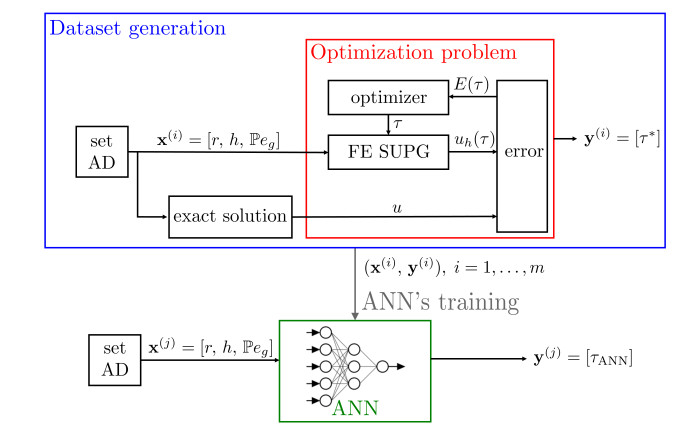
The molecular structure and spectroscopic analysis of the Temozolomide molecule have been performed using the density functional theory in neutral and anion states as well as with the addition of DMSO solvent. The 6-311G(d) basis set was employed to optimize the molecular structure of the TMZ molecule using the DFT/B3LYP method. The HOMO-LUMO energies and MEP map were computed to determine the energy gap and probable sites of electrophilic and nucleophilic reactivity in the 6-311G+(d) basis set. The vibrational frequencies were calculated using a computational method and the major fundamental modes of vibration were assigned to their respective frequencies. The potential of the computational method to explain the vibrational modes was determined by comparing simulated spectra with experimental spectra. On isotope labeling of carbon, the frequency was shifted significantly.
Citation: Ramesh Rijal, Hari Prasad Lamichhane, Kiran Pudasainee. Molecular structure, homo-lumo analysis and vibrational spectroscopy of the cancer healing pro-drug temozolomide based on dft calculations[J]. AIMS Biophysics, 2022, 9(3): 208-220. doi: 10.3934/biophy.2022018
[1] | Andrea Borio, Martina Busetto, Francesca Marcon . SUPG-stabilized stabilization-free VEM: a numerical investigation. Mathematics in Engineering, 2024, 6(1): 173-191. doi: 10.3934/mine.2024008 |
[2] | Siddhartha Mishra . A machine learning framework for data driven acceleration of computations of differential equations. Mathematics in Engineering, 2019, 1(1): 118-146. doi: 10.3934/Mine.2018.1.118 |
[3] | Daniele Cerroni, Florin Adrian Radu, Paolo Zunino . Numerical solvers for a poromechanic problem with a moving boundary. Mathematics in Engineering, 2019, 1(4): 824-848. doi: 10.3934/mine.2019.4.824 |
[4] | Yu Leng, Lampros Svolos, Dibyendu Adak, Ismael Boureima, Gianmarco Manzini, Hashem Mourad, Jeeyeon Plohr . A guide to the design of the virtual element methods for second- and fourth-order partial differential equations. Mathematics in Engineering, 2023, 5(6): 1-22. doi: 10.3934/mine.2023100 |
[5] | Paola F. Antonietti, Ilario Mazzieri, Laura Melas, Roberto Paolucci, Alfio Quarteroni, Chiara Smerzini, Marco Stupazzini . Three-dimensional physics-based earthquake ground motion simulations for seismic risk assessment in densely populated urban areas. Mathematics in Engineering, 2021, 3(2): 1-31. doi: 10.3934/mine.2021012 |
[6] | Andrea Manzoni, Alfio Quarteroni, Sandro Salsa . A saddle point approach to an optimal boundary control problem for steady Navier-Stokes equations. Mathematics in Engineering, 2019, 1(2): 252-280. doi: 10.3934/mine.2019.2.252 |
[7] | Jinghong Li, Hongyu Liu, Wing-Yan Tsui, Xianchao Wang . An inverse scattering approach for geometric body generation: a machine learning perspective. Mathematics in Engineering, 2019, 1(4): 800-823. doi: 10.3934/mine.2019.4.800 |
[8] | Giorgia Franchini, Valeria Ruggiero, Federica Porta, Luca Zanni . Neural architecture search via standard machine learning methodologies. Mathematics in Engineering, 2023, 5(1): 1-21. doi: 10.3934/mine.2023012 |
[9] | Thomas J. Radley, Paul Houston, Matthew E. Hubbard . Quadrature-free polytopic discontinuous Galerkin methods for transport problems. Mathematics in Engineering, 2024, 6(1): 192-220. doi: 10.3934/mine.2024009 |
[10] | Claudio Canuto, Davide Fassino . Higher-order adaptive virtual element methods with contraction properties. Mathematics in Engineering, 2023, 5(6): 1-33. doi: 10.3934/mine.2023101 |
The molecular structure and spectroscopic analysis of the Temozolomide molecule have been performed using the density functional theory in neutral and anion states as well as with the addition of DMSO solvent. The 6-311G(d) basis set was employed to optimize the molecular structure of the TMZ molecule using the DFT/B3LYP method. The HOMO-LUMO energies and MEP map were computed to determine the energy gap and probable sites of electrophilic and nucleophilic reactivity in the 6-311G+(d) basis set. The vibrational frequencies were calculated using a computational method and the major fundamental modes of vibration were assigned to their respective frequencies. The potential of the computational method to explain the vibrational modes was determined by comparing simulated spectra with experimental spectra. On isotope labeling of carbon, the frequency was shifted significantly.
The Galerkin-finite element (FE) method applied to partial differential equations (PDEs) with advection terms dominating over diffusion ones may suffer of numerical instability [22,34]. These numerical instabilities cause the numerical solution to exhibit oscillations that increase in amplitude with a local increment of the transport dominance over diffusion, i.e., as soon as the local Péclet number becomes larger than one. In order to eliminate (or at least mitigate) numerical instabilities, a general employed strategy is to consider the generalized Galerkin method, i.e., the Galerkin method with additional stabilization terms [34]. Examples of stabilization methods to reduce numerical oscillations in the advection dominated regimes are for instance the upwind and streamline-diffusion method, both methods that are not strongly consistent. Instead, examples of strongly consistent methods are the Galerkin-least-squares, the streamline upwind Petrov-Galerkin (SUPG), and the Douglas-Wang methods [6,34,41]. In particular, for these strongly consistent stabilization methods, the formulation depends on the residual of the PDE (in strong formulation), other than on a parameter – called stabilization parameter – whose definition is crucial for the success of the stabilization strategies. Determining the values of such stabilization parameter is not straightforward, especially for 2D and 3D problems. In addition, a universal formulation of such parameter is lacking, especially as it is strongly dependent on data of the model and the numerical setting used for the FE approximation of the PDEs, e.g., low and high order FE methods, spectral element methods, isogeometric methods, etc. [7,12,42]. Some of the formulations for the stabilization parameter have been derived from analytical considerations on the differential problem in 1D, others are suitable only for a specific numerical approximation method, while the most comprehensive ones incorporate some (empirical) dependence on numerical setting, as e.g., the order of the FE [5,6,8,9,10,16,18,38,43,44,45].
