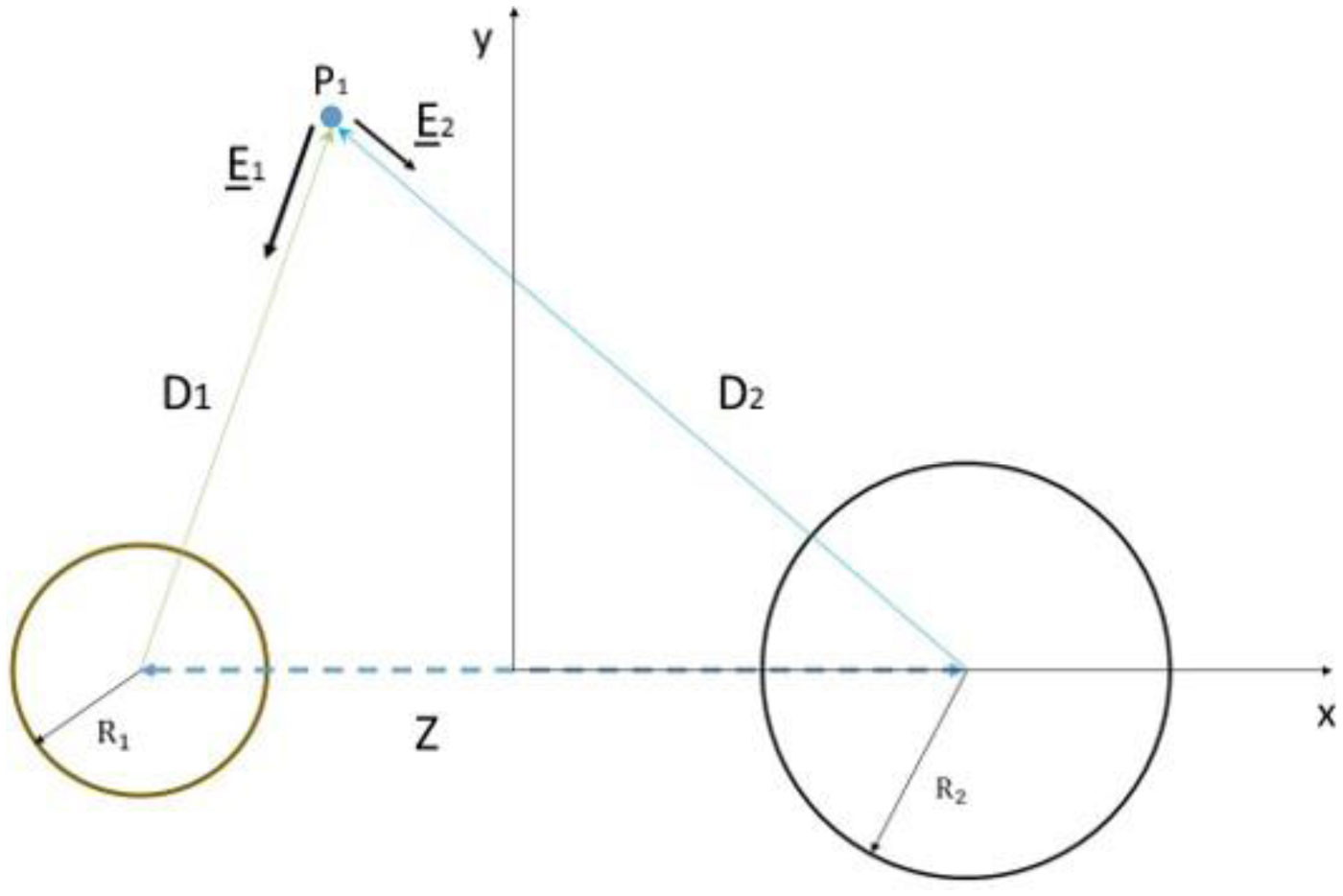
Based on the generalized version of Newton's Shell Theorem the electric field energy density, uF around two separated surface-charged spheres surrounded by electrolyte is calculated. According to the calculations when the surfaces of the charged spheres are farther from each other than four times of the Debye length the field energy density around one of the charged sphere is basically independent from the presence of the other sphere. In this case at low electrolyte ion concentration uF = 0 within the spheres and outside the sphere uF decreases with increasing distance from the surface of the sphere, while at high electrolyte ion concentration uF fast decreases with increasing inner and outer distance from the surface of the sphere. When the charged sheres are close to each other their electric interaction affects the field energy density especially where the surfaces of the spheres are close to each other. Also to model electrophoresis analytical equations are derived for the interaction energy between and the density of electric field energy around a charged flat surface and a charged sphere surrounded by neutral electrolyte.
