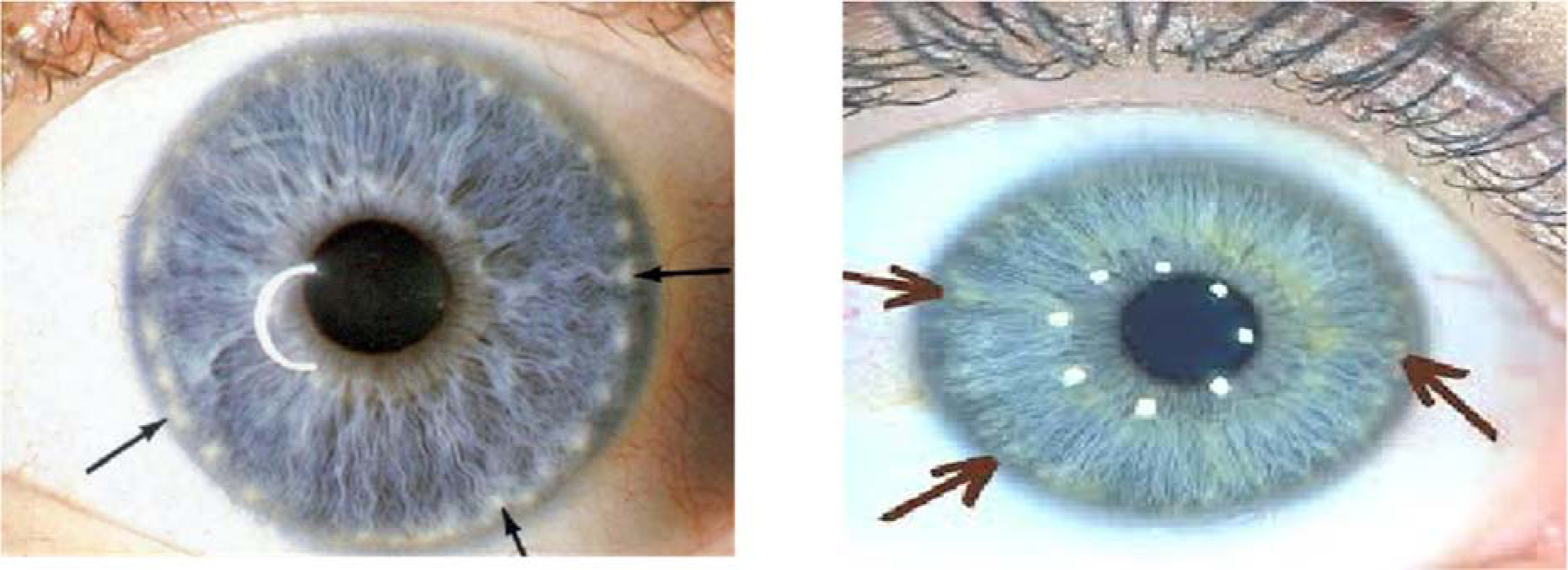
Lymphatic dysfunction is characterized by the sluggish movement of lymph fluids. It manifests as a “Lymphatic Rosary” in the iris which is often due to dehydration and inactivity. Detecting these signs early is crucial for diagnosis and treatment. Therefore, there is a need for an automated, accurate, and efficient method to detect lymphatic rosaries in the iris. This paper presents a novel approach for detecting a lymphatic rosary using advanced image processing techniques. The proposed method involves iris segmentation to isolate the iris from the eye, followed by normalization to standardize its structure, and concludes with the application of the modified Daugman method to identify the lymphatic rosary. This automated process aims to enhance the accuracy and reliability of detection by minimizing the subjectivity associated with manual analysis. The proposed approach was tested on various iris images and the results demonstrated impressive accuracy in detecting lymphatic rosaries. The use of different iris code bit representations allowed for a robust detection process, showcasing the method's effectiveness by achieving an accuracy of 94.5% in identifying the presence of a lymphatic rosary across different stages. The novel image processing technique outlined in this paper offers a promising solution for the automated detection of lymphatic rosaries in the iris. The approach not only improves diagnostic accuracy but also provides a standardized method that could be widely implemented in clinical settings. This advancement in iris diagnosis has the potential to play a significant role in the early detection and management of lymphatic dysfunction.
