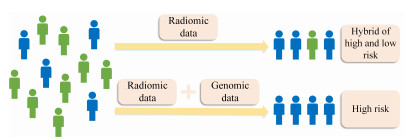
Benefits of biochar application on environment conservation and agricultural production have been widely studied. However, few studies were focused on root development. The objective of this study covered root and shoot development, yield, and soil properties associated with exposure of Oryza glaberrima rice during the early reproductive stage to drought stress in rice-husk amended soil. The biochar was amended at a rate of 10.5 g pot-1, equivalent to 3 ton ha-1. Biochar-amended and non-amended plants were exposed to drought stress after the panicles had visibly emerged in all plant populations. Biochar application caused less restriction on root elongation, volume, and surface area during water stress conditions. Enhanced root development was primarily associated with improvement in water status and chemical properties in biochar-amended soil. Soil chemical properties improved, including increased soil pH, available P, cation exchange capacity, and exchangeable Mg. Under drought stress conditions, shoot growth was more sensitive than root growth, as indicated by the significant reduction of stem dry weight (SDW) and leaf dry weight (LDW). Fine roots in biochar-amended soil were longer than those in non-amended soil. In general, Biochar application enable the O. glaberrima rice to maintain yield under drought stress condition.
Citation: Kartika Kartika, Jun-Ichi Sakagami, Benyamin Lakitan, Shin Yabuta, Isao Akagi, Laily Ilman Widuri, Erna Siaga, Hibiki Iwanaga, Arinal Haq Izzawati Nurrahma. Rice husk biochar effects on improving soil properties and root development in rice (Oryza glaberrima Steud.) exposed to drought stress during early reproductive stage[J]. AIMS Agriculture and Food, 2021, 6(2): 737-751. doi: 10.3934/agrfood.2021043
[1] | Jingren Niu, Qing Tan, Xiufen Zou, Suoqin Jin . Accurate prediction of glioma grades from radiomics using a multi-filter and multi-objective-based method. Mathematical Biosciences and Engineering, 2023, 20(2): 2890-2907. doi: 10.3934/mbe.2023136 |
[2] | Hakan Özcan, Bülent Gürsel Emiroğlu, Hakan Sabuncuoğlu, Selçuk Özdoğan, Ahmet Soyer, Tahsin Saygı . A comparative study for glioma classification using deep convolutional neural networks. Mathematical Biosciences and Engineering, 2021, 18(2): 1550-1572. doi: 10.3934/mbe.2021080 |
[3] | Sonam Saluja, Munesh Chandra Trivedi, Shiv S. Sarangdevot . Advancing glioma diagnosis: Integrating custom U-Net and VGG-16 for improved grading in MR imaging. Mathematical Biosciences and Engineering, 2024, 21(3): 4328-4350. doi: 10.3934/mbe.2024191 |
[4] | Yutao Wang, Qian Shao, Shuying Luo, Randi Fu . Development of a nomograph integrating radiomics and deep features based on MRI to predict the prognosis of high grade Gliomas. Mathematical Biosciences and Engineering, 2021, 18(6): 8084-8095. doi: 10.3934/mbe.2021401 |
[5] | Sonam Saluja, Munesh Chandra Trivedi, Ashim Saha . Deep CNNs for glioma grading on conventional MRIs: Performance analysis, challenges, and future directions. Mathematical Biosciences and Engineering, 2024, 21(4): 5250-5282. doi: 10.3934/mbe.2024232 |
[6] | Xiaowei Zhang, Jiayu Tan, Xinyu Zhang, Kritika Pandey, Yuqing Zhong, Guitao Wu, Kejun He . Aggrephagy-related gene signature correlates with survival and tumor-associated macrophages in glioma: Insights from single-cell and bulk RNA sequencing. Mathematical Biosciences and Engineering, 2024, 21(2): 2407-2431. doi: 10.3934/mbe.2024106 |
[7] | Hongwei Sun, Qian Gao, Guiming Zhu, Chunlei Han, Haosen Yan, Tong Wang . Identification of influential observations in high-dimensional survival data through robust penalized Cox regression based on trimming. Mathematical Biosciences and Engineering, 2023, 20(3): 5352-5378. doi: 10.3934/mbe.2023248 |
[8] | Moxuan Zhang, Quan Zhang, Jilin Bai, Zhiming Zhao, Jian Zhang . Transcriptome analysis revealed CENPF associated with glioma prognosis. Mathematical Biosciences and Engineering, 2021, 18(3): 2077-2096. doi: 10.3934/mbe.2021107 |
[9] | Yuan Yang, Lingshan Zhou, Xi Gou, Guozhi Wu, Ya Zheng, Min Liu, Zhaofeng Chen, Yuping Wang, Rui Ji, Qinghong Guo, Yongning Zhou . Comprehensive analysis to identify DNA damage response-related lncRNA pairs as a prognostic and therapeutic biomarker in gastric cancer. Mathematical Biosciences and Engineering, 2022, 19(1): 595-611. doi: 10.3934/mbe.2022026 |
[10] | Wei Niu, Lianping Jiang . A seven-gene prognostic model related to immune checkpoint PD-1 revealing overall survival in patients with lung adenocarcinoma. Mathematical Biosciences and Engineering, 2021, 18(5): 6136-6154. doi: 10.3934/mbe.2021307 |
Benefits of biochar application on environment conservation and agricultural production have been widely studied. However, few studies were focused on root development. The objective of this study covered root and shoot development, yield, and soil properties associated with exposure of Oryza glaberrima rice during the early reproductive stage to drought stress in rice-husk amended soil. The biochar was amended at a rate of 10.5 g pot-1, equivalent to 3 ton ha-1. Biochar-amended and non-amended plants were exposed to drought stress after the panicles had visibly emerged in all plant populations. Biochar application caused less restriction on root elongation, volume, and surface area during water stress conditions. Enhanced root development was primarily associated with improvement in water status and chemical properties in biochar-amended soil. Soil chemical properties improved, including increased soil pH, available P, cation exchange capacity, and exchangeable Mg. Under drought stress conditions, shoot growth was more sensitive than root growth, as indicated by the significant reduction of stem dry weight (SDW) and leaf dry weight (LDW). Fine roots in biochar-amended soil were longer than those in non-amended soil. In general, Biochar application enable the O. glaberrima rice to maintain yield under drought stress condition.