Citation: István P. Sugár. Density of electric field energy around two surface-charged spheres surrounded by electrolyte I. The spheres are separated from each other[J]. AIMS Biophysics, 2022, 9(2): 86-95. doi: 10.3934/biophy.2022008
[1] | István P. Sugár . Density of electric field energy around two surface-charged spheres surrounded by electrolyte II. The smaller sphere is inside the larger one. AIMS Biophysics, 2022, 9(1): 61-71. doi: 10.3934/biophy.2022006 |
[2] | István P. Sugár . Electric energies of a charged sphere surrounded by electrolyte. AIMS Biophysics, 2021, 8(2): 157-164. doi: 10.3934/biophy.2021012 |
[3] |
István P. Sugár .
A generalization of the Shell Theorem. Electric potential of charged spheres and charged vesicles surrounded by electrolyte. AIMS Biophysics, 2020, 7(2): 76-89. doi: 10.3934/biophy.2020007 |
[4] |
István P. Sugár .
A generalization of the Shell Theorem. Electric potential of charged spheres and charged vesicles surrounded by electrolyte. AIMS Biophysics, 2023, 10(1): 23-24. doi: 10.3934/biophy.2023003 |
[5] | Ganesh Prasad Tiwari, Santosh Adhikari, Hari Prasad Lamichhane, Dinesh Kumar Chaudhary . Natural bond orbital analysis of dication magnesium complexes [Mg(H2O)6]2+ and [[Mg(H2O)6](H2O)n]2+; n=1-4. AIMS Biophysics, 2023, 10(1): 121-131. doi: 10.3934/biophy.2023009 |
[6] | Hanieh Hadady, Doug Redelman, Sage R. Hiibel, Emil J. Geiger . Continuous-flow sorting of microalgae cells based on lipid content by high frequency dielectrophoresis. AIMS Biophysics, 2016, 3(3): 398-414. doi: 10.3934/biophy.2016.3.398 |
[7] | Klemen Bohinc, Leo Lue . On the electrostatics of DNA in chromatin. AIMS Biophysics, 2016, 3(1): 75-87. doi: 10.3934/biophy.2016.1.75 |
[8] | Piotr H. Pawłowski, Piotr Zielenkiewicz . The role of electric charge in SARS-CoV-2 and other viral infections. AIMS Biophysics, 2024, 11(2): 166-188. doi: 10.3934/biophy.2024011 |
[9] | Massimo Fioranelli, Maria Grazia Roccia, Aroonkumar Beesham, Dana Flavin, M. Ghaeni, Faissal AZIZ . A model for considering effects of extremely low frequency electromagnetic fields on quail embryonic cells. AIMS Biophysics, 2022, 9(3): 198-207. doi: 10.3934/biophy.2022017 |
[10] | David E. Shoup . Diffusion-controlled reaction rates for clusters of binding sites on a cell. AIMS Biophysics, 2016, 3(4): 522-528. doi: 10.3934/biophy.2016.4.522 |
Based on the generalized version of Newton's Shell Theorem the electric field energy density, uF around two separated surface-charged spheres surrounded by electrolyte is calculated. According to the calculations when the surfaces of the charged spheres are farther from each other than four times of the Debye length the field energy density around one of the charged sphere is basically independent from the presence of the other sphere. In this case at low electrolyte ion concentration uF = 0 within the spheres and outside the sphere uF decreases with increasing distance from the surface of the sphere, while at high electrolyte ion concentration uF fast decreases with increasing inner and outer distance from the surface of the sphere. When the charged sheres are close to each other their electric interaction affects the field energy density especially where the surfaces of the spheres are close to each other. Also to model electrophoresis analytical equations are derived for the interaction energy between and the density of electric field energy around a charged flat surface and a charged sphere surrounded by neutral electrolyte.