Citation: Poovayar Priya Mohan, Ezhilarasan Murugesan. A novel approach for detecting the presence of a lymphatic rosary in the iris using a 3-stage algorithm[J]. AIMS Bioengineering, 2024, 11(4): 506-526. doi: 10.3934/bioeng.2024023
[1] | Zheng Guo, Siqi Li, Kaicong Chen, Xuehui Zang . Robust QRS complex detection in noisy electrocardiogram based on underdamped periodic stochastic resonance. AIMS Bioengineering, 2023, 10(3): 283-299. doi: 10.3934/bioeng.2023018 |
[2] | Shital Hajare, Rajendra Rewatkar, K.T.V. Reddy . Design of an iterative method for enhanced early prediction of acute coronary syndrome using XAI analysis. AIMS Bioengineering, 2024, 11(3): 301-322. doi: 10.3934/bioeng.2024016 |
[3] | Joe A. Lemire, Marc A. Demeter, Iain George, Howard Ceri, Raymond J. Turner . A novel approach for harnessing biofilm communities in moving bed biofilm reactors for industrial wastewater treatment. AIMS Bioengineering, 2015, 2(4): 387-403. doi: 10.3934/bioeng.2015.4.387 |
[4] | Lamia Fatiha KAZI TANI, Mohammed Yassine KAZI TANI, Benamar KADRI . Gas-Net: A deep neural network for gastric tumor semantic segmentation. AIMS Bioengineering, 2022, 9(3): 266-282. doi: 10.3934/bioeng.2022018 |
[5] | Dominik Gaweł, Paweł Główka, Michał Nowak, Tomasz Kotwicki . Visualization of the medical imaging data in 3D space using portable format. AIMS Bioengineering, 2016, 3(2): 176-187. doi: 10.3934/bioeng.2016.2.176 |
[6] | Sumaiya Noor, Salman A. AlQahtani, Salman Khan . Chronic liver disease detection using ranking and projection-based feature optimization with deep learning. AIMS Bioengineering, 2025, 12(1): 50-68. doi: 10.3934/bioeng.2025003 |
[7] | Simge Çelebi, Mert Burkay Çöteli . Red and white blood cell classification using Artificial Neural Networks. AIMS Bioengineering, 2018, 5(3): 179-191. doi: 10.3934/bioeng.2018.3.179 |
[8] | Islam Uddin, Salman A. AlQahtani, Sumaiya Noor, Salman Khan . Deep-m6Am: a deep learning model for identifying N6, 2′-O-Dimethyladenosine (m6Am) sites using hybrid features. AIMS Bioengineering, 2025, 12(1): 145-161. doi: 10.3934/bioeng.2025006 |
[9] | Artur Luczak . How artificial intelligence reduces human bias in diagnostics?. AIMS Bioengineering, 2025, 12(1): 69-89. doi: 10.3934/bioeng.2025004 |
[10] | Azhar Alhasawi, Vasu D. Appanna . Enhanced extracellular chitinase production in Pseudomonas fluorescens: biotechnological implications. AIMS Bioengineering, 2017, 4(3): 366-375. doi: 10.3934/bioeng.2017.3.366 |
Lymphatic dysfunction is characterized by the sluggish movement of lymph fluids. It manifests as a “Lymphatic Rosary” in the iris which is often due to dehydration and inactivity. Detecting these signs early is crucial for diagnosis and treatment. Therefore, there is a need for an automated, accurate, and efficient method to detect lymphatic rosaries in the iris. This paper presents a novel approach for detecting a lymphatic rosary using advanced image processing techniques. The proposed method involves iris segmentation to isolate the iris from the eye, followed by normalization to standardize its structure, and concludes with the application of the modified Daugman method to identify the lymphatic rosary. This automated process aims to enhance the accuracy and reliability of detection by minimizing the subjectivity associated with manual analysis. The proposed approach was tested on various iris images and the results demonstrated impressive accuracy in detecting lymphatic rosaries. The use of different iris code bit representations allowed for a robust detection process, showcasing the method's effectiveness by achieving an accuracy of 94.5% in identifying the presence of a lymphatic rosary across different stages. The novel image processing technique outlined in this paper offers a promising solution for the automated detection of lymphatic rosaries in the iris. The approach not only improves diagnostic accuracy but also provides a standardized method that could be widely implemented in clinical settings. This advancement in iris diagnosis has the potential to play a significant role in the early detection and management of lymphatic dysfunction.
The study of iridology enhances our ability to see and understand the inner self. The world inside is a throbbing, beautiful tapestry of dynamic activity, living, moving chemistry, astonishing cellular metabolism processes, and waves of absorption and elimination that hold an immense amount of life, energy, and power. Our physical form is quite amazing [1]. People of different ages, races, and cultures may understand the language of iridology. The iris reveals its mysteries to those who study the markings of the individual and learn the language. Since iridology shows the underlying etiology of the disease in each individual, it does not take long to realize that every instance of arthritis is unique. Because the practitioner can precisely prescribe what each patient requires based on the interpretation of their iridology, treatment is particularly effective [2]. A highly potent remedy is doing the right thing at the right moment.
The lymphatic system is made up of a vascular network of capillaries with thin walls that remove lymph that is rich in protein from the extracellular spaces found in most organs [3]. The lymphatic capillaries are lined with an overlaying layer of continuous endothelial cells, which lack a continuous basement membrane and are thus extremely porous. Through the thoracic duct and the bigger lymphatic collecting channels, which have an adventitial layer and a muscular layer, lymph returns to the vein. The lymphatic system includes lymphoid organs, including the tonsils, spleen, lymph nodes, Peyer's patches, and thymus, all of which are crucial for the immune response [4].
In the lymphatic zone of the iris shown in Figure 1, which is adjacent to the skin zone in the ciliary border, the lymph system is readily visible. This zone exhibits abnormalities in several ways, such as a lymphatic rosary, as isolated lymphatic tophi in organs or other regions, and as atypical hues that indicate the degree of encumbrance and malfunction, such as grey, yellow, orange, brown, or black. Muscle tension prevents lymph from flowing through the lymph zone, as indicated by nerve rings that pass through it [5]. The lymph zone may be covered in a translucent to opaque white ring made of salt, calcium, or hypercholesterol. Additionally, mucus and catarrh accumulation in the circulation zone next to the ANW is a sign of compromised lymphatic function [6]. Examine the iris regions of the neck, breast, adenoids in the nose, tonsils in the neck, appendix in the caecum area, groin, underarm (axilla), and small intestine for Peyer's patches. The thymus region is situated in each eye close to the sternum [7]. If a person has weakened immunity or lymphatic problems, they are all significant components of the lymphatic system that need to be evaluated. Keep in mind that the colors of the lymphatic markings represent different states of activity: white denotes an active exudative state, grey denotes underactivity, yellow denotes obvious sluggishness, and brownish markings [8] suggest a chronic malfunctioning toxic situation.
