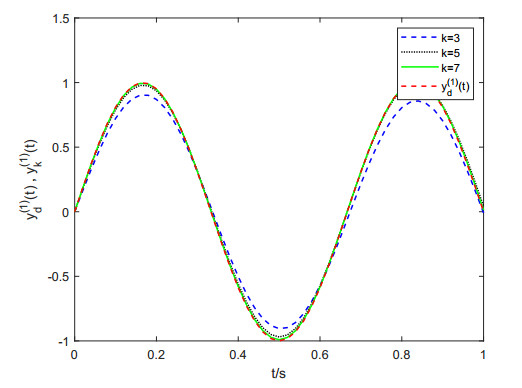
Citation: C. Dal Lin, M. Falanga, E. De Lauro, S. De Martino, G. Vitiello. Biochemical and biophysical mechanisms underlying the heart and the brain dialog[J]. AIMS Biophysics, 2021, 8(1): 1-33. doi: 10.3934/biophy.2021001
[1] | Haijun Wang, Jun Pan, Guiyao Ke . Multitudinous potential homoclinic and heteroclinic orbits seized. Electronic Research Archive, 2024, 32(2): 1003-1016. doi: 10.3934/era.2024049 |
[2] | Jun Pan, Haijun Wang, Feiyu Hu . Revealing asymmetric homoclinic and heteroclinic orbits. Electronic Research Archive, 2025, 33(3): 1337-1350. doi: 10.3934/era.2025061 |
[3] | Guowei Yu . Heteroclinic orbits between static classes of time periodic Tonelli Lagrangian systems. Electronic Research Archive, 2022, 30(6): 2283-2302. doi: 10.3934/era.2022116 |
[4] | Minzhi Wei . Existence of traveling waves in a delayed convecting shallow water fluid model. Electronic Research Archive, 2023, 31(11): 6803-6819. doi: 10.3934/era.2023343 |
[5] | Anna Gołȩbiewska, Marta Kowalczyk, Sławomir Rybicki, Piotr Stefaniak . Periodic solutions to symmetric Newtonian systems in neighborhoods of orbits of equilibria. Electronic Research Archive, 2022, 30(5): 1691-1707. doi: 10.3934/era.2022085 |
[6] | Yang Song, Beiyan Yang, Jimin Wang . Stability analysis and security control of nonlinear singular semi-Markov jump systems. Electronic Research Archive, 2025, 33(1): 1-25. doi: 10.3934/era.2025001 |
[7] | Xianjun Wang, Huaguang Gu, Bo Lu . Big homoclinic orbit bifurcation underlying post-inhibitory rebound spike and a novel threshold curve of a neuron. Electronic Research Archive, 2021, 29(5): 2987-3015. doi: 10.3934/era.2021023 |
[8] | Feng Wang, Taotao Li . Dynamics of a stochastic epidemic model with information intervention and vertical transmission. Electronic Research Archive, 2024, 32(6): 3700-3727. doi: 10.3934/era.2024168 |
[9] | Xiaochen Mao, Weijie Ding, Xiangyu Zhou, Song Wang, Xingyong Li . Complexity in time-delay networks of multiple interacting neural groups. Electronic Research Archive, 2021, 29(5): 2973-2985. doi: 10.3934/era.2021022 |
[10] | Yan Xie, Rui Zhu, Xiaolong Tan, Yuan Chai . Inhibition of absence seizures in a reduced corticothalamic circuit via closed-loop control. Electronic Research Archive, 2023, 31(5): 2651-2666. doi: 10.3934/era.2023134 |
Recently, fractional calculus emerged as a crucial tool for describing the dynamics of real-world problems [1,2,3]. Indeed, many fractional-order systems (FOSs) have been reported in the literature, focusing on stability, fault tolerant control, and sliding mode control, among other issues (see [4,5,6] and references therein). Iterative learning control (ILC) is an interesting approach to obtain trajectory tracking of repetitive systems operated over finite-time [7]. In recent years, FOSs and ILC have been merged with the goal of increasing tracking performance. In [8], a Dα-type ILC scheme was designed and its convergence was addressed. In [9,10,11], both the P- and D-type learning schemes were adopted in FOSs with Lipschitz nonlinearities. In [12], fractional-order PID learning control was proposed for linear FOSs, and output convergence was analyzed using the Lebesgue-p norm. In [13], the ILC framework was adopted for FOSs with randomly varying trial lengths. In [14,15], ILC problems of multi-agent systems with fractional-order models were investigated. Despite many relevant contributions, it should be pointed out that the above mentioned works mainly address linear and Lipschitz FOSs. Moreover, a variety of control strategies have been proposed for nonlinear systems (NSs) to achieve the desired performance. In [16,17], the convergence analysis for locally Lipschitz NSs was addessed based on the contraction mapping approach. In [18], adaptive optimal control was investigated for NSs based on the policy iteration algorithm. In [19], zero-sum control for tidal turbine systems was studied though a reinforcement learning method.
Compared with classical Lipschitz nonlinearity, one-sided Lipschitz (OSL) nonlinearity possesses less conservatism. Therefore, in recent years, is has often been used in control systems. Moreover, in many practical problems, the OSL constant is much smaller than the Lipschitz one, which simplifies the estimation of the influence of nonlinearities. OSL systems are a wide class of NSs, which contain Lipschitz systems as particular cases. Practical examples are Chua's circuits, Lorenz systems, and electromechanical systems [20,21,22]. In [23,24,25], observer design issues for OSL NSs were investigated. In [26], the classical OSL was considered, and an observer was designed by introducing the quadratically inner-bounded (QIB) constraint. In recent years, observer design and control of OSL NSs has attracted considerable attention. In [27], full- and reduced-order observers were derived via the Riccati equation. In [28], exponential observer design was investigated. In [29], tracking control for OSL nonlinear differential inclusions was considered. In [30], H∞ attenuation control was considered for OSL NSs in the finite frequency domain. In [31], event-triggered sliding mode control was studied for OSL NSs with uncertainties. In [32,33], consensus control was discussed for OSL nonlinear multi-agent systems. Other meaningful results on ILC of OSL NSs have also been reported [34,35,36]. In particular, the QIB constraint was employed to reach perfect trajectory tracking [34,35]. Note that the above-mentioned results are about classical integer-order systems. To the best of the authors' knowledge, for FOSs with OSL nonlinearity, the problem of how to achieve exact trajectory tracking through appropriate ILC design has not yet been investigated, which motivates the present study.
This paper deals with the ILC of a family of Caputo FOSs, where the fractional derivative is in the interval 0 and 1. The considered nonlinearity satisfies the OSL condition, which encompasses the classical Lipschitz condition. Open- and closed-loop P-type learning control algorithms are adopted. The convergence of the tracking error is guaranteed with the generalized Gronwall inequality. The novelty of this paper is summarized in the next two points.
● Unlike the control methods in references [18,19,29,30,31,32,33], the ILC method proposed in this paper can lead OSL nonlinear Caputo FOSs to exhibit perfect tracking capability;
● In contrast to the works of [34,35,36], the ILC theory is extended from integer-order OSL NSs to fractional-order OSL NSs.