Low-grade glioma (LGG) is a uniformly fatal tumor, and the survival from this tumor is approximately 7 years [1]. Because of the heterogeneity in LGG patients, different LGG subtypes increase the difficulty of optimizing management of adult low-grade gliomas [2,3]. Magnetic Resonance Imaging (MRI) is an imaging technique that can capture tumors of the brain clearly [4]. Clinicians often use MRI images to diagnose the agammaessiveness of the tumor. Therefore, the analysis of MRI data and feature extraction are becoming more challenging. To address these issues, many studies have used MRI data to extract prognostic factors for LGG patients. In a study by Pignatti et al. [5], the authors established a score system that can be used to determine the prognostic score. In adult patients with LGG, the age of the patients, the astrocytoma histology, the largest diameter of the tumor, the tumor crossing the midline and the presence of a neurologic deficit before surgery are all important prognostic factors for survival. These factors can be used to identify low-risk and high-risk patients. In a study by Chen et al. [6], the authors developed a computer-assisted algorithm for tumor segmentation and characterization using both kinetic information and morphological features of 3-D DCE-MRI. They differentiated benign and malignant lesions by analyzing 3-D morphological features including shape features and texture features of the segmented tumor. In a study by Agravat et al. [7], the authors implemented the DeepMedic CNN architecture for tumor segmentation and the extracted features are fed to a random forest classifier to obtain 59 overall survival accuracy. In another study by Shboul et al. [8], 40 features were extracted from the predicted brain tumor mask and fed to a random forest regression to predict the overall survival of a glioma patient, with an accuracy of 67 on the training dataset and 57.9 on the testing dataset. In an attempt at prediction of survival [9], the authors extracted 26 image-derived geometrical features and used SVM to predict the risk of death and classify glioma patients into three groups, with an accuracy of 56.8. In another attempt [10], hundreds of intensity and texture features were extracted from MR images of glioblastoma multiforme, and principal component analysis (PCA) was used to reduce dimensionality. Then, these features were fed to an artificial neural network (ANN). A result with accuracy of 65.1 was obtained based on two classes: short-overall survivor and long-overall survivor. In another study [11], Chato et al. attempted the use of support vector machines (SVMs), k-nearest neighbors (KNNs), linear discriminants, tree, ensembles and logistic regression to classify survivors into two or three classes. The features from segmentations are used to train the linear discriminant for prediction of survival. The texture features resulted in the accuracy of 46, and histogram features achieved an accuracy of 68.5 for the test dataset.
The above methods predicted survival by using only image information or clinical information. However, the tumor heterogeneity possibly comes from strong phenotypic differences, and it is difficult to predict prognosis accurately by using only medical imaging analysis (see Figure 1), thus motivating the need for integrating another kind of data. Along with the rapid development of deep-sequencing technology, the output of sequencing has made huge progress not only in equality but also in speed [12]. If radiomic data and genomic data can be integrated, this integration will build a bridge between micro and macro and increase the accuracy of the precision diagnosis and treatment of the brain tumor [13]. Grossmann et al. [14] found that prognostic biomarkers performed better in lung cancer when radiomic, genetic, and clinical information was combined. The C-index was 0.73, while the result is only 0.66 when lacking genetic information. Xia et al. [15] created a radiogenomic strategy that can obtain significant associations between imaging features and gene expression patterns in hepatocellular carcinoma. However, similar work is lacking in LGG. Therefore, in this study we integrated two different types of data, i.e., radiomic features of MRI and gene signatures, to develop a new integrated survival prediction measure for LGG.
The framework of this study is shown in Figure 2. First, we used gene expression data to construct a gene regulatory network and identify network modules and then used imaging data to extract significant radiomic biomarkers that are associated with the survival of the patient (Parts (a) and (b)), respectively. Then, we calculated the correlation between gene modules and image features to obtain a small number of gene signatures that are connected with these image features (Part (c)). Furthermore, we established a Lasso (least absolute shrinkage and selection operator) model to predict the image features with only gene expression values (Parts (d) and (e)). Based on gene expression data, we used support vector machines (SVMs) to identify the gene signatures (Parts (f) and (g)). We combined the predicted image features and the gene signatures to establish an integrated measure that can predict survival of the LGG patient (Parts (h) and (i)). The results show that the integrated measure performed better on survival prediction than any other single index.