The ability to superimpose zones on normal analytic charts has been one of the useful developments of iris charts. In Figure 2, there are seven zones:
1. The stomach region
2. Intestinal area
3. The pancreas, kidneys, solar plexus, heart, and aorta
4. Pituitary gland and bronchial tubes, pineal gland
5. Reproductive organs and the brain
6. Liver, thyroid, and spleen
7. Sweat glands, skin, lymphatic and circulatory systems, sensory and motor neurons [2]
Lymphatic rosary: In Zone 6, the lymphatic system is displayed. When toxic wastes cause the lymphatic circulation to become slow and clogged, tiny, cloud-like patches in the iris appear, as shown in Figure 3. This symbol could seem like a string of tiny white pearls or beads about in line with the outer rim's curve, although this is not usually the case. Moreover, lymphatic congestion may manifest as isolated spots in a single organ. Upon closer inspection, the individual spots appear bigger, elevated above the iris's surface, and crowded. White denotes acute activity, yellow subacute activity, brown chronic or degenerative activity, and occasionally poisonous activity. When there is a malfunction in the lymphatic system, mucus and catarrh have accumulated. Exercise and muscular contraction are the only factors that affect lymphatic circulation. Lymph tissue comprises most of the spleen, appendix, adenoids, and tonsils [9].
The illness progresses in three stages:
1. Incubation period: Zone 6 experiences a slight white appearance during the first stage of the process.
2. Prodromal stage: The second stage, which is surrounded by dots of white color in zone 6.
3. Illness: The third phase, where zone 6 shows dots of white color.
For this paper, the main contributions are as follows:
We propose a novel image processing technique specifically designed to detect lymphatic rosaries in the iris. This approach leverages the Iris U-Net model for accurate iris segmentation, followed by an image enhancement method to improve the clarity and quality of iris images, which is essential for accurate diagnosis.
A key innovation of this research is the development of a three-stage algorithm for lymphatic rosary detection. This algorithm incorporates a modified Daugman method with varied bits representation, enabling precise detection across different stages of lymphatic rosary presence.
The proposed method was rigorously tested on a diverse set of iris images, and the experimental results demonstrated a significant improvement in detection accuracy compared to traditional methods. The method's ability to consistently identify lymphatic rosaries across varying conditions highlights its robustness and practical applicability.
The research provides a new tool for automated iris analysis and lays the groundwork for future studies in iridology. The approach can be extended to detect other iris-based indicators of health disorders, offering a broader impact on diagnostic practices.
Using various attentions in the CNN architecture, Ran Gu [10] has developed a comprehensive attention-based CNN (CA-Net) model to produce a more accurate and understandable medical picture segmentation. The segmentation performance can be enhanced by focusing on the most pertinent scales and channels. When it comes to segmentation tasks, CA-Net provides a thorough description of how each geographic location, feature map channel, and scale is employed for prediction.
Compared to previous approaches, this network functions significantly more effectively and comprehensibly. The CA-Net approach yielded a Dice score of 92.08%. This technique can be readily expanded in the future to segment 3D images, as well.
To highlight the crucial elements that are transferred into the skip connections, Ozan Oktay et al. [11] proposed attention gates, which are integrated into the conventional U-Net architecture. In skip connections, information filtered from the coarse scale aids in separating the useful replies from the noisy ones. To ensure that only pertinent activations are linked, this is done right before concatenation. In addition, AGs filter neuronal activations in both the forward and reverse passes. The gradients that begin in background areas are downweighted during the backward pass. This enables the model parameters to be updated based on the spatial regions where the shallower layers are located.
For semantic segmentation, LinkNet is a light deep neural network architecture that can be applied to augmented reality and self-driving car applications. NL-LinkNet was proposed by Yooseung Wang et al. [12] and can find relationships between global properties. This enables every spatial feature point to make use of all other contextual data, resulting in more precise road segmentation. Details: In the official DeepGlobe Challenge, this single model outperformed all previously published state-of-the-art ensemble models without any post-processing, such as conditional random field (CRF) refinement. Furthermore, compared to D-LinkNet, the DeepGlobe Challenge winner, our nonlocal LinkNet (NL-LinkNet) performed better with 43% fewer parameters, fewer giga floating-point operations per second (GFLOPs), and a faster training convergence time.
Zhenghau Chen proposed multi-scale Retinex and non-local mean filtering [13]. To improve texture, NLM filtering first lessens the issue of speckle noise. The filtered SAR image is then combined with the MSR to enhance the textural features of the internal waves within the water. In the experiment, actual Gaofen-3 satellite SAR images were used to enhance the textural characteristics of SAR images of oceanic internal waves. The outcomes show that the suggested technique may considerably enhance internal wave texture while successfully reducing picture noise.
An image-processing method called histogram equalization (HE) is used to improve contrast in pictures. The main goal of HE is to alter an image's pixel values to produce a more uniform distribution throughout the whole range of available values [14]. The first step in the HE process is to create the image's histogram, which is a graphical depiction of how frequently each pixel value appears in the image [8]. To offer a more uniform distribution of values over the whole range of potential values, the histogram is then equalized by redistributing the pixel values [15].
