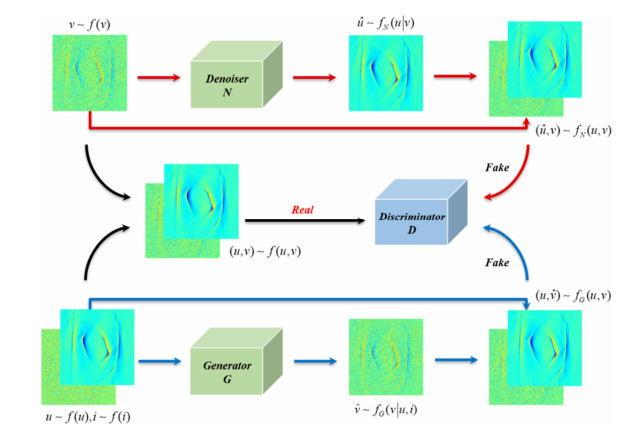
Natural indigo dye production produces indigo waste as a by-product. Our purpose of this study was to examine the effects of calcium hydroxide (Ca(OH)2), cellulase (CE), molasses (MO), and their combinations on the silage quality, in vitro degradability, and rumen fermentation parameters of indigo waste silage. A completely randomized design (CRD) was used for the experiment. Indigo waste was chopped and ensiled in a small-scale silo with no additive (control), Ca(OH)2, MO, CE, Ca(OH)2:MO, Ca(OH)2:CE, MO:CE, and MO:CE:Ca(OH)2. After 30 days of storage, the silages were tested for quality and chemical composition, as well as an in vitro fermentation. The ruminal fluid inoculum was collected from two beef cattle with a body weight (BW) of 200±10 kg, and the inoculum had been pre-heated before being transported to the laboratory. Silage with MO, CE, or their combination increased the amount of lactic acid (p < 0.01). The silage pH was lowest in MO:CE (4.5) and was highest in Ca(OH)2:CE (10.6) in indigo waste (p < 0.01). In comparison to the control (19.5% CP), the CP content of all additives increased by 20.7% to 21.5% (p = 0.02). The addition of Ca(OH)2:MO and Ca(OH)2:CE resulted in a reduction of NDF content by 60.7% and 59.4%, respectively, in comparison to the control group (72.4%) (p < 0.01). Silage with additives had no effect on the cumulative gas production or gas kinetics, except that the constant rate of gas production for the insoluble fraction (c) was higher in MO (p = 0.03). In vitro dry matter degradability (IVDMD) was higher in CE and MO and highest in MO:CE-treated silage (p < 0.01). The in vitro organic matter degradability (IVOMD) increased in Ca(OH)2:MO compared with the control (p = 0.03). The additives alone or in their two combinations in silage reduced the ruminal ammonia-nitrogen (NH3-N) concentration (28.0 to 31.5 mg/dL) when compared to the control (32.7 mg/dL) (p < 0.01). In addition, the highest total volatile fatty acid (VFA) level was found in the silage of the MO (92.9 mmol/L) compared with the control (71.3 mmol/l) (p < 0.01). The proportion of propionic acid and butyric acid increased (p < 0.01) whereas acetic acid decreased (p < 0.01) in the rumen of silage with MO and CE. In summary, the addition of MO and CE has the potential to be used in the silage of indigo waste.
Citation: Nirawan Gunun, Chatchai Kaewpila, Waroon Khota, Pongsatorn Gunun. Investigation of the effect of different additives on the qualities, in vitro degradation, and rumen fermentation profile of indigo waste silage[J]. AIMS Agriculture and Food, 2024, 9(1): 169-182. doi: 10.3934/agrfood.2024010
[1] | Shuaiyu Bu, Yuanyuan Li, Guoqiang Liu, Yifan Li . MAET-SAM: Magneto-Acousto-Electrical Tomography segmentation network based on the segment anything model. Mathematical Biosciences and Engineering, 2025, 22(3): 585-603. doi: 10.3934/mbe.2025022 |
[2] | Tong Sun, Xin Zeng, Penghui Hao, Chien Ting Chin, Mian Chen, Jiejie Yan, Ming Dai, Haoming Lin, Siping Chen, Xin Chen . Optimization of multi-angle Magneto-Acousto-Electrical Tomography (MAET) based on a numerical method. Mathematical Biosciences and Engineering, 2020, 17(4): 2864-2880. doi: 10.3934/mbe.2020161 |
[3] | Hui Yao, Yuhan Wu, Shuo Liu, Yanhao Liu, Hua Xie . A pavement crack synthesis method based on conditional generative adversarial networks. Mathematical Biosciences and Engineering, 2024, 21(1): 903-923. doi: 10.3934/mbe.2024038 |
[4] | Xing Hu, Minghui Yao, Dawei Zhang . Road crack segmentation using an attention residual U-Net with generative adversarial learning. Mathematical Biosciences and Engineering, 2021, 18(6): 9669-9684. doi: 10.3934/mbe.2021473 |
[5] | Peng Zhang, Mingfeng Jiang, Yang Li, Ling Xia, Zhefeng Wang, Yongquan Wu, Yaming Wang, Huaxiong Zhang . An efficient ECG denoising method by fusing ECA-Net and CycleGAN. Mathematical Biosciences and Engineering, 2023, 20(7): 13415-13433. doi: 10.3934/mbe.2023598 |
[6] | Si Li, Limei Peng, Fenghuan Li, Zengguo Liang . Low-dose sinogram restoration enabled by conditional GAN with cross-domain regularization in SPECT imaging. Mathematical Biosciences and Engineering, 2023, 20(6): 9728-9758. doi: 10.3934/mbe.2023427 |
[7] | Tong Shan, Jiayong Yan, Xiaoyao Cui, Lijian Xie . DSCA-Net: A depthwise separable convolutional neural network with attention mechanism for medical image segmentation. Mathematical Biosciences and Engineering, 2023, 20(1): 365-382. doi: 10.3934/mbe.2023017 |
[8] | Chen Yue, Mingquan Ye, Peipei Wang, Daobin Huang, Xiaojie Lu . SRV-GAN: A generative adversarial network for segmenting retinal vessels. Mathematical Biosciences and Engineering, 2022, 19(10): 9948-9965. doi: 10.3934/mbe.2022464 |
[9] | Bingyu Liu, Jiani Hu, Weihong Deng . Attention distraction with gradient sharpening for multi-task adversarial attack. Mathematical Biosciences and Engineering, 2023, 20(8): 13562-13580. doi: 10.3934/mbe.2023605 |
[10] | Xiaoyan Zhang, Mengmeng He, Hongan Li . DAU-Net: A medical image segmentation network combining the Hadamard product and dual scale attention gate. Mathematical Biosciences and Engineering, 2024, 21(2): 2753-2767. doi: 10.3934/mbe.2024122 |
Natural indigo dye production produces indigo waste as a by-product. Our purpose of this study was to examine the effects of calcium hydroxide (Ca(OH)2), cellulase (CE), molasses (MO), and their combinations on the silage quality, in vitro degradability, and rumen fermentation parameters of indigo waste silage. A completely randomized design (CRD) was used for the experiment. Indigo waste was chopped and ensiled in a small-scale silo with no additive (control), Ca(OH)2, MO, CE, Ca(OH)2:MO, Ca(OH)2:CE, MO:CE, and MO:CE:Ca(OH)2. After 30 days of storage, the silages were tested for quality and chemical composition, as well as an in vitro fermentation. The ruminal fluid inoculum was collected from two beef cattle with a body weight (BW) of 200±10 kg, and the inoculum had been pre-heated before being transported to the laboratory. Silage with MO, CE, or their combination increased the amount of lactic acid (p < 0.01). The silage pH was lowest in MO:CE (4.5) and was highest in Ca(OH)2:CE (10.6) in indigo waste (p < 0.01). In comparison to the control (19.5% CP), the CP content of all additives increased by 20.7% to 21.5% (p = 0.02). The addition of Ca(OH)2:MO and Ca(OH)2:CE resulted in a reduction of NDF content by 60.7% and 59.4%, respectively, in comparison to the control group (72.4%) (p < 0.01). Silage with additives had no effect on the cumulative gas production or gas kinetics, except that the constant rate of gas production for the insoluble fraction (c) was higher in MO (p = 0.03). In vitro dry matter degradability (IVDMD) was higher in CE and MO and highest in MO:CE-treated silage (p < 0.01). The in vitro organic matter degradability (IVOMD) increased in Ca(OH)2:MO compared with the control (p = 0.03). The additives alone or in their two combinations in silage reduced the ruminal ammonia-nitrogen (NH3-N) concentration (28.0 to 31.5 mg/dL) when compared to the control (32.7 mg/dL) (p < 0.01). In addition, the highest total volatile fatty acid (VFA) level was found in the silage of the MO (92.9 mmol/L) compared with the control (71.3 mmol/l) (p < 0.01). The proportion of propionic acid and butyric acid increased (p < 0.01) whereas acetic acid decreased (p < 0.01) in the rumen of silage with MO and CE. In summary, the addition of MO and CE has the potential to be used in the silage of indigo waste.