Computer-aided and manually corrected segmentation labels for the preoperative multi-institutional scans of 65 LGG patients and 724 radiomic features along with the corresponding skull-stripped and coregistered multimodal (i.e., T1, T1-Gd, T2, T2-FLAIR) MRI data were collected from the Cancer Imaging Archive (TCIA) [16,17,18]. The corresponding RNA-seq data and Disease Free Survival (DSS) data for these 65 patients were also obtained from The Cancer Genome Atlas (TCGA) database. These data were used in this study as the training dataset.
The gene expression data and the corresponding DSS data of 455 LGG patients were downloaded from TCGA and used in this study as the validation dataset.
A gene coexpression network was constructed using gene expression data in the training dataset. We deleted genes that express in less than 20 of the patients or have no expression values. Then, we retained genes that have the highest 25 variance. A pairwise correlation matrix was calculated, and then we adjusted the matrix by raising it to the power of five using the R package WGCNA [19,20]. The minimum module size was set to 50, and the minimum height for merging modules was set to 0.25.
We identified significant image features that are associated with patient DSS by training a multivariate Cox regression model [21] on the training dataset. Image features were filtered with the standard that p value must be less than 0.01. Then, these image features were treated as image biomarkers and survival prediction indexes. For each image feature, we divided patients on the validation dataset into two groups—high-risk group and low-risk group—by taking the median value of the feature as the threshold and plotted the Kaplan-Meier curves. The concordance index (C-index) [22] and the log-rank test were also used to assess the prognostic prediction performance.
The basic formula of the multivariate Cox regression model is described as follows:
(1) |
represents the hazard function and is the baseline hazard function. The factor , , ..., correspond to the image features here and , , ..., are the corresponding regression coefficients.
We calculated Pearson correlation coefficients and their statistical significance to obtain the correlations between gene modules and selected image features. Because there are many genes in each module, the principal component analysis (PCA) was used to reduce the dimension of gene expression data of 65 patients in the training dataset. Then, image features were filtered. Features that showed significant correlation (p value less than 0.05) with at least one gene module were retained, and others were removed. Then, gene modules associated with the same image feature were integrated. The enrichment analysis was performed to identify the significantly enriched molecular pathways on these modules.
We established a radiogenomic map by identifying gene signatures associated with the prognostic imaging features. Lasso (least absolute shrinkage and selection operator) is a regression analysis method that performs both variable selection and regularization [23,24]. This method can enhance the prediction accuracy and interpretability of the statistical model it produces.
(2) |
Among the above formulas, X is the variable and y is the label. is the coefficient that we want to optimize. is the objective function that we want to minimize. Compared with the method of least squares, the objective function in the Lasso model has a regularization term . With this norm regularization term, Lasso can control the number of variables used and improve the generalization ability of the model. For each image feature remaining in the gene module analysis, Lasso was trained to select gene signatures from related gene modules and make a prediction on image features with MRI data and gene expression data in the training dataset. We determined the regularization coefficient by minimizing the MSE (mean squared error) of the model.
In this step, we obtained a survival prediction index using only gene signatures, without the information of image features. SVMs (support vector machines) are supervised learning models that can be used for classification and regression problems [25,26,27]. For a classification problem, the optimal hyperplane is searched to separate data into two classes with the max margin. For new data, the trained hyperplane is used to predict the label or the probability of each class. Sometimes, data may not be separated completely, and a soft margin [25] can be used by adding a penalty parameter and slack variables to obtain the minimum error. The SVM optimization problem is
(3) |
subject to
(4) |
The vector is the vector orthogonal to the hyperplane. , are an observation pair of data points, and is the label of predicted by the SVM. SVM-RFE (support vector machine-recursive feature elimination) [28] is a powerful feature selection algorithm based on SVM that can avoid overfitting when the number of features is high. In each iteration, features are scored and sorted through model training and the least important feature is removed. Remaining features are used for a next training, and the above step is repeated. The score for sorting of the feature is defined as
(5) |
is the dimension of the hyperplane orthogonal vector in SVM. Finally, the optimal number of features that have the minimum error is determined.
We use SVM-FRE to select gene signatures and train a classification SVM model with expression data of these selected gene signatures and DSS data in the training dataset. The patient labels are set to 0 or 1 based on their prognostic situation—survival or death. Then, the predicted probability is treated as a survival prediction index. Survival curve and C-index are used to access the prediction performance.
Further, we consider a combination of selected image biomarkers and the index calculated by SVM with gene signatures. To ensure improvement of the new agammaegated index, we transform the calculation of optimal combination coefficients of all features into an optimization problem. Specifically, suppose that image features are considered to be associated with DSS independently—which are recorded as , , ..., and the gene index value from SVM is recorded as . The integrated measure we want to determine is recorded as . The optimization problem needing to be solved can be described as follows.
(6) |
subject to
(7) |
(8) |
where is the C-index of integrated measure on the training dataset. Our goal is to search optimal parameters , , ..., and in Eq (7) to maximize the in (6).
The Particle Swarm Optimization (PSO) algorithm [29] is used to solve the optimization problem (6) in this study. PSO is an evolutionary computation algorithm inspired by bird activities that can solve any optimization problem. Initial population with some random particle is created first. For each particle, the position represents a solution, and the corresponding fitness means a value of target function. The object of PSO is to find the optimal particle that has the minimized fitness by updating the velocity and position of particle as the following formula:
(9) |
(10) |
, is the position and velocity of the particle. is the best position of the particle in history and is the best position of all particles currently. , are random numbers between 0 and 1. is the inertia weight, and , are the acceleration constants.
