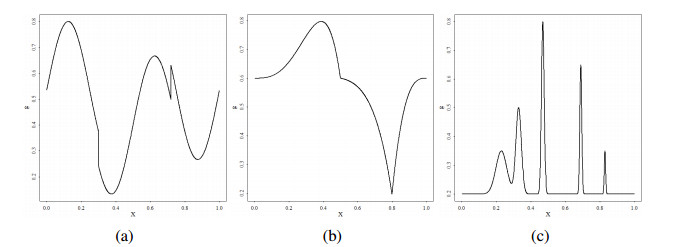
Development of Environmental Product Declarations (EPD)s used for green marketing, specification, procurement, certification and green building rating systems are important for documenting and understanding product environmental performance. Considering such applications any misleading of stakeholders has serious legal ramifications. Various studies have highlighted EPD veracity depends mainly on the data quality of underpinning life cycle assessment (LCA). This paper compares data quality across polyester product case studies, literature surveys and EPDs. Life Cycle Inventory (LCI) and Life Cycle Impact Assessment (LCIA) results are presented and interpreted. Surveys show recycled polyester fibre results are most sensitive to melt spinning energy data which varies over a wide range. The case studies compare results from median, lower and upper energy use in melt spinning. The work highlights that, accurate, clear definitions and vocabulary is as vital for specific foreground process data as it is for generic background supply chain data. This is to avoid misconceptions and mismatched assumptions in respect of EPD data quality and incorrect acceptance of inadequate charting of all essential processes. If product-specific accurate data is inaccessible, EPD options include presenting impact assessment results from LCI of best and worst-case scenarios. This is preferable to legal risks of using junk data that misleads stakeholders in marketing. General recommendations are presented for LCA practitioners to improve EPD data quality and accuracy. These include using multiple data sources to avoid reliance on any single database. Data also needs to be verified by a third-party with industry expertise independent of the specific manufacturer. It recommends using suitable, comprehensive and specific product-related scenarios for data development in any EPD.
Citation: Shadia Moazzem, Delwyn Jones, Mathilde Vlieg, Direshni Naiker. Inaccurate polyester textile environmental product declarations[J]. Clean Technologies and Recycling, 2022, 2(1): 47-63. doi: 10.3934/ctr.2022003
[1] | Yanyan Gao, Yangjiang Wei . Group codes over symmetric groups. AIMS Mathematics, 2023, 8(9): 19842-19856. doi: 10.3934/math.20231011 |
[2] | Chunqiang Cui, Jin Chen, Shixun Lin . Metric and strong metric dimension in TI-power graphs of finite groups. AIMS Mathematics, 2025, 10(1): 705-720. doi: 10.3934/math.2025032 |
[3] | Yunpeng Bai, Yuanlin Li, Jiangtao Peng . Unit groups of finite group algebras of Abelian groups of order 17 to 20. AIMS Mathematics, 2021, 6(7): 7305-7317. doi: 10.3934/math.2021428 |
[4] | Huani Li, Ruiqin Fu, Xuanlong Ma . Forbidden subgraphs in reduced power graphs of finite groups. AIMS Mathematics, 2021, 6(5): 5410-5420. doi: 10.3934/math.2021319 |
[5] | Yingyu Luo, Yu Wang . Supercommuting maps on unital algebras with idempotents. AIMS Mathematics, 2024, 9(9): 24636-24653. doi: 10.3934/math.20241200 |
[6] | Guoqing Wang . A generalization of Kruyswijk-Olson theorem on Davenport constant in commutative semigroups. AIMS Mathematics, 2020, 5(4): 2992-3001. doi: 10.3934/math.2020193 |
[7] | Yongli Zhang, Jiaxin Shen . Flag-transitive non-symmetric 2-designs with λ prime and exceptional groups of Lie type. AIMS Mathematics, 2024, 9(9): 25636-25645. doi: 10.3934/math.20241252 |
[8] | Hatice Gülsün Akay . (Co-)fibration of generalized crossed modules. AIMS Mathematics, 2024, 9(11): 32782-32796. doi: 10.3934/math.20241568 |
[9] | Xiaofei Cao, Yuyue Huang, Xue Hua, Tingyu Zhao, Sanzhang Xu . Matrix inverses along the core parts of three matrix decompositions. AIMS Mathematics, 2023, 8(12): 30194-30208. doi: 10.3934/math.20231543 |
[10] | Hui Yan, Hongxing Wang, Kezheng Zuo, Yang Chen . Further characterizations of the weak group inverse of matrices and the weak group matrix. AIMS Mathematics, 2021, 6(9): 9322-9341. doi: 10.3934/math.2021542 |
Development of Environmental Product Declarations (EPD)s used for green marketing, specification, procurement, certification and green building rating systems are important for documenting and understanding product environmental performance. Considering such applications any misleading of stakeholders has serious legal ramifications. Various studies have highlighted EPD veracity depends mainly on the data quality of underpinning life cycle assessment (LCA). This paper compares data quality across polyester product case studies, literature surveys and EPDs. Life Cycle Inventory (LCI) and Life Cycle Impact Assessment (LCIA) results are presented and interpreted. Surveys show recycled polyester fibre results are most sensitive to melt spinning energy data which varies over a wide range. The case studies compare results from median, lower and upper energy use in melt spinning. The work highlights that, accurate, clear definitions and vocabulary is as vital for specific foreground process data as it is for generic background supply chain data. This is to avoid misconceptions and mismatched assumptions in respect of EPD data quality and incorrect acceptance of inadequate charting of all essential processes. If product-specific accurate data is inaccessible, EPD options include presenting impact assessment results from LCI of best and worst-case scenarios. This is preferable to legal risks of using junk data that misleads stakeholders in marketing. General recommendations are presented for LCA practitioners to improve EPD data quality and accuracy. These include using multiple data sources to avoid reliance on any single database. Data also needs to be verified by a third-party with industry expertise independent of the specific manufacturer. It recommends using suitable, comprehensive and specific product-related scenarios for data development in any EPD.
This paper considers the following heteroscedastic model:
Yi=f(Xi)Ui+g(Xi),i∈{1,⋯,n}. | (1.1) |
In this equation, g(x) is a known mean function, and the variance function r(x)(r(x):=f2(x)) is unknown. Both the mean function g(x) and variance function r(x) are defined on [0,1]. The random variables U1,…,Un are independent and identically distributed (i.i.d.) with E[Ui]=0 and V[Ui]=1. Furthermore, the random variable Xi is independent of Ui for any i∈{1,⋯,n}. The purpose of this paper is to estimate the mth derivative functions r(m)(x)(m∈N) from the observed data (X1,Y1),⋯,(Xn,Yn) by a wavelet method.