Adaptive histogram equalization (AHE), a variation on the histogram equalization technique, can be used to increase an image's contrast, particularly in areas with low contrast or inconsistent illumination [16]. Unlike regular histogram equalization, which applies the same equalization function to the entire image, AHE applies different equalization functions to different picture regions based on the local image statistics. The AHE process involves dividing the image into distinct regions, or tiles, figuring out each tile's histogram, and then independently equalizing each histogram. This ensures that contrast enhancement is only added to the image's necessary regions. Maintaining the image's local contrast while enhancing overall contrast is one of AHE's primary benefits.
A method called contrast limited adaptive histogram equalization (CLAHE) can improve contrast in an image without introducing artifacts like the “halo effect” that can happen when using adaptive histogram equalization (AHE) [17]. In image regions with a small histogram, classical AHE may over-enhance contrast [18]. The “halo effect”, a noticeable grid-like pattern surrounding the borders, could be caused by noise and artifacts that are overly accentuated in certain areas. By restricting the contrast enhancement in each image tile, CLAHE gets around this restriction. A preset number known as the “clip limit” is used to limit the histogram by clipping it [19]. This ensures that contrast improvement in areas with a narrow histogram does not overamplify noise and artifacts [20].
In the context of a Medical Intensive Care Unit (MICU) [21], image super-resolution plays a pivotal role in enhancing the clarity and detail of medical images, which is crucial for accurate diagnosis and treatment planning. By employing advanced techniques such as U-Net architecture, which is specifically designed for biomedical image segmentation, healthcare professionals can achieve significant improvements in image quality. U-Net facilitates the effective segmentation and enhancement of medical images, thereby supporting the super-resolution process. Moreover, deep learning has revolutionized medical imaging by providing sophisticated algorithms that automate and enhance image processing. Generative adversarial networks (GANs) further contribute to this field by generating high-resolution images from low-resolution inputs, thereby improving diagnostic capabilities in the MICU. The integration of multi-level information compensation within these frameworks allows for a more nuanced approach to image enhancement, ensuring that critical details are preserved and highlighted. Ultimately, the combination of these technologies not only enhances image quality but also leads to better patient outcomes through improved visualization of medical data.
The DNNAM algorithm [22] for image inpainting leverages deep neural networks and an attention mechanism to enhance the reconstruction of missing image regions. Central to this approach is the use of a feed-forward, fully convolutional neural network, which is adept at processing images with multiple holes of varying sizes and locations, addressing the limitations of traditional convolutional networks in capturing distant spatial information. The algorithm incorporates a generative model-based approach that synthesizes novel image structures while utilizing surrounding features as references during training, thereby improving the coherence and quality of the inpainted results. The contextual attention mechanism also allows the model to effectively borrow information from distant areas, which is crucial for maintaining the visual integrity of the reconstructed textures and structures. Furthermore, the integration of patch-based inpainting techniques enhances the algorithm's ability to fill in missing regions by copying similar patches from surrounding areas, ensuring local continuity and visual consistency. DNNAM represents a significant advancement in image inpainting, combining deep learning methodologies with attention mechanisms to achieve superior results.
The MFMAM (multi-scale feature module with attention mechanisms) approach [23] enhances image inpainting by integrating multi-scale feature processing and contextual attention mechanisms. Image inpainting is fundamentally about reconstructing lost or damaged parts of an image using surrounding data, which is crucial for restoring visual integrity and quality. The multi-scale feature module captures both local and global information, allowing for a more nuanced understanding of the image context, which is essential for realistic inpainting. Additionally, the contextual attention module focuses on the relationships between surrounding pixels, improving the accuracy of the inpainting process by ensuring that the filled areas are coherent with the overall image context. This combination of techniques not only enhances the quality of the inpainted regions but also leverages semantic features that capture the meaning and context of the image, further contributing to the realism of the restoration. Ultimately, the integration of these advanced modules leads to significant improvements in image restoration, as evidenced by enhanced PSNR (Peak Signal-to-Noise Ratio) values in restored images.
Naeem A.B. et.al. explored the potential of integrating sensor data with artificial neural networks (ANNs) to enhance the early detection of heart disease [24]. By leveraging various sensors, such as ECG and PPG, crucial physiological data can be collected and analyzed. Through advanced feature extraction techniques, relevant indicators like heart rate variability and waveform patterns are derived from the sensor data. These features are then fed into an ANN model, specifically designed to identify patterns associated with heart disease. The ANN's ability to learn from large datasets enables it to distinguish between healthy and at-risk individuals with high accuracy. This approach not only offers a non-invasive, real-time solution for monitoring heart health but also promises to improve early diagnosis, thereby facilitating timely intervention and reducing the overall burden of heart disease.
To address the challenge of automatically identifying oral disease in white-light smartphone photos, Eman Shawky Mira [21] provided an easy-to-use yet effective image-collection and resampling approach that leverages deep learning algorithms. We use our collection of photos from five different sickness categories to test the latest HR-Net, which was pre-trained on ImageNet. The results demonstrate that our methods can greatly improve smartphone photography image prediction ability for early cancer diagnosis. Kailash Kumar [25] focuses on creating a hybrid fuzzy deep neural network model for early-stage Chronic Kidney Disease prediction. This model is then assessed and compared to the existing radioimmunoassay technique. The findings demonstrate that the suggested model performs better at accurately identifying diseases than the current approach.