In this work, we propose using machine learning (ML) [19,30,50] to make computers learn autonomously the values of the stabilization parameter for the FE approximation of advection dominated PDEs. Artificial neural networks (ANNs) [27,46] are widely popular in ML and Deep Learning for a wide array of applications: they are indeed very versatile tools that are increasingly finding their way in scientific computing [29,31], especially in the context of numerical approximation of PDEs. For instance, as substitute to standard numerical methods, ANNs can be employed as meshless methods in physics informed neural networks (PINNs) to directly approximate the solution of the PDE as it is trained by minimizing the (strong) residual of the PDE [35,36,37]. ANNs are also largely employed in a data-driven fashion in the context of model order reduction for parametric PDEs [17,20,21,40,51] and to enhance the stability properties of numerical methods for PDEs [13]. In fluid dynamics modelling, ANNs are massively adopted for flow features extractions, modelling, optimization and control. For flow features extractions, ANNs are used through clustering and classification to classify wake topologies [11]; for modeling fluid dynamics by reconstructing specific flows such as the near wall field in a turbulent flow [28] and for flow optimization [32], and control for aerodynamics applications [4]. ANNs are also used in a data-driven framework as a manner for providing alternative closure models for stress tensor [14] in Reynolds-Average Navier-Stokes (RANS) equations, for sub-grid scale models in large eddy simulation (LES) turbulence models [23,24,48,49,52], or for model learning input-output relationships in complex physical processes [39].
In this work, we use ANNs to learn the optimal stabilization parameter in advection dominated PDEs that are discretized by means of the FE method: the goal is to enhance the accuracy of the SUPG FE method by optimally selecting the stabilization parameter under different data of the PDE and numerical settings of the FE method. We found that the proposed ANN-enhanced stabilization method allows to improve accuracy and stabilization properties of the numerical solution compared to those results obtained by analytical expressions of the stabilization parameter. The numerical results obtained shed light also on the possibility to apply the presented strategy to learn closure laws for stabilization and turbulence models of fluid dynamics, as for instance to learn the stabilization parameters in the Variational Multiscale–LES model to model transitional and turbulent flows [3,15,54].
This work is organized as follows: in Section 2, we recall the SUPG stabilization method for advection-diffusion equations; in Section 3, we present our numerical strategy to compute an optimal SUPG stabilization parameter through a feed-forward fully connected ANN. In Section 4 we validate our method by comparing the ANN results with those obtained with the 1D advection-diffusion problem from which the expression of the theoretical stabilization parameter has been derived. In Section 5 we first show the ANN's training and we present our numerical results on the 2D advection-diffusion problem used for training and we finally generalize our findings using the ANN's prediction on a different advection-diffusion problem. Finally, in Section 7 we draw our conclusions highlighting possible future developments.
We briefly recall the advection-diffusion differential problem and the SUPG stabilization method for the advection dominated regime.
Let Ω∈Rd,d=1,2,3 be the physical domain with ∂Ω being its boundary. We consider the following problem in the unknown function u:
{−∇⋅(μ∇u)+β⋅∇u=finΩ,u=gon∂Ω, | (2.1) |
where μ, β, and f are assigned functions or constants, with μ∈L∞(Ω), β∈[L∞(Ω)]d, with ∇⋅β∈L2(Ω), and f∈L2(Ω). The Dirichlet datum on the boundary is g∈H1/2(∂Ω). Let Vg={v∈H1(Ω):v|∂Ω=g} and V0=H10(Ω); the weak formulation of Eq (2.1) reads
findu∈Vg : a(u,v)=F(v)for all v∈V0. | (2.2) |
with the bilinear form a(u,v) and the linear functional F(v) respectively defined as:
a(u,v):=∫Ωμ∇u⋅∇vdΩ+∫Ωvβ⋅∇udΩ,F(v):=∫ΩfvdΩ. |
We consider a family of function spaces Vh⊂V (either for Vg and V0) dependent on a parameter h such that dim(Vh)=Nh<∞. Let Xhr={vh∈C0(¯Ω):vh|K∈Pr,for all K∈Th} be the function space of the FE discretization with piecewise Lagrange polynomials of degree r≥1, Th a triangulation of Ω and h the characteristic size of the mesh, comprised of elements K∈Th. By setting Vh=Xrh⋂V0, the Galerkin FE method applied to Eq (2.2) reads
finduh∈Vg,h : a(uh,vh)=F(vh)for all vh∈Vh, | (2.3) |
where Vg,h=Xrh⋂Vg. The standard Galerkin-FE method, in Eq (2.3), can generate numerical oscillations on uh if the problem is dominated by the advection term. In particular, these numerical instabilities can arise if the local Péclet number is Peh>1, where
Peh=|β|h2μ. | (2.4) |
The generalized Galerkin method ([33]) allows for eliminating or mitigating numerical oscillations by adding stabilization terms to the standard Galerkin formulation as
finduh∈Vg,h : a(uh,vh)+bh(uh,vh)=F(vh)for all vh∈Vh. | (2.5) |
In the SUPG method, the additional stabilization term reads:
bh(uh,vh)=∑K∈Th∫KR(uh)τK12(∇⋅(βvh)+β⋅∇vh)dΩ, | (2.6) |
where R(uh) is the residual in strong formulation of Eq (2.1), which is defined as:
R(uh):=−∇⋅(μ∇uh)+β⋅∇uh−f. |
The term τK, appearing in Eq (2.6), is the stabilization parameter, which is the focus of this work. The stabilization parameter τK is generally defined locally, i.e., mesh element by element. In this paper, we consider a uniform stabilization parameter, thus τK=τ for all K∈Th.