Heteroscedastic models are widely used in economics, engineering, biology, physical sciences and so on; see Box [1], Carroll and Ruppert [2], Härdle and Tsybakov [3], Fan and Yao [4], Quevedo and Vining [5] and Amerise [6]. For the above estimation model (1.1), the most popular method is the kernel method. Many important and interesting results of kernel estimators have been obtained by Wang et al. [7], Kulik and Wichelhaus [8] and Shen et al. [9]. However, the optimal bandwidth parameter of the kernel estimator is not easily obtained in some cases, especially when the function has some sharp spikes. Because of the good local properties in both time and frequency domains, the wavelet method has been widely used in nonparametric estimation problems; see Donoho and Johnstone [10], Cai [11], Nason et al. [12], Cai and Zhou [13], Abry and Didier [14] and Li and Zhang [15]. For the estimation problem (1.1), Kulik and Raimondo [16] studied the adaptive properties of warped wavelet nonlinear approximations over a wide range of Besov scales. Zhou et al. [17] developed wavelet estimators for detecting and estimating jumps and cusps in the mean function. Palanisamy and Ravichandran [18] proposed a data-driven estimator by applying wavelet thresholding along with the technique of sparse representation. The asymptotic normality for wavelet estimators of variance function under α−mixing condition was obtained by Ding and Chen [19].
In this paper, we focus on nonparametric estimation of the derivative function r(m)(x) of the variance function r(x). It is well known that derivative estimation plays an important and useful role in many practical applications (Woltring [20], Zhou and Wolfe, [21], Chacón and Duong [22], Wei et al.[23]). For the estimation model (1.1), a linear wavelet estimator and an adaptive nonlinear wavelet estimator for the derivative function r(m)(x) are constructed. Moreover, the convergence rates over L˜p(1≤˜p<∞) risk of two wavelet estimators are proved in Besov space Bsp,q(R) with some mild conditions. Finally, numerical experiments are carried out, where an automatic selection method is used to obtain the best parameters of two wavelet estimators. According to the simulation study, both wavelet estimators can efficiently estimate the derivative function. Furthermore, the nonlinear wavelet estimator shows better performance than the linear estimator.
This paper considers wavelet estimations of a derivative function in Besov space. Now, we first introduce some basic concepts of wavelets. Let ϕ be an orthonormal scaling function, and the corresponding wavelet function is denoted by ψ. It is well known that {ϕτ,k:=2τ/2ϕ(2τx−k),ψj,k:=2j/2ψ(2jx−k),j≥τ,k∈Z} forms an orthonormal basis of L2(R). This paper uses the Daubechies wavelet, which has a compactly support. Then, for any integer j∗, a function h(x)∈L2([0,1]) can be expanded into a wavelet series as
h(x)=∑k∈Λj∗αj∗,kϕj∗,k(x)+∞∑j=j∗∑k∈Λjβj,kψj,k(x),x∈[0,1]. | (1.2) |
In this equation, Λj={0,1,…,2j−1}, αj∗,k=⟨h,ϕj∗,k⟩[0,1] and βj,k=⟨h,ψj,k⟩[0,1].
Lemma 1.1. Let a scaling function ϕ be t-regular (i.e., ϕ∈Ct and |Dαϕ(x)|≤c(1+|x|2)−l for each l∈Z and α=0,1,…,t). If {αk}∈lp and 1≤p≤∞, there exist c2≥c1>0 such that
c12j(12−1p)‖(αk)‖p≤‖∑k∈Λjαk2j2ϕ(2jx−k)‖p≤c22j(12−1p)‖(αk)‖p. |
Besov spaces contain many classical function spaces, such as the well known Sobolev and Hölder spaces. The following lemma gives an important equivalent definition of a Besov space. More details about wavelets and Besov spaces can be found in Meyer [24] and Härdle et al. [25].
Lemma 1.2. Let ϕ be t-regular and h∈Lp([0,1]). Then, for p,q∈[1,∞) and 0<s<t, the following assertions are equivalent:
(i) h∈Bsp,q([0,1]);
(ii) {2js‖h−Pjh‖p}∈lq;
(iii) {2j(s−1p+12)‖βj,k‖p}∈lq.
The Besov norm of h can be defined by
‖h‖Bsp,q=‖(ατ,k)‖p+‖(2j(s−1p+12)‖βj,k‖p)j≥τ‖q, |
where ‖βj,k‖pp=∑k∈Λj|βj,k|p.
In this section, we will construct our wavelet estimators, and give the main theorem of this paper. The main theorem shows the convergence rates of wavelet estimators under some mild assumptions. Now, we first give the technical assumptions of the estimation model (1.1) in the following.
A1: The variance function r:[0,1]→R is bounded.
A2: For any i∈{0,…,m−1}, variance function r satisfies r(i)(0)=r(i)(1)=0.
A3: The mean function g:[0,1]→R is bounded and known.
A4: The random variable X satisfies X∼U([0,1]).
A5: The random variable U has a moment of order 2˜p(˜p≥1).
In the above assumptions, A1 and A3 are conventional conditions for nonparametric estimations. The condition A2 is used to prove the unbiasedness of the following wavelet estimators. In addition, A4 and A5 are technique assumptions, which will be used in Lemmas 4.3 and 4.5.
According to the model (1.1), our linear wavelet estimator is constructed by
ˆrlinn(x):=∑k∈Λj∗ˆαj∗,kϕj∗,k(x). | (2.1) |
In this definition, the scale parameter j∗ will be given in the following main theorem, and
ˆαj,k:=1nn∑i=1Y2i(−1)mϕ(m)j,k(Xi)−∫10g2(x)(−1)mϕ(m)j,k(x)dx. | (2.2) |
More importantly, it should be pointed out that this linear wavelet estimator is an unbiased estimator of the derivative function r(m)(x) by Lemma 4.1 and the properties of wavelets.
On the other hand, a nonlinear wavelet estimator is defined by
ˆrnonn(x):=∑k∈Λj∗ˆαj∗,kϕj∗,k(x)+j1∑j=j∗ˆβj,kI{|ˆβj,k|≥κtn}ψj,k(x). | (2.3) |
In this equation, IA denotes the indicator function over an event A, tn=2mj√lnn/n,
ˆβj,k:=1nn∑i=1(Y2i(−1)mψ(m)j,k(Xi)−wj,k)I{|Y2i(−1)mψ(m)j,k(Xi)−wj,k|≤ρn}, | (2.4) |
ρn=2mj√n/lnn, and wj,k=∫10g2(x)(−1)mψ(m)j,k(x)dx. The positive integer j∗ and j1 will also be given in our main theorem, and the constant κ will be chosen in Lemma 4.5. In addition, we adopt the following symbol: x+:=max{x,0}. A≲B denotes A≤cB for some constant c>0; A≳B means B≲A; A∼B stands for both A≲B and B≲A.