We take a log-rank test on 724 image features using DSS data of 65 patients in TCIA and filter these features with a standard that the p value is less than 0.01. Then, 21 features remain. Features with high similarity to each other are removed: we calculate the Pearson correlation coefficient between features and remove the one that has the bigger log-rank p value if the Pearson correlation coefficient between two image features is greater than 0.8. After this step, 6 features are removed, and 15 features remain. Based on the above univariable analysis, we first implement the proportional hazard test [21]. Each image feature meets the proportional hazard assumption (detailed information is shown in Additional file 1: Table S1). Then, we train a multivariate Cox regression model on these remaining image features with gene expression data and DSS data in the training dataset. The result is shown in Table 1, and eight features marked with are considered to be independently correlated with DSS .
Image features | exp(coef) | exp(coef) lower 95% | exp(coef) upper 95% | Wald test | p value |
TEXTURE_GLSZM_ET_T1Gd_SZLGE* | 0 | 0 | 0 | 3.12 | 0.00178 |
HISTO_ED_T2_Bin8* | 0.7 | 0.55 | 0.88 | 3.02 | 0.00254 |
TEXTURE_GLOBAL_ET_T1Gd_Skewness* | 3.03E+05 | 47.29 | 1.94E+09 | 2.82 | 0.00477 |
TEXTURE_GLRLM_NET_FLAIR_LRHGE* | 1 | 0.99 | 1 | 2.66 | 0.0078 |
HISTO_NET_T1_Bin4* | 0.89 | 0.8 | 0.98 | 2.42 | 0.01559 |
HISTO_ET_T1Gd_Bin10* | 1.19 | 1.03 | 1.38 | 2.35 | 0.01877 |
TEXTURE_GLSZM_NET_T1Gd_ZSV* | 0 | 0 | 0 | 2.34 | 0.01906 |
TEXTURE_GLRLM_NET_T1Gd_GLV* | 2.00E+42 | 2230.45 | 1.79E+81 | 2.13 | 0.0333 |
HISTO_ET_T1_Bin10 | 0.81 | 0.63 | 1.05 | 1.59 | 0.11219 |
TEXTURE_GLCM_ET_T2_SumAverage | 0 | 0 | 9.95E+83 | 1.57 | 0.11569 |
TEXTURE_GLRLM_NET_T1_LGRE | 0 | 0 | 2.73E+38 | 1.49 | 0.13526 |
TEXTURE_GLRLM_ED_T1_RLV | inf | 0 | inf | 1.4 | 0.16185 |
HISTO_ED_T2_Bin4 | 0.96 | 0.88 | 1.05 | 0.91 | 0.36241 |
TEXTURE_GLCM_ED_FLAIR_Energy | inf | 0 | inf | 0.78 | 0.43387 |
TEXTURE_GLSZM_NET_T1_LZLGE | 0.99 | 0.96 | 1.03 | 0.39 | 0.69976 |
A gene coexpression network is constructed using gene expression data of 65 patients in the training dataset. We delete genes that express in less than 20 of the patients or have no expression values (n = 1875). Then, we retain genes that have the highest 25 variance (n = 4663). A pairwise correlation matrix is calculated, and then we adjust the matrix by raising it to the power of five using the R package WGCNA [19,20]. The minimum module size is set to 50 and the minimum height for merging modules is set to 0.25. Then, we get 12 gene modules. Detailed information on the modules is shown in Additional file 2: Table S2.
The Pearson correlation coefficient and their statistical significance were calculated between the 12 gene modules and the 8 image features. The result is shown in Figure 3. Four image features that show significant correlation with at least one gene module were obtained. HISTOEDT2Bin8 is the 8-bin histogram feature of the peritumoral edema in T2-weighted precontrast, TEXTUREGLSZMNETT1GdZSV is the zone size variance of gray level size zone matrix (GLSZM) of the nonenhancing part of the tumor core in T1-weighted postcontrast, TEXTUREGLRLMNETFLAIRLRHGE is the long run high gray level emphasis of gray level run length matrix (GLRLM) of the nonenhancing part of the tumor core in T2 Fluid-Attenuated Inversion Recovery, and TEXTUREGLRLMNETT1GdGLV is the gray level variance of GLRLM of the nonenhancing part of the tumor core in T1-weighted postcontrast. Then, their corresponding gene modules were integrated. The statistical results are shown in Table 2 and the detailed list of genes is shown in Additional file 3: Table S3.
Image features | Associated gene modules | Number of associated genes |
HISTO_ED_T2_Bin8 | module2, module4, module5, module7 | 2794 |
TEXTURE_GLSZM_NET_T1Gd_ZSV | module6 | 506 |
TEXTURE_GLRLM_NET_FLAIR_LRHGE | module6 | 506 |
TEXTURE_GLRLM_NET_T1Gd_GLV | module2, module4, module6, module8, module10 | 1421 |
A further KEGG enrichment analysis was performed on integrated gene modules using the Metascape website [30], which is shown in Figure 4. The complete list of biological annotations is shown in Additional file 4: Table S4. Among these, the neuroactive ligand-receptor interaction pathway is mostly enriched in all integrated gene modules with the minimum p value of , which is reported to be associated with glioma [31,32].
Then, the Lasso method described in section 2.5 was used to select gene signatures from the related gene modules and establish a map from genes to image features. We determined the regularization coefficient by minimizing the MSE (mean squared error) of the model. The process is shown in Figure 6. The optimal coefficient and the corresponding RMSE (root mean squared error) of 65 patients are shown in Table 3. The number of selected gene signatures is also shown. The detailed list of gene signatures is shown in Additional file 5: Table S5.