A universal and optimal definition of τ in terms of the problem data and numerical settings like the mesh size and FE degree is lacking. An extensive review of stabilization parameters for the SUPG method is reported in [25]. The most common choices for τ come from an analytic derivation made for the advection-diffusion problem in 1D with f=0 and approximated by means of linear finite elements, which reads:
˜τ1=h2|β|ξ(Peh), | (2.7) |
where ξ(θ) is the upwind function:
ξ(θ)=coth(θ)−1θ,θ>0. |
If a uniform mesh is used, as we consider in this paper, then the value of h is uniform over Th; if in addition β and μ are constant, then this implies that τ is uniform over Th. The choice of τ made in Eq (2.7) represents an optimal choice of the stabilization parameter as it yields a nodally exact numerical solution for the 1D advection-diffusion problem if f=0 and the FE polynomial order is r=1 [18,34]. Thus, the stabilization parameter of Eq (2.7) may not be fully effective to provide the optimal stabilization for a general advection-diffusion problem, e.g., to guarantee a nodally exact solution in 2D/3D or when using FE degrees larger than r=1. A commonly used generalization of the formula of Eq (2.7) to higher FE degrees (r>1) is presented in [18] and reads:
˜τr=h2|β|rξ(Pehr). | (2.8) |
Differently from Eq (2.7), the stabilization parameter ˜τr in Eq (2.8) takes into account the contribution of higher polynomial degrees r. Still, this formula is not optimal as it does not guarantee a nodally exact numerical solution. Our goal is to find a general and optimal expression of the stabilization parameter holding for advection-diffusion problems and FE approximations of degree r≥1 to be dependent on: the dimension d, the FE degree r, the mesh size h, the forcing term f, the diffusion coefficient μ and the transport coefficient β.
We present our approach to determine the optimal stabilization parameter τ for the SUPG stabilization method by using an ANN. We consider as features (inputs) of our ANN: the FE degree r, the mesh size h and the global Péclet number Peg:=|β|L/(2μ) of the advection-diffusion problem, where L is the characteristic length of the problem, β∈Rd and μ∈R. As we consider β to be uniform and fixed, using Peg as input corresponds to varying the value of the diffusion coefficient μ. The features (input) of the ANN read:
x(i)=[r,h,Peg]; | (3.1) |
the target (output) of interest is the optimal SUPG stabilization parameter that we denote with τ∗:
y(i)=[τ∗]. | (3.2) |
The first step consist in generating the dataset, i.e., pairs of inputs and outputs to be used for training the ANN (data generation step). This consists in choosing repeatedly and randomly values of the features x(i) in given ranges. These features are used to feed an optimization problem that, through an optimizer and a suitable error measure, provide an optimal stabilization parameter y(i), i.e., the target of the given feature. Such optimization problem considers as error measure E(τ): the mismatch between the numerical solution uh and the exact one uex over the nodes of the FE mesh. E(τ) reads as:
E(τ)=Kh∑k=1|e(xk,τ)|,e(xk,τ)=uh(xk;τ)−u(xk). | (3.3) |
being xk the k-th node of the FE mesh Th and Kh the number of nodes. E(τ) is an approximation of the L1(Ω) norm. To find the minimum of E(τ) for different problem configurations we solve, for each instance of the input parameters x(i), the following optimization problem:
findτ∗ :minτE(τ). | (3.4) |
Thus, the dataset generated consists of m pairs of inputs-outputs (x(i),y(i)), with i=1,…,m. The latter is used for training the ANN. The latter will be then used to predict the optimal stabilization parameter y(j)=τANN to be used for the FE approximation of the advection-diffusion problem in the settings provided by x(j).
We report in Figure 1 a sketch of the procedure used to build the ANN for the prediction of the optimal stabilization parameter for any new feature x(j).
In this section, we validate the proposed numerical strategy by means of a 1D advection-diffusion problem from which the expression of ˜τ1 in Eq (2.7) has been derived. In particular, we consider the advection-diffusion problem in Eq (2.1) with Ω=(0,1), f=0 and with Dirichlet BCs u=0 on x=0 and u=1 on x=1. It admits the following exact solution:
u(x)=exp(βμx)−1exp(βμ)−1. |
We recall that the stabilization parameter ˜τ1 of Eq (2.7) yields a nodally exact numerical solution if Eq (2.1) is solved by linear FE (r=1).
We plot in Figure 2 the error measure E(τ) against the value of the stabilization parameter τ, with h=1/20, Peh=12.5 and by using r=1 (Figure 2a) and r=3 (Figure 2b). We observe that E(τ) shows a minimum in τ∗, thus suggesting the possibility to use an optimization algorithm to solve the problem. Specifically, we employ the L-BFGS-B optimization algorithm from SciPy, an open source library for Python [47]. Instead, the advection-diffusion problem has been solved using the FE open source library FEniCS [2], by applying the SUPG method on a uniform mesh. Particularly, the optimal value τ∗ found for the case r=1 corresponds to the one provided by the theory in Eq (2.7). For the case r=3, we observe a optimal value of τ∗ for which the error is minimized: in this case, it does not corresponds to the stabilization parameter ˜τr provided by the theory in Eq (2.8): as a matter of fact, the latter arises from empirical considerations to extend the parameter ˜τ1 in Eq (2.7) to polynomials degrees r>1. However, this does not ensure a nodally exact numerical solution.
We solve the optimization problem for different values of the local Péclet number 1≤Peh≤250 and we plot in Figure 3 the optimal stabilization parameter τ∗ against the local Péclet number for r=1,2, and 3.
Figure 3a shows that, by employing a linear FE space r=1, the optimal stabilization parameter τ∗ obtained by minimizing E(τ) exactly matches the theoretical one ˜τ1 for every value of Peh, thus confirming the findings of Figure 2a. This also serves as validation of the optimization procedure that we proposed. By recalling that ˜τ1 of Eq (2.7) guarantees the numerical solution to be exact at nodes for this particular advection-diffusion problem and r=1, we can infer that the optimization procedure is meaningful and it can be therefore exploited further, for example for r>1. On the other hand, Figure 3b and 3c show instead a mismatch between the theoretical ˜τr of Eq (2.8) and the optimal one τ∗, thus confirming the findings reported in Figure 2b.