In this position, the convergence rates of two wavelet estimators are given in the following main theorem.
Main theorem For the estimation model (1.1) with the assumptions A1-A5, r(m)(x)∈Bsp,q([0,1])(p,q∈[1,∞), s>0) and 1≤˜p<∞, if {p>˜p≥1,s>0} or {1≤p≤˜p,s>1/p}.
(a) the linear wavelet estimator ˆrlinn(x) with s′=s−(1p−1˜p)+ and 2j∗∼n12s′+2m+1 satisfies
E[‖ˆrlinn(x)−r(m)(x)‖˜p˜p]≲n−˜ps′2s′+2m+1. | (2.5) |
(b) the nonlinear wavelet estimator ˆrnonn(x) with 2j∗∼n12t+2m+1 (t>s) and 2j1∼(nlnn)12m+1 satisfies
E[‖ˆrnonn(x)−r(m)(x)‖˜p˜p]≲(lnn)˜p−1(lnnn)˜pδ, | (2.6) |
where
δ=min{s2s+2m+1,s−1/p+1/˜p2(s−1/p)+2m+1}={s2s+2m+1p>˜p(2m+1)2s+2m+1s−1/p+1/˜p2(s−1/p)+2m+1p≤˜p(2m+1)2s+2m+1. |
Remark 1. Note that n−s˜p2s+1(n−(s−1/p+1/˜p)˜p2(s−1/p)+1) is the optimal convergence rate over L˜p(1≤˜p<+∞) risk for nonparametric wavelet estimations (Donoho et al. [26]). The linear wavelet estimator can obtain the optimal convergence rate when p>˜p≥1 and m=0.
Remark 2. When m=0, this derivative estimation problem reduces to the classical variance function estimation. Then, the convergence rates of the nonlinear wavelet estimator are same as the optimal convergence rates of nonparametric wavelet estimation up to a lnn factor in all cases.
Remark 3. According to main theorem (a) and the definition of the linear wavelet estimator, it is easy to see that the construction of the linear wavelet estimator depends on the smooth parameter s of the unknown derivative function r(m)(x), which means that the linear estimator is not adaptive. Compared with the linear estimator, the nonlinear wavelet estimator only depends on the observed data and the sample size. Hence, the nonlinear estimator is adaptive. More importantly, the nonlinear wavelet estimator has a better convergence rate than the linear estimator in the case of p≤˜p.
In order to illustrate the empirical performance of the proposed estimators, we produce a numerical illustration using an adaptive selection method, which is used to obtain the best parameters of the wavelet estimators. For the problem (1.1), we choose three common functions, HeaviSine, Corner and Spikes, as the mean function g(x); see Figure 1. Those functions are usually used in wavelet literature. On the other hand, we choose the function f(x) by f1(x)=3(4x−2)2e−(4x−2)2, f2(x)=sin(2πsinπx) and f3(x)=−(2x−1)2+1, respectively. In addition, we assume that the random variable U satisfies U∼N[0,1]. The aim of this paper is to estimate the derivative function r(m)(x) of the variance function r(x)(r=f2) by the observed data (X1,Y1),…,(Xn,Yn). In this section, we adopt r1(x)=[f1(x)]2, r2(x)=[f2(x)]2 and r3(x)=[f3(x)]2. For the sake of simplicity, our simulation study focuses on the derivative function r′(x)(m=1) and r(x)(m=0) by the observed data (X1,Y1),…,(Xn,Yn)(n=4096). Furthermore, we use the mean square error (MSE(ˆr(x),r(x))=1nn∑i=1(ˆr(Xi)−r(Xi))2) and the average magnitude of error (AME(ˆr(x),r(x))=1nn∑i=1|ˆr(Xi)−r(Xi)|) to evaluate the performances of the wavelet estimators separately.
For the linear and nonlinear wavelet estimators, the scale parameter j∗ and threshold value λ(λ=κtn) play important roles in the function estimation problem. In order to obtain the optimal scale parameter and threshold value of wavelet estimators, this section uses the two-fold cross validation (2FCV) approach (Nason [27], Navarro and Saumard [28]). During the first example of simulation study, we choose HeaviSine as the mean function g(x), and f1(x)=3(4x−2)2e−(4x−2)2. The estimation results of two wavelet estimators are presented by Figure 2. For the optimal scale parameter j∗ of the linear wavelet estimator, we built a collection of j∗ and j∗=1,…,log2(n)−1. The best parameter j∗ is selected by minimizing a 2FCV criterion denoted by 2FCV(j∗); see Figure 2(a). According to Figure 2(a), it is easy to see that the 2FCV(j∗) and MSE both can get the minimum value when j∗=4. For the nonlinear wavelet estimator, the best threshold value λ is also obtained by the 2FCV(λ) criterion in Figure 2(b). Meanwhile, the parameter j∗ is same as the linear estimator, and the parameter j1 is chosen as the maximum scale parameter log2(n)−1. From Figure 2(c) and 2(d), the linear and nonlinear wavelet estimators both can get a good performance with the best scale parameter and threshold value. More importantly, the nonlinear wavelet estimator shows better performance than the linear estimator.
In the following simulation study, more numerical experiments are presented to sufficiently verify the performance of the wavelet method. According to Figures 3–10, the wavelet estimators both can obtain good performances in different cases. Especially, the nonlinear wavelet estimator gets better estimation results than the linear estimator. Also, the MSE and AME of the wavelet estimators in all examples are provided by Table 1. Meanwhile, it is easy to see from Table 1 that the nonlinear wavelet estimators can have better performance than the linear estimators.