Image feature | Number of genes in associated modules | Optimal | RMSE | Number of genes selected by Lasso |
HISTO_ED_T2_Bin8 | 2794 | 1.6627 | 6.0847 | 12 |
TEXTURE_GLSZM_NET_T1Gd_ZSV | 506 | 7.81E-06 | 2.0195E-5 | 3 |
TEXTURE_GLRLM_NET_FLAIR_LRHGE | 506 | 163.9677 | 528.16 | 6 |
TEXTURE_GLRLM_NET_T1Gd_GLV | 1421 | 3.01E-03 | 0.0120 | 18 |
We made a prediction on the 4 image features using Lasso with gene expression data of 455 patients in TCGA as the validation dataset. We then took the value of each image feature as a survival prediction index. We calculated the C-index and plotted the Kaplan-Meier curves on the validation dataset. The result is shown in Figure 7. The C-index of these four survival prediction indexes are 0.6945, 0.7321, 0.7926, and 0.7985. These results indicate that these four image features perform well in survival prediction.
From the selected 4663 genes with high variance, we fed gene expression data and DSS data of 65 patients in TCIA to SVM-FRE and obtained 43 gene signatures (shown in Additional file 6: Table S6). Then, we trained a classification SVM model with these selected genes. The variables were gene expression data of 65 patients, and the labels were set to 0 or 1 based on the patient prognostic situation—survival or death. Penalty parameter was set to 2 and 5-fold cross-validation was used to evaluate the error in the recursive feature elimination process. We trained the SVM model and took the predicted probability of survival as a survival prediction index. C-index and survival curve are shown in Figure 8. The C-index is 0.7627.
We took a linear combination of four significant image features and the index calculated by SVM with gene signatures. A better integrated measure was obtained that represents patient survival situation. Set in formula (7). Four normalized image feature values were recorded as , , , and , and the index value from SVM was recorded as . The integrated measure is recorded as . Then, we get
(11) |
We used PSO algorithm to calculate the optimal coefficient to maximize the C-index of 65 patients in the training dataset, with parameters , and of 0.8, 0.5 and 0.5. The initial population size was set to 20, 25, 30, 35 and 40, and the corresponding iteration number was set to 30 to ensure the convergence of PSO. We repeated numerical experiments 10 times and recorded the average result for different parameters. Detailed results of each experiment are shown in Additional file 7: Table S7. For each population size, we then brought the coefficients into formula (11) and obtained integrated measure with different forms. C-index was calculated using gene expression data on the validation dataset. The validation result is shown in Table 4.
Populations sizes | 20 | 25 | 30 | 35 | 40 |
0.2926 | 0.3187 | 0.2792 | 0.3303 | 0.276 | |
0.0663 | 0.0394 | 0.0739 | 0.0505 | 0.068 | |
0.2171 | 0.2329 | 0.2076 | 0.2214 | 0.2102 | |
0.0091 | 0.019 | 0.0107 | 0.0298 | 0.0159 | |
0.4149 | 0.39 | 0.4288 | 0.368 | 0.4298 | |
C-index | 0.8065 | 0.807 | 0.8061 | 0.807 | 0.8057 |
From Table 4, we observe that is more or less than 0.4 with different parameters. Therefore, the proportion of gene signatures in integration is approximately 40. is approximately 0.3, is approximately 0.06 and is approximately 0.24. is nearly 0, indicating that the gray level variance of GLRLM of the nonenhancing part of the tumor core in T1-weighted postcontrast can be removed in the integration. We then set parameters , , , and to 0.3, 0.06, 0.24, 0 and 0.4. We brought these coefficients into formula (11) and calculated the integrated measure on the validation dataset. The Kaplan-Meier curve is shown in Figure 9. The C-index of the four independent image features, gene signatures and integrated measures are shown in Table 5.
Image features | ||||||
C-index | 0.6945 | 0.7321 | 0.7926 | 0.7985 | 0.7627 | 0.8071 |
The C-index of the integrated measure is 0.8071 and is higher than any other measure based on image signatures or gene signature. This result indicates that the integrated measure can improve the prediction accuracy. The integrated measure is recorded as follows.
(12) |
Furthermore, we use the time dependent Receiver Operating Characteristic (ROC) [33] to further assess the predictive power and compare different prediction models. Time-dependent ROC analysis showed that the integrated measure improved our ability to predict prognosis [AUC, 0.79; and 95 confidence intervals (CI), 0.71 to 0.87] (see Figure 10), when compared with other measures based on image signatures or gene signatures.
Patients are defined into two groups—high-risk group and low-risk group, based on their prognosis—DSS value in this study, by taking the median value of DSS of 65 patients in the training dataset as a threshold. Then, classification is conducted on 455 patients in the validation dataset by taking a threshold of the median value of the integrated measure in the training dataset. The accuracy is 72.1, which is higher than the accuracy of the published studies [7,8,9,10,11].
The primary goal of phenotyping and classifying a human tumor is to capture tumor heterogeneity and realize personalized precision diagnosis and therapy. In clinical practice, the massive and multiple types of big medical data are available with the rapid development of biomedical engineering and computer application technology. However, one of the biggest challenges in clinical applications is how to integrate these different types of data to extract accuracy information.