The headgroups of membrane lipids have either single charge (e.g. tetraether lipids [1],[2] phosphatidic acid (PA), phosphatidylserine (PS), phosphatidylethanolamine (PE), and phosphatidylinositol (PI)) or electric dipole (e.g. phospholipids [1],[3] like dipalmitoyl-, distearoyl-, dimyristoylphosphatidylcholine). Theoretical models of lipid membranes usually focus on short range (Van der Waals) lateral interactions between nearest neighbor lipids and ignore the long range charge-charge interactions [3],[4]. This is because in the case of long range interactions one has to consider the entire system rather than the interactions between the nearest neighbor lipids. Charges, like head group charges, that are embedded into electrolyte (electroneutral ion solution), but not part of the electrolyte itself, are called external charges. The potential, V, generated by external charges can be calculated by solving the Screened Poisson Equation, ([5] and see Eq 3 in ref. [6] or Eq A17 in Appendix 4).
By using the solution of the Screened Poisson Equation Newton's Shell Theorem was generalized [6],[7] and then it was possible to calculate analytically different electrical properties of one and two concentric surface-charged spheres (surrounded inside and outside by electrolyte) such as: electric energy required to build up the charged sphere(s) [8], electric field energy inside and outside the sphere(s) [8], polarization energy of the electrolyte ions [8] and electric interaction energy between the charged spheres [9].
In this work the density of the electric field energy inside and outside of two charged spheres are calculated at different locations. The density of the electric field energy at a point can be calculated by the following equation [10]:
where E is the vector of the electric field strength at the considered point, ϵ0 is the absolute vacuum permittivity and ϵr is the relative permittivity of the electrolyte.
Note that the Poisson-Boltzmann equation
By using the generalized Shell Theorem [6] we calculate the density of electric field energy, uF produced by two surface-charged spheres (see Figure 1) surrounded outside and inside by electrolyte. The surface of each sphere is homogeneously charged. The total charge of the smaller and larger sphere is Q1 and Q2, respectively.
In
In order to calculate the density of electric field energy one needs the electric field strength (see Eq 1), i.e. the gradient of the electric potential. The potential produced by the smaller sphere, V1 can be calculated by Eqs 9,10 in ref. [6] (see also Eqs A1, A2 in Appendix 1) and from the gradient of this potential the electric field strength created by the smaller sphere at point P1 (see Figure 1) is:
where
where λD is the Debye length (see
Similarly, the electric field strength created by the larger sphere at point P1 (see Figure 1) is:
where one can construct dV2/dD2 from Eq 3 by substituting D1 by D2, R1 by R2 and Q1 by Q2.
Finally, based on Eq 1 the density of the electric field energy at point P1 is:
Here by using
The connection point between the spheres of the same size is at xP = 0 and yP = 0 (see black dot in
The connection point between the spheres of different sizes is at xP = −0.5 R1 and yP = 0. At this point there is no electrolyte and
and
The density of the electric field energy depends on the electric field strength (Eq 1), i.e. the gradient of the electric potential. In the case of a single charged sphere surrounded by electrolyte with low ion concentration the potential inside the sphere is close to constant (see red curve in Figure 3A in ref. [6]) and thus the absolute value of the electric field strength is close to zero. On the other hand, outside the sphere the absolute value of the potential and also the electric field strength decrease with increasing distance from the surface of the sphere (see red curve in Figure 3A in ref. [6]). At higher electrolyte ion concentration, because of the increased screening effect, the absolute value of the potential and also the electric field strength decrease faster with increasing distance from the surface of the sphere. In this case inside the sphere toward its center the absolute value of the potential and the electric field strength also decrease (see curves in Figure 3A,B in ref. [6]).
These electric properties of a single charged sphere remain unchanged in the case of two charged spheres if the surfaces of the spheres are far enough from each other (farther than 4λD), i.e. the potential of one of the sphere decreases close to zero near the surface of the other sphere. However, when the two spheres are close enough to each other one sphere contributes to the potential and electric field strength around the other sphere. For example, let us consider two spheres of the same size located close to each other (see the left and right sphere in
At the midpoint between the two separated spheres of the same size (at xP = 0 and yP = 0) uF = 0 J m−3 because at the symmetry center the absolute value of electric field created by the left sphere is equal with the absolute value of electric field created by the right sphere, but their direction is opposite (thus
At xP = 0 and
The connection point between the spheres of the same size is at xP = 0 and yP = 0 (see black dot in Figure 2A). At this point there is no electrolyte and Eqs 2–5 are not applicable. The field energy densities approaching this connection point from left (i.e. xp → 0−; yp = 0) and from right (i.e. xp → 0+; yp = 0) are shown by the blue curves in Figures 3A–C. It is pointed out in Appendix 2 that, at any electrolyte ion concentration, in the case of R1 = R2 the density of the field energy is the same either approaching the connection point from the left or right (see Eqs A5, A7). However, if the right sphere is larger than the left sphere (i.e. R2 > R1) then uF near the right side of the connection point is larger than uF near the left side of the connection point (see Eqs A8, A9). Note, that the difference between these field energy densities decreases with decreasing electrolyte ion concentration and the difference becomes zero at zero electrolyte ion concentration (see Eqs A12, A13).
