:0.95/% |
mAP @0.5/% |
mAP @0.75/% |
mAR @0.5/% |
FPS | #Params |
Original model | - | 74.3 | 95.3 | 87.3 | 95.8 | 22.2 | 41.0M |
f3 | Ratio=1 | 75.6 | 96.4 | 87.0 | 97.0 | 21.7 | 41.3M |
f4 | W0=0.7,W1=0.3 | 75.2 | 95.9 | 86.7 | 96.7 | 22.8 | 41.0M |
W0=0.6,W1=0.4 | 75.5 | 96.4 | 87.7 | 97.2 | 22.6 | ||
W0=0.4,W1=0.6 | 75.1 | 95.3 | 87.8 | 96.0 | 22.7 | ||
W0=0.3,W1=0.7 | 74.8 | 95.8 | 86.3 | 96.7 | 22.6 | ||
f5 | W0=0.7,W1=0.3 | 74.3 | 95.3 | 86.6 | 96.1 | 15.8 | 41.1M |
W0=0.6,W1=0.4 | 75.0 | 95.3 | 86.8 | 96.1 | 15.7 | ||
W0=0.4,W1=0.6 | 74.5 | 95.7 | 88.1 | 96.5 | 15.6 | ||
W0=0.3,W1=0.7 | 74.0 | 95.1 | 86.8 | 95.8 | 15.7 | ||
f6 Ratio=1 |
W0=0.7,W1=0.3 | 74.9 | 95.4 | 87.7 | 96.5 | 21.5 | 41.3M |
W0=0.6,W1=0.4 | 74.8 | 95.6 | 86.9 | 96.1 | 21.3 | ||
W0=0.4,W1=0.6 | 75.1 | 95.7 | 86.4 | 96.4 | 21.4 | ||
W0=0.3,W1=0.7 | 74.9 | 95.3 | 87.3 | 95.9 | 21.4 |
From Table 3, it can be seen that after introducing stage query recollection, using the f4 with W0=0.6 and W1=0.4, the experimental results show that its metrics of mAP@0.5:0.95 and mAP@0.5 are similar to those of the f3 with Ratio=1, and it outperforms the latter in terms of mAP@0.75 and does not introduce additional parameter load. Using f5 and f6 with Ratio=1 as integration functions, after introducing hyperparameters, these two integration functions did not show a significant overall improvement. Among them, the mAP@0.75 metric of f5 with W0=0.4 and W1=0.6 was the highest. In conclusion, this paper considers that f3 with Ratio=1 and f4 with W0=0.6 and W1=0.4 are more suitable for the insulator defect dataset in this paper.
Figure 14 shows the visual feature map comparison of the original model, f3 with Ratio=1, and f4 with W0=0.6 and W1=0.4. From left to right, the order is the original model, f3, and f4, with the detection targets highlighted in green boxes. In Figure 14, the color range from blue to green and then to red represents the attention level from low to high. Blue represents low attention, while red represents high attention.
In Figure 14, the green boxes represent the annotated boxes. It can be seen that, in the feature map generated by the original model on the far left, some annotated box positions either do not pay attention to features, or the level of attention is low, which cannot effectively assist in the subsequent classification stage, resulting in missed detections and false detections. And, other conclusions can be drawn: After adding f3 with Ratio=1 and f4 with W0=0.6 and W1=0.4, the model pays more attention to some detection targets with complex backgrounds, occlusions, and some targets at the edges, avoiding some missed detections and false positives, thereby improving the detection accuracy of the model.
To further validate the effectiveness of all the proposed improvements in this paper, a detailed ablation experiment is conducted on the proposed improvements. Table 4 shows the results of the ablation experiment.