In this study, we attempted to integrate both MRI data and gene expression data to propose a new feature measure that could be used to identify subsets of LGG patients at low and high risk for progression to DSS. Based on gene expression data, we first used the WGCNA method to construct the network and identify twelve network modules. With MRI data, eight image biomarkers were obtained by using the Cox regression model. Furthermore, through correlation analysis between gene modules and image features, four radiomic biomarkers were identified. Because MRI data are not available in our test dataset, the Lasso method was applied to build a map from gene expression data to these image features. In addition, we also independently used gene expression data to predict image biomarkers through the SVM method. Finally, an integrated measure (IM) for combining image and gene signatures was obtained through the PSO algorithm. We validated IM with gene expression data and DSS data on 455 patients in the validation dataset. The C-index of IM is 0.8071 and its Area Under Curve (AUC) of the ROC curve is 0.79, higher than any other single measure. The accuracy of classification of patients is 72.1, which is higher than the accuracy of the published work using only radiomic data [7,8,9,10,11]. The results demonstrate that the proposed IM enhances the prediction accuracy for lower grade gliomas.
In summary, the accuracy of DSS prediction of LGG patients is successfully improved by integrating radiomic features in Macro with the gene expression data in Micro. The proposed method in this study can also be extended to analyze different data sources of other tumors.
This work was supported by the National Key Research and Development Program of China (No. 2018YFC1314600), the Key Program of the National Natural Science Foundation of China (No. 11831015) and the Chinese National Natural Science Foundation (No. 61672388).
All authors declare no conflicts of interest in this paper.
[1] | Widuri LI, Lakitan B, Sodikin E, et al. (2018) Shoot and root growth in common bean (Phaseolus vulgaris L.) exposed to gradual drought stress. Agrivita 40: 442-449. |
[2] | Lakitan B, Hadi B, Herlinda S, et al. (2018) Recognizing farmers' practices and constraints for intensifying rice production at Riparian Wetlands in Indonesia. NJAS-Wageningen J Life Sci 85: 10-20. |
[3] | Lakitan B, Widuri LI, Meihana M, et al. (2017) Simplifying procedure for a non-destructive, inexpensive, yet accurate trifoliate leaf area estimation in snap bean (Phaseolus vulgaris). J Appl Hort 19: 15-21. |
[4] | Kartika K, Lakitan B, Sanjaya N, et al. (2018a) Internal versus edge row comparison in Jajar legowo 4: 1 rice planting pattern at different frequency of fertilizer applications. Agrivita 40: 222-232. |
[5] | Yang J, Zhang J (2006) Grain filling of cereals under soil drying. New Phytol 169: 223-236. |
[6] | Keshavarz AR, Hashemi M, DaCosta M, et al. (2016) Biochar application and drought stress effects on physiological characteristics of Silybum marianum. Commun Soil Sci Plant Anal 47: 743-752. |
[7] | Yamato M, Okimori Y, Wibowo IF, et al. (2006). Effects of the application of charred bark of Acacia mangium on the yield of maize, cowpea and peanut, and soil chemical properties in South Sumatra, Indonesia. Soil Sci Plant Nutr 52: 489-495. |
[8] | Dunnigan L, Ashman PJ, Zhang X, et al. (2018) Production of biochar from rice husk: Particulate emissions from the combustion of raw pyrolysis volatiles. J Clean Prod 172: 1639-1645. |
[9] | Abrishamkesh S, Gorji M, Asadi H, et al. (2015) Effects of rice husk biochar application on the properties of alkaline soil and lentil growth. Plant Soil & Environ 61: 475-482. |
[10] | Kartika K, Lakitan B, Wijaya A, et al. (2018b) Effects of particle size and application rate of rice-husk biochar on chemical properties of tropical wetland soil, rice growth and yield. Aust J Crop Sci 12: 817-826. |
[11] | Liu S, Meng J, Jiang L, et al. (2017) Rice husk biochar impacts soil phosphorous availability, phosphatase activities and bacterial community characteristics in three different soil types. Appl Soil Ecol 116: 12-22. |
[12] | Brennan A, Jiménez EM, Puschenreiter M, et al. (2014) Effects of biochar amendment on root traits and contaminant availability of maize plants in a copper and arsenic impacted soil. Plant Soil 379: 351-360. |