This paper is divided into 5 sections. Section 2 establishes some elemental assumptions and formulates the ILC problem of fractional OSL NSs. Section 3 constructs the open- and closed-loop P-type control algorithms, and presents the corresponding convergence results. Section 4 includes a numerical example to show the suitability of the algorithms. Finally, Section 5 summarizes the conclusions.
Some relevant lemmas and definitions are introduced. Afterwards, the problem to be tackled is formulated.
Definition 1 [37]. The Riemann-Liouville integral of order α>0 of a function x(t) is
Iα0,tx(t)=1Γ(α)∫t0(t−ξ)α−1x(ξ)dξ,t∈[0,∞), |
where Γ(α) stands for the Gamma function.
Definition 2 [37]. The Caputo derivative of order 0<α<1 of a function x(t) is
CDα0,tx(t)=I1−α0,tddtx(t)=1Γ(1−α)∫t0(t−ξ)−α˙x(ξ)dξ,t∈[0,∞). |
Lemma 1 [38]. Consider the differentiable vector x(t)∈Rn. It follows that, for any time instant t≥0, we have
CDα0,t(xT(t)x(t))≤2xT(t)CDα0,tx(t),∀α∈(0,1), |
where the superscript T denotes the vector (or matrix) transpose.
Lemma 2. (Generalized Gronwall Inequality) [9] Consider that the function u(t) is continuous on the interval t∈[0,T], and let v(t−ξ) be nonnegative and continuous on 0≤ξ≤t≤T. Additionally, consider that the function w(t) is positive continuous and nondecreasing on t∈[0,T]. If
u(t)≤w(t)+∫t0v(t−ξ)u(ξ)dξ,t∈[0,T], |
then we have
u(t)≤w(t)e∫t0v(t−ξ)dξ,t∈[0,T]. |
To simplify the notation, in the following, we use Dα to refer to the Caputo derivative CDα0,t.
Let us consider the nonlinear FOS
{Dαxk(t)=Axk(t)+Buk(t)+f(xk(t)),yk(t)=Cxk(t)+Duk(t), | (2.1) |
where α∈(0,1), t∈[0,T], and k=0,1,2,⋅⋅⋅ is the repetition. Moreover, xk(t)∈Rn, uk(t)∈Rm, and yk(t)∈Rp represent the state, control, and output of (2.1), respectively; f(xk(t))∈Rn stands for a continuous nonlinear function; and A, B, C, and D are constant coefficients matrices.
Assumption 1. The nonlinear function f(⋅) is OSL, meaning that, for ∀x(t),ˆx(t)∈Rn,
⟨f(x(t))−f(ˆx(t)),x(t)−ˆx(t)⟩≤σ‖x(t)−ˆx(t)‖2, |
where ‖⋅‖ denotes the Euclidean norm, ⟨⋅,⋅⟩ represents the inner product, and σ∈R is the OSL constant.
Remark 1. Note that the above constant σ can assume any real value, while the Lipschitz constant is positive. From [26], a Lipschitz function is OSL (σ>0), but the converse may not hold.
Assumption 2. The desired trajectory yd(t) is possible, meaning that a control ud(t) exists, guaranteeing
{Dαxd(t)=Axd(t)+Bud(t)+f(xd(t)),yd(t)=Cxd(t)+Dud(t), |
with xd(t) being the desired state.
Assumption 3. The system defined by expression (2.1) meets the initial condition
xk(0)=xd(0),k=0,1,2,⋯, |
where xd(0) represents the desired initial state.
Remark 2. Assumption 2 is a representative condition for OSL Caputo FOSs in control law design. Assumption 3 is the identical initialization condition, which has been widely used in ILC design to obtain perfect tracking [7].
The main objective herein is to design a control sequence uk(t) so that the output yk(t) of (1) can track the specified trajectory yd(t), with t∈[0,T], as k→∞.
For the nonlinear FOS (2.1), we design an open-loop P-type learning control algorithm
uk+1(t)=uk(t)+Ψek(t), | (3.1) |
where the output tracking error at the kth iteration is defined as ek(t)=yd(t)−yk(t) and the learning gain matrix is Ψ∈Rm×p.
Theorem 1. Let us assume that Assumptions 1–3 hold for the FOS (2.1) with algorithm (3.1). If Ψ can be chosen such that
ρ1=‖I−DΨ‖<1, | (3.2) |
then yk(t) converges to yd(t) for t∈[0,T].
Proof. Let us use δ(⋅)k(t)=(⋅)k+1(t)−(⋅)k(t), where (⋅) stands for the variables x, u, and f. It follows from (2.1) and (3.1) that
Dα(δxk(t))=Aδxk(t)+Bδuk(t)+δfk(t)=Aδxk(t)+δfk(t)+BΨek(t). | (3.3) |
If we left-multiply (3.3) by (δxk(t))T and use Assumption 1, then we have
(δxk(t))TDα(δxk(t))=⟨Aδxk(t),δxk(t)⟩+⟨BΨek(t),δxk(t)⟩+⟨δfk(t),δxk(t)⟩≤(Aδxk(t))Tδxk(t)+(BΨek(t))Tδxk(t)+σ‖δxk(t)‖2≤(‖A‖+|σ|)‖δxk(t)‖2+‖BΨ‖‖δxk(t)‖‖ek(t)‖. | (3.4) |
According to Lemma 1,
Dα((δxk(t))Tδxk(t))≤2(δxk(t))TDα(δxk(t)). | (3.5) |
From (3.4) and (3.5), we get
Dα(‖δxk(t)‖2)≤2(‖A‖+|σ|)‖δxk(t)‖2+2‖BΨ‖‖δxk(t)‖‖ek(t)‖≤(2‖A‖+2|σ|+1)‖δxk(t)‖2+‖BΨ‖2‖ek(t)‖2=c1‖δxk(t)‖2+c2‖ek(t)‖2, | (3.6) |
where c1=2‖A‖+2|σ|+1 and c2=‖BΨ‖2. Applying the α-order integral on (3.6), we get
Iα0,tDα(‖δxk(t)‖2)≤Iα0,t(c1‖δxk(t)‖2+c2‖ek(t)‖2). | (3.7) |
It follows from Assumption 3 that ‖δxk(0)‖2=0, and we further get
Iα0,tDα(‖δxk(t)‖2)=Iα0,tI1−α0,tddt(‖δxk(t)‖2)=I10,tddt(‖δxk(t)‖2)=‖δxk(t)‖2−‖δxk(0)‖2=‖δxk(t)‖2, |
which, together with (3.7), leads to
‖δxk(t)‖2≤Iα0,t(c1‖δxk(t)‖2+c2‖ek(t)‖2)=c1Γ(α)∫t0(t−ξ)α−1‖δxk(ξ)‖2dξ+c2Γ(α)∫t0(t−ξ)α−1‖ek(ξ)‖2dξ=c1Γ(α)∫t0(t−ξ)α−1‖δxk(ξ)‖2dξ+c2Γ(α)∫t0(t−ξ)α−1e2λξ{e−2λξ‖ek(ξ)‖2}dξ≤c1Γ(α)∫t0(t−ξ)α−1‖δxk(ξ)‖2dξ+c2Γ(α)∫t0(t−ξ)α−1e2λξdξ‖ek‖2λ. | (3.8) |
We can see that
∫t0(t−ξ)α−1e2λξdξt−ξ=τ→∫t0τα−1e2λ(t−τ)dτ=e2λt∫t0τα−1e−2λτdτ2λτ=ξ→e2λt(2λ)α∫2λt0ξα−1e−ξdξ<e2λt(2λ)α∫+∞0ξα−1e−ξdξ=e2λt(2λ)αΓ(α). | (3.9) |
From (3.8) and (3.9), we have
‖δxk(t)‖2≤c1Γ(α)∫t0(t−ξ)α−1‖δxk(ξ)‖2dξ+c2e2λt(2λ)α‖ek‖2λ. |
Setting
v(t−ξ)=c1Γ(α)(t−ξ)α−1,w(t)=c2e2λt(2λ)α‖ek‖2λ, |
and using Lemma 2, we get
‖δxk(t)‖2≤c2e2λt(2λ)αec1Γ(α)∫t0(t−ξ)α−1dξ‖ek‖2λ=c2e2λt(2λ)αec1Γ(α)tαα‖ek‖2λ≤c2e2λt(2λ)αec1TαΓ(α+1)‖ek‖2λ. |
Multiplying the above inequality by e−2λt, and using the λ-norm ‖⋅‖λ, we have
‖δxk‖2λ≤c2ec1TαΓ(α+1)(2λ)α‖ek‖2λ, |
where ‖⋅‖λ=supt∈[0,T]{e−λt‖⋅‖}.