SPM Cluster NMS | f6 (Ratio=1) |
f4 (W0=0.6,W1=0.4) |
mAP @0.5:0.95/% |
mAP @0.5/% |
mAP @0.75/% |
74.3 | 95.3 | 87.3 | |||
✔ | 75.4 | 95.4 | 87.4 | ||
✔ | 75.6 | 96.4 | 87.0 | ||
✔ | 75.5 | 96.4 | 87.7 | ||
✔ | ✔ | 76.0 | 96.3 | 87.1 | |
✔ | ✔ | 76.1 | 96.2 | 87.9 |
From Table 4, the following conclusions can be drawn. The original MViTv2-T model's mAP@0.5:0.95 and mAP@0.5 stabilize at 74.3% and 95.3% respectively; the improved MViTv2-T model outlined above surpasses the original model in terms of both mAP@0.5:0.95 and mAP@0.5 performance metrics. Specifically, with the inclusion of f3 with Ratio=1 and the SPM cluster NMS algorithm, the improved MViTv2-T model achieves mAP@0.5:0.95 and mAP@0.5 stabilizing at 76.0% and 96.3%, respectively; with the inclusion of f4 with W0=0.6 and W1=0.4, and the SPM Cluster NMS algorithm, the improved MViTv2-T model achieves mAP@0.5:0.95 and mAP@0.5 stabilizing at 76.1% and 96.2%, respectively. Hence, when contrasting the improved MViTv2-T model, which employs two distinct integration functions, with the original MViTv2-T model, there is an increase of approximately 1.8% in mAP@0.5:0.95 and about 1.0% in mAP@0.5.
This paper compares the improved MViTv2-T model with the original MViTv2-T model, and the detection results are shown in Figure 15. The types are defined as follows: type 0 for broken, type 1 for defect, and type 2 for normal.
From Figure 15, the improved MViTv2-T model in this paper performs better in detecting some missed and false detection targets compared to the original model. Additionally, for some detection targets with relatively low scores, the improved MViTv2-T model will assign higher scores.
Figure 16 shows the contrast in mAP@0.5:0.95 and mAP@0.5 curves for our models. The red curve represents the original MViTv2-T model, the blue curve represents the MViTv2-T model using f3 with Ratio=1, and the green curve represents the MViTv2-T model using f4 with W0=0.6 and W1=0.4.
Figure 17 shows the loss iteration process of the MViTv2-T model using f3 with Ratio=1 and f4 with W0=0.6 and W1=0.4. The entire training process used an NVIDIA GeForce RTX 4090 GPU with 24GB memory and lasted 14 hours.
To objectively assess the advantages of the improvements in this paper, the improved MViTv2-T model is compared with commonly used defect detection models in industry. All defect detection models are trained on the same augmented dataset with the same software/hardware environment, using the same parameters during training. In the same insulator defect dataset, the comparative experiments with other models are shown in Table 5.
Models | mAP @0.5:0.95/% |
mAP@0.5/% | mAP@0.75/% | mAR@0.5/% | FPS | GFLOPs |
RetinaNet | 64.3 | 86.8 | 74.1 | 92.4 | 12.6 | 93.6 |
Faster-RCNN | 66.1 | 88.6 | 76.7 | 90.4 | 13.4 | 38.5 |
Mask-RCNN | 65.2 | 87.1 | 77.4 | 88.7 | 13.4 | 9.2 |
Cascade-RCNN | 69.0 | 87.9 | 78.6 | 90.2 | 8.5 | 9.2 |
FCOS | 53.2 | 81.6 | 60.7 | 90.6 | 13.3 | 80.1 |
YOLOv5n | 64.8 | 90.8 | 74.1 | 85.2 | 52.9 | 4.2 |
YOLOv5m | 73.5 | 94.5 | 85.3 | 91.6 | 31.1 | 48.2 |
YOLOv5l | 74.4 | 95.5 | 86.9 | 93.3 | 25.8 | 108.3 |
YOLOv7t | 66.2 | 90.8 | 74.9 | 86.1 | 44.4 | 12.9 |
YOLOv7 | 73.8 | 93.9 | 86.5 | 89.6 | 22.8 | 104.5 |
YOLOv8s | 72.3 | 91.8 | 81.3 | 88.4 | 41.3 | 28.3 |
YOLOv8m | 73.1 | 93.0 | 82.3 | 90.1 | 37.9 | 78.1 |
YOLOv8l | 73.2 | 92.7 | 81.7 | 88.1 | 29.2 | 163.9 |