Magneto-Acousto-Electrical Tomography (MAET) integrates the advantages of ultrasound imaging and electrical impedance imaging, representing a promising electro-characteristic imaging technique with broad clinical application prospects. It has demonstrated significant potential, particularly in early diagnosis of diseases such as breast cancer and liver cancer [1]. However, as a complex coupled imaging method, effectively mitigating the impact of noise on MAET images is undoubtedly a critical challenge. Interference generated by experimental equipment and environmental white noise can potentially degrade image quality [2]. Crucially, the electrical conductivity of normal biological tissue is as low as 0.2 S/m or even lower. To further detect signals equivalent to the conductivity of biological tissues, it is necessary to reduce the conductivity of the tissues being examined. However, when the conductivity of these tissues decreases, the signal becomes more susceptible to environmental or experimental noise. This underscores the pressing need to enhance image quality against a backdrop of weak electrical signals. Therefore, effective denoising of magneto-acousto-electrical images not only enhances image quality but also more accurately reflects the actual conditions within the patient's body, which is of significant importance for improving the application effectiveness of MAET technology in the medical field. We will explore the feasibility and efficacy of using deep learning algorithms for magneto-acousto-electrical image denoising, with the aim of providing new perspectives and methods for current medical imaging research.
In recent years, numerous scholars have dedicated their efforts to enhancing the quality of magneto-acousto-electrical images. In previous studies, researchers have attempted to improve image quality through various methods such as adjusting the signal pattern and energy of the ultrasonic excitation source [3,4,5,6,7,8,9,10], and enhancing the intensity of the static magnetic field [11,12,13,14,15,16], among others. Additionally, some studies have focused on the characteristics of MAET signals, adopting wavelet filtering methods to enhance the signal-to-noise ratio and utilizing the reciprocity theorem to derive the fluctuation equation of the detection signal. This equation, combined with the time reversal imaging algorithm, allows for more precise imaging of targets with low conductivity using the filtered signals [17]. However, despite some progress, current denoising methods still exhibit significant limitations due to the unique properties of magneto-acousto-electrical images, such as their weak electrical signals and complex noise environments. Specifically, existing denoising techniques lack effective modeling capabilities for local and global features of magneto-acousto-electrical images and are unable to extract the most relevant multi-scale contextual information to model the joint distribution of clean and noisy image pairs. However, by constructing such a joint distribution, the model can more accurately understand and depict the noise patterns in the image, enabling the denoising algorithm to more effectively distinguish between noise and useful signals. Moreover, this approach can capture the characteristics and variations of the image at different scales, which is crucial for handling complex noise environments and preserving detailed information in the image. Therefore, finding more effective denoising strategies to further enhance the quality of magneto-acousto-electrical images remains an important goal in current research.
In the field of image denoising, methods based on deep learning have achieved significant accomplishments in recent years [18,19,20]. However, training these deep learning models requires a large number of pairs of clean and noisy images, the collection of which is both time-consuming and costly. To address this issue, researchers have proposed various noise generation techniques to simulate more pairs of clean and noisy images, thereby facilitating the training of deep learning models. The core idea of these methods primarily involves utilizing Generative Adversarial Networks (GAN) [21] to directly learn the noise distribution f(v) [22,23]. However, these methods face challenges when simulating complex noise images, where the noise level and characteristics vary with the signal and may differ across multiple dimensions.
To address these issues, we propose a Dual Generative Adversarial Network Based on Attention Residual U-Net (ARU-DGAN), which has been successfully applied to magneto-acousto-electrical image denoising. By approximating the joint distribution of magneto-acousto-electrical clean and noisy images from both the perspectives of noise removal and noise generation, we propose an effective method that leverages attention residual U-Net to learn image information and extract the most relevant multi-scale contextual information. The major contributions of this paper are as follows:
1) We propose a novel Dual Generative Adversarial Network (DGAN) architecture that allows the model to approximate the joint distribution f(u,v) of magneto-acousto-electrical clean and noisy images from two perspectives: Noise removal and noise generation, within a unified Bayesian framework as illustrated in Figure 1. More crucially, by using the additional clean and noisy image pairs simulated by our trained generator, we can expand the scale of the training set, thereby further enhancing the denoising performance of the model.
2) We design an Attention Residual U-Net (ARU) as the backbone for both the denoiser and generator within the DGAN framework. The ARU architecture employs a residual mechanism and incorporates the proposed linear Self-Attention based on Cross-Normalization (CNorm-SA). This design enables the model to maintain high resolution while efficiently extracting the most relevant multi-scale contextual information, thus enhancing its capability to model local and global features of magneto-acousto-electrical images.
3) We build a Magneto-Acousto-Electrical Imaging (MAEI) dataset, aiming to establish a standardized benchmark for the field of magneto-acousto-electrical image denoising. To validate the practical performance of ARU-DGAN, we conduct extensive experiments on the MAEI dataset. The experimental findings underscore the exceptional ability of ARU-DGAN in restoring sharp edges and detailed textures, resulting in denoised outputs that closely resemble real-world scenarios. Furthermore, ARU-DGAN outperforms the state-of-the-art competitive methods in denoising effects, with an increase of 0.3 dB in PSNR and a 0.47% improvement in SSIM.
The remainder of this paper is organized as follows. In Section 2, a brief review of the relevant literature on magneto-acousto-electrical image processing and image denoising is provided. In Section 3, the overall architecture and individual modules of the proposed model are described in detail. Section 4 presents experiments conducted on a real-world dataset of magneto-acousto-electrical images to validate the effectiveness of the proposed model. Finally, in Section 5, we conclude the paper and discuss future directions.
The fundamental principle of MAET involves positioning the target object within an ultrasonic field, which induces local ions to oscillate in tandem with the propagation of ultrasonic waves. These oscillating ions are subjected to the Lorentz force within a magnetic field, leading to ion separation and the generation of a localized electric field. Concurrently, electrodes attached to the imaging body receive electrical signals, which are further utilized for the realization of electrical property imaging.
Many teams have made significant contributions to the MAET process. Initially, MAET was referred to as Hall Effect Imaging, first proposed by Han et al. [2]. Their research primarily focused on imaging the interfaces between regions of differing conductivities. Subsequently, some scholars explored the gradient changes in conductivity by studying the correlation between ultrasound and conductivity images [24]. However, due to the interaction of multiple physical fields such as sound field, electric field and magnetic field, the signal amplitude is extremely low, only at the μV level. Moreover, interference in the experimental system and environmental noise may lead to a reduction in the signal-to-noise ratio.
In order to enhance the imaging quality of magneto-acousto-electrical images, researchers have conducted in-depth theoretical and applied explorations from multiple perspectives. These explorations include adjusting the signal pattern and energy of the ultrasonic excitation source, enhancing the intensity of the static magnetic field and employing strategies such as filtering algorithms. Specifically, the evolution of the ultrasonic excitation source includes a transition from differential frequency signal excitation [15] and linear frequency modulation [14] to the use of single pulse excitation [3,4]. This transition not only optimized the generation of ultrasound waves but also improved their propagation effects within objects. The ultrasonic transducer has also undergone a technical upgrade from planar transducers [16] to focused transducers [5,6,7]. This advancement allows for more precise localization and irradiation of the target area, thereby enhancing the quality of imaging. In terms of detection, we have progressed from being able to use only uniform static magnetic field stimulation to now being capable of imaging under non-uniform static magnetic field excitation [25]. As for the detection mode, researchers have shifted from using coils [8,9] to electrodes [10,11,12,26]. This transition has improved the efficiency of signal reception and contributed to enhancing the signal-to-noise ratio. Moreover, in accordance with the characteristics of MAET signals, researchers have also introduced wavelet filtering methods to effectively boost the signal-to-noise ratio, thereby improving image quality [17].
Image denoising is a significant research direction in the field of computer vision, aiming to recover clear images from those contaminated with noise. Traditional image denoising strategies largely rely on prior knowledge of the image, including but not limited to sparsity [27,28], low-rank [29], self-similarity [30,31] and smoothness [32,33]. However, these priors are severely constrained when dealing with image denoising tasks under extreme conditions, making it exceptionally challenging to denoise severely corrupted images. Furthermore, discriminative learning methods have provided new insights and directions for image denoising research. These methods mainly include Markov Random Fields (MRF) [34,35,36], Cascade of Shrinkage Fields (CSF) [37,38] and Trainable Nonlinear Reaction Diffusion (TNRD) [39] approaches. By learning the distribution characteristics of data, these methods attempt to establish more accurate noise models, thereby achieving more effective image denoising.