HeaviSine | Corner | Spikes | |||||||
r1 | r2 | r3 | r1 | r2 | r3 | r1 | r2 | r3 | |
MSE(ˆrlin,r) | 0.0184 | 0.0073 | 0.0071 | 0.0189 | 0.0075 | 0.0064 | 0.0189 | 0.0069 | 0.0052 |
MSE(ˆrnon,r) | 0.0048 | 0.0068 | 0.0064 | 0.0044 | 0.0070 | 0.0057 | 0.0042 | 0.0061 | 0.0046 |
MSE(ˆr′lin,r′) | 0.7755 | 0.0547 | 0.0676 | 0.7767 | 0.1155 | 0.0737 | 0.7360 | 0.2566 | 0.0655 |
MSE(ˆr′non,r′) | 0.2319 | 0.0573 | 0.0560 | 0.2204 | 0.0644 | 0.0616 | 0.2406 | 0.2868 | 0.0539 |
AME(ˆrlin,r) | 0.0935 | 0.0653 | 0.0652 | 0.0973 | 0.0667 | 0.0615 | 0.0964 | 0.0621 | 0.0550 |
AME(ˆrnon,r) | 0.0506 | 0.0641 | 0.0619 | 0.0486 | 0.0649 | 0.0583 | 0.0430 | 0.0595 | 0.0518 |
AME(ˆr′lin,r′) | 0.6911 | 0.1876 | 0.2348 | 0.7021 | 0.2686 | 0.2451 | 0.6605 | 0.4102 | 0.2320 |
AME(ˆr′non,r′) | 0.3595 | 0.1862 | 0.2125 | 0.3450 | 0.2020 | 0.2229 | 0.3696 | 0.4198 | 0.2095 |
Now, we provide some lemmas for the proof of the main Theorem.
Lemma 4.1. For the model (1.1) with A2 and A4,
E[ˆαj,k]=αj,k, | (4.1) |
E[1nn∑i=1(Y2i(−1)mψ(m)j,k(Xi)−wj,k)]=βj,k. | (4.2) |
Proof. According to the definition of ˆαj,k,
E[ˆαj,k]=E[1nn∑i=1Y2i(−1)mϕ(m)j,k(Xi)−∫10g2(x)(−1)mϕ(m)j,k(x)dx]=1nn∑i=1E[Y2i(−1)mϕ(m)j,k(Xi)]−∫10g2(x)(−1)mϕ(m)j,k(x)dx=E[Y21(−1)mϕ(m)j,k(X1)]−∫10g2(x)(−1)mϕ(m)j,k(x)dx=E[r(X1)U21(−1)mϕ(m)j,k(X1)]+2E[f(X1)U1g(X1)(−1)mϕ(m)j,k(X1)]+E[g2(X1)(−1)mϕ(m)j,k(X1)]−∫10g2(x)(−1)mϕ(m)j,k(x)dx. |
Then, it follows from A4 that
E[g2(X1)(−1)mϕ(m)j,k(X1)]=∫10g2(x)(−1)mϕ(m)j,k(x)dx. |
Using the assumption of independence between Ui and Xi,
E[r(X1)U21(−1)mϕ(m)j,k(X1)]=E[U21]E[r(X1)(−1)mϕ(m)j,k(X1)], |
E[f(X1)U1g(X1)(−1)mϕ(m)j,k(X1)]=E[U1]E[f(X1)g(X1)(−1)mϕ(m)j,k(X1)]. |
Meanwhile, the conditions V[U1]=1 and E[U1]=0 imply E[U21]=1. Hence, one gets
E[ˆαj,k]=E[r(X1)(−1)mϕ(m)j,k(X1)]=∫10r(x)(−1)mϕ(m)j,k(x)dx=(−1)m∫10r(x)ϕ(m)j,k(x)dx=∫10r(m)(x)ϕj,k(x)dx=αj,k |
by the assumption A2.
On the other hand, one takes ψ instead of ϕ, and wj,k instead of ∫10g2(x)(−1)mϕ(m)j,k(x)dx. The second equation will be proved by the similar mathematical arguments.
Lemma 4.2. (Rosenthal's inequality) Let X1,…,Xn be independent random variables such that E[Xi]=0 and E[|Xi|p]<∞. Then,
E[|n∑i=1Xi|p]≲{n∑i=1E[|Xi|p]+(n∑i=1E[|Xi|2])p2, p > 2 ,(n∑i=1E[|Xi|2])p2,1≤p≤2. |
Lemma 4.3. For the model (1.1) with A1–A5, 2j≤n and 1≤˜p<∞,
E[|ˆαj,k−αj,k|˜p]≲n−˜p22˜pmj, | (4.3) |
E[|ˆβj,k−βj,k|˜p]≲(lnnn)−˜p22˜pmj. | (4.4) |
Proof. By (4.1) and the independence of random variables Xi and Ui, one has
|ˆαj,k−αj,k|=|1nn∑i=1Y2i(−1)mϕ(m)j,k(Xi)−∫10g2(x)(−1)mϕ(m)j,k(x)dx−E[ˆαj,k]|=1n|n∑i=1(Y2i(−1)mϕ(m)j,k(Xi)−E[Y2i(−1)mϕ(m)j,k(Xi)])|=1n|n∑i=1Ai|. |
In this above equation, Ai:=Y2i(−1)mϕ(m)j,k(Xi)−E[Y2i(−1)mϕ(m)j,k(Xi)].
According to the definition of Ai, one knows that E[Ai]=0 and
E[|Ai|˜p]=E[|Y2i(−1)mϕ(m)j,k(Xi)−E[Y2i(−1)mϕ(m)j,k(Xi)]|˜p]≲E[|Y2i(−1)mϕ(m)j,k(Xi)|˜p]≲E[|(r(X1)U21+g2(X1))(−1)mϕ(m)j,k(Xi)|˜p]≲E[U2˜p1]E[|r(X1)ϕ(m)j,k(Xi)|˜p]+E[|g2(X1)ϕ(m)j,k(Xi)|˜p]. |
The assumption A5 shows E[U2˜p1]≲1. Furthermore, it follows from A1 and A3 that
E[U2˜p1]E[|r(X1)ϕ(m)j,k(X1)|˜p]≲E[|ϕ(m)j,k(X1)|˜p],E[g2˜p(X1)|ϕ(m)j,k(X1)|˜p]≲E[|ϕ(m)j,k(X1)|˜p]. |
In addition, and the properties of wavelet functions imply that
E[|ϕ(m)j,k(Xi)|˜p]=∫10|ϕ(m)j,k(x)|˜pdx=2j(˜p/2+m˜p−1)∫10|ϕ(m)(2jx−k)|˜pd(2jx−k)=2j(˜p/2+m˜p−1)||ϕ(m)||˜p˜p≲2j(˜p/2+m˜p−1). |
Hence,
E[|Ai|˜p]≲2j(˜p/2+m˜p−1). |
Especially in ˜p=2, E[|Ai|2]≲22mj.
Using Rosenthal's inequality and 2j≤n,
E[|ˆαj,k−αj,k|˜p]=1n˜pE[|n∑i=1Ai|˜p]≲{1n˜p(n∑i=1E[|Ai|˜p]+(n∑i=1E[|Ai|2])˜p2),˜p>2,1n˜p(n∑i=1E[|Ai|2])˜p2,1≤˜p≤2,≲{1n˜p(n⋅2j(˜p2+m˜p−1)+(n⋅22mj)˜p2),˜p>2,1n˜p(n⋅22mj)˜p2,1≤˜p≤2,≲n−˜p22˜pmj. |
Then, the first inequality is proved.