In order to better appreciate the differences in the numerical solutions due to the choice of the stabilization parameters, we report, in Figure 4, a comparison of the error E(τ) obtained by means of the optimal τ∗ and theoretical ˜τr stabilization parameters for r=2 and 3. Moreover, we report in Figure 5 a comparison among the exact solution u, the SUPG-stabilized numerical solution u∗h obtained with the optimal stabilization parameter τ∗, and the numerical solution ˜uh obtained with theoretical one ˜τr. Specifically, we consider FE of degree r=3 and two different values of Peh. In both these cases, the optimal parameter τ∗ leads to a more accurate solution with respect to using the theoretical parameter ˜τr. In particular, when Peh is "small", ˜τr leads to overshooting in the numerical solution, while if Peh is "large" the theoretical stabilization parameter leads to undershooting. Conversely, the optimal parameter τ∗ accurately intercepts the exact solution at the nodes.
We first introduce the training set of our problem and we detail the setup of the ANN that we use in this work. Then, we show the prediction of the stabilization parameter by means of the ANN on different advection-diffusion problems.
We apply the strategy presented so far to a 2D advection-diffusion problem to generate the dataset for the training of the ANN. Specifically, we consider in Eq (2.1): Ω=(0,1)2, f=0, and β=(1,1). We prescribe the following exact solution on the whole boundary ∂Ω:
u(x,y)=e(x/μ)−1e(1/μ)−1+e(y/μ)−1e(1/μ)−1. | (5.1) |
We generate in Ω a structured mesh Th of triangles with FEniCS [2], as shown in Figure 6. We generate the dataset by repeatedly solving the optimization problem of Eq (3.4) for varying set of features as described in Section 3; specifically, we choose the features as reported in Eq (3.1). The complete dataset contains m=900 examples with r={1,2,3}, h={√210,√220,√240} and values of Peg randomly chosend in the range [7,70′710] (uniform distribution), which yields μ∈[10−5,10−1]. We summarize the details for generating the dataset in Table 1. Figure 7 provides an overview of the dataset used for the training of the ANN, specifically the features x(i)=[r,h,Peg] and the target y(i)=[τ∗]. In this case, the characteristic length L is fixed and set equal to L=1.
# Data set (m) | # Training set | # Validation set | r | h | Peg |
900 | 720(80%) | 180(20%) | {1,2,3} | {√210,√220,√240} | randomly in [7,70′710]. |
We train a fully-connected feed-forward ANN on the generated dataset by using the open source library Keras [26] built on top of TensorFlow [1]. We divide the dataset into two parts: a training dataset that takes 80% of the examples to be used for the ANN training and a validation dataset that takes the remaining 20%. We choose the loss function as the mean squared error that measures, for each training feature, the squared mismatch between the prediction of the ANN ˆy(j) and the actual target y(j). Specifically, the loss function is defined as
J=12mm∑j=1(ˆy(j)−y(j))2. | (5.2) |
We normalize the features by subtracting their sample mean and dividing by their sample standard deviation in order to help the weights to better adapt to the different scales of the features. Moreover, the targets and the feature Peg need special care as they are distributed over a wide range of values. Thus, we normalize them using by applying a base 10 logarithm. The normalized features and targets read:
˜x=[r−¯rσr,h−¯hσh,log10(Peg)−¯log10(Peg)σlog10(Peg)],˜y=[−log10(τ∗)], |
where ¯r, ¯h, ¯log10(Peg) are the sample mean of the training features r, h and the logarithm of Peg respectively, while σr, σh and σlog10(Peg) are their sample standard deviations.
In order to find the best performing ANN architecture, we carried out a study by testing different numbers of hidden layers, nodes per layer, optimization algorithm, its learning rate, and batch size. A comparison of the loss function with different architectures is given in Figure 8. Using 3 hidden layers is beneficial in terms of validation error drops, while using more than 64 nodes per layer does not bring to considerable advantages. Finally, we choose an ANN with 3 hidden layers, 64 nodes per layer and an output layer with a single node, all using a rectified linear unit (ReLU) activation function. We display the ANN architecture in Figure 9. We trained the ANN with the SGD optimization algorithm, a constant learning rate of 0.01, and mini-batch size of 32 samples. Moreover, we employed a momentum of 0.9 in the optimization algorithm to update the weights. The trained ANN is available in the GitLab repository [53].
Regarding the computational efficiency of the proposed strategy, we stress the clear distinction between the offline phase (dataset generation and ANN's training) and the online phase, where we use the ANN to predict a new stabilization parameter – alongside the use of the FE solver – for unseen input parameter values. The most demanding part of the strategy is the dataset generation, requiring approximately 2 h on a standard laptop to repeatedly solve the optimization problem 900 times. Moreover, the ANN's training phase required approximately 10'. The testing (online) phase, which is the one that is performed for application purposes, is considerably inexpensive, requiring only the real-time evaluation of a composition of linear functions, comparable with the evaluation of the empirical relation that brings to the theoretical values (few milliseconds).
Now, we compare the predictions of the ANN with the theoretical stabilization parameter ˜τr of Eq (2.8) [18,34]. We show in Figure 10 (left) the stabilization parameter τANN predicted by the ANN by varying mesh size h and global Péclet number Peg. For comparison, we report in Figure 10 (right) the corresponding theoretical stabilization parameter ˜τr. We observe that the overall behavior of the trained ANN's predictions are qualitatively similar of the theoretical stabilization parameter ˜τr. Nevertheless, it can be inferred that the values of the stabilization parameters are almost completely matched with linear FE (r=1), while they quantitatively differ for r=2 and r=3. This was expected as ˜τr for r>1 is an empirical extension of the formula for the case r=1.
The ANN allows to make predictions with features outside the range of values for which it has been trained, that is for unseen values of such features. With this aim, we report in Figure 11 the comparison of the ANN's predictions τANN with ˜τr for the FE degree r=4. This comparison shows a clear difference between the theoretical and ANN's τ for r=4 even though the general trend is maintained similar.