In recent years, image denoising methods based on deep learning have achieved significant breakthroughs in the field of image denoising. For instance, Jain and Seung [40] first employed a five-layer network for denoising tasks, while Burger et al. [41] used a simple Multi-Layer Perceptron (MLP) for image denoising, successfully achieving performance comparable to the BM3D algorithm. Zhang et al. [20] proposed the convolutional denoising network DnCNN, which achieved state-of-the-art performance in Gaussian denoising. In addition, numerous other network architectures have been designed and applied to image denoising tasks, including but not limited to RED [42], NLRN [43], N3Net [44], RIDNet [18], VDN [19] and DANet [45]. Moreover, in the field of image segmentation, Mu et al. [46] proposed an attention-based residual U-Net method to learn how to segment intracranial aneurysms through various preprocessing and geometric post-processing techniques. On the other hand, Liu et al. [47] proposed a dual-branch network based on Transformer and convolution for retinal vessel segmentation in OCTA images. These image segmentation approaches provide valuable insights for the image denoising method ARU-DGAN proposed in this paper.
Moreover, for image denoising tasks affected by uncertainty and/or imprecision, some studies suggest to exploit fuzzy image preprocessors based on fuzzy logic [48]. This approach proposes a blur method based on fuzzy similarity computation, which has very low computational complexity and can effectively handle images with blurry content to approximate the real noise environment more accurately.
Similar to most supervised deep learning denoising methods, our approach is constructed based on a given training dataset, which contains a large number of pairs of magneto-acousto-electrical clean and noisy images. Our learning objective is not to rigidly force the model to learn the mapping from noisy image v to clean image u, but rather to approximate the latent joint distribution f(u,v) between the clean and noisy image pairs. Next, we will introduce our method from a Bayesian perspective.
This section decomposes the joint distribution f(u,v) of magneto-acousto-electrical clean and noisy image pairs from two different perspectives [49]. First, we approach from the perspective of noise removal, focusing on minimizing the impact of the noisy image to restore a more accurate clean image. By modeling the relationship between the noisy and clean images, we aim to enhance the performance and effectiveness of the denoiser. Second, we delve into the generation of noise, studying how to produce corresponding noisy images from clean ones. Such analysis aids in better simulating and understanding the characteristics of noise in real images. By comprehending the generation process and features of noise, we can more accurately evaluate and improve the generator.
Noise removal: In Bayesian inference, we aim to deduce the probability distribution of the corresponding clean image u through the observed magneto-acousto-electrical noise image v. The conditional distribution f(u|v) represents the probability distribution of the clean image u given the noisy image v. However, since the actual distribution f(u|v) is often difficult to model in practice, we have designed a denoiser N, which approximates the real distribution f(u|v) by learning the mapping relationship from the magneto-acousto-electrical noise image v to the clean image u.
Through the training process, the denoiser N learns an implicit distribution fN(u|v) that approximates the real distribution f(u|v) as closely as possible. This allows it to generate a corresponding clean image u when given a magneto-acousto-electrical noise image v. Therefore, the output of the denoiser N can be viewed as an image sampled from this learned implicit distribution fN(u|v).
With this understanding, we can obtain the pseudo magneto-acousto-electrical clean-noise image pair (ˆu,v) as follows:
ˆu=N(v),v∼f(v)⇒(ˆu,v) | (1) |
This can be viewed as an instance sampled from the pseudo joint distribution fN(u|v). Clearly, the better the performance of the denoiser N, the higher the accuracy with which it approximates the real joint distribution f(u|v).
fN(u,v)=fN(u|v)f(v) | (2) |
Noise generation: In real magneto-acousto-electrical imaging systems, image noise primarily originates from interference within the experimental system and white noise in the environment. To more comprehensively describe the generation process from clean magneto-acousto-electrical images u to noisy images v, we introduce an additional latent variable i. This latent variable i represents the random noise in the magneto-acousto-electrical imaging system. Therefore, the noise generation process can be characterized by the conditional distribution f(v|u,i). In this task, the role of the generator G is to learn the implicit distribution fG(v|u,i) to approximate the real distribution f(v|u,i) as closely as possible. Hence, the output of the generator G can be considered as instances sampled from fG(v|u,i), that is, G(u,i)∼fG(v|u,i). Similar to Eq (1), we can obtain the pseudo pair of magneto-acousto-electrical clean-noise image pair (u,ˆv) as follows:
u∼f(u),i∼f(i),ˆv=G(u,i)⇒(u,ˆv) | (3) |
By introducing the latent variable i and using the generator G to approximate the real conditional distribution f(v|u,i), we can better understand and simulate the image noise phenomenon in magneto-acousto-electrical imaging. Theoretically, the latent variable i can be marginalized to obtain the following pseudo joint distribution fG(u,v), which serves as an approximation to the real joint distribution f(u,v):
fG(u,v)=∫ifG(v|u,i)f(u)f(i)di≈1M∑MmfG(v|u,im)f(u) | (4) |
As suggested in [50], we can set the number of samples M to 1, provided that the batch size is sufficiently large. Under this setting, the pseudo pair of clean and noisy images (u,ˆv) obtained through the generation process of Eq (3) can be approximated as a sample drawn from the pseudo joint distribution fG(u,v). Such a sampling process can effectively simulate the correlation between real images and noise, thereby providing beneficial training samples for the noise removal task. Using these samples, we can better train the generator to more accurately restore clean images and remove noise, thereby improving the model's performance and robustness.
In the preceding section, two pseudo joint distributions, namely fN(u,v) and fG(u,v), are derived from the perspectives of noise removal and noise generation. The critical question now is how to effectively train the denoiser N and generator G so that they can approximate the real joint distribution f(u,v) well. We can control the training process through the sampling process defined in Eqs (1) and (3), thereby making it possible to use methods similar to GAN [21]. This approach approximates the real joint distribution by gradually optimizing the two pseudo joint distributions. Specifically, we articulate this idea as a dual generative adversarial problem inspired by Triple-GAN [51].
minN,GmaxDLDGAN(N,G,D)=E(u,v)[D(u,v)]−αE(ˆu,v)[D(ˆu,v)]−(1−α)E(u,ˆv)[D(u,ˆv)] | (5) |
where ˆu=N(v), ˆv=G(u,i). D represents the discriminator, whose primary task is to distinguish between real clean-noise image pairs (u,v) and pseudo image pairs, i.e., (ˆu,v) and (u,ˆv). The hyperparameter α is used to adjust the relative weights between the denoiser N and generator G. In DGAN, the discriminator D attempts to differentiate the distribution of real images from the distribution of images generated by the denoiser N and generator G. Compared to traditional distance measures such as Jensen-Shannon (JS) divergence or Kullback-Leibler (KL) divergence, the Wasserstein distance can better address the vanishing gradient problem in certain cases and it provides more stable results when there are overlapping areas between distributions [52]. Therefore, we use the Wasserstein-1 distance as the loss function to make the images generated by the denoiser N and generator G closer to the real distribution, thereby enhancing the performance and stability of DGAN.
The working mechanism of DGAN is illustrated in Figure 1. The denoiser N aims to approximate the joint distribution fN(u,v) in Eq (2) by passing the conditional distribution fN(u|v). Furthermore, the generator G targets approximating the joint distribution fG(u,v) in Eq (4) by passing the conditional distribution fG(v|u,i). Through the adversarial action of the discriminator D, the denoiser N and generator G gradually optimize during the training process, making their pseudo joint distributions fN(u,v) and fG(u,v) progressively approach the real joint distribution f(u,v). This adversarial training mechanism allows the denoiser and generator to learn from each other's information, thereby better simulating the joint distribution between real images and noise images.
In addition, we employ a dual regularization between the denoiser N and the generator G to mutually enhance their capabilities. During the training process, for any given real noisy-clean image pair (u,v) as well as the pseudo image pair (ˆu,v) generated by the denoiser N or the pseudo image pair (u,ˆv) produced by the generator G, the discriminator D is updated based on the adversarial loss. Subsequently, we fix the parameters of the discriminator D and simultaneously update the denoiser N and the generator G. This implies that in each iteration, the denoiser N and the generator G maintain interaction and guide each other's optimization process. Through this adversarial training approach, the denoiser and generator are able to collaborate, gradually improving the performance of image denoising and noise generation, while also enhancing the robustness of the model.