For the second inequality, note that
βj,k=E[1nn∑i=1(Y2i(−1)mψ(m)j,k(Xi)−wj,k)]=1nn∑i=1E[(Y2i(−1)mψ(m)j,k(Xi)−∫10g2(x)(−1)mψ(m)j,k(x)dx)]=1nn∑i=1E[Ki] |
with (4.2) and Ki:=Y2i(−1)mψ(m)j,k(Xi)−∫10g2(x)(−1)mψ(m)j,k(x)dx.
Let Bi:=KiI{|Ki|≤ρn}−E[KiI{|Ki|≤ρn}]. Then, by the definition of ˆβj,k in (2.4),
|ˆβj,k−βj,k|=|1nn∑i=1KiI{|Ki|≤ρn}−βj,k|≤1n|n∑i=1Bi|+1nn∑i=1E[|Ki|I{|Ki|>ρn}]. | (4.5) |
Similar to the arguments of Ai, it is easy to see that E[Bi]=0 and
E[|Bi|˜p]≲E[|KiI{|Ki|≤ρn}|˜p]≲E[|Ki|˜p]≲2j(˜p2+m˜p−1). |
Especially in the case of ˜p=2, one can obtain E[|Bi|2]≲22mj. On the other hand,
E[|Ki|I{|Ki|>ρn}]≲E[|Ki|⋅|Ki|ρn]=E[K21]ρn≲22mjρn=tn=2mj√lnnn. | (4.6) |
According to Rosenthal's inequality and 2j≤n,
E[|ˆβj,k−βj,k|˜p]≲1n˜pE[|n∑i=1Bi|˜p]+(tn)˜p≲{1n˜p(n∑i=1E[|Bi|˜p]+(n∑i=1E[|Bi|2])˜p2)+(tn)˜p,˜p>2,1n˜p(n∑i=1E[|Bi|2])˜p2+(tn)˜p,1≤˜p≤2,≲{1n˜p(n⋅2j(˜p2+m˜p−1)+(n⋅22mj)˜p2)+(lnnn)−˜p2⋅2˜pmj,˜p>2,1n˜p(n⋅22mj)˜p2+(lnnn)−˜p2⋅2˜pmj,1≤˜p≤2,≲(lnnn)−˜p22˜pmj. |
Then, the second inequality is proved.
Lemma 4.4. (Bernstein's inequality) Let X1,…,Xn be independent random variables such that E[Xi]=0, |Xi|<M and E[|Xi|2]:=σ2. Then, for each ν>0
P(1n|n∑i=1Xi|≥ν)≤2exp{−nν22(σ2+νM/3)}. |
Lemma 4.5. For the model (1.1) with A1–A5 and 1≤˜p<+∞, there exists a constant κ>1 such that
P(|ˆβj,k−βj,k|≥κtn)≲n−˜p. | (4.7) |
Proof. According to (4.5), one gets Ki=Y2i(−1)mψ(m)j,k(Xi)−∫10g2(x)(−1)mψ(m)j,k(x)dx, Bi=KiI{|Ki|≤ρn}−E[KiI{|Ki|≤ρn}] and
|ˆβj,k−βj,k|≤1n|n∑i=1Bi|+1nn∑i=1E[|Ki|I{|Ki|>ρn}]. |
Meanwhile, (4.6) shows that there exists c>0 such that E[|Ki|I{|Ki|>ρn}]≤ctn. Furthermore, the following conclusion is true.
{|ˆβj,k−βj,k,u|≥κtn}⊆{[1n|n∑i=1Bi|+1nn∑i=1E(|Ki|I{|Ki|>ρn})]≥κtn}⊆{1n|n∑i=1Bi|≥(κ−c)tn}. |
Note that the definition of Bi implies that |Bi|≲ρn and E[Bi]=0. Using the arguments of Lemma 4.3, E[B2i]:=σ2≲22mj. Furthermore, by Bernstein's inequality,
P(1n|n∑i=1Bi|≥(κ−c)tn)≲exp{−n(κ−c)2tn22(σ2+(κ−c)tnρn/3)}≲exp{−n(κ−c)222mj⋅lnnn2(22mj+(κ−c)⋅22mj/3)}=exp{−(lnn)(κ−c)22(1+(κ−c)/3)}=n−(κ−c)22(1+(κ−c)/3). |
Then, one can choose large enough κ such that
P(|ˆβj,k−βj,k|≥κtn)≲n−(κ−c)22(1+(κ−c)/3)≲n−˜p. |
Proof of (a): Note that
‖ˆrlinn(x)−r(m)(x)‖˜p˜p≲‖ˆrlinn(x)−Pj∗r(m)(x)‖˜p˜p+‖Pj∗r(m)(x)−r(m)(x)‖˜p˜p |
Hence,
E[‖ˆrlinn(x)−r(m)(x)‖˜p˜p]≲E[‖ˆrlinn(x)−Pj∗r(m)(x)‖˜p˜p]+‖Pj∗r(m)(x)−r(m)(x)‖˜p˜p. | (4.8) |
◼ The stochastic term E[‖ˆrlinn(x)−Pj∗r(m)(x)‖˜p˜p].
It follows from Lemma 1.1 that
E[‖ˆrlinn(x)−Pj∗r(m)(x)‖˜p˜p]=E[‖∑k∈Λj∗(ˆαj∗,k−αj∗,k)ϕj∗,k(x)‖˜p˜p]∼2j∗(12−1˜p)˜p∑k∈Λj∗E[|ˆαj∗,k−αj∗,k|˜p]. |
Then, according to (4.3), |Λj∗|∼2j∗ and 2j∗∼n12s′+2m+1, one gets
E[‖ˆrlinn(x)−Pj∗r(m)(x)‖˜p˜p]∼2j∗˜p2(2m+1)⋅n−˜p2∼n−˜ps′2s′+2m+1. | (4.9) |
◼ The bias term ‖Pj∗r(m)(x)−r(m)(x)‖˜p˜p.
When p>˜p≥1, s′=s−(1p−1˜p)+=s. Using Hölder inequality, Lemma 1.2 and r(m)∈Bsp,q([0,1]),
‖Pj∗r(m)(x)−r(m)(x)‖˜p˜p≲‖Pj∗r(m)(x)−r(m)(x)‖˜pp≲2−j∗˜ps=2−j∗˜ps′∼n−˜ps′2s′+2m+1. |
When 1≤p≤˜p and s>1p, one knows that Bsp,q([0,1])⊆Bs′˜p,∞([0,1]) and
‖Pj∗r(m)(x)−r(m)(x)‖˜p˜p≲2−j∗˜ps′∼n−˜ps′2s′+2m+1. |
Hence, the following inequality holds in both cases.