A model for deep learning, the MobileNetV2 architecture served as the foundation for TL-MobileNetV2. The suggested model by Yonis Gulzar [26] was trained and tested on a dataset of forty different fruit varieties. To increase the model's accuracy and efficiency, the MobileNetV2 architecture's classification layer was removed and five more layers were added to the TL-MobileNetV2 model. Future research will improve a mobile application by incorporating a greater variety of fruits, to expand the scope of fruit classification. People with little experience will find this program useful in learning how to categorize various fruit species. Additionally, the dataset will be used to train various CNN models, and the outcomes will be compared to determine which model fits the data the best in terms of efficiency and accuracy.
Iris images were gathered using a Mobile-Eyes™ sensor, a product of L-1 Identify Solutions, across two sessions. The patient database consists of 1000 iris images, with 500 left and 500 right images taken in and around Puducherry Hospitals and clinics. During image capture, patients were asked if they wore contact lenses, and this information was recorded. Images were captured using near-infrared (NIR) light, producing SVGA images with a width of 752 × 480 at a rate of 30 frames per second. The device features dual-iris enrollment, allowing simultaneous auto-capture of both the left and right eyes. It operates effectively in both dim and bright lighting conditions. The captured images were saved in PNG format with a resolution of 640 × 480.
The Mobile-Eyes™ sensors are powered by the Iris Analysis Software SDK, which includes modules for focus measurement, image segmentation, encoding, and scoring. During the capture process, patients were positioned about 5 cm from the scanner, and images were taken under standard room lighting conditions. To avoid occlusions, patients were instructed to keep their eyes wide open. The SDK's image assessment scoring module ensures that only images exceeding a predetermined evaluation score were stored or captured.
The acquired eye image was used to segment the iris using the Iris U-Net architecture [27]. The Iris U-Net architecture processes 572 × 572 input images and is structured with five layers. It leverages skip connections and detailed localization for enhanced performance. Skip connections link the encoder and decoder layers, merging high-resolution encoder features with upsampled decoder features. This design retains spatial information and improves pixel-level prediction accuracy. Additionally, the Iris U-Net achieves precise localization by integrating low-level and high-level data, which is critical for tasks like medical imaging where minute details are essential. The architecture consists of three main components: the upsampling path (decoder) for high-resolution reconstruction, the downsampling path (encoder) for capturing contextual information, and the bottleneck layer that facilitates feature integration between the encoder and decoder. This structure enables accurate segmentation, as shown in Figure 4, and effective context capture.
Iris enhancement was performed using CLAHE [19] and the Single Scale Retinex [28] technique. Data for training and testing came from the following subject sets: 140 images with 640 × 480 resolution in .png format from the PEC dataset. The CLAHE model only enhances the local features of iris images. Nevertheless, as Figure 5 illustrates, when CLAHE and SSR are coupled, local and global features are retrieved, yielding more alluring results.
During the normalization process, the iris area is altered to meet predetermined dimensions. In addition, variations in picture distance, head tilt, camera rotation, and eye rotation can all cause dilation. A successful normalization process should provide different iris regions for different iris types in the same situations as well as uniform dimensions for the same iris types in different circumstances. Daugman's rubber-sheet model [29] explains the remap of each iris region's point to the polar coordinates (r, Ɵ) in Figure 6.
Iris recognition is accomplished using a 1-bit iris code in the Daugman method [29]. Several samples have been taken to identify the presence of a lymphatic rosary. After the iris has been normalized, we have divided the normalized image into the seven zones shown by [2] to detect the presence of a lymphatic rosary in the iris. Next, we processed the iris image by selecting the sixth zone. Three phases of lymphatic rosary detection have been identified.
During the incubation phase of lymphatic rosary detection, the following 2-bit iris code representation is implemented in Table 1.
Lymphatic Rosary – Incubation Stage |
Step 1: Normalize and extract the 6th zone. Step 1a: The normalized iris image I has dimensions “h × w” (height h and width w) where each element I [i, j] is a grayscale pixel value in the range [0, 255]. Step 1b: Let the 6th zone of I [i, j] be denoted by R. Therefore, R = I [5, :] {using zero-based indexing}. Step 2: Divide the grayscale pixel values into four intensity levels. Pixel values in image R are in the range [0, 255]. Divide this range into four intervals: Step 3: Apply quantization. For each pixel value p in R, apply Q(p) to get the corresponding 2-bit code. Step 4: Form the iris code. The final iris code CR is the concatenation of the 2-bit codes for all pixels in R, which is expressed as where |
In the prodromal stage of the detection of the lymphatic rosary, a 3-bit representation of the iris code is implemented as follows in Table 2.
Lymphatic Rosary – Prodromal Stage |
Step 1: Normalize and extract the 7th zone. Step 1a: The normalized iris image I has dimensions “h × w” (height h and width w) where each element I [i, j] is a grayscale pixel value in the range [0, 255]. Step 1b: Let the 7th zone of I [i, j] be denoted by R. Therefore, R = I [5, :] {using zero-based indexing}. Step 2: Divide the grayscale pixel values into four intensity levels. Pixel values in image R are in the range [0, 255]. Divide this range into eight intervals: Step 3: Apply quantization. For each pixel value p in R, apply Q(p) to get the corresponding 3-bit code. Step 4: Form the iris code. The final iris code CR is the concatenation of the 3-bit code for all pixels in R, which is expressed as where |
In the illness stage of the detection of a lymphatic rosary, a 4-bit representation of the iris code is implemented as follows in Table 3.