We compare the numerical solutions uANNh and ˜uh obtained by means of the SUPG stabilization method with the parameter τANN predicted by the ANN and the theoretical one ˜τr, respectively; the comparison also involves the exact solution u (5.1) of the 2D advection-diffusion problem used for the training of the ANN. In particular, we display the comparison of the former 2D solutions in Figure 12 along the line (1−h,y) for any y∈[0,1] with: (a) Peh=2, r=1, and h=√2/10 (Figure 12a); (b) Peh=500, r=3, and and h=√2/20 (Figure 12b). We notice that in both the cases, the numerical solution uANNh involving the stabilization parameter τANN provides more accurate results than with the theoretical stabilization paramter ˜τr. In particular, in the case (a), ˜uh involves a much smoother boundary layer and overshoots the exact solutions u, conversely to the nearly nodally exact numerical solution uANNh. In the case (b), uANNh provides a much better representation of the bundary layer, without the undershooting of the solution u exhibited by ˜uh. Furthermore, we report in Table 2 absolute errors between the numerical solutions (uANNh and ˜uh) and the exact one u. The errors, computed in L2(Ω) and H1(Ω) norms, show that the ANN-based SUPG stabilization method very often produces more accurate results than the ones obtained with theoretical stabilization parameter ˜τr, especially for r=3.
˜τr (e=˜uh−u) | τANN (e=uANNh−u) | |||||
r | h | Peh | ||e||L2(Ω) | ||e||H1(Ω) | ||e||L2(Ω) | ||e||H1(Ω) |
1 | √2/10 | 2 | 9.56⋅10−2 | 7.93⋅10−1 | 6.49⋅10−2 | 5.31⋅10−1 |
2 | √2/10 | 2 | 6.48⋅10−2 | 5.31⋅10−1 | 6.50⋅10−2 | 5.33⋅10−1 |
3 | √2/10 | 2 | 6.50⋅10−2 | 5.33⋅10−1 | 6.49⋅10−2 | 5.32⋅10−1 |
1 | √2/20 | 500 | 2.99⋅10−4 | 5.30⋅10−3 | 3.11⋅10−5 | 5.50⋅10−4 |
2 | √2/20 | 500 | 2.71⋅10−2 | 4.86⋅10−1 | 2.50⋅10−2 | 4.48⋅10−1 |
3 | √2/20 | 500 | 1.05⋅10−2 | 1.90⋅10−1 | 1.68⋅10−3 | 3.46⋅10−2 |
Moreover, to assess the ability of the ANN to predict the stabilization parameter out of the training range, we compare in Figure 13 the numerical solutions for high Péclet in the case r=4. We observe that uANNh is more accurate than ˜uh even with FE degree out of the training range.
We check the model generalization of the ANN trained for the 2D advection-diffusion problem with exact solution u of Eq (5.1) by predicting τANN for an unseen 2D advection-diffusion problem. In particular, we consider the advection-diffusion problem of Eq (2.1) in Ω=(0,1)2, with f=1 and β=(1,1). We prescribe the following exact solution on the whole boundary ∂Ω:
u(x,y)=12(x+y)+1−12(e(x/μ)+e(y/μ))e(1/μ)−1. | (5.3) |
We compare in Figure 14 the numerical solutions uANNh and ˜uh with the novel exact solution u for different Péclet and mesh sizes. As for the previous numerical tests, the stabilization parameter τANN predicted by the network provides more accurate numerical solutions uANNh than with the theoretical stabilization parameter ˜τr. In particular, the boundary layers and overall solution behaviours are better represented.
We better assess the former qualitative consideration, by computing the error E(τ) associated with the numerical solutions with SUPG stabilization for the parameters τANN and ˜τr; different values of Peg and r are considered. In particular, Figure 15 compares E(τ) against Peg (in logarithmic scale) for different values of r: the error obtained by using τANN is always lower than the one achieved with ˜τr. These results indicate that τANN, although trained on a specific advection-diffusion problem, can be used to make predictions of the optimal τ for an unseen advection-diffusion problem in place of the theoretical stabilization parameter.
In this section, we consider an advection-diffusion problem with constant forcing term f=1, advection coefficient β=(1,1), and boundary conditions u=0 on ∂Ω. Although there is not an exact solution to the given problem, we consider as "ground truth" (reference) solution a numerical solution obtained on a much finer grid (h=√2/400) without stabilization. In Figure 16, we compare the SUPG numerical solutions ˜uh, uANNh against our "ground truth" solution for two different Péclet numbers. The numerical solution with the stabilization parameter τANN provides more accurate results with respect to the the solution obtained with the theoretical parameter. In particular, we highlight the largest discrepancies observed in the corners of the extracted solution.
In this section, we cope the case of a non costant forcing term by considering advection-diffusion problem of Eq (2.1) in Ω=(0,1)2 with β=(1,1), and we prescribe the following exact solution on the whole boundary ∂Ω:
u(x,y)=−atan((x−1/2)2+(y−1/2)2−1/16)√μ. | (5.4) |
We display the exact solution of the considered problem in Figure 17.
In particular, Figures 18 and 19 show E(τ) against Peg for different values of r with two different meshes with h=√2/10 and h=√2/20, respectively. The error obtained by using τANN is always comparable to the one achieved with ˜τr, for both mesh levels and for all the FE degrees considered. These results suggest that τANN, although trained on a specific advection-diffusion problem with f=0, is robust with respect to unseen parameters and data in the advection-diffusion problem, including non constant f. Nevertheless, further studies can be conducted to better assess the role of a non-constant forcing term into the ANN's training phase, possibly encompassing the accuracy of the theoretical stabilization parameter in this scenario too.
We investigate the effect of the advection direction on the value of the stabilization parameter. We define θ as the angle between the advection velocity and the x-axis: β=(|β|cosθ,|β|sinθ)T. We carry out the optimization strategy introduced in Section 3 including also θ in the input parameter x(i). We apply the optimization to the advection-diffusion problem with exact solution in Eq (5.3) varying θ in [π/12,π/2] and we report a comparison between the optimal, theoretical and ANN's stabilization parameter in Figure 20 (left). We set |β|=√2, Peg=7′071, h=√2/20, r=3. We recall that, differently from the optimization strategy, in this plot we are still employing the ANN trained without accounting for the advection direction. We can observe that the optimal τ is affected by θ, a feature that is not accounted by the theoretical stabilization parameter. Furthermore, in Figure 20 (right), we compare the mean errors obtained with the optimal, theoretical and ANN's stabilization parameter. It can be observed that the ANN still provides an advantage with respect to the theoretical stabilization. However, we believe that by including the advection direction as an additional feature in the training phase of the ANN, a higher accuracy can be obtained that the one achieved in this paper, where a single direction (θ=π4) has been considered.