YOLOv9c | 67.0 | 89.4 | 76.4 | 84.4 | 17.4 | 102.1 |
Ours-1 | 76.0 | 96.3 | 87.1 | 96.7 | 16.3 | 9.9 |
Ours-2 | 76.1 | 96.2 | 87.9 | 97.0 | 17.4 | 9.7 |
From Table 5, the following conclusions can be drawn: The improved MViTv2-T model presented in this paper demonstrates superior detection accuracy and recall performance compared to other defect detection models. Furthermore, the improved MViTv2-T model demonstrates quicker detection times compared to other analyzed models with the same number of parameters. However, it is worth noting that the improved MViTv2-T model lags slightly in FPS compared to YOLO. Given the standard 5-minute sampling interval for monitoring equipment in industrial defect detection, the higher detection accuracy provided by the improved MViTv2-T model is particularly advantageous.
In this paper, we propose the improved MViTv2-T model by replacing the batched NMS algorithm with the SPM cluster NMS algorithm, proposing stage query recollection, and further experimenting with various integration functions, the model's mAP@0.5:0.95 for detecting insulator defects in power transmission lines increases by about 1.7%, mAP@0.5 increases by about 1.0%, and mAR@0.5 increases by about 1.2%.
Unlike the pure CNN models, YOLO focuses on being lightweight and fast, and RCNN focuses on higher accuracy. This paper introduces a model based on transformers, which increases the parameters and achieves higher detection accuracy without slowing down the detection speed. This model can not only be applied to insulator defect detection, but also to other defect detection areas.
Furthermore, the experiments in this paper still have shortcomings and limitations, such as: (1) not involving broader integration functions; (2) integrating more modules of Query; (3) more fully utilizing the Query, Key, and Value matrices; (4) using mathematical methods to select the optimal hyperparameters; (5) emulating the attention mechanism in deep convolutional networks to extend the hyperparameters to the dimensions of length, width, and channel of matrices. The upcoming research will continue to delve deeper based on the improved model. Our goal is to reduce the number of model layers and explore more effective integration functions to fully utilize the feature information generated by each layer of the model. We aim to achieve higher detection performance while reducing the number of parameters, maintaining or surpassing the model's performance, making it more suitable for insulator defect detection and potentially applicable to other fields.
Fuhong Meng: Conceptualization, Software, Validation, Investigation, Resources, Writing – review & editing, Methodology, Validation; Guowu Yuan: Writing-original draft, Software, Validation, Supervision; Hao Zhou: Formal analysis; Hao Wu: Investigation, Project administration; Yi Ma: Data curation, Visualization. All authors have read and approved the final version of the manuscript for publication.
This research was financially supported by the Key R & D Projects of Yunnan Province, China (Grant No. 202202AD080004) and the Yunnan Provincial Department of Science and Technology-Yunnan University Joint Special Project for Double-Class Construction, China (Grant No. 202201BF070001-005).
The authors declare no conflicts of interest in this paper.