‖Pj∗r(m)(x)−r(m)(x)‖˜p˜p≲n−˜ps′2s′+2m+1. | (4.10) |
Finally, the results (4.8)–(4.10) show
E[‖ˆrlinn(x)−r(m)(x)‖˜p˜p]≲n−˜ps′2s′+2m+1. |
Proof of (b): By the definitions of ˆrlinn(x) and ˆrnonn(x), one has
‖ˆrnonn(x)−r(m)(x)‖˜p˜p≲‖ˆrlinn(x)−Pj∗r(m)(x)‖˜p˜p+‖r(m)(x)−Pj1+1r(m)(x)‖˜p˜p+‖j1∑j=j∗∑k∈Λj(ˆβj,kI{|ˆβj,k|≥κtn}−βj,k)ψj,k(x)‖˜p˜p. |
Furthermore,
E[‖ˆrnonn(x)−r(m)(x)‖˜p˜p]≲T1+T2+Q. | (4.11) |
In this above inequality,
T1:=E[‖ˆrlinn(x)−Pj∗r(m)(x)‖˜p˜p],T2:=‖r(m)(x)−Pj1+1r(m)(x)‖˜p˜p,Q:=E[‖j1∑j=j∗∑k∈Λj(ˆβj,kI{|ˆβj,k|≥κtn}−βj,k)ψj,k(x)‖˜p˜p]. |
◼ For T1. According to (4.9) and 2j∗∼n12t+2m+1 (t>s),
T1∼2j∗˜p2(2m+1)⋅n−˜p2∼n−˜pt2t+2m+1<n−˜ps2s+2m+1≤n−˜pδ. | (4.12) |
◼ For T2. Using similar mathematical arguments as (4.10), when p>˜p≥1, one can obtain T2:=‖r(m)(x)−Pj1+1r(m)(x)‖˜p˜p≲2−j1˜ps. This with 2j1∼(nlnn)12m+1 leads to
T2≲2−j1˜ps<(lnnn)˜ps2m+1≤(lnnn)˜ps2s+2m+1≤(lnnn)˜pδ. |
On the other hand, when 1≤p≤˜p and s>1p, one has Bsp,q([0,1])⊆Bs−1p+1˜p˜p,∞([0,1]) and
T2≲2−j1˜p(s−1/p+1/˜p)∼(lnnn)˜p(s−1/p+1/˜p)2m+1<(lnnn)˜p(s−1/p+1/˜p)2(s−1/p)+2m+1≤(lnnn)˜pδ. |
Therefore, for each 1≤˜p<∞,
T2≲(lnnn)˜pδ. | (4.13) |
◼ For Q. According to Hölder inequality and Lemma 1.1,
Q≲(j1−j∗+1)˜p−1j1∑j=j∗E[‖∑k∈Λj(ˆβj,kI{|ˆβj,k|≥κtn}−βj,k)ψj,k(x)‖˜p˜p]≲(j1−j∗+1)˜p−1j1∑j=j∗2j(12−1˜p)˜p∑k∈ΛjE[|ˆβj,kI{|ˆβj,k|≥κtn}−βj,k|˜p]. |
Note that
|ˆβj,kI{|ˆβj,k|≥κtn}−βj,k|˜p=|ˆβj,k−βj,k|˜pI{|ˆβj,k|≥κtn,|βj,k|<κtn2}+|ˆβj,k−βj,k|˜pI{|ˆβj,k|≥κtn,|βj,k|≥κtn2}+|βj,k|˜pI{|ˆβj,k|<κtn,|βj,k|>2κtn}+|βj,k|˜pI{|ˆβj,k|<κtn,|βj,k|≤2κtn}. |
Meanwhile,
{|ˆβj,k|≥κtn,|βj,k|<κtn2}⊆{|ˆβj,k−βj,k|>κtn2},{|ˆβj,k|<κtn,|βj,k|>2κtn}⊆{|ˆβj,k−βj,k|>κtn}⊆{|ˆβj,k−βj,k|>κtn2}. |
Then, Q can be decomposed as
Q≲(j1−j∗+1)˜p−1(Q1+Q2+Q3), | (4.14) |
where
Q1:=j1∑j=j∗2j(12−1˜p)˜p∑k∈ΛjE[|ˆβj,k−βj,k|˜pI{|ˆβj,k−βj,k|>κtn2}],Q2:=j1∑j=j∗2j(12−1˜p)˜p∑k∈ΛjE[|ˆβj,k−βj,k|˜pI{|βj,k|≥κtn2}],Q3:=j1∑j=j∗2j(12−1˜p)˜p∑k∈Λj|βj,k|˜pI{|βj,k|≤2κtn}. |
◼ For Q1. It follows from the Hölder inequality that
E[|ˆβj,k−βj,k|˜pI{|ˆβj,k−βj,k|>κtn2}]≤(E[|ˆβj,k−βj,k|2˜p])12[P(|ˆβj,k−βj,k|>κtn2)]12. |
By Lemma 4.3, one gets
E[|ˆβj,k−βj,k|2˜p]≲(lnnn)−˜p⋅22˜pmj. |
This with Lemma 4.5, |Λj|∼2j and 2j1∼(nlnn)12m+1 shows that
Q1≲j1∑j=j∗2j(12−1˜p)˜p2j⋅(lnnn)˜p22˜pmj⋅n−˜p2≲n−˜p2<n−˜pδ. | (4.15) |
◼ For Q2. One defines
2j′∼(nlnn)12s+2m+1. |
Clearly, 2j∗∼n12t+2m+1(t>s)≤2j′∼(nlnn)12s+2m+1<2j1∼(nlnn)12m+1. Furthermore, one rewrites
Q2=(j′∑j=j∗+j1∑j=j′+1)2j(12−1˜p)˜p∑k∈ΛjE[|ˆβj,k−βj,k|˜pI{|βj,k|≥κtn2}]:=Q21+Q22. | (4.16) |
◼ For Q21. By Lemma 4.3 and 2j′∼(nlnn)12s+2m+1,
Q21:=j′∑j=j∗2j(12−1˜p)˜p∑k∈ΛjE[|ˆβj,k−βj,k|˜pI{|βj,k|≥κtn2}]≤j′∑j=j∗2j(12−1˜p)˜p∑k∈ΛjE[|ˆβj,k−βj,k|˜p]≲(lnnn)˜p2j′∑j=j∗2j(2m+1)˜p2≲(lnnn)˜p22j′(2m+1)˜p2∼(lnnn)˜ps2s+2m+1≤(lnnn)˜pδ. | (4.17) |
For . Using Lemma 4.3, one has
When , by the Hölder inequality, , and Lemma 1.2, one can obtain that
(4.18) |
When , it follows from Lemma 1.2 that
(4.19) |
Take
Then, (4.19) can be rewritten as
(4.20) |
When holds if and only if , and
(4.21) |
When holds if and only if , . Define
and obviously, . Furthermore, one rewrites
(4.22) |
For . Note that in the case of . Then, by the same arguments of (4.20), one gets
(4.23) |
For . The conditions and imply . Similar to (4.18), one obtains
(4.24) |
Combining (4.18), (4.21), (4.23) and (4.24),
This with (4.16) and (4.17) shows that
(4.25) |
For . According to the definition of , one can write
For . It is easy to see that
For . One rewrites . When , using the Hölder inequality and Lemma 1.2,
When , one has
For the case of , one can easily obtain that and
When , . Moreover, by the definition of , one rewrites
Note that
On the other hand, similar to the arguments of (4.24), one has
Therefore, in all of the above cases,
(4.26) |
Finally, combining the above results (4.14), (4.15), (4.25) and (4.26), one gets
This with (4.11)–(4.13) shows
This paper considers wavelet estimations of the derivatives of the variance function in a heteroscedastic model. The upper bounds over risk of the wavelet estimators are discussed under some mild assumptions. The results show that the linear wavelet estimator can obtain the optimal convergence rate in the case of . When , the nonlinear wavelet estimator has a better convergence rate than the linear estimator. Moreover, the nonlinear wavelet estimator is adaptive. Finally, some numerical experiments are presented to verify the good performances of the wavelet estimators.