[13] | Vanek SJ, Lehmann J (2015) Phosphorus availability to beans via interactions between mycorrhizas and biochar. Plant Soil 395: 105-123. |
[14] | Xiang Y, Deng Q, Duan H, et al. (2017) Effects of biochar application on root traits: A meta-analysis. GCB Bioenergy 9: 1563-1572. |
[15] | Kim Y, Chung YS, Lee E, et al. (2020) Root response to drought stress in rice (Oryza sativa L.). Int J Mol Sci 21: 122. |
[16] | Sarla N, Swamy BPM (2005) Oryza glaberrima: A source for the improvement of Oryza sativa. Curr Sci 89: 955-963. |
[17] | Kartika K, Sakagami JI, Lakitan B, et al. (2020) Morpho-physiological response of Oryza glaberrima to gradual soil drying. Rice Science 27: 67-74. |
[18] | Lu SG, Sun FF, Zong YT (2014) Effect of rice husk biochar and coal fly ash on some physical properties of expansive clayey soil (Vertisol). Catena 114: 37-44. |
[19] | Akhtar SS, Andersen MN, Liu F (2015) Biochar mitigates salinity stress in potato. J Agron Crop Sci 201: 368-378. |
[20] | Foster EJ, Hansen N, Wallenstein M, et al. (2016) Biochar and manure amendments impact soil nutrients and microbial enzymatic activities in a semi-arid irrigated maize cropping system. Agric Ecosyst Environ 233: 404-414. |
[21] | Asai H, Samson BK, Stephan HM, et al. (2009) Biochar amendment techniques for upland rice production in Northern Laos. 1. Soil physical properties, leaf SPAD and grain yield. Field Crop Res 111: 81-84. |
[22] | Artiola JF, Rasmussen C, Freitas R (2012) Effects of a biochar-amended alkaline soil on the growth of romaine lettuce and bermudagrass. Soil Sci 177: 561-570. |
[23] | Abel S, Peters A, Trinks S, et al. (2013) Impact of biochar and hydrochar addition on water retention and water repellency of sandy soil. Geoderma 202: 183-191. |
[24] | Novak JM, Busscher WJ, Watts DW, et al. (2012) Biochars impact on soil-moisture storage in an ultisol and two aridisols. Soil Sci 177: 310-320. |
[25] | Laird DA (2008) The charcoal vision: A win-win-win scenario for simultaneously producing bioenergy, permanently sequestering carbon, while improving soil and water quality. Agron J 100: 178-181. |
[26] | Sohi SP, Krull E, Lopez-Capel E, et al. (2010) A review of biochar and its use and function in soil. Adv Agronomy 105: 47-82. |
[27] | Van Zwieten L, Kimber S, Morris S, et al. (2010) Effects of biochar from slow pyrolysis of papermill waste on agronomic performance and soil fertility. Plant Soil 327: 235-246. |
[28] | Obia A, Cornelissen G, Mulder J, et al. (2015) Effect of soil pH increase by biochar on NO, N2O and N2 production during denitrification in acid soils. PLoS One 10: e0138781. |
[29] | Liang B, Lehmann J, Solomon D, et al. (2006) Black carbon increases cation exchange capacity in soils. Soil Sci Soc Amer J 70: 1719-1730. |
[30] | Jien SH, Wang CS (2013) Effects of biochar on soil properties and erosion potential in a highly weathered soil. Catena 110: 225-233. |
[31] | Cui HJ, Wang MK, Fu ML, et al. (2011) Enhancing phosphorus availability in phosphorus-fertilized zones by reducing phosphate adsorbed on ferrihydrite using rice straw-derived biochar. J Soil Sediment 11: 1135-1141. |
[32] | Li D, Shi X, Zhao Y, et al. (2016) Rice-husk biochar improved soil properties and wheat yield on an acidified purple soil. In: Kim YH, The 5th International Conference on Civil, Architectural and Hydraulic Engineering (ICCAHE), Dordrecht, The Netherlands: Atlantis Press, 294-302. |
[33] | Mahajan S, Tuteja N (2005) Cold, salinity and drought stresses: An overview. Arch Biochem Biophys 444: 139-158. |
[34] | Widuri LI, Lakitan B, Hasmeda M, et al. (2017) Relative leaf expansion rate and other leaf-related indicators for detection of drought stress in chili pepper (Capsicum annuum L.). Aust J Crop Sci 11: 1617-1625. |
[35] | Sakagami JI (2012) Submergence tolerance of rice species Oryza glaberrima Steud. In: Najafpour M, Applied Photosynthesis, Germany: Books on Demand, 353-364. |
[36] | Bañon S, Fernandez JA, Franco JA, et al. (2004) Effects of water stress and night temperature preconditioning on water relations and morphological and anatomical changes of Lotus creticus plants. Sci Hortic 101: 333-342. |