In this work, we presented an approach based on machine learning and ANN to compute the optimal stabilization parameter to be used in the SUPG FE approximation of advection-diffusion problems. Indeed, albeit the expression of the stabilization parameter is available for 1D problems and FE of degree r=1, its extension to more general advection-diffusion problems (2D and 3D) and FE degrees r>1 is still lacking.
We validated our approach against the 1D case, for which the ANN-stabilization parameter matches the already optimal, theoretical one for r=1, leading to nodally exact numerical solutions Instead, for higher polynomials degrees r>1, remarkable differences are observed among the theoretical and optimal stabilization parameter: we observed better accuracy and stabilization properties of the numerical solution with the optimal stabilization parameter with respect to the theoretical one.
Then, we generated the dataset on a 2D advection-diffusion problem, and we used it to train a fully-connected feed-forward ANN. We applied the predictions of the network on the original advection-diffusion problem for unseen input values and we also apply it to an unseen advection-diffusion problem to check for model generalization. Our numerical results showed that the proposed ANN-based approach provides more accurate numerical solutions than using the theoretical stabilization parameter for the SUPG method.
This work represents a step towards the enhancements of stabilization methods for the FE approximation of advection-dominated differential problems. In particular, this work is limited to few features for the ANN-based stabilization parameter and provides as output a single optimal stabilization parameter meant for the whole mesh FE Th. Natural extensions of this work will therefore involve local stabilization parameters, i.e., element by element over the mesh Th, to better account for non-uniform meshes, differential problems with varying coefficients, and capturing the local behaviour of the solution. In addition, to enhance the robustness of the ANN-based approach, possible additional inputs of the network are the angle of the advection velocity and the nodal value of the forcing term. A future development consists in the extension of the proposed approach to the 3D case for which, as for the 2D case, a universal definition of the stabilization parameter does not exist. The extension to the 3D case surely would present a larger cost in the training phase; however, once trained, the ANN could be used online real-time.
Finally, we envision extending the proposed machine learning approach to SUPG and variational multiscale stabilization methods for the FE approximation of the Navier-Stokes equations.
The authors acknowledge Prof. C. Canuto, Politecnico di Torino, for the fruitful discussions and useful suggestions. L. D. and A. Z. are members of the INdAM Research group GNCS. L. D. has been partially funded by the research project PRIN 2020, n.20204LN5N5, by MIUR. The authors ackwnowledge the anonymous reviewers for their insightful comments and suggestions.
The authors declare no conflict of interest.
[1] |
Newlands ES, Stevens MFG, Wedge SR, et al. (1997) Temozolomide: a review of its discovery, chemical properties, pre-clinical development and clinical trials. Cancer Treat Rev 23: 35-61. https://doi.org/10.1016/S0305-7372(97)90019-0 ![]() |
[2] |
Svec RL, McKee SA, Berry MR, et al. (2022) Novel imidazotetrazine evades known resistance mechanisms and is effective against temozolomide-resistant brain cancer in cell culture. ACS Chem Biol 17: 299-313. https://doi.org/10.1021/acschembio.2c00022 ![]() |
[3] |
Wesolowski JR, Rajdev P, Mukherji SK (2010) Temozolomide (Temodar). Am J Neuroradiol 31: 1383-1384. https://doi.org/10.3174/ajnr.A2170 ![]() |
[4] | National Center for Biotechnology Information: PubChem Compound Summary for CID 11830328, Temozolomide Acid, 2022. Available from: https://pubchem.ncbi.nlm.nih.gov/compound/Temozolomide-Acid |
[5] |
Villano JL, Seery TE, Bressler LR (2009) Temozolomide in malignant gliomas: current use and future targets. Cancer Chemoth Pharm 64: 647-655. https://doi.org/10.1007/s00280-009-1050-5 ![]() |
[6] |
Sansom C (1999) Temozolomide presents breakthrough in glioblastoma multiforme treatment. Pharm Sci Technol Toda y 4: 131-133. ![]() |
[7] |
Louis DN, Perry A, Reifenberger G, et al. (2016) The 2016 World Health Organization classification of tumors of the central nervous system: a summary. Acta Neuropathol 131: 803-820. https://doi.org/10.1007/s00401-016-1545-1 ![]() |
[8] |
O'reilly SM, Newlands ES, Brampton M, et al. (1993) Temozolomide: A new oral cytotoxic chemotherapeutic agent with promising activity against primary brain tumours. Eur J Cancer 29: 940-942. https://doi.org/10.1016/S0959-8049(05)80198-4 ![]() |
[9] |
Bower M, Newlands ES, Bleehen NM, et al. (1997) Multicentre CRC phase II trial of temozolomide in recurrent or progressive high-grade glioma. Cancer Chemoth Pharm 40: 484-488. https://doi.org/10.1007/s002800050691 ![]() |
[10] |