We would like to thank the reviewers for their valuable comments and suggestions, which helped us to improve the quality of the manuscript. This paper is supported by the Guangxi Natural Science Foundation (No. 2022JJA110008), National Natural Science Foundation of China (No. 12001133), Center for Applied Mathematics of Guangxi (GUET), and Guangxi Colleges and Universities Key Laboratory of Data Analysis and Computation.
All authors declare that they have no conflicts of interest.
[1] | ISO 14020: 2000, Environmental Labels & Declarations—General Principles. ISO, 2000. Available from: https://www.iso.org/standard/34425.html. |
[2] | ISO 14025: 2006, Environmental Labels & Declarations—Type III Environmental Declarations—Principles & Procedures. BSI, 2016. Available from: https://www.iso.org/standard/38131.html. |
[3] | ISO 14040: 2006, LCA: Principles & Framework. ISO, 2006. Available from: https://www.iso.org/standard/37456.html. |
[4] | ISO 14044: 2006, EMS: LCA: Requirements & Guidelines. ISO, 2006. Available from: https://www.iso.org/standard/38498.html. |
[5] | EN 15804: 2012+A2: 2019/AC: 2021, Sustainability of Construction Works—EPDs—Core Rules for Construction Products. European Committee for Standardisation, 2021. Available from: https://standards.iteh.ai/catalog/standards/cen/c98127b4-8dc2-48a4-9338-3e1366b16669/en-15804-2012a2-2019. |
[6] |
Del Rosario P, Palumbo E, Traverso M (2021) Environmental product declarations as data source for the environmental assessment of buildings in the context of level (s) and DGNB: How feasible is their adoption? Sustainability 13: 6143. https://doi.org/10.3390/su13116143 doi: 10.3390/su13116143
![]() |
[7] | Jelse K, Peerens K (2018) Using LCA and EPD in public procurement within the construction sector, In: Benetto E, Gericke K, Guiton M, Designing Sustainable Technologies, Products and Policies, 1 Ed., Heidelberg: Springer Nature, 499–502. https://doi.org/10.1007/978-3-319-66981-6_55 |
[8] | United Nations (UN) (2021) UN Sustainable Development Goals (SDG)s, UN Geneva. Available from: https://www.un.org/sustainabledevelopment/. |
[9] | German Sustainable Building Council (DGNB) (2018) DGNB System—Criteria Set New Construction Building. Available from: https://static.dgnb.de/fileadmin/dgnb-system/downloads/criteria/DGNB-System-2018-EN.pdf. |
[10] | The Fibre Year Consulting GmBH (2019) The Fibre Year 2019. World Survey on Textile & Nonwovens. Available from: https://www.textiletechnology.net/technology/trendreports/World-fiber-production-2018-The-Fiber-Year-2019-18677. |
[11] | Biaz O, Rimando PA, Jones DG, et al. (2015) Synthetic Fibre LCA For Ecolabelling. Available from: https://www.researchgate.net/publication/359021941_Synthetic_Fibre_LCA_for_Ecolabelling. |
[12] | Global GreenTagcertTM (2021) GreenTag™ Certification. Available from: https://www.globalgreentag.com/. |
[13] | Global GreenTagcertTM (2021) GreenTag™ EPDs: Autex Industries Ltd/Autex Pty Ltd. Available from: https://www.globalgreentag.com/published-epds-new/. |
[14] |
Modahl IS, Askham C, Lyng KA, et al. (2013) Comparison of two versions of an EPD, using generic and specific data for the foreground system, and some methodological implications. Int J Life Cycle Ass 18: 241–251. https://doi.org/10.1007/s11367-012-0449-0 doi: 10.1007/s11367-012-0449-0
![]() |
[15] | Ferranti P, Berry E, Jock A (2019) Encyclopedia of Food Security and Sustainability, Amsterdam: Elsevier. |
[16] | European Commission, Joint Research Centre, Institute for Environment and Sustainability (2010) International Reference Life Cycle Data System (ILCD) Handbook—General Guide for Life Cycle Assessment—Detailed Guidance, 1 Ed., Luxembourg: Publications Office of the European Union. |
[17] |
Lasvaux S, Habert G, Peuportier B, et al. (2015) Comparison of generic and product-specific Life Cycle Assessment databases: application to construction materials used in building LCA studies. Int J Life Cycle Ass 20: 1473–1490. https://doi.org/10.1007/s11367-015-0938-z doi: 10.1007/s11367-015-0938-z
![]() |
[18] |
Strazza C, Del Borghi A, Magrassi F, et al. (2016) Using environmental product declaration as source of data for life cycle assessment: a case study. J Cleaner Prod 112: 333–342. https://doi.org/10.1016/j.jclepro.2015.07.058 doi: 10.1016/j.jclepro.2015.07.058
![]() |
[19] |
Palumbo E (2021) Effect of LCA data sources on GBRS reference values: The envelope of an Italian passive house. Energies 14: 1883. https://doi.org/10.3390/en14071883 doi: 10.3390/en14071883