Therefore, we get
‖δxk‖λ≤c3√λα‖ek‖λ, | (3.10) |
where
c3=√c2ec1TαΓ(α+1)2α. |
It is obvious that
ek+1(t)=ek(t)−Cδxk(t)−Dδuk(t)=(I−DΨ)ek(t)−Cδxk(t). | (3.11) |
It follows from (3.2), (3.10), and (3.11) that
‖ek+1‖λ≤‖I−DΨ‖‖ek‖λ+‖C‖‖δxk‖λ≤ρ1‖ek‖λ+‖C‖‖δxk‖λ≤ρ1‖ek‖λ+c3‖C‖√λα‖ek‖λ=ˆρ1‖ek‖λ, | (3.12) |
where
ˆρ1=ρ1+c3‖C‖√λα. |
As 0≤ρ1<1 by (3.2), we can select λ as large as needed so that ˆρ1<1. Thus, we obtain
limk→∞‖ek‖λ=0. |
Note that ‖ek‖s≤eλT‖ek‖λ, with ‖⋅‖s=supt∈[0,T]‖⋅‖ denoting the supremum norm. Therefore, limk→∞‖ek‖s=0, meaning that
limk→∞yk(t)=yd(t),t∈[0,T]. |
This ends the proof.
Now, we design a closed-loop P-type learning control algorithm, such that
uk+1(t)=uk(t)+Φek+1(t), | (3.13) |
where the learning gain is Φ∈Rm×p.
Theorem 2. Consider that Assumptions 1–3 hold for the FOS (2.1) with the learning algorithm (3.13). If the gain Φ can be chosen such that
ρ2=‖(I+DΦ)−1‖<1, | (3.14) |
then yk(t) converges to yd(t) for t∈[0,T].
Proof. From (2.1) and (3.13), we get
Dα(δxk(t))=Aδxk(t)+Bδuk(t)+δfk(t)=Aδxk(t)+δfk(t)+BΦek+1(t). | (3.15) |
Left multiplying (3.15) by (δxk(t))T and considering Assumption 1, we obtain
(δxk(t))TDα(δxk(t))=⟨Aδxk(t),δxk(t)⟩+⟨BΦek+1(t),δxk(t)⟩+⟨δfk(t),δxk(t)⟩≤(Aδxk(t))Tδxk(t)+(BΦek+1(t))Tδxk(t)+σ‖δxk(t)‖2≤(‖A‖+|σ|)‖δxk(t)‖2+‖BΦ‖‖δxk(t)‖‖ek+1(t)‖. | (3.16) |
Obviously, (3.16) together with (3.5) implies
Dα(‖δxk(t)‖2)≤2(‖A‖+|σ|)‖δxk(t)‖2+2‖BΦ‖‖δxk(t)‖‖ek+1(t)‖≤(2‖A‖+2|σ|+1)‖δxk(t)‖2+‖BΨ‖2‖ek+1(t)‖2=c1‖δxk(t)‖2+c4‖ek+1(t)‖2, | (3.17) |
where c4=‖BΦ‖2. Similarly to the procedure adopted in Theorem 1, we get
‖δxk‖λ≤c5√λα‖ek+1‖λ, | (3.18) |
where
c5=√c4ec1TαΓ(α+1)2α. |
From expressions (2.1) and (3.13), we have
ek+1(t)=ek(t)−Cδxk(t)−Dδuk(t)=ek(t)−Cδxk(t)−DΦek+1(t), |
that is
(I+DΦ)ek+1(t)=ek(t)−Cδxk(t), |
where the symbol I stands for the identity matrix. Since I+DΦ is nonsingular, we get
ek+1(t)=(I+DΦ)−1(ek(t)−Cδxk(t)). |
Furthermore, we derive
‖ek+1‖λ≤‖(I+DΦ)−1‖‖ek‖λ+‖(I+DΦ)−1C‖‖δxk‖λ≤ρ2‖ek‖λ+‖(I+DΦ)−1C‖‖δxk‖λ. | (3.19) |
Substituting (3.18) into (3.19) yields
‖ek+1‖λ≤ρ2‖ek‖λ+c5√λα‖(I+DΦ)−1C‖‖ek+1‖λ. |
Taking λ such that
c5√λα‖(I+DΦ)−1C‖<1, |
then we have
‖ek+1‖λ≤ˆρ2‖ek‖λ, | (3.20) |
where
ˆρ2=ρ21−c5√λα‖(I+DΦ)−1C‖. |
As 0≤ρ2<1, we can choose λ as large as needed so that ˆρ2<1. From expression (3.20), we can obtain
limk→∞‖ek‖λ=0. |
As ‖ek‖s≤eλT‖ek‖λ, we know that limk→∞‖ek‖s=0, and it follows that
limk→∞yk(t)=yd(t),t∈[0,T]. |
This completes the proof.
We illustrate the applicability of the P-type learning algorithms by means of a practical example.