In the overall architecture of DGAN, the denoiser N, generator G and discriminator D are all parameterized through deep neural networks. As shown in Figure 1, the denoiser N takes the noisy image v as input and generates the denoised image ˆu. The generator G, on the other hand, takes the clean image u and latent variable i as inputs to generate an image ˆv with simulated noise. To construct the denoiser N and generator G, we adopt the attention residual U-Net architecture proposed in this paper as the backbone (see Section 3.2.1 for details). In addition, both employ a residual learning strategy [20] to enhance the model's performance and training efficiency. The discriminator D consists of a series of stride convolutional layers and a fully connected layer, which serve to reduce image size and fuse information, aiding in distinguishing between real and generated images. Through the collaborative action of these networks, DGAN demonstrates greater effectiveness in image denoising and noise generation tasks.
As previously mentioned, DGAN comprises three major components: The denoiser N, generator G and discriminator D. Starting from this section, we will provide a detailed description of the model structure for these components.
Inspired by U-Net [53], we propose a novel Attention Residual U-Net (ARU) as the backbone architecture for both the denoiser N and generator G. Figure 2 illustrates the overall structure of the ARU network, where din and dout represent the number of input and output channels, respectively. "Conv (k, s, p)" denotes the convolution operation with a kernel size of (k,k), stride of s and padding of p. Similarly, "TransConv (k, s, p)" refers to the transposed convolution operation with a kernel size of (k,k), stride of s and padding of p. As can be seen from the figure, the ARU network primarily consists of three parts.
1) Global Feature Extraction Layer. This layer transforms the input feature map x(H×W×din) into a global feature map g1(x) using the linear self-attention operation based on cross-normalization proposed in this paper (see Section 3.2.2 for details). Through the processing of the global feature extraction layer, the original input image can be converted into a more enriched and high-dimensional global feature map, providing beneficial feature representations for subsequent processing steps.
2) Based on the global feature map g1(x), we adopt a symmetric encoder-decoder structure of the residual U-Net for learning to extract and encode the most relevant multi-scale contextual information F(g1(x)), as shown in Figure 2. Within this network structure, we utilize progressively downsampled feature maps to extract multi-scale features. During the step-by-step upsampling process, the output feature map is concatenated with the feature map before upsampling, and after convolution operations, it is progressively encoded into a high-resolution feature map. This process aids in mitigating the potential loss of fine details that may result from direct large-scale upsampling. Through this structure, we are able to extract the most relevant multi-scale contextual features, thereby enabling the model to better capture the rich local and global information within the image. Local features are crucial for capturing subtle structures and detail information in the image, while global features assist in understanding the overall semantics and structure of the image. In tasks of image denoising and noise generation, the extraction of such multi-scale features is particularly critical.
3) Residual connections that fuse global features and multi-scale features through addition or subtraction operations: g1(x)±F(g1(x)), where g1(x) represents the global features obtained from the input feature x through the global feature extraction layer, and F(g1(x)) represents the multi-scale features processed by the symmetric encoder-decoder structure of the residual U-Net after extracting global features. Specifically, the corresponding operation processes for the denoiser N and generator G are as follows:
N(v)=g1(v)−F(g1(v)) | (6) |
G(u,i)=g1(u)+F(g1(u,i)) | (7) |
We name this design as attention residual U-Net and compare it with the traditional residual blocks [54] to elucidate the underlying intuition.
In the original residual block, the operation can be summarized as RES(x)=x+g2(g1(x)), where RES(x) represents the desired mapping of the input feature x, and g1 and g2 represent the weight layers of the convolution operation. The primary design difference between ARU and traditional residual blocks is that ARU replaces the ordinary convolution weight layer with a symmetric encoder-decoder structure of the residual U-Net and replaces the original feature x with the global feature g1(x) transformed through the CNorm-SA: ARU(x)=g1(x)±F(g1(x)), where F represents the ARU structure shown in Figure 2. Such a design enables the network to directly extract the most relevant multi-scale contextual features from each residual block, thereby enhancing the network's understanding of the image.
In order to extract the most task-relevant multi-scale features within the ARU network, we propose a novel linear Self-Attention based on Cross-Normalization (CNorm-SA). The design of this mechanism aims to mitigate the excessive dependency on initial weights. Furthermore, CNorm-SA eliminates the nonlinearity by replacing the Softmax non-linear activation function and altering the operation sequence, thereby reducing the computational complexity of this module to O(H×W), where H and W represent the height and width of the original input feature map respectively. Consequently, our model can efficiently handle high-resolution inputs, leading to enhanced performance in image processing tasks.
For an input feature map, the formula for the traditional self-attention mechanism is as follows [55]:
Q=xWQ,K=xWK,V=xWV | (8) |
Att(x)=Softmax(QKT√dk)V | (9) |
Where Q, K and V represent the Query, Key and Value matrices generated by linear transformations on the input feature map, respectively, and Wq∈Rdin×dk, Wk∈Rdin×dk and Wv∈Rdin×dout are weight matrices.
As shown in Figure 3, within CNorm-SA, we introduce the CNorm operation and eliminate the Softmax non-linear activation function. Simultaneously, this self-attention mechanism allows the module to compute KTV first, then multiply it with Q. The computational and storage complexity of this operation is O(H×W), thus the process is linearly related to the size of the input feature map.
Specifically, CNorm-SA is defined as follows:
Att(x)=CNormRow(Q)(CNormCol(KTV)) | (10) |
CNorm(a)=βa√‖a‖2+ϵ | (11) |
where a represents a vector and β is a learnable parameter. ϵ represents a positive number close to zero, typically chosen to be very small, such as 1e−8. This setting ensures that the denominator does not become zero during the computation process. CNormRow or CNormcol respectively denote the application of cross-normalization operation to a matrix row-wise or column-wise, that is:
Att(x)=[ˆq0⋅ˆo0⋯ˆq0⋅ˆodout⋮⋱⋮ˆqH×W⋅ˆo0⋯ˆqH×W⋅ˆodout] | (12) |
ˆqj=CNorm(Qj:) | (13) |
ˆoj=CNorm((KTV):j) | (14) |
According to Eq (12), the relational feature can be defined as the cosine similarity between q and o. To ensure the effectiveness of the relational feature, we employ the CNorm operation to constrain q and o to unit vectors and limit their magnitudes within a finite range through regularization. This treatment prevents their values from having a suppressive effect on the relational feature. Without such handling, the scope of attention would depend on initialization, leading to instability in the attention mechanism.
In the architecture of GAN [21], the discriminator plays a crucial role. Its primary task is to distinguish real and fake instances, providing guidance for the generator to move in the correct direction of generation. In the framework we propose, we adopt the discriminator structure widely used in literature [56,57]. This structure consists of a series of convolutional layers and a fully connected layer, which are used to gradually reduce the feature size and fuse the extracted features. Figure 4 provides a detailed depiction of the overall design of the discriminator. The numbers next to each feature map represent its spatial dimensions and depth. The input to the discriminator is a pair of concatenated magneto-acousto-electrical images with dimensions of 128 ⊆ 128 ⊆ 6. After processing by the discriminator, a scalar value is outputted. This scalar value can be interpreted as the discriminator's assessment of the authenticity of the input image pair, thereby guiding the denoiser and generator for more precise image denoising and noise generation.
In previous studies [58,59], scholars found that combining adversarial loss with traditional loss functions could effectively accelerate and stabilize the training process of GANs. In our image denoising task, we choose to use L1 loss, i.e., ‖ˆu−u‖1, which can make the output of the denoiser N closer to the real image. However, for the generator G, due to the randomness of noise, directly applying L1 loss may not bring the expected effect. Therefore, we apply the L1 constraint to the statistical features of the noise distribution:
‖G(ˆv−u)−G(v−u)‖1 | (15) |
where G(⋅) represents the Gaussian filter used to extract the first-order statistical information of noise. By integrating these two regularization factors into the adversarial loss in Eq (5), we can obtain the final objective function:
L=minN,GmaxDLDGAN(N,G,D)+γ‖ˆu−u‖1+η‖G(ˆv−u)−G(v−u)‖1 | (16) |
where γ and η represent the hyperparameters balancing the losses of the denoiser and generator.
In the objective function defined by Eq (16), three key components need to be optimized: The denoiser N, the generator G and the discriminator D. This is consistent with most methods in GAN-based research literature [21,51,52], i.e., jointly training N, G and D. In implementing the training process, we first fix the denoiser N and the generator G, then update the parameters of the discriminator D. Next, while keeping the discriminator D and one other component fixed, we sequentially update the parameters of the denoiser N and the generator G. This alternating update strategy aids in balancing the learning progression among different components, thereby enhancing the overall performance of the model. To ensure the stability of the training process, we draw upon the gradient penalty technique from WGAN-GP [56]. By introducing an additional gradient penalty term, the discriminator is forced to satisfy the 1-Lipschitz constraint, which in turn improves the stability and effectiveness of the model.