The analytical equation for the calculation of the electric field energy density of two charged spheres, Eq 5 is generalized for N charged spheres in Appendix 3.
In this paper two surface-charged spheres surrounded by electrolyte is considered. This system is close to the case of electrophoresis where charged cells surrounded by electrolyte interact with a charged flat surface
Based on the generalized version of Newton's Shell Theorem [6] analytical equation is derived to calculate the electric field energy density, uF around two separated surface-charged spheres surrounded by electrolyte. According to the calculations when the surfaces of the charged spheres are farther from each other than four times of the Debye length the field energy density around one of the charged sphere is basically independent from the presence of the other sphere. In this case at low electrolyte ion concentration uF = 0 within the spheres and outside the sphere uF decreases with increasing distance from the surface of the sphere, while at high electrolyte ion concentration uF fast decreases with increasing inner and outer distance from the surface of the sphere. When the charged spheres are close to each other their electric interaction affects the field energy density especially where the surfaces of the spheres are close to each other. Also, to model electrophoresis analytical equations are derived for the interaction energy between and the density of electric field energy around a charged flat surface and a surface-charged sphere surrounded by neutral electrolyte.
[1] |
Gabriel JL, Chong PLG (2000) Molecular modeling of archaebacterial bipolar tetraether lipid membranes. Chem Phys Lipids 105: 193-200. https://doi.org/10.1016/S0009-3084(00)00126-2 ![]() |
[2] |
Chong PLG (2010) Archaebacterial bipolar tetraether lipids: Physico-chemical and membrane properties. Chem Phys Lipids 163: 253-265. https://doi.org/10.1016/j.chemphyslip.2009.12.006 ![]() |
[3] |
Almeida PFF (2009) Thermodynamics of lipid interactions in complex bilayers. BBA-Biomembranes 1788: 72-85. https://doi.org/10.1016/j.bbamem.2008.08.007 ![]() |
[4] |
Sugár IP, Thompson TE, Biltonen RL (1999) Monte Carlo simulation of two-component bilayers: DMPC/DSPC mixtures. Biophys J 76: 2099-2110. https://doi.org/10.1016/S0006-3495(99)77366-2 ![]() |
[5] | Fetter AL, Walecka JD (2003) Theoretical Mechanics of Particles and Continua. New York: Dover Publications 307-310. |
[6] |
Sugár IP (2020) A generalization of the shell theorem. Electric potential of charged spheres and charged vesicles surrounded by electrolyte. AIMS Biophys 7: 76-89. https://doi.org/10.3934/biophy.2020007 ![]() |
[7] | Newton I (1999) The Principia: Mathematical Principles of Natural Philosophy. Berkeley: University of California Press. |
[8] |
Sugár IP (2021) Electric energies of a charged sphere surrounded by electrolyte. AIMS Biophys 8: 157-164. https://doi.org/10.3934/biophy.2021012 ![]() |
[9] | Sugár IP (2021) Interaction energy between two separated charged spheres surrounded inside and outside by electrolyte. arXiv:2103.13959 . |
[10] |
Griffiths DJ (2005) Introduction to electrodynamics. AM J Phys 73: 574. https://doi.org/10.1119/1.4766311 ![]() |
[11] |
Holtzer AM (1954) The collected papers of Peter JW Debye. Interscience, New York-London, 1954. xxi+ 700 pp., $9.50. J Polym Sci 13: 548-548. https://doi.org/10.1002/pol.1954.120137203 ![]() |
[12] |
Levin S, Levin M, Sharp AK, et al. (1983) Theory of electrokinetic behavior of human erythrocytes. Biophys J 42: 127-135. https://doi.org/10.1016/S0006-3495(83)84378-1 ![]() |
[13] | Korohoda W, Wilk A (2008) Cell Electrophoreses–a method for cell separation and research into cell surface properties. Cell Mol Biol Lett 18: 312-326. https://doi.org/10.2478/s11658-008-0004-y |
![]() |
![]() |