Let us choose the following nonlinear FOS, which can be used to describe the motion of a moving object in Cartesian coordinates [39]
{D0.5xk(t)=Axk(t)+Buk(t)+f(xk(t)),yk(t)=Cxk(t)+Duk(t), |
where xk(t)=[x1k(t)x2k(t)]T, with t∈[0,1],
A=[1−2−11],B=[1021],C=[1002],D=[1001], |
f(xk(t))=[−x1k(t)(x21k(t)+x22k(t))−x2k(t)(x21k(t)+x22k(t))]. |
We know from [26] that the nonlinear function f(⋅) is globally OSL with σ=0 in R2. Let us use
yd(t)=[sin(3πt)te−0.1t], |
and consider
xk(0)=[00],u0(t)=[00]. |
i) Open-loop algorithm (3.1).
Using the gain matrix
Ψ=[0.5000.5], |
we then have
ρ1=‖I−DΨ‖=0.5<1. |
Figures 1 and 2 depict the desired trajectories y(1)d(t) and y(2)d(t), and the outputs y(1)k(t) and y(2)k(t), respectively, at the 3rd, 5th, and 7th iterations, obtained with the learning algorithm (3.1). Figure 3 represents the errors, showing that perfect tracking is reached as the number of iterations increases.
ii) Closed-loop algorithm (3.13).
Using the gain matrix
Φ=[1001], |
then we have
ρ2=‖(I+DΦ)−1‖=0.5<1, |
meaning that the convergence is verified. Figures 4 and 5 illustrate that y(1)k(t) and y(2)k(t) follow the desired trajectories from the 6th iteration. Figure 6 shows that the error converges under algorithm (3.13).
We also verify that, at the 10th iteration, ||e(i)k||s(i=1,2) {achieves} [1.4×10−3, 5.7×10−3] and [0.5×10−3, 1.4×10−3] when using algorithms (3.1) and (3.13), respectively. This confirms the results observed in Figures 3 and 6, meaning that algorithm (3.13) performs better than (3.1) in terms of convergence speed.
The ILC for a class of Caputo FOSs with OSL nonlinearity was investigated. Open- and closed-loop P-type learning algorithms were designed to guarantee perfect tracking of a desired trajectory, and their convergence was verified using the generalized Gronwall inequality. An example was provided to verify the theoretical results. It should be noted that the QIB constraint was used in the framework of ILC for OSL NSs with irregular dynamics [34,35]. To some extent, this limits the applicability of NSs due to their need to simultaneously satisfy the OSL and QIB constraints. Therefore, the ILC for irregular OSL nonlinear FOSs needs to be further investigated by relaxing or removing the QIB constraint.
The authors declare they have not used Artificial Intelligence (AI) tools in the creation of this article.
This work was supported by the National Natural Science Foundation of China (Nos. 62073114 and 11971032) and Anhui Provincial Key Research and Development Project (202304a05020060).
The authors declare there is no conflict of interest.
[1] |
Kocica MJ, Corno AF, Carreras-Costa F, et al. (2006) The helical ventricular myocardial band: global, three-dimensional, functional architecture of the ventricular myocardium. Eur J Cardiothorac Surg 29: S21-S40. doi: 10.1016/j.ejcts.2006.03.011
![]() |
[2] |
Dal Lin C, Tona F, Osto E (2018) The heart as a psychoneuroendocrine and immunoregulatory organ. Sex-Specific Analysis of Cardiovascular Function 1065: 225-239. doi: 10.1007/978-3-319-77932-4_15
![]() |
[3] | Marinelli RA, Penney DG, Marinelli W, et al. (1991) Rotary motion in the heart and blood vessels: a review. J Appl Cardiol 6: 421-431. |
[4] |
Sengupta PP, Narula J, Chandrashekhar Y (2014) The dynamic vortex of a beating heart: Wring out the old and ring in the new!. J Am Coll Cardiol 64: 1722-1724. doi: 10.1016/j.jacc.2014.07.975
![]() |
[5] | Bottio T, Buratto E, Dal Lin C, et al. (2012) Aortic valve hydrodynamics: considerations on the absence of sinuses of Valsalva. J Heart Valve Dis 21: 718-723. |
[6] | Calgary University Medicine: Blood Moving Through the Heart - 4D Flow Available from: https://www.youtube.com/watch?v=sMeaD3Jh64E. |
[7] |
Dal Lin C, Marinova M, Rubino G, et al. (2018) Thoughts modulate the expression of inflammatory genes and may improve the coronary blood flow in patients after a myocardial infarction. J Tradit Complement Med 8: 150-163. doi: 10.1016/j.jtcme.2017.04.011
![]() |
[8] |
Armour JA (2007) The little brain on the heart. Cleve Clin J Med 74: S48-S51. doi: 10.3949/ccjm.74.Suppl_1.S48
![]() |
[9] | Lane RD, Reiman EM, Ahern GL, et al. (1982) Activity in medial prefrontal cortex correlates with vagal component of heart rate variability during emotion. Brain Cognition 47: 97-100. |
[10] |
Jennings JR, Sheu LK, Kuan DCH, et al. (2016) Resting state connectivity of the medial prefrontal cortex covaries with individual differences in high-frequency heart rate variability. Psychophysiology 53: 444-454. doi: 10.1111/psyp.12586
![]() |
[11] |
Schandry R, Montoya P (1996) Event-related brain potentials and the processing of cardiac activity. Biol Psychol 42: 75-85. doi: 10.1016/0301-0511(95)05147-3
![]() |
[12] |
Garfinkel SN, Barrett AB, Minati L, et al. (2013) What the heart forgets: Cardiac timing influences memory for words and is modulated by metacognition and interoceptive sensitivity. Psychophysiology 50: 505-512. doi: 10.1111/psyp.12039
![]() |
[13] |
Azevedo RT, Garfinkel SN, Critchley HD, et al. (2017) Cardiac afferent activity modulates the expression of racial stereotypes. Nat Commun 8: 13854. doi: 10.1038/ncomms13854
![]() |
[14] |
Garfinkel SN, Minati L, Gray MA, et al. (2014) Fear from the heart: Sensitivity to fear stimuli depends on individual heartbeats. J Neurosci 34: 6573-6582. doi: 10.1523/JNEUROSCI.3507-13.2014
![]() |
[15] |
Montoya P, Schandry R, Müller A (1993) Heartbeat evoked potentials (HEP): topography and influence of cardiac awareness and focus of attention. Electroencephalogr Clin Neurophysiol Evoked Potentials 88: 163-172. doi: 10.1016/0168-5597(93)90001-6
![]() |
[16] |
Thayer JF, Lane RD (2000) A model of neurovisceral integration in emotion regulation and dysregulation. J Affect Disord 61: 201-216. doi: 10.1016/S0165-0327(00)00338-4
![]() |
[17] |