After training, the generator G has acquired the ability to simulate additional noise images given any magneto-acousto-electrical clean image. This capability allows us to augment the training dataset by incorporating a large number of magneto-acousto-electrical clean images produced by generator G along with their corresponding noise images, thereby retraining the denoiser N. This process not only enhances the diversity of the training dataset but also aids in further optimizing the performance of the denoiser N, enabling it to better adapt to various noise environments.
The algorithm complexity of ARU-DGAN can be calculated by analyzing the complexities of its three major components, namely the denoiser N, generator G and discriminator D.
1) The denoiser N and the generator G both utilize the ARU network as the backbone architecture. The ARU network consists of key components such as a global feature extraction layer and a symmetric encoder-decoder structure of the residual U-Net. Specifically, the global feature extraction layer employs CNorm-SA operations. By replacing the softmax non-linear activation function and altering the operation sequence, CNorm-SA eliminates the non-linearity in the self-attention mechanism, thereby reducing the computational complexity of this module to O(H×W), where H and W represent the height and width of the input feature map, respectively. In the symmetric encoder-decoder structure of the residual U-Net, the upsampling and downsampling processes are composed of a series of convolutional layers. Assuming the input feature map size is H×W×din, the kernel size is K×K and the output channel number is dout, the computational complexity of a single convolution operation is given by H×W×din×K×K×dout, where din represents the number of input channels. Based on the settings of kernel size, input and output channel numbers in each convolutional layer as shown in Figure 2, the main computational cost of the convolution operations is determined by the size of the input feature map, H×W. Therefore, the algorithm complexity of the symmetric encoder-decoder structure of the residual U-Net is also O(H×W). In summary, both the denoiser N and the generator G have an algorithm complexity of O(H×W).
2) As shown in Figure 4, the discriminator D consists of a series of convolutional layers and a fully connected layer. Based on the above analysis, the algorithm complexity of the discriminator D is mainly determined by the convolutional layers, thus being O(H×W).
In summary, the overall algorithm complexity of ARU-DGAN is O(H×W), which is directly proportional to the size of the input feature map.
To comprehensively evaluate the proposed ARU-DGAN model, we conducted a series of experiments on a real-world magneto-acousto-electrical image dataset. These experiments aim to answer the following key research questions:
Q1: How does the performance of ARU-DGAN compare with current image denoising methods?
Q2: Do the core components such as the generator G, ARU network and CNorm-SA play a crucial role in enhancing the performance of ARU-DGAN?
In order to propel research and development in the field of magneto-acousto-electrical image processing and to provide a standard benchmark testing platform for academia, we conducted a large number of real-world measurements and constructed a Magneto-Acousto-Electrical Image (MAEI) dataset. This dataset not only includes magneto-acousto-electrical images from various real-world environments but also reflects the impact of environmental and experimental noise on image quality. By utilizing this dataset, we are able to validate the performance of ARU-DGAN under real-world conditions and ascertain its potential and applicability in the task of magneto-acousto-electrical image denoising.
In the MAEI dataset, it comprises 50 sets of magneto-acoustic-electric clean images and their corresponding noisy image pairs, all of which are obtained from real measurements. Each original image has a size of 3968 ⊆ 3968 pixels. To further augment the dataset and increase the diversity of samples, we adopted a cropping strategy: each original image was cropped into 100 patches of 512 ⊆ 512 pixels with a stride of 384 pixels. Through this approach, we successfully expanded the scale of the dataset to 5000 pairs of magneto-acoustic-electric images. This augmentation strategy not only elevated the order of magnitude of the dataset but also enriched the diversity of image samples, thereby aiding us in more comprehensively and accurately evaluating and optimizing the performance of ARU-DGAN.
In the measurement experiments, each real magneto-acoustic-electric noise image was obtained through precise measurements on specific phantoms. These phantoms possess low conductivity characteristics with a conductivity value of 0.2 S/m and they contain anomalies that we preset internally. This measurement method enabled us to generate a series of real and representative magneto-acoustic-electric noise images. These images reflect the conductivity distribution inside the phantom and also reveal the existence and location of the anomalies.
In the preparation phase of the experiment, we first prepared the required phantoms. This process involved dissolving an appropriate amount of sodium chloride in water to form a specific solution. To accurately measure the conductivity value of this solution, we employed the Zurich Instruments MFIA device for detection. Next, we mixed agar with the aforementioned solution at a ratio of 1 g/100 ml of water. This mixture was subsequently heated to boiling and cooled to solidify, successfully creating the phantom. This phantom served as the foundation of our experiment, providing an environment that simulates real biological tissues. Within this phantom, we designed and set anomalies of different sizes and shapes, including circular, elliptical and rectangular forms. The purpose of these anomalies is to simulate potential tumor changes within biological tissues.
In the experimental process, we initially applied a single pulse signal with a center frequency of 0.5 MHz to the ultrasonic transducer. This procedure generates ultrasonic waves that penetrate the phantom in the designated direction of the transducer. Subsequently, under the influence of the static magnetic field, magneto-acousto-electrical signals are produced. These signals are captured and measured by electrodes and then amplified by 56 dB to enhance their intensity and detectability. Finally, these amplified signals are collected by the NI data acquisition card. By varying the number, position, orientation and size of the anomalies, we were able to generate multiple magneto-acousto-electrical noise images. These images not only reflect the distribution of electrical conductivity within the phantom but also reveal the presence and characteristics of the anomalies.
In the MAEI dataset, the clean images are obtained through a series of processing steps. Initially, the noise images corresponding to the clean images were preprocessed in the measurement experiment using the filtering means described in [17]. Subsequently, these processed images were further optimized and adjusted by medical professionals, resulting in the clean images present in our dataset.
To validate the effectiveness of our proposed method in the task of magneto-acousto-electrical image denoising, we compared it with several advanced competing methods as follows:
1) WNNM [29]: We discuss the Weighted Nuclear Norm Minimization (WNNM) problem and applies it to image denoising.
2) CBDNet [60]: Convolutional Blind Denoising Network (CBDNet) is an advanced deep learning method that enhances denoising effects by predicting noise levels and incorporating noise uncertainty mapping.
3) RIDNet [18]: Real Image Denoising Network (RIDNet) is a single-stage blind denoising method that adopts a modular architecture and utilizes residual structures and feature attention mechanisms.
4) VDN [19]: Variational Denoising Network (VDN) is a novel blind image denoising method that integrates noise estimation and image denoising into a unique Bayesian framework, which can be flexibly used for estimating and eliminating complex non-independent and identically distributed noise collected in real scenarios.
5) DANet [45]: Dual Adversarial Network (DANet) is a new unified framework capable of handling both noise removal and noise generation tasks by learning the joint distribution of clean-noise image pairs, rather than merely inferring the posterior distribution of the underlying clean image.
To evaluate the performance of image denoising tasks, we employed two widely used metrics: Peak Signal-to-Noise Ratio (PSNR) [61] and Structural Similarity Index (SSIM) [62]. These two metrics assess image quality from different perspectives.
PSNR is a commonly used metric for assessing image quality, primarily utilized to measure the overall error between the denoised image and the clean image. A higher PSNR indicates less impact from noise. The formula for calculating PSNR is as follows:
PSNR=10⋅log10(MAX2IMSE) | (17) |
MSE=1mn∑m−1i=0∑n−1j=0[I(i,j)−K(i,j)]2 | (18) |
where MAXI represents the possible maximum pixel value (for an 8-bit image, MAXI=255). I and K respectively denote the denoised image and the clean image, while m and n represent the height and width of the image. MSE stands for Mean Squared Error, which is the average of the squared differences between the pixels of the denoised image and the clean image.
SSIM, on the other hand, places more emphasis on measuring the visual quality of the denoised image compared to the clean image. It takes into account the brightness, contrast and structural information of the image, aligning more closely with human subjective perception of image quality. A larger SSIM value indicates higher similarity. The formula for calculating SSIM is as follows:
SSIM(x,y)=(2μxμy+C1)(2σxy+C2)(μ2x+μ2y+C1)(σ2x+σ2y+C2) | (19) |
where x and y represent the denoised image and the clean image respectively, μx and μy are the averages of x and y, σx and σy are the variances of x and y, σxy is the covariance of x and y, and C1 and C2 are stability constants to avoid division by zero.
Both of these metrics can be used to evaluate the effect of image denoising. However, since they focus on different aspects, they are typically used in conjunction in practical applications to obtain a more comprehensive evaluation result.