[1] |
Zhao ZB, Jiang ZG, Li YX, Qi YC, Zhai YJ, Zhao WQ, et al. (2021) Overview of visual defect detection of transmission line components. Journal of Image and Graphics 26: 2545–2560. https://doi.org/10.11834/jig.200689 doi: 10.11834/jig.200689
![]() |
[2] |
Chen C, Yuan GW, Zhou H, Ma Y (2023) Improved YOLOv5s model for key components detection of power transmission lines. Math Biosci Eng 20: 7738–7761. https://doi.org/10.3934/mbe.2023334 doi: 10.3934/mbe.2023334
![]() |
[3] |
Liu HY, Yuan GW (2022) Cigarette appearance defect detection method based on improved YOLOv5s. Comput Technol Dev 32: 161–167. https://doi.org/10.3969/j.issn.1673-629X.2022.08.026 doi: 10.3969/j.issn.1673-629X.2022.08.026
![]() |
[4] |
Zhang Y, Dou Y, Yang K, Song X, Wang J, Zhao L (2024) Insulator defect detection based on BaS-YOLOv5. Multimed Syst 30: 212. https://doi.org/10.1007/s00530-024-01413-w doi: 10.1007/s00530-024-01413-w
![]() |
[5] |
Chen H, He Z, Shi B, Zhong T (2019) Research on recognition method of electrical components based on YOLO V3. IEEE Access 7: 157818–157829. https://doi.org/10.1109/ACCESS.2019.2950053 doi: 10.1109/ACCESS.2019.2950053
![]() |
[6] |
Su J, Yuan Y, Przystupa K, Kochan O (2024) Insulator defect detection algorithm based on improved YOLOv8 for electric power. Signal Image Video Process 18: 6197–6209. https://doi.org/10.1007/s11760-024-03307-w doi: 10.1007/s11760-024-03307-w
![]() |
[7] |
Li D, Yang P, Zou Y (2024) Optimizing Insulator Defect Detection with Improved DETR Models. Mathematics 12: 1507. https://doi.org/10.3390/math12101507 doi: 10.3390/math12101507
![]() |
[8] |
Yuan H, Wang J (2023) Power Insulator Defect Detection Based on Multi-scale Dense Adaptive Sensing. Mathematics 2661: 012001. https://doi.org/10.1088/1742-6596/2661/1/012001 doi: 10.1088/1742-6596/2661/1/012001
![]() |
[9] |
Zhang T, Zhong S, Xu W, Yan L, Zou X (2024) Catenary Insulator Defects Detection: A Dataset and an Unsupervised Baseline. IEEE T Instrum Meas 73: 1–15. https://doi.org/10.1109/TIM.2024.3390695 doi: 10.1109/TIM.2024.3390695
![]() |
[10] |
Wang S, Liu Y, Qing Y, Wang C, Lan T, Yao R (2020) Detection of insulator defects with improved ResNeSt and region proposal network. IEEE Access 8: 184841–184850. https://doi.org/10.1109/ACCESS.2020.3029857 doi: 10.1109/ACCESS.2020.3029857
![]() |
[11] |