Park G, Thayer JF (2014) From the heart to the mind: cardiac vagal tone modulates top-down and bottom-up visual perception and attention to emotional stimuli. Front Psychol 5: 278. doi: 10.3389/fpsyg.2014.00278
![]() |
[18] |
Thayer JF, Hansen AL, Saus-Rose E, et al. (2009) Heart rate variability, prefrontal neural function, and cognitive performance: the neurovisceral integration perspective on self-regulation, adaptation, and health. Ann Behav Med 37: 141-153. doi: 10.1007/s12160-009-9101-z
![]() |
[19] |
Thayer JF, Sternberg E (2006) Beyond heart rate variability: vagal regulation of allostatic systems. Ann N Y Acad Sci 1088: 361-372. doi: 10.1196/annals.1366.014
![]() |
[20] |
Lin PF, Lo MT, Tsao J, et al. (2014) Correlations between the signal complexity of cerebral and cardiac electrical activity: a multiscale entropy analysis. PLoS One 9: e87798. doi: 10.1371/journal.pone.0087798
![]() |
[21] | Aftanas LI, Brak IV, Reva NV, et al. (2013) Brain oscillations and individual variability of cardiac defense in human. Ross Fiziol Zh Im IM Sechenova 99: 1342-1356. |
[22] |
McCraty R, Atkinson M, Bradley RT (2004) Electrophysiological evidence of intuition: Part 2. A system-wide process? J Altern Complement Med 10: 325-336. doi: 10.1089/107555304323062310
![]() |
[23] |
Gray MA, Beacher FD, Minati L, et al. (2012) Emotional appraisal is influenced by cardiac afferent information. Emotion 12: 180-191. doi: 10.1037/a0025083
![]() |
[24] |
Craig ADB (2009) How do you feel—now? The anterior insula and human awareness. Nat Rev Neurosci 10: 59-70. doi: 10.1038/nrn2555
![]() |
[25] |
Craig ADB (2014) How do you feel? An interoceptive moment with your neurobiological self Princeton: Princeton University Press. doi: 10.23943/princeton/9780691156767.001.0001
![]() |
[26] |
Grossmann I, Sahdra BK, Ciarrochi J, et al. (2016) Heart and a mind: Self-distancing facilitates the association between heart rate variability, and wise reasoning. Front Behav Neurosci 10: 68. doi: 10.3389/fnbeh.2016.00068
![]() |
[27] | Rahman SU, Hassan M (2013) Heart's role in the human body: A literature review. ICCSS 2: 1-6. |
[28] | McCraty R, Trevor Bradley R, Tomasino D (2004) The resonant heart. Front Counsciousness 5: 15-19. |
[29] | McCraty R, Atkinson M, Tomasino D, et al. (2009) The coherent heart: Heart-brain interactions, psychophysiological coherence, and the emergence of system-wide order. Integr Rev 5: 10-115. |
[30] |
Goldstein DS (2012) Neurocardiology: therapeutic implications for cardiovascular disease. Cardiovasc Ther 30: 89-106. doi: 10.1111/j.1755-5922.2010.00244.x
![]() |
[31] | Dal Lin C, Poretto A, Scodro M, et al. (2015) Coronary microvascular and endothelial function regulation: Crossroads of psychoneuroendocrine immunitary signals and quantum physics. J Integr Cardiol 1: 132-163. |
[32] |
Dal Lin C, Tona F, Osto E (2019) The crosstalk between the cardiovascular and the immune system. Vasc Biol 1: H83-H88. doi: 10.1530/VB-19-0023
![]() |
[33] |
Dal Lin C, Tona F, Osto E (2015) Coronary microvascular function and beyond: The crosstalk between hormones, cytokines, and neurotransmitters. Int J Endocrinol 2015: 1-17. doi: 10.1155/2015/312848
![]() |
[34] | Lashley KS (1942) The problem of cerebral organization in vision. Visual Mechanisms. Biological Symposia Lancaster: Jaques Cattell Press, 301-322. |
[35] | Pribram KH (1991) Brain and Perception Hillsdale: Lawrence Erlbaum. |
[36] | Freeman WJ (1975) Mass Action in the Nervous System New York: Academic Press. |
[37] |
Freeman WJ (2000) Neurodynamics: An Exploration of Mesoscopic Brain Dynamics Berlin: Springer. doi: 10.1007/978-1-4471-0371-4
![]() |
[38] |
Ricciardi LM, Umezawa H (1967) Brain and physics of many-body problems. Kybernetik 4: 44-48. doi: 10.1007/BF00292170
![]() |
[39] |
Goldstone J, Salam A, Weinberg S (1962) Broken Symmetries. Phys Rev 127: 965-970. doi: 10.1103/PhysRev.127.965
![]() |
[40] | Umezawa H (1995) Advanced field theory: Micro, macro, and thermal physics New York: AIP. |
[41] |
Blasone M, Jizba P, Vitiello G (2011) Quantum Field Theory and its macroscopic manifestations: Boson condensation, ordered patterns, and topological defects London: Imperial College Press. doi: 10.1142/p592
![]() |
[42] |
Jibu M, Yasue K (1995) Quantum brain dynamics and consciousness Amsterdam: John Benjamins Publ. doi: 10.1075/aicr.3
![]() |
[43] | Umezawa H (1995) Development in concepts in quantum field theory in half century. Math Jpn 41: 109-124. |
[44] |
Vitiello G (1995) Dissipation and memory capacity in the quantum brain model. Int J Mod Phys B 9: 973-989. doi: 10.1142/S0217979295000380
![]() |
[45] |
Vitiello G (2001) My double unveiled Amsterdam: John Benjamins Publ. doi: 10.1075/aicr.32
![]() |
[46] |
Freeman WJ, Vitiello G (2006) Nonlinear brain dynamics as macroscopic manifestation of underlying many-body dynamics. Phys Life Rev 3: 93-118. doi: 10.1016/j.plrev.2006.02.001
![]() |
[47] |
Freeman WJ, Livi R, Obinata M (2012) Cortical phase transitions, non-equilibrium thermodynamics and the time dependent Ginzburg-Landau equation. Int J Mod Phys B 26: 1250035. doi: 10.1142/S021797921250035X
![]() |
[48] | Alfinito E, Vitiello G (2000) Formation and lifetime of memory domains in the dissipative quantum model of brain. Int J Mod Phys B 14: 853-868. |
[49] |
Vitiello G (2012) Fractals, coherent states and self-similarity induced noncommutative geometry. Phys Lett A 376: 2527-2532. doi: 10.1016/j.physleta.2012.06.035
![]() |
[50] | Freeman W, Vitiello G (2016) Matter and mind are entangled in two streams of images guiding behavior and informing the subject through awareness. Mind Matter 14: 7-24. |
[51] |
Del Giudice E, Doglia S, Milani M, et al. (1985) A quantum field theoretical approach to the collective behavior of biological systems. Nucl Phys B 251: 375-400. doi: 10.1016/0550-3213(85)90267-6
![]() |
[52] |