Lymphatic Rosary Detection – Illness Stage |
Step 1: Normalize and extract the 7th zone. Step 1a: The normalized iris image I has dimensions “h × w” (height h and width w) where each element I [i, j] is a grayscale pixel value in the range [0, 255]. Step 1b: Let the 7th zone of I [i, j] be denoted by R. Therefore, R = I [6, :] {using zero-based indexing}. Step 2: Divide the grayscale pixel values into four intensity levels. Pixel values in image R are in the range [0, 255]. Divide this range into eight intervals: Step 3: Apply quantization. For each pixel value p in R, apply Q(p) to get the corresponding 4-bit code. Step 4: Form the iris code. The final iris code CR is the concatenation of the 4-bit code for all pixels in R, which is expressed as where |
Lymphatic rosary detection utilizes varying quantization schemes tailored to different stages of lymphatic conditions. The algorithm applies a systematic approach for processing iris images, where the 6th zone of the normalized grayscale image is analyzed for skin disorder detection. For the Incubation stage, a 2-bit quantization method segments grayscale pixel values into four distinct intensity levels facilitating preliminary detection. In the prodromal stage, a 3-bit quantization provides a more granular classification by dividing the grayscale values into eight intervals enhancing detection precision. Finally, the illness stage employs a 4-bit quantization which further refines classification by distinguishing between sixteen intensity levels allowing for accurate identification of high-risk conditions. By leveraging these quantization techniques, the system effectively categorizes lymphatic rosaries into incubation, prodromal, and illness stages, thus offering a robust system for early detection and monitoring of lymphatic rosary health issues.
Figure 7 illustrates samples of the iris at the early stage of the presence of a lymphatic rosary. We have taken 30 samples of iris images, from which zone 6 is extracted. Figure 8 represents the presence of a lymphatic rosary in iris images.
Figure 9 illustrates samples of the iris at the next stage of the presence of a lymphatic rosary in the iris. We have taken 30 samples of iris images, from which zone 6 is extracted. Figure 10 represents the presence of a lymphatic rosary in iris images.
Figure 11 illustrates samples of the iris at the last stage of the presence of a lymphatic rosary. We have taken 30 samples of iris images, from which zone 6 is extracted. Figure 12 represents the presence of a lymphatic rosary in iris images.
The line plot displays the relationship between pixel values and intensity levels in the lymphatic rosary image. Figure 13 provides insights into the distribution of intensity values across 2-bit pixels in the lymphatic rosary image.
Figure 14 provides insights into the distribution of intensity values across 3-bit pixels in the lymphatic rosary image.
Figure 15 provides insights into the distribution of intensity values across 4-bit pixels in the lymphatic rosary image.
It creates a line plot using the “line plot” function, where the x-axis represents the Pixel column, the y-axis represents the Intensity Value column, and different colors are used to differentiate data from different sources. Figure 16 provides insights into the distribution of intensity values across different pixels in lymphatic rosary images.
As shown in Table 1, we compare our method with existing network models. The Authors column of Table 1 shows similar work that was done by other authors with different applications. The Accuracy column shows the percentage of subjects classified through iris images and algorithms as lymphatic rosary subjects. From Table 1, the results show that the proposed method has 95% accuracy compared with other methods. This indicates that the findings obtained are indeed effective and promising.
S. No | Authors | Accuracy |
1. | Naeem A.B. et al. [24] | 86% |
2. | Mira E.S. et al. [25] | 92% |
3. | Kumar K. et al. [26] | 93% |
4. | Proposed method | 94.5% |
The proposed image processing technique for detecting lymphatic rosaries in the iris has demonstrated significant improvements over traditional manual methods. By leveraging the Iris U-Net model for precise iris segmentation and employing an image enhancement approach, we improved the quality of iris images, which is crucial for accurate detection. The normalization process focused on extracting zone 6 of the iris, and the application of the Daugman technique, allowed for the precise identification of the lymphatic rosary. The 3-stage algorithm, which utilizes a modified Daugman method with varied bits representation, proved to be highly effective in detecting lymphatic rosaries at different stages. The experimental results showcased the method's superior performance with a 94.5% accuracy and reliability in identifying the presence of lymphatic rosaries.
This research highlights the proposed method's effectiveness and underscores its significance in advancing diagnostic practices. The automated detection approach minimizes subjectivity and errors associated with manual iridology, offering a standardized solution that can be widely implemented in clinical settings. Furthermore, future research focuses on using iridology to detect a broader range of disorders. The results affirm that this technique can play a pivotal role in early diagnosis and the management of lymphatic dysfunction and related health conditions.
The authors declare they have not used Artificial Intelligence (AI) tools in the creation of this article.