Mei H, Jiang H, Chen J, Yin F, Wang L, Farzaneh M (2021) Detection of internal defects of full-size composite insulators based on microwave technique. IEEE T Instrum Meas 70: 1–10. https://doi.org/10.1109/TIM.2021.3085111 doi: 10.1109/TIM.2021.3085111
![]() |
[12] |
Cao Z, Chen K, Chen J, Chen Z, Zhang M (2024) CACS-YOLO: A Lightweight Model for Insulator Defect Detection based on Improved YOLOv8m. IEEE T Instrum Meas 73: 1–10. https://doi.org/10.1109/TIM.2024.3453332 doi: 10.1109/TIM.2024.3453332
![]() |
[13] |
Han G, Yuan Q, Zhao F, Wang R, Zhao L, Li S, et al. (2023) An improved algorithm for insulator and defect detection based on yolov4. Electronics 12: 933. https://doi.org/10.3390/ELECTRONICS12040933 doi: 10.3390/ELECTRONICS12040933
![]() |
[14] |
Zhao ZB, Li Y, Qi YC, Kong YH, Nie LQ (2020) Insulator defect detection method based on dynamic focus loss function and sample balance method. Electric Power Automation Equipment 40: 205–211. https://doi.org/10.16081/j.epae.202010008 doi: 10.16081/j.epae.202010008
![]() |
[15] |
Guo L, Liao Y, Yao H, Chen J, Wang M (2018) An electrical insulator defects detection method combined human receptive field model. J Control Sci Eng 2018: 2371825. https://doi.org/10.1155/2018/2371825 doi: 10.1155/2018/2371825
![]() |
[16] |
Li T, Hao T (2022) Damage detection of insulators in catenary based on deep learning and Zernike moment algorithms. Appl Sci 12: 5004. https://doi.org/10.3390/app12105004 doi: 10.3390/app12105004
![]() |
[17] |
Qi Y, Li Y, Du A (2023) Research on an insulator defect detection method based on improved yolov5. Appl Sci 13: 5741. https://doi.org/10.3390/app13095741 doi: 10.3390/app13095741
![]() |
[18] |
Zhang H, Huang G, Yang L (2023) Insulator defect detection algorithm based on multi-scale feature fusion optimization. International Conference on Algorithms, High Performance Computing, and Artificial Intelligence (AHPCAI 2023) 12941: 226–232. https://doi.org/10.1117/12.3011642 doi: 10.1117/12.3011642
![]() |
[19] |
Fan H, Xiong B, Mangalam K, Li Y, Yan Z, Malik J, et al. (2021) Multiscale vision transformers. Proceedings of the IEEE/CVF international conference on computer vision 2021: 6824–6835. https://doi.org/10.48550/arXiv.2104.11227 doi: 10.48550/arXiv.2104.11227
![]() |
[20] |
Li Y, Wu CY, Fan H, Mangalam K, Xiong B, Malik J, et al. (2022) Mvitv2: Improved multiscale vision transformers for classification and detection. Proceedings of the IEEE/CVF conference on computer vision and pattern recognition 2022: 4804–4814. https://doi.org/10.48550/arXiv.2112.01526 doi: 10.48550/arXiv.2112.01526