Del Giudice E, Doglia S, Milani M, et al. (1986) Electromagnetic field and spontaneous symmetry breakdown in biological matter. Nucl Phys B 275: 185-199. doi: 10.1016/0550-3213(86)90595-X
![]() |
[53] |
Del Giudice E, Preparata G, Vitiello G (1988) Water as a free electric dipole laser. Phys Rev Lett 61: 1085-1088. doi: 10.1103/PhysRevLett.61.1085
![]() |
[54] |
Engel GS, Calhoun TR, Read EL, et al. (2007) Evidence for wavelike energy transfer through quantum coherence in photosynthetic systems. Nature 446: 782-786. doi: 10.1038/nature05678
![]() |
[55] |
Peacock JA (1990) An in vitro study of the onset of turbulence in the sinus of valsalva. Circ Res 67: 448-460. doi: 10.1161/01.RES.67.2.448
![]() |
[56] | Mettauer B, Levy F, Richard R, et al. (2005) Exercising with a denervated heart after cardiac transplantation. Ann Transplant 10: 35-42. |
[57] | Armour JA, Ardell JL (2004) Basic and Clinical Neurocardiology Oxford: Oxford University Press. |
[58] |
Biasetti J, Hussain F, Gasser TC (2011) Blood flow and coherent vortices in the normal and aneurysmatic aortas: a fluid dynamical approach to intra-luminal thrombus formation. J R Soc Interface 8: 1449-1461. doi: 10.1098/rsif.2011.0041
![]() |
[59] |
Matsumoto H, Papastamatiou NJ, Umezawa H, et al. (1975) Dynamical rearrangement in the Anderson-Higgs-Kibble mechanism. Nucl Phys B 97: 61-89. doi: 10.1016/0550-3213(75)90215-1
![]() |
[60] |
Matsumoto H, Papastamatiou NJ, Umezawa H (1975) The boson transformation and the vortex solution. Nucl Phys B 97: 90-124. doi: 10.1016/0550-3213(75)90216-3
![]() |
[61] |
Manka R, Vitiello G (1990) Topological solitons and temperature effects in gauge field theory. Ann Phys 199: 61-83. doi: 10.1016/0003-4916(90)90368-X
![]() |
[62] |
Vitiello G (2000) Defect formation through boson condensation in quantum field theory. Topological Defects and the Non-Equilibrium Dynamics of Symmetry Breaking Phase Transitions Dordrecht: Springer, 171-191. doi: 10.1007/978-94-011-4106-2_9
![]() |
[63] |
Meyer G, Vitiello G (2018) On the molecular dynamics in the hurricane interactions with its environment. Phys Lett A 382: 1441-1448. doi: 10.1016/j.physleta.2018.03.044
![]() |
[64] |
Da Silva AF, Carpenter T, How TV, et al. (1997) Stable vortices within vein cuffs inhibit anastomotic myointimal hyperplasia? Eur J Vasc Endovasc Surg 14: 157-163. doi: 10.1016/S1078-5884(97)80185-2
![]() |
[65] |
Kefayati S, Amans M, Faraji F, et al. (2017) The manifestation of vortical and secondary flow in the cerebral venous outflow tract: An in vivo MR velocimetry study. J Biomech 50: 80-187. doi: 10.1016/j.jbiomech.2016.11.041
![]() |
[66] |
Lurie F, Kistner RL, Eklof B, et al. (2003) Mechanism of venous valve closure and role of the valve in circulation: a new concept. J Vasc Surg 38: 955-961. doi: 10.1016/S0741-5214(03)00711-0
![]() |
[67] | Boisseau MR (1997) Venous valves in the legs: hemodynamic and biological problems and relationship to physiopathology. J Mal Vasc 22: 122-127. |
[68] |
Machi J, Sigel B, Ramos JR, et al. (1986) Sonographic evaluation of platelet aggregate retention in a vortex within a simulated venous sinus. J Ultrasound Med 5: 685-689. doi: 10.7863/jum.1986.5.12.685
![]() |
[69] |
Meyer G, Vitiello G (2019) On the hurricane collective molecular dynamics. J Phys Conf Ser 1275: 012017. doi: 10.1088/1742-6596/1275/1/012017
![]() |
[70] |
Higgs PW (1966) Spontaneous symmetry breakdown without massless bosons. Phys Rev 145: 1156-1163. doi: 10.1103/PhysRev.145.1156
![]() |
[71] |
Freeman W, Vitiello G (2010) Vortices in brain waves. Int J Mod Phys B 24: 3269-3295. doi: 10.1142/S0217979210056025
![]() |
[72] | Del Giudice E, Vitiello G (2006) The role of the electromagnetic field in the formation of domains in the process of symmetry breaking phase transitions. Phys Rev A 74: 02210. |
[73] | De Lauro E, De Martino S (2019) On the heart vibrations: Some insights from ECG and laser doppler vibrometry.p. atticon11705 V-C-5in press. |
[74] | Scalise L (2012) Non contact heart monitoring. Advances in Electrocardiograms-Methods and Analysis Rijeka, Croatia: InTech, 81-106. |
[75] | Tomasini E, Pinotti M, Paone N (1998) Carotid artery pulse wave measured by a laser vibrometer.3411: 611-616. |
[76] |
Morbiducci U, Scalise L, De Melis M (2006) Optical vibrocardiography: a novel tool for optical monitoring of cardiac activity. Ann Biomed Eng 35: 45-58. doi: 10.1007/s10439-006-9202-9
![]() |
[77] |
Buccheri G, De Lauro E, De Martino S, et al. (2016) Experimental study of self-oscillations of the trachea–larynx tract by laser doppler vibrometry. Biomed Phys Eng Express 2: 055009. doi: 10.1088/2057-1976/2/5/055009
![]() |
[78] | Tavakolian K, Dumont GA, Blaber AP (2012) Analysis of seismocardiogram capability for trending stroke volume changes: A lower body negative pressure study. Computing in Cardiology 2012: 733-736. |
[79] | Desjardins CL, Antonelli LT (2007) A remote and non-contact method for obtaining the blood pulse waveform with a laser Doppler vibrometer. Advanced Biomedical and Clinical Diagnostic Systems V, Proc. SPIE . |
[80] |
Hyvärinen A, Karhunen J, Oja E (2001) Independent Component Analysis Hoboken, USA: John Wiley & Sons. doi: 10.1002/0471221317
![]() |
[81] |
Capuano P, De Lauro E, De Martino S, et al. (2017) Convolutive independent component analysis for processing massive datasets: a case study at Campi Flegrei (Italy). Nat Hazards 86: 417-429. doi: 10.1007/s11069-016-2545-0
![]() |
[82] |
Capuano P, De Lauro E, De Martino S (2016) Detailed investigation of long-period activity at Campi Flegrei by convolutive independent component analysis. Phys Earth Planet Inter 253: 48-57. doi: 10.1016/j.pepi.2016.02.003
![]() |
[83] |
Capuano P, De Lauro E, De Martino S (2011) Water-level oscillations in the Adriatic Sea as coherent self-oscillations inferred by independent component analysis. Prog Oceanogr 91: 447-460. doi: 10.1016/j.pocean.2011.06.001