![]() |
[20] |
Scrucca F, Baldassarri C, Baldinelli G, et al. (2020) Uncertainty in LCA: An estimation of practitioner-related effects. J Cleaner Prod 268: 122304. https://doi.org/10.1016/j.jclepro.2020.122304 doi: 10.1016/j.jclepro.2020.122304
![]() |
[21] | Roos S (2019) Polyester Fabrics EPD, Smartex Solution Co., Ltd. EPD International. Available from: https://portal.environdec.com/api/api/v1/EPDLibrary/Files/123f5ad6-8cb9-4a8a-afad-751c6a9d6647/Data. |
[22] |
Hasanbeigi A, Price L (2012) A review of energy use and energy efficiency technologies for the textile industry. Renewable Sustainable Energy Rev 16: 3648–3665. https://doi.org/10.1016/j.rser.2012.03.029 doi: 10.1016/j.rser.2012.03.029
![]() |
[23] |
van der Velden NM, Patel MK, Vogtlä nder JG (2014) LCA benchmarking study on textiles made of cotton, polyester, nylon, acryl, or elastane. Int J Life Cycle Ass 19: 331–356. https://doi.org/10.1007/s11367-013-0626-9 doi: 10.1007/s11367-013-0626-9
![]() |
[24] | Sandin G, Roos S, Johansson M (2019) Environmental impact of textile fibers—what we know and what we don't know: fiber bible part 2. Available from: https://www.researchgate.net/publication/331980907_Environmental_impact_of_textile_fibres_-_what_we_know_and_what_we_don't_know_Fiber_Bible_part_2. |
[25] |
Shen L, Worrell E, Patel MK (2010) Open-loop recycling: A LCA case study of PET bottle-to-fibre recycling. Resour Conserv Recy 55: 34–52. https://doi.org/10.1016/j.resconrec.2010.06.014 doi: 10.1016/j.resconrec.2010.06.014
![]() |
[26] | Laursen SE, Hansen J, Bagh J, et al. (1997) Environmental Assessment of Textiles. Life Cycle Screening of Textiles Containing Cotton, Wool, Viscose, Polyester or Acrylic Fibres, København: Danish Environmental Protection Agency, 369. |
[27] |
Hufenus R, Yan Y, Dauner M, et al. (2020) Melt-spun fibers for textile applications. Materials 13: 4298. https://doi.org/10.3390/ma13194298 doi: 10.3390/ma13194298
![]() |
[28] | International Energy Agency (2021) Energy Statistics. Available from: http://www.iea.org/countries. |
[29] | IBISWorld (2021) Market Research. Available from: http://www.ibisworld.com.au/. |
[30] | U.S. Geological Survey (2021) USGS Minerals. Available from: http://minerals.usgs.gov/minerals/pubs/. |
[31] | Franklin Associates (2021) US LCI Database. Available from: http://www.fal.com. |
[32] | Plastics Europe (2021) Eco-Profiles for Determining Environmental Impacts of Plastics. Available from: https://plasticseurope.org/sustainability/circularity/life-cycle-thinking/eco-profiles-set/. |
[33] | NREL USLCI (2021) Life-Cycle Inventory Database. Available from: https://www.lcacommons.gov/nrel. |
[34] | EcoInvent (2021) LCI Databases. Available from: https://ecoinvent.org/the-ecoinvent-database/data-releases/#1598281190996-3f7ad0f3-9003. |
[35] | UNEP/SETAC (2011) Global LCI Database Quality. Available from: https://www.lifecycleinitiative.org/wp-content/uploads/2012/12/2011%20-%20Global%20Guidance%20Principles.pdf. |
1. | Junke Kou, Hao Zhang, Partial Derivatives Estimation of Multivariate Variance Function in Heteroscedastic Model via Wavelet Method, 2024, 13, 2075-1680, 69, 10.3390/axioms13010069 |
0.0184 | 0.0073 | 0.0071 | 0.0189 | 0.0075 | 0.0064 | 0.0189 | 0.0069 | 0.0052 | |
0.0048 | 0.0068 | 0.0064 | 0.0044 | 0.0070 | 0.0057 | 0.0042 | 0.0061 | 0.0046 | |
0.7755 | 0.0547 | 0.0676 | 0.7767 | 0.1155 | 0.0737 | 0.7360 | 0.2566 | 0.0655 | |
0.2319 | 0.0573 | 0.0560 | 0.2204 | 0.0644 | 0.0616 | 0.2406 | 0.2868 | 0.0539 | |
0.0935 | 0.0653 | 0.0652 | 0.0973 | 0.0667 | 0.0615 | 0.0964 | 0.0621 | 0.0550 | |
0.0506 | 0.0641 | 0.0619 | 0.0486 | 0.0649 | 0.0583 | 0.0430 | 0.0595 | 0.0518 | |
0.6911 | 0.1876 | 0.2348 | 0.7021 | 0.2686 | 0.2451 | 0.6605 | 0.4102 | 0.2320 | |
0.3595 | 0.1862 | 0.2125 | 0.3450 | 0.2020 | 0.2229 | 0.3696 | 0.4198 | 0.2095 |
0.0184 | 0.0073 | 0.0071 | 0.0189 | 0.0075 | 0.0064 | 0.0189 | 0.0069 | 0.0052 | |
0.0048 | 0.0068 | 0.0064 | 0.0044 | 0.0070 | 0.0057 | 0.0042 | 0.0061 | 0.0046 | |
0.7755 | 0.0547 | 0.0676 | 0.7767 | 0.1155 | 0.0737 | 0.7360 | 0.2566 | 0.0655 | |
0.2319 | 0.0573 | 0.0560 | 0.2204 | 0.0644 | 0.0616 | 0.2406 | 0.2868 | 0.0539 | |
0.0935 | 0.0653 | 0.0652 | 0.0973 | 0.0667 | 0.0615 | 0.0964 | 0.0621 | 0.0550 | |
0.0506 | 0.0641 | 0.0619 | 0.0486 | 0.0649 | 0.0583 | 0.0430 | 0.0595 | 0.0518 | |
0.6911 | 0.1876 | 0.2348 | 0.7021 | 0.2686 | 0.2451 | 0.6605 | 0.4102 | 0.2320 | |
0.3595 | 0.1862 | 0.2125 | 0.3450 | 0.2020 | 0.2229 | 0.3696 | 0.4198 | 0.2095 |