![]() |
[21] |
Zheng Z, Wang P, Ren D, Liu W, Ye R, Hu Q, et al. (2021) Enhancing geometric factors in model learning and inference for object detection and instance segmentation. IEEE Trans Cybern 52: 8574–8586. https://doi.org/10.1109/TCYB.2021.3095305 doi: 10.1109/TCYB.2021.3095305
![]() |
[22] | Lou M, Zhou HY, Yang S, Yu Y (2023) TransXNet: learning both global and local dynamics with a dual dynamic token mixer for visual recognition. arXiv preprint arXiv: 2310.19380. https://doi.org/10.48550/arXiv.2310.19380 |
[23] |
Bodla N, Singh B, Chellappa R, Davis LS (2017) Soft-NMS–improving object detection with one line of code. Proceedings of the IEEE international conference on computer vision 2017: 5561–5569. https://doi.org/10.48550/arXiv.1704.04503 doi: 10.48550/arXiv.1704.04503
![]() |
[24] |
Zheng Z, Wang P, Liu W, Li J, Ye R, Ren D (2020) Distance-IoU loss: Faster and better learning for bounding box regression. Proceedings of the AAAI conference on artificial intelligence 34: 12993–13000. https://doi.org/10.1609/aaai.v34i07.6999 doi: 10.1609/aaai.v34i07.6999
![]() |
[25] |
Bolya D, Zhou C, Xiao F, Lee YJ (2019) Yolact: Real-time instance segmentation. Proceedings of the IEEE/CVF international conference on computer vision 2019: 9157–9166. https://doi.org/10.48550/arXiv.1904.02689 doi: 10.48550/arXiv.1904.02689
![]() |
Method | mAP @0.5:0.95/% |
mAP@0.5/% | mAP@0.75/% | mAR@0.5/% | FPS |
Original model | 74.3 | 95.3 | 87.3 | 95.8 | 22.2 |
Soft NMS | 75.3 | 95.4 | 87.6 | 96.1 | 0.5 |
DIoU NMS | 74.5 | 95.4 | 87.9 | 96.1 | 0.8 |
Fast NMS | 67.9 | 88.2 | 79.7 | 89.3 | 20.6 |
Cluster NMS | 74.7 | 95.4 | 87.1 | 96.2 | 17.2 |
SPM Cluster NMS | 75.4 | 95.4 | 87.4 | 96.2 | 17.1 |
method | hyperparameter | mAP @0.5:0.95/% |
mAP@0.5/% | mAP@0.75/% | mAR@0.5/% | FPS | #Params |
Original model | - | 74.3 | 95.3 | 87.3 | 95.8 | 22.2 | 41.0M |
f1 | - | 75.2 | 95.7 | 87.3 | 95.3 | 22.6 | 41.0M |
f2 | - | 74.0 | 95.3 | 86.9 | 96.0 | 15.7 | 41.1M |
f3 | Ratio=0.5 | 75.3 | 96.0 | 87.7 | 95.3 | 21.4 | 41.6M |
Ratio=1 | 75.6 | 96.4 | 87.0 | 97.0 | 21.7 | 41.3M | |
Ratio=4 | 75.0 | 95.6 | 86.9 | 96.4 | 21.9 | 41.1M |
method | hyperparameter | mAP @0.5:0.95/% |
mAP @0.5/% |
mAP @0.75/% |
mAR @0.5/% |
FPS | #Params |
Original model | - | 74.3 | 95.3 | 87.3 | 95.8 | 22.2 | 41.0M |