Klepper B, Pauker D (2006) Medicare's drug plan: huge price disparities for common cancer drugs. Community Oncol 12: 753-755. https://doi.org/10.1016/S1548-5315(11)70942-1 ![]() |
[11] |
Thomas A, Tanaka M, Trepel J, et al. (2017) Temozolomide in the era of precision medicine. Cancer Res 77: 823-826. https://doi.org/10.1158/0008-5472.CAN-16-2983 ![]() |
[12] |
Kumer A, Sarker MN, Paul S (2019) The thermo physical, HOMO, LUMO, vibrational spectroscopy and QSAR study of morphonium formate and acetate ionic liquid salts using computational method. Turk Comput Theor Chem 3: 59-68. https://doi.org/10.33435/tcandtc.481878 ![]() |
[13] | Kumer A, Paul S, Sarker M, et al. (2019) The prediction of thermo physical, vibrational spectroscopy, chemical reactivity, biological properties of morpholinium borate, phosphate, chloride and bromide ionic liquid: a DFT study. Int J New Chem 6: 236-253. https://dx.doi.org/10.22034/ijnc.2019.110412.1053 |
[14] |
Yahiaoui K, Seridi L, Mansouri K (2021) Temozolomide binding to Cucurbit[7]uril: QTAIM, NCI-RDG and NBO analyses. J Incl Phenom Macro Chem 99: 61-77. https://doi.org/10.1007/s10847-020-01027-5 ![]() |
[15] | Frisch MJ, Trucks GW, Schlegel HB, et al. (2009) Gaussian 09 Package. Wallingford, CT: Gaussian. Inc.. |
[16] |
Becke A (1993) Density-functional thermochemistry.III. The role of exact exchange. J Chem Phys 98: 5648-5652. https://doi.org/10.1063/1.464913 ![]() |
[17] |
Lee C, Yang W, Parr RG (1998) Development of the Colle-Salvetti correlation-energy formula into a functional of the electron density. Phys Rev B 37: 785-789. https://doi.org/10.1103/PhysRevB.37.785 ![]() |
[18] | Dennington R, Keith T, Millam J (2009) GaussView, Version 5.0. 8, R. Dennington: Semichem Inc. |
[19] |
Koopmans T (1934) Über die zuordnung von wellenfunktionen und eigenwerten zu den einzelnen elektronen eines atoms. Physica 1: 104-113. https://doi.org/10.1016/S0031-8914(34)90011-2 ![]() |
[20] |
O'boyle NM, Tenderholt AL, Langner KM (2008) A library for package-independent computational chemistry algorithms. J Comput Chem 29: 839-845. https://doi.org/10.1002/jcc.20823 ![]() |
[21] |
Jamróz MH (2013) Vibrational energy distribution analysis (VEDA): scopes and limitations. Spectrochim Acta A Mol Biomol Spectrosc 114: 220-230. https://doi.org/10.1016/j.saa.2013.05.096 ![]() |
[22] |
Nkungli NK, Ghogomu JN (2017) Theoretical analysis of the binding of iron (III) protoporphyrin IX to 4-methoxyacetophenone thiosemicarbazone via DFT-D3, MEP, QTAIM, NCI, ELF, and LOL studies. J Mol Model 23: 200. https://doi.org/10.1007/s00894-017-3370-4 ![]() |
[23] |
Krack M, Jug K (1996) Molecular electrostatic potentials for large systems. Theoretical and Computational Chemistry. Amsterdam: Elsevier 297-331. https://doi.org/10.1016/S1380-7323(96)80047-1 ![]() |
[24] | Gunasekaran S, Balaji RA, Kumaresan S, et al. (2008) Experimental and theoretical investigations of spectroscopic properties of N-acetyl-5-methoxytryptamine. Can J Anal Sci Spectrosc 53: 149-162. |
[25] |
Kumar PS, Vasudevan K, Prakasam A, et al. (2010) Quantum chemistry calculations of 3-Phenoxyphthalonitrile dye sensitizer for solar cells. Spectrochim Acta A Mol Biomol Spectrosc 77: 45-50. https://doi.org/10.1016/j.saa.2010.04.021 ![]() |
[26] |
Sang-Aroon W, Ruangpornvisuti V, Amornkitbamrung V (2016) Tautomeric transformation of temozolomide, their proton affinities and chemical reactivities: a theoretical approach. J Mol Graph Model 66: 76-84. https://doi.org/10.1016/j.jmgm.2016.03.016 ![]() |
[27] |
AlRabiah H, Muthu S, Al-Omary F, et al. (2017) Molecular structure, vibrational spectra, NBO, Fukui function, HOMO-LUMO analysis and molecular docking study of 6-[(2-methylphenyl)sulfanyl]-5-propylpyrimidine-2,4(1H,3H)-dione. Maced J Chem Chem Eng 36: 59-80. https://doi.org/10.20450/mjcce.2017.1001 ![]() |
[28] |
Chen M, Waghmare UV, Friend CM, et al. (1998) A density functional study of clean and hydrogen-covered α-MoO3 (010): Electronic structure and surface relaxation. J Chem Phys 109: 6854-6860. https://doi.org/10.1063/1.477252 ![]() |
[29] |
Mulliken RS (1955) Electronic population analysis on LCAO–MO molecular wave functions. J Chem Phys 23: 1833-1840. https://doi.org/10.1063/1.1740588 ![]() |
[30] | Socrates G (2004) Infrared and Raman Characteristic Group Frequencies: Tables and Charts. Chichester: John Wiley and Sons Ltd 12-14. |
[31] |
Sui H, Ju X, Liu X, et al. (2014) Primary thermal degradation effects on the polyurethane film. Polym Degrad Stabil 101: 109-113. https://doi.org/10.1016/j.polymdegradstab.2013.11.021 ![]() |
[32] |
Wolpert M, Hellwig P (2006) Infrared spectra and molar absorption coefficients of the 20 alpha amino acids in aqueous solutions in the spectral range from 1800 to 500 cm−1. Spectrochim Acta A Mol Biomol Spectrosc 64: 987-1001. https://doi.org/10.1016/j.saa.2005.08.025 ![]() |
[33] |
Tanskanen H, Khriachtchev L, Lundell J, et al. (2004) Organo-noble-gas hydride compounds HKrCCH, HXeCCH, HXeCC, and HXeCCXeH: Formation mechanisms and effect of 13 C isotope substitution on the vibrational properties. J Chem Phys 121: 8291-8298. https://doi.org/10.1063/1.1799611 ![]() |