In the training process of ARU-DGAN, we adopted specific initialization and optimization strategies. Specifically, all convolutional layer weights of the denoiser N and the generator G were initialized according to the Xavier method [63]. This approach ensures that the network weights have an appropriate scale at the beginning of the training, thereby accelerating convergence. The weights of the discriminator D, on the other hand, were initialized from a zero-centered normal distribution with a standard deviation of 0.02 [57].
For network training, we selected the Adam optimizer [64], an adaptive learning rate optimization algorithm that can effectively handle sparse gradients and non-stationary objectives. Furthermore, we trained the model for 70 epochs with learning rates set to 1e−4, 1e−4 and 2e−4 for N, G and D respectively. Moreover, we stipulated that the learning rate would decay linearly by half every 10 epochs. This setting aims to gradually reduce the learning step size as training progresses, allowing the model to fine-tune parameters more delicately when approaching the optimal solution, thereby enhancing the performance of the model.
During the model training phase, we randomly selected 128 ⊆ 128 pixels patches from the input images for training. This method can enhance the robustness of the model as it forces the model to learn a more diverse set of image features. The convolutional layer's kernel size, stride and padding, as well as the dimensions and channel numbers of all input and output feature maps, are thoroughly defined for both the ARU network and the discriminator D. The specific values of these parameters are presented in Figures 2 and 4, respectively. In the optimization process, before each update of the denoiser N and generator G, we first updated the discriminator D three times. This approach helps maintain the stability of the model and prevent oscillations during the training process. In terms of experimental parameter settings, we set γ=1000, η=10 and α=0.5. The setting of α implies that the contributions of the denoiser N and generator G are considered equally important. Additionally, following the default settings of WGAN-GP [56], its penalty coefficient was set to 10. As described in Section 3.4, the trained generator G in ARU-DGAN can augment the original training set by generating more synthetic pairs of magneto-acousto-electrical clean and noisy images. Therefore, we retrained the denoiser N based on the expanded training dataset.
All models were trained using PyTorch [65]. We ensured optimal performance for all baseline models by carefully adjusting the parameters. All experiments were run independently in the same experimental environment with Intel(R) Xeon(R) Platinum 8358P host and NVIDIA Tesla A40-48GB GPU.
To demonstrate the superiority of ARU-DGAN, we compared its results with five baselines on the MAEI dataset, with specific results shown in Table 1. The optimal and suboptimal results are highlighted in bold and underlined, respectively. From the experimental results, it can be observed that:
1) Leveraging the powerful fitting capability of Deep Neural Networks (DNN), deep learning-based methods significantly outperform traditional WNNM methods in terms of performance. This is primarily due to the self-learning and self-optimizing characteristics of deep learning, enabling it to better understand and handle complex image noise.
2) ARU-DGAN outperforms the state-of-the-art competitive methods in denoising effects, with an improvement of 0.3 dB in PSNR and a 0.47% increase in SSIM. The significant improvements of our model over the baseline models can be attributed to two key factors: First, ARU-DGAN employs a dual generative adversarial network, where the synthetic data generated by G actively promotes the training of the denoiser N; second, the major structures of the denoiser N and generator G adopt the attention residual U-Net structure and CNorm-SA mechanism, which help extract more relevant multi-scale features, thereby effectively enhancing the image denoising effect. These innovative designs endow ARU-DGAN with higher accuracy and stability in handling magneto-acousto-electrical image denoising tasks.
Methods | Metrics | |
PSNR | SSIM | |
WNNM | 15.59 | 0.6735 |
CBDNet | 19.32 | 0.7537 |
RIDNet | 19.54 | 0.7654 |
VDN | 20.13 | 0.7664 |
DANet | 20.22 | 0.7636 |
ARU-DGAN | 20.52 | 0.7700 |
Figure 5 presents the results of various methods in terms of visual denoising. As can be seen from the figure, WNNM does not perform well in dealing with noise in magneto-acousto-electrical images. Although CBDNet and RIDNet alleviate some noise during the denoising process, extensive noise remains. VDN and DANet often cause over-smoothing of edges and textures during denoising, resulting in loss of image detail. In particular, DANet loses important information. In contrast, ARU-DGAN excels in restoring sharp edges and detailed textures and its denoising results are closer to the real situation.
To further investigate the contribution of the generator G to the overall performance of the model, we trained ARU-DGAN under conditions without generator G. As shown in Table 2, ARU-DGAN achieved superior performance. This demonstrates that the interactive relationship between the denoiser N and the generator G plays a crucial role in enhancing the performance of the model.
Variants | Metrics | |
PSNR | SSIM | |
ARU-DGAN w/o G | 20.38 | 0.7654 |
ARU-DGAN | 20.52 | 0.7700 |
In this section, our primary goal is to validate the effectiveness of ARU. Specifically, we employ U-Net and Residual U-Net to respectively replace ARU as the backbone structure for the denoiser N and the generator G. Table 3 presents the quantitative results from these ablation studies.
Variants | Metrics | |
PSNR | SSIM | |
U-Net | 20.03 | 0.7529 |
Residual U-Net | 20.21 | 0.7639 |
Attention Residual U-Net | 20.52 | 0.7700 |
The results clearly show that the performance of U-Net is the poorest, while that of Residual U-Net surpasses U-Net. However, their performances are inferior to ARU. The significant improvement of ARU can be attributed to the CNorm-SA mechanism and the residual mechanism employed in its structure. These two mechanisms allow the network to directly extract the most relevant multi-scale contextual features from each residual block for image denoising.
We propose a dual generative adversarial network based on attention residual U-Net for magneto-acousto-electrical image denoising. Specifically, our model simulates the relationship between magneto-acousto-electrical clean and noisy images from two perspectives: First, it maps the noisy image to the clean image through a denoiser; second, it maps the clean image to the noisy image through a generator. Then, we employ a dual adversarial strategy to train both the denoiser and the generator simultaneously. After this, the trained denoiser can be directly applied to actual denoising tasks, or its performance can be further enhanced by simulating new pairs of clean and noisy images using the trained generator. Furthermore, we design an attention residual U-Net to serve as the backbone for the denoiser and generator within the dual generative adversarial network. The ARU network incorporates a residual mechanism and introduces a linear self-attention based on cross-normalization, proposed in this paper. This design allows the model to effectively extract the most relevant multi-scale contextual information while maintaining high resolution, thereby better modeling the local and global features of magneto-acousto-electrical images. Furthermore, the composite loss function and training strategy within the model can better preserve image details during denoising. Finally, extensive experiments on a real-world magneto-acousto-electrical image dataset constructed for this study demonstrate that ARU-DGAN exhibits excellent performance in both quantitative and qualitative analyses.
Despite the impressive performance of our model in experiments, there are certain potential limitations.
1) Our model heavily relies on a substantial amount of training data. The performance of the model might be affected if there is an insufficient number of training samples available in specific application scenarios.
2) While our model has demonstrated significant achievements in the task of magneto-acousto-electrical image denoising, its applicability to other types of images or datasets requires further validation.
In future research, we intend to explore additional data augmentation techniques to address the issue of limited training samples. Furthermore, we plan to test our model on a broader range of image types and datasets to verify its generality and robustness.
The authors declare that they have not used Artificial Intelligence (AI) tools in the creation of this article.
This work was supported in part by the National Natural Science Foundation of China under Grant No. 52377227, 52007182 and 51937010.
The authors declare that there are no conflicts of interest.