f3 | Ratio=1 | 75.6 | 96.4 | 87.0 | 97.0 | 21.7 | 41.3M |
f4 | W0=0.7,W1=0.3 | 75.2 | 95.9 | 86.7 | 96.7 | 22.8 | 41.0M |
W0=0.6,W1=0.4 | 75.5 | 96.4 | 87.7 | 97.2 | 22.6 | ||
W0=0.4,W1=0.6 | 75.1 | 95.3 | 87.8 | 96.0 | 22.7 | ||
W0=0.3,W1=0.7 | 74.8 | 95.8 | 86.3 | 96.7 | 22.6 | ||
f5 | W0=0.7,W1=0.3 | 74.3 | 95.3 | 86.6 | 96.1 | 15.8 | 41.1M |
W0=0.6,W1=0.4 | 75.0 | 95.3 | 86.8 | 96.1 | 15.7 | ||
W0=0.4,W1=0.6 | 74.5 | 95.7 | 88.1 | 96.5 | 15.6 | ||
W0=0.3,W1=0.7 | 74.0 | 95.1 | 86.8 | 95.8 | 15.7 | ||
f6 Ratio=1 |
W0=0.7,W1=0.3 | 74.9 | 95.4 | 87.7 | 96.5 | 21.5 | 41.3M |
W0=0.6,W1=0.4 | 74.8 | 95.6 | 86.9 | 96.1 | 21.3 | ||
W0=0.4,W1=0.6 | 75.1 | 95.7 | 86.4 | 96.4 | 21.4 | ||
W0=0.3,W1=0.7 | 74.9 | 95.3 | 87.3 | 95.9 | 21.4 |
SPM Cluster NMS | f6 (Ratio=1) |
f4 (W0=0.6,W1=0.4) |
mAP @0.5:0.95/% |
mAP @0.5/% |
mAP @0.75/% |
74.3 | 95.3 | 87.3 | |||
✔ | 75.4 | 95.4 | 87.4 | ||
✔ | 75.6 | 96.4 | 87.0 | ||
✔ | 75.5 | 96.4 | 87.7 | ||
✔ | ✔ | 76.0 | 96.3 | 87.1 | |
✔ | ✔ | 76.1 | 96.2 | 87.9 |
Models | mAP @0.5:0.95/% |
mAP@0.5/% | mAP@0.75/% | mAR@0.5/% | FPS | GFLOPs |
RetinaNet | 64.3 | 86.8 | 74.1 | 92.4 | 12.6 | 93.6 |
Faster-RCNN | 66.1 | 88.6 | 76.7 | 90.4 | 13.4 | 38.5 |
Mask-RCNN | 65.2 | 87.1 | 77.4 | 88.7 | 13.4 | 9.2 |
Cascade-RCNN | 69.0 | 87.9 | 78.6 | 90.2 | 8.5 | 9.2 |
FCOS | 53.2 | 81.6 | 60.7 | 90.6 | 13.3 | 80.1 |
YOLOv5n | 64.8 | 90.8 | 74.1 | 85.2 | 52.9 | 4.2 |
YOLOv5m | 73.5 | 94.5 | 85.3 | 91.6 | 31.1 | 48.2 |
YOLOv5l | 74.4 | 95.5 | 86.9 | 93.3 | 25.8 | 108.3 |
YOLOv7t | 66.2 | 90.8 | 74.9 | 86.1 | 44.4 | 12.9 |
YOLOv7 | 73.8 | 93.9 | 86.5 | 89.6 | 22.8 | 104.5 |
YOLOv8s | 72.3 | 91.8 | 81.3 | 88.4 | 41.3 | 28.3 |
YOLOv8m | 73.1 | 93.0 | 82.3 | 90.1 | 37.9 | 78.1 |
YOLOv8l | 73.2 | 92.7 | 81.7 | 88.1 | 29.2 | 163.9 |
YOLOv9c | 67.0 | 89.4 | 76.4 | 84.4 | 17.4 | 102.1 |
Ours-1 | 76.0 | 96.3 | 87.1 | 96.7 | 16.3 | 9.9 |
Ours-2 | 76.1 | 96.2 | 87.9 | 97.0 | 17.4 | 9.7 |
Method | mAP @0.5:0.95/% |
mAP@0.5/% | mAP@0.75/% | mAR@0.5/% | FPS |
Original model | 74.3 | 95.3 | 87.3 | 95.8 | 22.2 |
Soft NMS | 75.3 | 95.4 | 87.6 | 96.1 | 0.5 |
DIoU NMS | 74.5 | 95.4 | 87.9 | 96.1 | 0.8 |
Fast NMS | 67.9 | 88.2 | 79.7 | 89.3 | 20.6 |
Cluster NMS | 74.7 | 95.4 | 87.1 | 96.2 | 17.2 |
SPM Cluster NMS | 75.4 | 95.4 | 87.4 | 96.2 | 17.1 |
method | hyperparameter | mAP @0.5:0.95/% |
mAP@0.5/% | mAP@0.75/% | mAR@0.5/% | FPS | #Params |