[1] |
Sharan F (1989) Iridology: A Complete Guide to Diagnosing through the Iris and to Related Forms of Treatment. New York: HarperThorsons. |
[2] | Jensen B (1980) Iridology Simplified. Canada: Book Publishing Company. |
[3] | Kryzhanivska O (2020) Iris changes at patients with temporomandibular joint diseases and urinary system pathology. MSU 16: 28-34. https://doi.org/10.32345/2664-4738.4.2020.5 |
[4] |
Sen M, Honavar SG (2022) Theodor karl gustav von leber: the sultan of selten. Indian J Ophthalmol 70: 2218-2220. https://doi.org/10.4103/ijo.IJO_1379_22 ![]() |
[5] | de Oliveira ER, de Souza Cardoso J, da Silva Rodrigues VT, et al. (2023) Nocardia niwae infection in dogs. Acta Sci Vet 51: 900. https://doi.org/10.22456/1679-9216.131057 |
[6] |
Caruso M, Catalano O, Bard R, et al. (2022) Non-glandular findings on breast ultrasound. Part I: a pictorial review of superficial lesions. J ultrasound 25: 783-797. https://doi.org/10.1007/s40477-021-00619-2 ![]() |
[7] | Jogi SP, Sharma BB Retracted: methodology of iris image analysis for clinical diagnosis. (2014)2014: 235-240. https://doi.org/10.1109/MedCom.2014.7006010 |
[8] | Atkin S (2013) Bilateral pitting oedema with multiple aetiologies. Aust J Herbal Med 25: 79-82. https://doi.org/10.3316/informit.481869825723186 |
[9] | Jensen B (1982) Iridology: Science and Practice in the Healing Arts. Canada: Book Publishing Company. |
[10] |
Gu R, Wang G, Song T, et al. (2020) CA-Net: comprehensive attention convolutional neural networks for explainable medical image segmentation. IEEE T Med Imaging 40: 699-711. https://doi.org/10.1109/TMI.2020.3035253 ![]() |
[11] | Touvron H, Cord M, Douze M, et al. Training data-efficient image transformers & distillation through attention. (2021)139: 10347-10357. |
[12] | Wang Y, Seo J, Jeon T (2021) NL-LinkNet: toward lighter but more accurate road extraction with nonlocal operations. IEEE Geosci Remote S 19: 1-5. https://doi.org/10.1109/LGRS.2021.3050477 |
[13] | Chen Z, Zeng H, Yang W, et al. Texture enhancement method of oceanic internal waves in SAR images based on non-local mean filtering and multi-scale retinex. (2022)2022: 1-5. https://doi.org/10.1109/CISS57580.2022.9971169 |
[14] | Agrawal S, Panda R, Mishro PK, et al. (2022) A novel joint histogram equalization-based image contrast enhancement. J King Saud Univ-Com 34: 1172-1182. https://doi.org/10.1016/j.jksuci.2019.05.010 |
[15] |
Rao BS (2020) Dynamic histogram equalization for contrast enhancement for digital images. Appl Soft Comput 89: 106114. https://doi.org/10.1016/j.asoc.2020.106114 ![]() |
[16] |
Doshvarpassand S, Wang X, Zhao X (2022) Sub-surface metal loss defect detection using cold thermography and dynamic reference reconstruction (DRR). Struct Health Monit 21: 354-369. https://doi.org/10.1177/1475921721999599 ![]() |
[17] | Murugachandravel J, Anand S (2021) Enhancing MRI brain images using contourlet transform and adaptive histogram equalization. J Med Imag Health In 11: 3024-3027. https://doi.org/10.1166/jmihi.2021.3906 |
[18] |
Radzi SFM, Karim MKA, Saripan MI, et al. (2020) Impact of image contrast enhancement on the stability of radiomics feature quantification on a 2D mammogram radiograph. IEEE Access 8: 127720-127731. https://doi.org/10.1109/ACCESS.2020.3008927 ![]() |
[19] | Kuran U, Kuran EC (2021) Parameter selection for CLAHE using multi-objective cuckoo search algorithm for image contrast enhancement. Intell Syst Appl 12: 200051. https://doi.org/10.1016/j.iswa.2021.200051 |
[20] |
Islam MR, Nahiduzzaman M (2022) Complex features extraction with deep learning model for the detection of COVID-19 from CT scan images using ensemble-based machine learning approach. Expert Syst Appl 195: 116554. https://doi.org/10.1016/j.eswa.2022.116554 ![]() |
[21] |
Chen Y, Xia R, Yang K, et al. (2024) MICU: image super-resolution via multi-level information compensation and U-net. Expert Syst Appl 245: 123111. https://doi.org/10.1016/j.eswa.2023.123111 ![]() |
[22] |
Chen Y, Xia R, Yang K, et al. (2024) DNNAM: image inpainting algorithm via deep neural networks and attention mechanism. Appl Soft Comput 154: 111392. https://doi.org/10.1016/j.asoc.2024.111392 ![]() |
[23] |
Chen Y, Xia R, Yang K, et al. (2024) MFMAM: image inpainting via multi-scale feature module with attention module. Comput Vis Image Und 238: 103883. https://doi.org/10.1016/j.cviu.2023.103883 ![]() |
[24] |
Naeem AB, Senapati B, Bhuva D, et al. (2024) Heart disease detection using feature extraction and artificial neural networks: a sensor-based approach. IEEE Access 12: 37349-37362. https://doi.org/10.1109/ACCESS.2024.3373646 ![]() |
[25] |
Mira ES, Sapri AMS, Aljehanı RF, et al. (2024) Early diagnosis of oral cancer using image processing and artificial intelligence. Fusion: Pract Appl 14: 293-308. https://doi.org/10.54216/FPA.140122 ![]() |
[26] |
Kumar K, Pradeepa M, Mahdal M, et al. (2023) A deep learning approach for kidney disease recognition and prediction through image processing. Appl Sci 13: 3621. https://doi.org/10.3390/app13063621 ![]() |