1. | Ignacio Brevis, Ignacio Muga, Kristoffer G. van der Zee, Neural control of discrete weak formulations: Galerkin, least squares & minimal-residual methods with quasi-optimal weights, 2022, 402, 00457825, 115716, 10.1016/j.cma.2022.115716 | |
2. | Sangeeta Yadav, Sashikumaar Ganesan, Ai-Augmented Stabilized Finite Element Method, 2023, 1556-5068, 10.2139/ssrn.4353564 | |
3. | Teeratorn Kadeethum, Daniel O’Malley, Francesco Ballarin, Ida Ang, Jan N. Fuhg, Nikolaos Bouklas, Vinicius L. S. Silva, Pablo Salinas, Claire E. Heaney, Christopher C. Pain, Sanghyun Lee, Hari S. Viswanathan, Hongkyu Yoon, Enhancing high-fidelity nonlinear solver with reduced order model, 2022, 12, 2045-2322, 10.1038/s41598-022-22407-6 | |
4. | Paola F. Antonietti, Matteo Caldana, Luca Dede’, Accelerating Algebraic Multigrid Methods via Artificial Neural Networks, 2023, 51, 2305-221X, 1, 10.1007/s10013-022-00597-w | |
5. | Axel Klawonn, Martin Lanser, Janine Weber, Learning adaptive coarse basis functions of FETI-DP, 2024, 496, 00219991, 112587, 10.1016/j.jcp.2023.112587 | |
6. | Ignacio Brevis, Ignacio Muga, David Pardo, Oscar Rodriguez, Kristoffer G. van der Zee, Learning quantities of interest from parametric PDEs: An efficient neural-weighted Minimal Residual approach, 2024, 164, 08981221, 139, 10.1016/j.camwa.2024.04.006 | |
7. | Matteo Caldana, Paola F. Antonietti, Luca Dede', Discovering artificial viscosity models for discontinuous Galerkin approximation of conservation laws using physics-informed machine learning, 2025, 520, 00219991, 113476, 10.1016/j.jcp.2024.113476 | |
8. | Léo Bois, Emmanuel Franck, Laurent Navoret, Vincent Vigon, An Optimal Control Deep Learning Method to Design Artificial Viscosities for Discontinuous Galerkin Schemes, 2024, 101, 0885-7474, 10.1007/s10915-024-02698-9 | |
9. | Tsung-Yeh Hsieh, Tsung-Hui Huang, A multiscale stabilized physics informed neural networks with weakly imposed boundary conditions transfer learning method for modeling advection dominated flow, 2024, 40, 0177-0667, 3353, 10.1007/s00366-024-01981-5 | |
10. | Sangeeta Yadav, Sashikumaar Ganesan, Artificial neural network-augmented stabilized finite element method, 2024, 499, 00219991, 112702, 10.1016/j.jcp.2023.112702 | |
11. | ||
12. | Johannes Haubner, Ottar Hellan, Marius Zeinhofer, Miroslav Kuchta, Learning mesh motion techniques with application to fluid–structure interaction, 2024, 424, 00457825, 116890, 10.1016/j.cma.2024.116890 | |
13. | ||
14. | ||
15. | ||
16. | ||
17. | ||
18. | ||
19. | ||
20. | ||
21. | ||
22. | ||
23. | ||
24. | ||
25. | ||
26. | ||
27. | ||
28. | ||
29. | ||
30. | ||
31. | ||
32. | ||
33. | ||
34. | ||
35. | ||
36. | ||
37. | ||
38. | ||
39. | ||
40. | ||
41. | ||
42. | ||
43. | ||
44. | ||
45. | ||
46. | ||
47. | ||
48. | ||
49. | ||
50. | ||
51. | ||
52. | ||
53. | ||
54. | ||
55. | ||
56. | ||
57. | ||
58. | ||
59. | ||
60. | ||
61. | ||
62. | ||
63. | ||
64. | ||
65. | ||
66. | ||
67. | ||
68. | ||
69. | ||
70. | ||
71. | ||
72. | ||
73. | ||
74. | ||
75. | ||
76. | ||
77. | ||
78. | ||
79. | ||
80. | ||
81. | ||
82. | ||
83. | ||
84. | ||
85. | ||
86. | ||
87. | ||
88. | ||
89. | ||
90. | ||
91. | ||
92. | ||
93. | ||
94. | ||
95. | ||
96. | ||
97. | ||
98. | ||
99. | ||
100. | ||
101. | ||
102. | ||
103. | ||
104. | ||
105. | ||
106. | ||
107. | ||
108. | ||
109. | ||
110. | ||
111. | ||
112. | ||
113. | ||
114. | ||
115. | ||
116. | ||
117. | ||
118. | ||
119. | ||
120. | ||
121. | ||
122. | ||
123. | ||
124. | ||
125. | ||
126. | ||
127. | ||
128. | ||
129. | ||
130. | ||
131. | ||
132. | ||
133. | ||
134. | ||
135. | ||
136. | ||
137. | ||
138. | ||
139. | ||
140. | ||
141. | ||
142. | ||
143. | ||
144. | ||
145. | ||
146. | ||
147. | ||
148. | ||
149. | ||
150. | ||
151. | ||
152. | ||
153. | ||
154. |
# Data set (m) | # Training set | # Validation set | r | h | Peg |
900 | 720(80%) | 180(20%) | {1,2,3} | {√210,√220,√240} | randomly in [7,70′710]. |
˜τr (e=˜uh−u) | τANN (e=uANNh−u) | |||||
r | h | Peh | ||e||L2(Ω) | ||e||H1(Ω) | ||e||L2(Ω) | ||e||H1(Ω) |
1 | √2/10 | 2 | 9.56⋅10−2 | 7.93⋅10−1 | 6.49⋅10−2 | 5.31⋅10−1 |
2 | √2/10 | 2 | 6.48⋅10−2 | 5.31⋅10−1 | 6.50⋅10−2 | 5.33⋅10−1 |
3 | √2/10 | 2 | 6.50⋅10−2 | 5.33⋅10−1 | 6.49⋅10−2 | 5.32⋅10−1 |
1 | √2/20 | 500 | 2.99⋅10−4 | 5.30⋅10−3 | 3.11⋅10−5 | 5.50⋅10−4 |
2 | √2/20 | 500 | 2.71⋅10−2 | 4.86⋅10−1 | 2.50⋅10−2 | 4.48⋅10−1 |
3 | √2/20 | 500 | 1.05⋅10−2 | 1.90⋅10−1 | 1.68⋅10−3 | 3.46⋅10−2 |
# Data set (m) | # Training set | # Validation set | r | h | Peg |
900 | 720(80%) | 180(20%) | {1,2,3} | {√210,√220,√240} | randomly in [7,70′710]. |
˜τr (e=˜uh−u) | τANN (e=uANNh−u) | |||||
r | h | Peh | ||e||L2(Ω) | ||e||H1(Ω) | ||e||L2(Ω) | ||e||H1(Ω) |
1 | √2/10 | 2 | 9.56⋅10−2 | 7.93⋅10−1 | 6.49⋅10−2 | 5.31⋅10−1 |
2 | √2/10 | 2 | 6.48⋅10−2 | 5.31⋅10−1 | 6.50⋅10−2 | 5.33⋅10−1 |
3 | √2/10 | 2 | 6.50⋅10−2 | 5.33⋅10−1 | 6.49⋅10−2 | 5.32⋅10−1 |
1 | √2/20 | 500 | 2.99⋅10−4 | 5.30⋅10−3 | 3.11⋅10−5 | 5.50⋅10−4 |
2 | √2/20 | 500 | 2.71⋅10−2 | 4.86⋅10−1 | 2.50⋅10−2 | 4.48⋅10−1 |
3 | √2/20 | 500 | 1.05⋅10−2 | 1.90⋅10−1 | 1.68⋅10−3 | 3.46⋅10−2 |