Original model | - | 74.3 | 95.3 | 87.3 | 95.8 | 22.2 | 41.0M |
f1 | - | 75.2 | 95.7 | 87.3 | 95.3 | 22.6 | 41.0M |
f2 | - | 74.0 | 95.3 | 86.9 | 96.0 | 15.7 | 41.1M |
f3 | Ratio=0.5 | 75.3 | 96.0 | 87.7 | 95.3 | 21.4 | 41.6M |
Ratio=1 | 75.6 | 96.4 | 87.0 | 97.0 | 21.7 | 41.3M | |
Ratio=4 | 75.0 | 95.6 | 86.9 | 96.4 | 21.9 | 41.1M |
method | hyperparameter | mAP @0.5:0.95/% |
mAP @0.5/% |
mAP @0.75/% |
mAR @0.5/% |
FPS | #Params |
Original model | - | 74.3 | 95.3 | 87.3 | 95.8 | 22.2 | 41.0M |
f3 | Ratio=1 | 75.6 | 96.4 | 87.0 | 97.0 | 21.7 | 41.3M |
f4 | W0=0.7,W1=0.3 | 75.2 | 95.9 | 86.7 | 96.7 | 22.8 | 41.0M |
W0=0.6,W1=0.4 | 75.5 | 96.4 | 87.7 | 97.2 | 22.6 | ||
W0=0.4,W1=0.6 | 75.1 | 95.3 | 87.8 | 96.0 | 22.7 | ||
W0=0.3,W1=0.7 | 74.8 | 95.8 | 86.3 | 96.7 | 22.6 | ||
f5 | W0=0.7,W1=0.3 | 74.3 | 95.3 | 86.6 | 96.1 | 15.8 | 41.1M |
W0=0.6,W1=0.4 | 75.0 | 95.3 | 86.8 | 96.1 | 15.7 | ||
W0=0.4,W1=0.6 | 74.5 | 95.7 | 88.1 | 96.5 | 15.6 | ||
W0=0.3,W1=0.7 | 74.0 | 95.1 | 86.8 | 95.8 | 15.7 | ||
f6 Ratio=1 |
W0=0.7,W1=0.3 | 74.9 | 95.4 | 87.7 | 96.5 | 21.5 | 41.3M |
W0=0.6,W1=0.4 | 74.8 | 95.6 | 86.9 | 96.1 | 21.3 | ||
W0=0.4,W1=0.6 | 75.1 | 95.7 | 86.4 | 96.4 | 21.4 | ||
W0=0.3,W1=0.7 | 74.9 | 95.3 | 87.3 | 95.9 | 21.4 |
SPM Cluster NMS | f6 (Ratio=1) |
f4 (W0=0.6,W1=0.4) |
mAP @0.5:0.95/% |
mAP @0.5/% |
mAP @0.75/% |
74.3 | 95.3 | 87.3 | |||
✔ | 75.4 | 95.4 | 87.4 | ||
✔ | 75.6 | 96.4 | 87.0 | ||
✔ | 75.5 | 96.4 | 87.7 | ||
✔ | ✔ | 76.0 | 96.3 | 87.1 | |
✔ | ✔ | 76.1 | 96.2 | 87.9 |
Models | mAP @0.5:0.95/% |
mAP@0.5/% | mAP@0.75/% | mAR@0.5/% | FPS | GFLOPs |
RetinaNet | 64.3 | 86.8 | 74.1 | 92.4 | 12.6 | 93.6 |
Faster-RCNN | 66.1 | 88.6 | 76.7 | 90.4 | 13.4 | 38.5 |
Mask-RCNN | 65.2 | 87.1 | 77.4 | 88.7 | 13.4 | 9.2 |
Cascade-RCNN | 69.0 | 87.9 | 78.6 | 90.2 | 8.5 | 9.2 |
FCOS | 53.2 | 81.6 | 60.7 | 90.6 | 13.3 | 80.1 |
YOLOv5n | 64.8 | 90.8 | 74.1 | 85.2 | 52.9 | 4.2 |
YOLOv5m | 73.5 | 94.5 | 85.3 | 91.6 | 31.1 | 48.2 |
YOLOv5l | 74.4 | 95.5 | 86.9 | 93.3 | 25.8 | 108.3 |
YOLOv7t | 66.2 | 90.8 | 74.9 | 86.1 | 44.4 | 12.9 |
YOLOv7 | 73.8 | 93.9 | 86.5 | 89.6 | 22.8 | 104.5 |
YOLOv8s | 72.3 | 91.8 | 81.3 | 88.4 | 41.3 | 28.3 |
YOLOv8m | 73.1 | 93.0 | 82.3 | 90.1 | 37.9 | 78.1 |
YOLOv8l | 73.2 | 92.7 | 81.7 | 88.1 | 29.2 | 163.9 |
YOLOv9c | 67.0 | 89.4 | 76.4 | 84.4 | 17.4 | 102.1 |
Ours-1 | 76.0 | 96.3 | 87.1 | 96.7 | 16.3 | 9.9 |
Ours-2 | 76.1 | 96.2 | 87.9 | 97.0 | 17.4 | 9.7 |