[1] |
Senasri N, Sriyasak P, Suwanpakdee S, et al. (2022) Toxicity of indigo dye-contaminated water on silver barbs (Barbonymus gonionotus) and pathology in the gills. EnvironmentAsia 15: 106–115. https://doi.org/10.14456/ea.2022.52 doi: 10.14456/ea.2022.52
![]() |
[2] | Srisamran J, Wimolsujarit B, Supuma Y, et al. (2015) Research and development on indigo in sakonnakhon province. Available from: https://www.doa.go.th/research/attachment.php?aid = 2190. |
[3] |
Pattanaik L, Padhi SK, Hariprasad P, et al. (2020) Life cycle cost analysis of natural indigo dye production from Indigofera tinctoria L. plant biomass: A case study of India. Clean Technol Environ Policy 22: 1639–1654. https://doi.org/10.1007/s10098-020-01914-y doi: 10.1007/s10098-020-01914-y
![]() |
[4] |
Pattanaik L, Naik SN, Hariprasad P (2019) Valorization of waste Indigofera tinctoria L. biomass generated from indigo dye extraction process-potential towards biofuels and compost. Biomass Convers Biorefin 9: 445–457. https://doi.org/10.1007/s13399-018-0354-2 doi: 10.1007/s13399-018-0354-2
![]() |
[5] |
Gunun N, Kaewpila C, Khota W, et al. (2023) The effect of indigo (Indigofera tinctoria L.) waste on growth performance, digestibility, rumen fermentation, hematology and immune response in growing beef cattle. Animals 13: 84. https://doi.org/10.3390/ani13010084 doi: 10.3390/ani13010084
![]() |
[6] |
Kaewpila C, Khota W, Gunun P, et al. (2020) Strategic addition of different additives to improve silage fermentation, aerobic stability and in vitro digestibility of Napier grasses at late maturity stage. Agriculture 10: 262. https://doi.org/10.3390/agriculture10070262 doi: 10.3390/agriculture10070262
![]() |
[7] | Gunun N, Gunun P, Wanapat M, et al. (2017) Improving the quality of sugarcane bagasse by urea and calcium hydroxide on gas production, degradability and rumen fermentation characteristics. J Anim Plant Sci 27: 1758–1765. |
[8] |
So S, Cherdthong A, Wanapat M (2020) Improving sugarcane bagasse quality as ruminant feed with Lactobacillus, cellulase, and molasses. J Anim Sci Technol 62: 648. https://doi.org/10.5187/jast.2020.62.5.648 doi: 10.5187/jast.2020.62.5.648
![]() |
[9] |
Gunun N, Wanapat M, Gunun P, et al. (2016) Effect of treating sugarcane bagasse with urea and calcium hydroxide on feed intake, digestibility, and rumen fermentation in beef cattle. Trop Anim Health Prod 48: 1123–1128. https://doi.org/10.1007/s11250-016-1061-2 doi: 10.1007/s11250-016-1061-2
![]() |
[10] |
Zhang YC, Wang XK, Li DX, et al. (2020) Impact of wilting and additives on fermentation quality and carbohydrate composition of mulberry silage. Asian-Australas J Anim Sci 33: 254–263. https://doi.org/10.5713/ajas.18.0925 doi: 10.5713/ajas.18.0925
![]() |
[11] |
Irawan A, Sofyan A, Ridwan R, et al. (2021) Effects of different lactic acid bacteria groups and fibrolytic enzymes as additives on silage quality: A meta-analysis. Bioresour Technol Reports 14: 100654. https://doi.org/10.1016/j.biteb.2021.100654 doi: 10.1016/j.biteb.2021.100654
![]() |
[12] |
Lynch JP, Baah J, Beauchemin KA. (2015) Conservation, fiber digestibility, and nutritive value of corn harvested at 2 cutting heights and ensiled with fibrolytic enzymes, either alone or with a ferulic acid esterase-producing inoculant. J Dairy Sci 98: 1214–1224. https://doi.org/10.3168/jds.2014-8768 doi: 10.3168/jds.2014-8768
![]() |
[13] |
Chen L, Guo G, Yuan X, et al. (2016) Effects of applying molasses, lactic acid bacteria and propionic acid on fermentation quality, aerobic stability and in vitro gas production of total mixed ration silage prepared with oat-common vetch intercrop on the Tibetan Plateau. J Sci Food Agric 96: 1678–1685. https://doi.org/10.1002/jsfa.7271 doi: 10.1002/jsfa.7271
![]() |
[14] |
So S, Cherdthong A, Wanapat M, et al. (2020) Fermented sugarcane bagasse with Lactobacillus combined with cellulase and molasses promotes in vitro gas kinetics, degradability, and ruminal fermentation patterns compared to rice straw. Anim Biotechnol 33: 116–127. https://doi.org/10.1080/10495398.2020.1781146 doi: 10.1080/10495398.2020.1781146
![]() |
[15] |
Kaewpila C, Khota W, Gunun P, et al. (2022) Characterization of green manure sunn hemp crop silage prepared with additives: aerobic instability, nitrogen value, and in vitro rumen methane production. Fermentation 8: 104. https://doi.org/10.3390/fermentation8030104 doi: 10.3390/fermentation8030104
![]() |
[16] |
Khota W, Panyakaew P, Kesorn P, et al. (2023) In vitro rumen fermentation of coconut, sugar palm, and durian peel silages, prepared with selected additives. Fermentation 9: 567. https://doi.org/10.3390/fermentation9060567 doi: 10.3390/fermentation9060567
![]() |
[17] | Wanapat M, Polyorach S, Boonnop K, et al. (2009) Effects of treating rice straw with urea or urea and calcium hydroxide upon intake, digestibility, rumen fermentation and milk yield of dairy cows. Livest Sci 125: 238–243. |
[18] | Trach N, Mo M, Dan C (2001) Effects of treatment of rice straw with lime and/or urea on its chemical composition, in-vitro gas production and in-sacco degradation characteristics. Livest Res Rural Dev 13: 5–12. |
[19] |
Cai Y, Benno Y, Ogawa M, et al. (1999) Effect of applying lactic acid bacteria isolated from forage crops on fermentation characteristics and aerobic deterioration of silage. J Dairy Sci 82: 520–526. https://doi.org/10.3168/jds.S0022-0302(99)75263-X doi: 10.3168/jds.S0022-0302(99)75263-X
![]() |
[20] |
Darwin, WipaCharles, Cord-Ruwisch R (2018) Concurrent lactic and volatile fatty acid analysis of microbial fermentation samples by gas chromatography with heat pre-treatment. J Chromatogr Sci 56: 1–5. https://doi.org/10.1093/chromsci/bmx086 doi: 10.1093/chromsci/bmx086
![]() |
[21] | AOAC (2016) Official Method of Analysis, 20th Ed., Arlington, VA, USA: Association of Official Analytical Chemists. |
[22] |
Van Soest PJ, Robertson JB, Lewis BA (1991) Methods for dietary fiber neutral detergent fiber, and nonstarch polysaccharides in relation to animal nutrition. J Dairy Sci 74: 3583–3597. https://doi.org/10.3168/jds.S0022-0302(91)78551-2 doi: 10.3168/jds.S0022-0302(91)78551-2
![]() |
[23] | Menke KH, Steingass H (1988) Estimation of the energetic feed value obtained from chemical analysis and gas production using rumen fluid. Anim Res Dev 28: 7–55. |
[24] |
Ørskov ER, McDonald I (1979) The estimation of protein degradability in the rumen from incubation measurements weighted according to rate of passage. J Agric Sci 92: 499–503. https://doi.org/10.1017/S0021859600063048 doi: 10.1017/S0021859600063048
![]() |
[25] |
Tilley J, Terry R (1963) A two-stage technique for the in vitro digestion of forage crops. Grass Forage Sci 18: 104–111. https://doi.org/10.1111/j.1365-2494.1963.tb00335.x doi: 10.1111/j.1365-2494.1963.tb00335.x
![]() |
[26] | AOAC (1995) Official methods of analysis, 16th Ed., Arlington, VA, USA: Association of Official Analytical Chemists. |
[27] | Cai Y (2004) Analysis method for silage Japanese Society of Grassland Science Field and Laboratory Methods for Grassland Science, Tokyo: Tosho Printing Co. Ltd., Tokyo, 279–282. |
[28] | Statistical Analysis System (SAS) (2013) User's guide: Statistic (Version 9), 4th Ed., Cary, NC, USA: SAS Institute Inc. |
[29] |
Álvarez S, Méndez P, Martí-Fernández A (2015) Fermentative and nutritive quality of banana by-product silage for goats. J Appl Anim Res 43: 396–401. http://dx.doi.org/10.1080/09712119.2014.978782 doi: 10.1080/09712119.2014.978782
![]() |
[30] |