![]() |
[84] |
De Lauro E, De Martino S, Falanga M, et al. (2006) Statistical analysis of Stromboli VLP tremor in the band [0.1–0.5] Hz: some consequences for vibrating structures. Nonlinear Process Geophys 13: 393-400. doi: 10.5194/npg-13-393-2006
![]() |
[85] |
Rüssel IK, Götte MJW, Bronzwaer JG, et al. (2009) Left ventricular torsion: an expanding role in the analysis of myocardial dysfunction. JACC Cardiovasc Imaging 2: 648-655. doi: 10.1016/j.jcmg.2009.03.001
![]() |
[86] |
Loppini A, Capolupo A, Cherubini C (2012) On the coherent behavior of pancreatic beta cell clusters. Phys Lett A 378: 3210-3217. doi: 10.1016/j.physleta.2014.09.041
![]() |
[87] |
Dal Lin C, Brugnolo L, Marinova M, et al. (2020) Toward a unified view of cognitive and biochemical activity: Meditation and linguistic self-reconstructing may lead to inflammation and oxidative stress improvement. Entropy 22: 818. doi: 10.3390/e22080818
![]() |
[88] | Dal Lin C, Radu CM, Vitiello G, et al. (2020) In vitro effects on cellular shaping, contratility, cytoskeletal organization and mitochondrial activity in HL1 cells after different sounds stimulation. A qualitative pilot study and a theoretical physical model, 2020. |
[89] | McFadden J, Al-Khalili J (2014) Life on the edge: the coming of age of quantum biology London: Bantam Press. |
[90] |
McFadden J, Al-Khalili J (1999) A quantum mechanical model of adaptive mutation. Biosystems 50: 203-211. doi: 10.1016/S0303-2647(99)00004-0
![]() |
[91] |
Misra B, Sudarshan ECG (1977) The Zeno's paradox in quantum theory. J Math Phys 18: 756-763. doi: 10.1063/1.523304
![]() |
[92] |
Kraus K (1981) Measuring processes in quantum mechanics I. Continuous observation and the watchdog effect. Found Phys 11: 547-576. doi: 10.1007/BF00726936
![]() |
[93] |
Meloni M (2014) The social brain meets the reactive genome: neuroscience, epigenetics and the new social biology. Front Hum Neurosci 8: 309. doi: 10.3389/fnhum.2014.00309
![]() |
[94] |
Vitiello G (2014) On the isomorphism between dissipative systems, fractal self-similarity and electrodynamics. Toward an integrated vision of nature. Systems 2: 203-216. doi: 10.3390/systems2020203
![]() |
[95] |
Novack DH, Cameron O, Epel E, et al. (2007) Psychosomatic medicine: The scientific foundation of the biopsychosocial model. Acad Psychiatry 31: 388-401. doi: 10.1176/appi.ap.31.5.388
![]() |
[96] |
Dal Lin C, Gola E, Brocca A, et al. (2018) miRNAs may change rapidly with thoughts: The relaxation response after myocardial infarction. Eur J Integr Med 20: 63-72. doi: 10.1016/j.eujim.2018.03.009
![]() |
[97] |
Chrousos GP (2009) Stress and disorders of the stress system. Nat Rev Endocrinol 5: 374-381. doi: 10.1038/nrendo.2009.106
![]() |
[98] |
Muehsam D, Ventura C (2014) Life rhythm as a symphony of oscillatory patterns: electromagnetic energy and sound vibration modulates gene expression for biological signaling and healing. Glob Adv Health Med 3: 40-55. doi: 10.7453/gahmj.2014.008
![]() |
[99] | (2015) Heart Beat Made Visible on CymaScope Avaibale from: https://www.youtube.com/watch?v=2kuY98F7o_0. |
[100] |
Ingber DE, Wang N, Stamenović D (2014) Tensegrity, cellular biophysics, and the mechanics of living systems. Rep Prog Phys 77: 046603. doi: 10.1088/0034-4885/77/4/046603
![]() |
[101] |
Wang N, Tytell JD, Ingber DE (2009) Mechanotransduction at a distance: Mechanically coupling the extracellular matrix with the nucleus. Nat Rev Mol Cell Biol 10: 75-82. doi: 10.1038/nrm2594
![]() |
[102] |
Martino F, Perestrelo AR, Vinarsky V, et al. (2018) Cellular mechanotransduction: from tension to function. Frony Physiol 9: 824. doi: 10.3389/fphys.2018.00824
![]() |
[103] |
Buxbaum O (2016) Key Insights into Basic Mechanisms of Mental Activity Switzerland: Springer International Publishing. doi: 10.1007/978-3-319-29467-4
![]() |
[104] |
Jamieson JP, Crum AJ, Goyer JP, et al. (2018) Optimizing stress responses with reappraisal and mindset interventions: an integrated model. Anxiety, Stress, Coping 31: 245-261. doi: 10.1080/10615806.2018.1442615
![]() |
[105] |
Pulvermüller F (2013) How neurons make meaning: Brain mechanisms for embodied and abstract-symbolic semantics. Trends Cogn Sci 17: 458-470. doi: 10.1016/j.tics.2013.06.004
![]() |
[106] |
Segall JM, Allen EA, Jung RE, et al. (2012) Correspondence between structure and function in the human brain at rest. Front Neuroinform 6: 10. doi: 10.3389/fninf.2012.00010
![]() |
[107] |
Alexander-Bloch A, Shou H, Liu S, et al. (2018) On testing for spatial correspondence between maps of human brain structure and function. Neuroimage 178: 540-551. doi: 10.1016/j.neuroimage.2018.05.070
![]() |
[108] |
Rebollo I, Devauchelle AD, Béranger B, et al. (2018) Stomach-brain synchrony reveals a novel, delayed-connectivity resting-state network in humans. Elife 7: e33321. doi: 10.7554/eLife.33321
![]() |
[109] | Ventura C (2017) Seeing cell biology with the eyes of physics. NanoWorld J 3: S1-S8. |
[110] | Fredericks S, Saylor JR (2013) Shape oscillation of a levitated drop in an acoustic field, 2013 Available from: arXiv:1310.2967. |
[111] |
Zhang CY, Wang Y, Schubert R, et al. (2016) Effect of audible sound on protein crystallization. Cryst Growth Des 16: 705-713. doi: 10.1021/acs.cgd.5b01268
![]() |
[112] |
Guo F, Li P, French JB, et al. (2015) Controlling cell–cell interactions using surface acoustic waves. Proc Natl Acad Sci 112: 43-48. doi: 10.1073/pnas.1422068112
![]() |
[113] |
Vogel V, Sheetz M (2006) Local force and geometry sensing regulate cell functions. Nat Rev Mol Cell Biol 7: 265-275. doi: 10.1038/nrm1890
![]() |
[114] |
Shaobin G, Wu Y, Li K, et al. (2010) A pilot study of the effect of audible sound on the growth of Escherichia coli. Colloid Surface B 78: 367-371. doi: 10.1016/j.colsurfb.2010.02.028