[27] | Priyal MP, Ezhilarasan M IRIS segmentation technique using IRIS-UNet method. (2023)14124: 235. https://doi.org/10.1007/978-3-031-45382-3_20 |
[28] | Yang Y, Jiang Z, Yang C, et al. Improved retinex image enhancement algorithm based on bilateral filtering. (2015)2015: 1363-1369. https://doi.org/10.2991/icmmcce-15.2015.427 |
[29] |
Daugman J (2007) New methods in iris recognition. IEEE T Syst Man Cy B 37: 1167-1175. https://doi.org/10.1109/TSMCB.2007.903540 ![]() |
Lymphatic Rosary – Incubation Stage |
Step 1: Normalize and extract the 6th zone. Step 1a: The normalized iris image I has dimensions “h × w” (height h and width w) where each element I [i, j] is a grayscale pixel value in the range [0, 255]. Step 1b: Let the 6th zone of I [i, j] be denoted by R. Therefore, R = I [5, :] {using zero-based indexing}. Step 2: Divide the grayscale pixel values into four intensity levels. Pixel values in image R are in the range [0, 255]. Divide this range into four intervals: Step 3: Apply quantization. For each pixel value p in R, apply Q(p) to get the corresponding 2-bit code. Step 4: Form the iris code. The final iris code CR is the concatenation of the 2-bit codes for all pixels in R, which is expressed as where |
Lymphatic Rosary – Prodromal Stage |
Step 1: Normalize and extract the 7th zone. Step 1a: The normalized iris image I has dimensions “h × w” (height h and width w) where each element I [i, j] is a grayscale pixel value in the range [0, 255]. Step 1b: Let the 7th zone of I [i, j] be denoted by R. Therefore, R = I [5, :] {using zero-based indexing}. Step 2: Divide the grayscale pixel values into four intensity levels. Pixel values in image R are in the range [0, 255]. Divide this range into eight intervals: Step 3: Apply quantization. For each pixel value p in R, apply Q(p) to get the corresponding 3-bit code. Step 4: Form the iris code. The final iris code CR is the concatenation of the 3-bit code for all pixels in R, which is expressed as where |
Lymphatic Rosary Detection – Illness Stage |
Step 1: Normalize and extract the 7th zone. Step 1a: The normalized iris image I has dimensions “h × w” (height h and width w) where each element I [i, j] is a grayscale pixel value in the range [0, 255]. Step 1b: Let the 7th zone of I [i, j] be denoted by R. Therefore, R = I [6, :] {using zero-based indexing}. Step 2: Divide the grayscale pixel values into four intensity levels. Pixel values in image R are in the range [0, 255]. Divide this range into eight intervals: Step 3: Apply quantization. For each pixel value p in R, apply Q(p) to get the corresponding 4-bit code. Step 4: Form the iris code. The final iris code CR is the concatenation of the 4-bit code for all pixels in R, which is expressed as where |
Lymphatic Rosary – Incubation Stage |
Step 1: Normalize and extract the 6th zone. Step 1a: The normalized iris image I has dimensions “h × w” (height h and width w) where each element I [i, j] is a grayscale pixel value in the range [0, 255]. Step 1b: Let the 6th zone of I [i, j] be denoted by R. Therefore, R = I [5, :] {using zero-based indexing}. Step 2: Divide the grayscale pixel values into four intensity levels. Pixel values in image R are in the range [0, 255]. Divide this range into four intervals: Step 3: Apply quantization. For each pixel value p in R, apply Q(p) to get the corresponding 2-bit code. Step 4: Form the iris code. The final iris code CR is the concatenation of the 2-bit codes for all pixels in R, which is expressed as where |
Lymphatic Rosary – Prodromal Stage |
Step 1: Normalize and extract the 7th zone. Step 1a: The normalized iris image I has dimensions “h × w” (height h and width w) where each element I [i, j] is a grayscale pixel value in the range [0, 255]. Step 1b: Let the 7th zone of I [i, j] be denoted by R. Therefore, R = I [5, :] {using zero-based indexing}. Step 2: Divide the grayscale pixel values into four intensity levels. Pixel values in image R are in the range [0, 255]. Divide this range into eight intervals: Step 3: Apply quantization. For each pixel value p in R, apply Q(p) to get the corresponding 3-bit code. Step 4: Form the iris code. The final iris code CR is the concatenation of the 3-bit code for all pixels in R, which is expressed as where |
Lymphatic Rosary Detection – Illness Stage |
Step 1: Normalize and extract the 7th zone. Step 1a: The normalized iris image I has dimensions “h × w” (height h and width w) where each element I [i, j] is a grayscale pixel value in the range [0, 255]. Step 1b: Let the 7th zone of I [i, j] be denoted by R. Therefore, R = I [6, :] {using zero-based indexing}. Step 2: Divide the grayscale pixel values into four intensity levels. Pixel values in image R are in the range [0, 255]. Divide this range into eight intervals: Step 3: Apply quantization. For each pixel value p in R, apply Q(p) to get the corresponding 4-bit code. Step 4: Form the iris code. The final iris code CR is the concatenation of the 4-bit code for all pixels in R, which is expressed as where |
S. No | Authors | Accuracy |
1. | Naeem A.B. et al. [24] | 86% |
2. | Mira E.S. et al. [25] | 92% |
3. | Kumar K. et al. [26] | 93% |
4. | Proposed method | 94.5% |