Cook DE, Bender RW, Shinners KJ, et al. (2016) The effects of calcium hydroxide—treated whole-plant and fractionated corn silage on intake, digestion, and lactation performance in dairy cows. J Dairy Sci 99: 5385–5393. http://dx.doi.org/10.3168/jds.2015-10402 doi: 10.3168/jds.2015-10402
![]() |
[31] | Pahlow G, Muck RE, Driehuis F, et al. (2003) Microbiology of ensiling. In: Buxton DR, Muck DR, Harrison JH, Silage Science and Technology, Madison, WI: American Society of Agronomy, 31–93. |
[32] |
Kung Jr L, Shaver RD, Grant RJ, et al. (2018) Silage review: Interpretation of chemical, microbial, and organoleptic components of silages. J Dairy Sci 101: 4020–4033. https://doi.org/10.3168/jds.2017-13909 doi: 10.3168/jds.2017-13909
![]() |
[33] |
Xia C, Liang Y, Bai S, et al. (2018) Effects of harvest time and added molasses on nutritional content, ensiling characteristics and in vitro degradation of whole crop wheat. Asian-Australas J Anim Sci 31: 354–362. https://doi.org/10.5713/ajas.17.0542 doi: 10.5713/ajas.17.0542
![]() |
[34] | Dahlke GR, Euken RM (2013) Calcium oxide and calcium hydroxide treatment of corn silage. Iowa State University Animal Industry Report 2013. |
[35] | Zhang Z (2021) Chapter 5—Waste pretreatment technologies for hydrogen production. In: Zhang Q, He C, Ren J, et al. (Eds.), Waste to Renewable Biohydrogen: Volume 1: Advances in Theory and Experiments, Academic Press, 109–122. https://doi.org/10.1016/B978-0-12-821659-0.00004-6 |
[36] |
Lunsin R, Duanyai S, Pilajun R, et al. (2018) Effect of urea-and molasses-treated sugarcane bagasse on nutrient composition and in vitro rumen fermentation in dairy cows. Agric Nat Resour 52: 622–627. https://doi.org/10.1016/j.anres.2018.11.010 doi: 10.1016/j.anres.2018.11.010
![]() |
[37] |
Xu J, Zhang K, Lin Y, et al. (2022) Effect of cellulase and lactic acid bacteria on the fermentation quality, carbohydrate conversion, and microbial community of ensiling oat with different moisture contents. Front Microbiol 13: 1013258. https://doi.org/10.3389/fmicb.2022.1013258 doi: 10.3389/fmicb.2022.1013258
![]() |
[38] |
Santos MC, Nussio LG, Mourão GB, et al. (2009) Nutritive value of sugarcane silage treated with chemical additives. Sci Agric (Piracicaba, Braz) 66: 159–163. https://doi.org/10.1590/S0103-90162009000200003 doi: 10.1590/S0103-90162009000200003
![]() |
[39] |
Li M, Zhou H, Zi X, et al. (2017) Silage fermentation and ruminal degradation of stylo prepared with lactic acid bacteria and cellulase. Anim Sci J 88: 1531–1537. https://doi.org/10.1111/asj.12795 doi: 10.1111/asj.12795
![]() |
[40] |
Mu L, Wang Q, Wang Y, et al. (2023) Effects of cellulase and xylanase on fermentative profile, bacterial diversity, and in vitro degradation of mixed silage of agro-residue and alfalfa. Chem Biol Technol Agric 10: 40. https://doi.org/10.1186/s40538-023-00409-4 doi: 10.1186/s40538-023-00409-4
![]() |
[41] | Sutaryono YA, Putra RA, Mardiansyah M, et al. (2023) Mixed Leucaena and molasses can increase the nutritional quality and rumen degradation of corn stover silage. J Adv Vet Anim Res 10: 118. |
[42] |
Cherdthong A, Suntara C, Khota W, Wanapat M. 2021. Feed utilization and rumen fermentation characteristics of Thai-indigenous beef cattle fed ensiled rice straw with Lactobacillus casei TH14, molasses, and cellulase enzymes. Livest Sci 245: 104405. https://doi.org/10.1016/j.livsci.2021.104405 doi: 10.1016/j.livsci.2021.104405
![]() |
[43] |
Carrillo-Díaz MI, Miranda-Romero LA, Chávez-Aguilar, G, et al. (2022) Improvement of ruminal neutral detergent fiber degradability by obtaining and using exogenous fibrolytic enzymes from white-rot fungi. Animals 12: 843. https://doi.org/10.3390/ani12070843 doi: 10.3390/ani12070843
![]() |
[44] |
Gunun N, Wanapat M, Kaewpila, C, et al. (2023) Effect of heat processing of rubber seed kernel on in vitro rumen biohydrogenation of fatty acids and fermentation. Fermentation 9: 143. https://doi.org/10.3390/fermentation9020143 doi: 10.3390/fermentation9020143
![]() |
[45] |
Satter LD, Slyter LL. (1974) Effect of ammonia concentration of rumen microbial protein production in vitro. Br J Nutr 32: 199–208. https://doi.org/10.1079/BJN19740073 doi: 10.1079/BJN19740073
![]() |
[46] |
Guo G, Shen C, Liu Q, et al. (2020) The effect of lactic acid bacteria inoculums on in vitro rumen fermentation, methane production, ruminal cellulolytic bacteria populations and cellulase activities of corn stover silage. J Integr Agric 19: 838–847. https://doi.org/10.1016/S2095-3119(19)62707-3 doi: 10.1016/S2095-3119(19)62707-3
![]() |
[47] |
Suntara S, Cherdthong A, Uriyapongson S, et al. (2020) Comparison effects of ruminal crabtree-negative yeasts and crabtree-positive yeasts for improving ensiled rice straw quality and ruminal digestion using in vitro gas production. J Fungi 6: 109. https://doi.org/10.3390/jof6030109. doi: 10.3390/jof6030109
![]() |
[48] |
Wang Q, Wang R, Wang C, et al. (2022) Effects of cellulase and Lactobacillus plantarum on fermentation quality, chemical composition, and microbial community of mixed silage of whole-plant corn and peanut vines. Appl Biochem Biotechnol 194: 2465–2480. https://doi.org/10.1007/s12010-022-03821-y doi: 10.1007/s12010-022-03821-y
![]() |
[49] |
Morvay Y, Bannink A, France J, et al. (2011) Evaluation of models to predict the stoichiometry of volatile fatty acid profiles in rumen fluid of lactating Holstein cows. J Dairy Sci 94: 3063–3080. https://doi.org/10.3168/jds.2010-3995 doi: 10.3168/jds.2010-3995
![]() |
[50] |
Ungerfeld EM (2020) Metabolic hydrogen flows in rumen fermentation: principles and possibilities of interventions. Front Microbiol 11: 589. https://doi.org/10.3389/fmicb.2020.00589 doi: 10.3389/fmicb.2020.00589
![]() |
[51] |
Børsting CF, Brask M, Hellwing, ALF, et al. (2020) Enteric methane emission and digestion in dairy cows fed wheat or molasses. J Dairy Sci 103: 1448–1462. https://doi.org/10.3168/jds.2019-16655 doi: 10.3168/jds.2019-16655
![]() |
[52] |
Gunun P, Wanapat M, Anantasook N (2013) Rumen fermentation and performance of lactating dairy cows affected by physical forms and urea treatment of rice straw. Asian-Australas J Anim Sci 26: 1295–1303. https://doi.org/10.5713/ajas.2013.13094 doi: 10.5713/ajas.2013.13094
![]() |
[53] |
So S, Wanapat M, Cherdthong A (2021) Effect of sugarcane bagasse as industrial by-products treated with Lactobacillus casei TH14, cellulase and molasses on feed utilization, ruminal ecology and milk production of mid-lactating Holstein Friesian cows. J Sci Food Agric 101: 4481–4489. https://doi.org/10.1002/jsfa.11087 doi: 10.1002/jsfa.11087
![]() |
1. | Xiaoli Du, Yintang Wen, Fubo Chen, Yangjun Liu, Yuyan Zhang, Xiaoyuan Luo, 2024, Hydroacoustic Target Recognition Based on Multidiscriminative Generative Adversarial Network, 979-8-3503-8418-5, 188, 10.1109/ICUS61736.2024.10839813 |
Methods | Metrics | |
PSNR | SSIM | |
WNNM | 15.59 | 0.6735 |
CBDNet | 19.32 | 0.7537 |
RIDNet | 19.54 | 0.7654 |
VDN | 20.13 | 0.7664 |
DANet | 20.22 | 0.7636 |
ARU-DGAN | 20.52 | 0.7700 |
Variants | Metrics | |
PSNR | SSIM | |
ARU-DGAN w/o G | 20.38 | 0.7654 |
ARU-DGAN | 20.52 | 0.7700 |
Variants | Metrics | |
PSNR | SSIM | |
U-Net | 20.03 | 0.7529 |
Residual U-Net | 20.21 | 0.7639 |
Attention Residual U-Net | 20.52 | 0.7700 |
Methods | Metrics | |
PSNR | SSIM | |
WNNM | 15.59 | 0.6735 |
CBDNet | 19.32 | 0.7537 |
RIDNet | 19.54 | 0.7654 |
VDN | 20.13 | 0.7664 |
DANet | 20.22 | 0.7636 |
ARU-DGAN | 20.52 | 0.7700 |
Variants | Metrics | |
PSNR | SSIM | |
ARU-DGAN w/o G | 20.38 | 0.7654 |
ARU-DGAN | 20.52 | 0.7700 |
Variants | Metrics | |
PSNR | SSIM | |
U-Net | 20.03 | 0.7529 |
Residual U-Net | 20.21 | 0.7639 |
Attention Residual U-Net | 20.52 | 0.7700 |