![]() |
[115] |
Gu SB, Yang B, Wu Y, et al. (2013) Growth and physiological characteristics of E. coli in response to the exposure of sound field. Pakistan J Biol Sci 16: 969-975. doi: 10.3923/pjbs.2013.969.975
![]() |
[116] |
Sahu S, Ghosh S, Fujita D, et al. (2014) Live visualizations of single isolated tubulin protein self-assembly via tunneling current: effect of electromagnetic pumping during spontaneous growth of microtubule. Sci Rep 4: 7303. doi: 10.1038/srep07303
![]() |
[117] |
Acbas G, Niessen KA, Snell EH, et al. (2014) Optical measurements of long-range protein vibrations. Nat Commun 5: 3076. doi: 10.1038/ncomms4076
![]() |
[118] |
Christians ES, Benjamin IJ (2012) Proteostasis and REDOX state in the heart. Am J Physiol Heart Circ Physiol 302: H24-H37. doi: 10.1152/ajpheart.00903.2011
![]() |
[119] |
Christians ES, Mustafi SB, Benjamin IJ (2014) Chaperones and cardiac misfolding protein diseases. Curr Protein Pept Sci 15: 189-204. doi: 10.2174/1389203715666140331111518
![]() |
[120] |
Naviaux RK (2014) Metabolic features of the cell danger response. Mitochondrion 16: 7-17. doi: 10.1016/j.mito.2013.08.006
![]() |
[121] |
Crum A, Zuckerman B (2017) Changing mindsets to enhance treatment effectiveness. J Am Med Assoc 317: 2063-2064. doi: 10.1001/jama.2017.4545
![]() |
[122] |
Maas C, Belgardt D, Han KL, et al. (2009) Synaptic activation modifies microtubules underlying transport of postsynaptic cargo. Proc Natl Acad Sci 106: 8731-8736. doi: 10.1073/pnas.0812391106
![]() |
[123] |
Lo LP, Liu SH, Chang YC (2007) Assembling microtubules disintegrate the postsynaptic density in vitro. Cell Motil Cytoskeleton 64: 6-18. doi: 10.1002/cm.20163
![]() |
[124] |
Arimura N, Kaibuchi K (2007) Neuronal polarity: From extracellular signals to intracellular mechanisms. Nat Rev Neurosci 8: 194-205. doi: 10.1038/nrn2056
![]() |
[125] |
Macario AJL, Conway de Macario E (2000) Stress and molecular chaperones in disease. Int J Clin Lab Res 30: 49-66. doi: 10.1007/s005990070016
![]() |
[126] |
Dal Lin C, Marinova M, Brugnolo L, et al. Rapid senectome and alternative splicing miRNAs changes with the relaxation response: A one year follow-up study, 2020 Available from: |
[127] |
Picard M, McManus MJ, Gray JD, et al. (2015) Mitochondrial functions modulate neuroendocrine, metabolic, inflammatory, and transcriptional responses to acute psychological stress. Proc Natl Acad Sci 112: E6614-E6623. doi: 10.1073/pnas.1515733112
![]() |
[128] | Piattelli-Palmarini M, Vitiello G (2015) Linguistics and some aspects of its underlying dynamics. Biolinguistics 9: 96-115. |
[129] |
Mańka R, Ogrodnik B (1991) A model of soliton transport along microtubules. J Biol Phys 18: 85-189. doi: 10.1007/BF00417807
![]() |
[130] |
Kučera O, Havelka D (2012) Mechano-electrical vibrations of microtubules-Link to subcellular morphology. BioSystems 109: 346-355. doi: 10.1016/j.biosystems.2012.04.009
![]() |
[131] |
Benias PC, Wells RG, Sackey-Aboagye B, et al. (2018) Structure and distribution of an unrecognized interstitium in human tissues. Sci Rep 8: 4947. doi: 10.1038/s41598-018-23062-6
![]() |
[132] |
Brizhik L, Chiappini E, Stefanini P, et al. (2019) Modeling meridians within the quantum field theory. J Acupunct Meridian Stud 12: 29-36. doi: 10.1016/j.jams.2018.06.009
![]() |
[133] | Bentov I (1977) Stalking the wild pendulum Glasgow: William Collins Sons & Co. Ltd. |
[134] |
Pavanello S, Campisi m, Tona F, et al. (2019) Exploring epigenetic age in response to intensive relaxing training: A pilot study to slow down biological age. Int J Env Res Pub He 16: 3074. doi: 10.3390/ijerph16173074
![]() |
[135] | Dal Lin C, Grasso R, Scordino A, et al. (2020) Ph, electric conductivity and delayed luminescence changes in human sera of subjects undergoing the relaxation response: A pilot study Available from: doi:10.20944/PREPRINTS202004.0202.V1. |
[136] |
Cifra M, Brouder C, Nerudová M, et al. (2015) Biophotons, coherence and photocount statistics: A critical review. J Lumin 164: 38-51. doi: 10.1016/j.jlumin.2015.03.020
![]() |
[137] |
Boveris A, Cadenas E, Reiter R (1980) Organ chemiluminescence: noninvasive assay for oxidative radical reactions. Proc Natl Acad Sci 77: 347-351. doi: 10.1073/pnas.77.1.347
![]() |
[138] |
Krasovitski B, Frenkel V, Shoham S (2011) Intramembrane cavitation as a unifying mechanism for ultrasound-induced bioeffects. Proc Natl Acad Sci 108: 3258-3263. doi: 10.1073/pnas.1015771108
![]() |
[139] |
Brujan EA (2000) Collapse of cavitation bubbles in blood. Europhys Lett 50: 175. doi: 10.1209/epl/i2000-00251-7
![]() |
[140] |
Brennen CE (2015) Cavitation in medicine. Interface Focus 5: 20150022. doi: 10.1098/rsfs.2015.0022
![]() |
[141] |
Didenko YT, Suslick KS (2002) The energy efficiency of formation of photons radicals and ions during single-bubble cavitation. Nature 418: 394-397. doi: 10.1038/nature00895
![]() |
[142] |
Sabbadini SA, Vitiello G (2019) Entanglement and phase-mediated correlations in quantum field theory. Application to brain-mind states. Appl Sci 9: 3203. doi: 10.3390/app9153203
![]() |
[143] |
Shaffer F, McCraty R, Zerr CL (2014) A healthy heart is not a metronome: an integrative review of the heart's anatomy and heart rate variability. Front Psychol 5: 1040. doi: 10.3389/fpsyg.2014.01040
![]() |
[144] |
Grippo A (2011) The utility of animal models in understanding links between psychosocial processes and cardiovascular health. Soc Pers Psychol Compass 5: 164-179. doi: 10.1111/j.1751-9004.2011.00342.x
![]() |
[145] |
Mensah G, Collins P (2015) Understanding mental health for the prevention and control of cardiovascular diseases. Glob Heart 10: 221. doi: 10.1016/j.gheart.2015.08.003
![]() |
1. | Bin Long, Yiying Yang, Applying Lin's method to constructing heteroclinic orbits near the heteroclinic chain, 2024, 47, 0170-4214, 10235, 10.1002/mma.10118 |