[37] | Comas LH, Mueller KE, Taylor LL, et al. (2012) Evolutionary patterns and biogeochemical significance of angiosperm root traits. Int J Plant Sci 173: 584-595. |
[38] | Dien DC, Yamakawa T, Mochizuki T, et al. (2017) Dry weight accumulation, root plasticity, and stomatal conductance in rice (Oryza sativa L.) varieties under drought stress and re-watering conditions. Amer J Plant Sci 8: 3189-3206. |
[39] | Wasaya A, Zhang X, Fang Q, et al. (2018) Root phenotyping for drought tolerance: A review. Agronomy 8: 241-260. |
[40] | Lakitan B, Alberto A, Lindiana L, et al. (2018b) The benefits of biochar on rice growth and yield in tropical riparian wetland, South Sumatra, Indonesia. Chiang Mai Univ J Nat Sci 17: 111-126. |
[41] | Cornelissen G, Martinsen V, Shitumbanuma V, et al. (2013). Biochar effect on maize yield and soil characteristics in five conservation farming sites in Zambia. Agronomy, 3: 256-274. |
1. | Farinaz Forouzannia, Vahid Shahrezaei, Mohammad Kohandel, The impact of random microenvironmental fluctuations on tumor control probability, 2021, 509, 00225193, 110494, 10.1016/j.jtbi.2020.110494 | |
2. | Anuraag Bukkuri, Kenneth J. Pienta, Robert H. Austin, Emma U. Hammarlund, Sarah R. Amend, Joel S. Brown, A mathematical investigation of polyaneuploid cancer cell memory and cross-resistance in state-structured cancer populations, 2023, 13, 2045-2322, 10.1038/s41598-023-42368-8 | |
3. | Anuraag Bukkuri, Modeling stress-induced responses: plasticity in continuous state space and gradual clonal evolution, 2024, 143, 1431-7613, 63, 10.1007/s12064-023-00410-3 |
Image features | exp(coef) | exp(coef) lower 95% | exp(coef) upper 95% | Wald test | p value |
TEXTURE_GLSZM_ET_T1Gd_SZLGE* | 0 | 0 | 0 | 3.12 | 0.00178 |
HISTO_ED_T2_Bin8* | 0.7 | 0.55 | 0.88 | 3.02 | 0.00254 |
TEXTURE_GLOBAL_ET_T1Gd_Skewness* | 3.03E+05 | 47.29 | 1.94E+09 | 2.82 | 0.00477 |
TEXTURE_GLRLM_NET_FLAIR_LRHGE* | 1 | 0.99 | 1 | 2.66 | 0.0078 |
HISTO_NET_T1_Bin4* | 0.89 | 0.8 | 0.98 | 2.42 | 0.01559 |
HISTO_ET_T1Gd_Bin10* | 1.19 | 1.03 | 1.38 | 2.35 | 0.01877 |
TEXTURE_GLSZM_NET_T1Gd_ZSV* | 0 | 0 | 0 | 2.34 | 0.01906 |
TEXTURE_GLRLM_NET_T1Gd_GLV* | 2.00E+42 | 2230.45 | 1.79E+81 | 2.13 | 0.0333 |
HISTO_ET_T1_Bin10 | 0.81 | 0.63 | 1.05 | 1.59 | 0.11219 |
TEXTURE_GLCM_ET_T2_SumAverage | 0 | 0 | 9.95E+83 | 1.57 | 0.11569 |
TEXTURE_GLRLM_NET_T1_LGRE | 0 | 0 | 2.73E+38 | 1.49 | 0.13526 |
TEXTURE_GLRLM_ED_T1_RLV | inf | 0 | inf | 1.4 | 0.16185 |
HISTO_ED_T2_Bin4 | 0.96 | 0.88 | 1.05 | 0.91 | 0.36241 |
TEXTURE_GLCM_ED_FLAIR_Energy | inf | 0 | inf | 0.78 | 0.43387 |
TEXTURE_GLSZM_NET_T1_LZLGE | 0.99 | 0.96 | 1.03 | 0.39 | 0.69976 |
Image features | Associated gene modules | Number of associated genes |
HISTO_ED_T2_Bin8 | module2, module4, module5, module7 | 2794 |
TEXTURE_GLSZM_NET_T1Gd_ZSV | module6 | 506 |
TEXTURE_GLRLM_NET_FLAIR_LRHGE | module6 | 506 |
TEXTURE_GLRLM_NET_T1Gd_GLV | module2, module4, module6, module8, module10 | 1421 |
Image feature | Number of genes in associated modules | Optimal | RMSE | Number of genes selected by Lasso |
HISTO_ED_T2_Bin8 | 2794 | 1.6627 | 6.0847 | 12 |
TEXTURE_GLSZM_NET_T1Gd_ZSV | 506 | 7.81E-06 | 2.0195E-5 | 3 |
TEXTURE_GLRLM_NET_FLAIR_LRHGE | 506 | 163.9677 | 528.16 | 6 |
TEXTURE_GLRLM_NET_T1Gd_GLV | 1421 | 3.01E-03 | 0.0120 | 18 |
Populations sizes | 20 | 25 | 30 | 35 | 40 |
0.2926 | 0.3187 | 0.2792 | 0.3303 | 0.276 | |
0.0663 | 0.0394 | 0.0739 | 0.0505 | 0.068 | |
0.2171 | 0.2329 | 0.2076 | 0.2214 | 0.2102 | |
0.0091 | 0.019 | 0.0107 | 0.0298 | 0.0159 | |
0.4149 | 0.39 | 0.4288 | 0.368 | 0.4298 | |
C-index | 0.8065 | 0.807 | 0.8061 | 0.807 | 0.8057 |
Image features | ||||||
C-index | 0.6945 | 0.7321 | 0.7926 | 0.7985 | 0.7627 | 0.8071 |
Image features | exp(coef) | exp(coef) lower 95% | exp(coef) upper 95% | Wald test | p value |
TEXTURE_GLSZM_ET_T1Gd_SZLGE* | 0 | 0 | 0 | 3.12 | 0.00178 |
HISTO_ED_T2_Bin8* | 0.7 | 0.55 | 0.88 | 3.02 | 0.00254 |
TEXTURE_GLOBAL_ET_T1Gd_Skewness* | 3.03E+05 | 47.29 | 1.94E+09 | 2.82 | 0.00477 |
TEXTURE_GLRLM_NET_FLAIR_LRHGE* | 1 | 0.99 | 1 | 2.66 | 0.0078 |
HISTO_NET_T1_Bin4* | 0.89 | 0.8 | 0.98 | 2.42 | 0.01559 |
HISTO_ET_T1Gd_Bin10* | 1.19 | 1.03 | 1.38 | 2.35 | 0.01877 |
TEXTURE_GLSZM_NET_T1Gd_ZSV* | 0 | 0 | 0 | 2.34 | 0.01906 |
TEXTURE_GLRLM_NET_T1Gd_GLV* | 2.00E+42 | 2230.45 | 1.79E+81 | 2.13 | 0.0333 |
HISTO_ET_T1_Bin10 | 0.81 | 0.63 | 1.05 | 1.59 | 0.11219 |
TEXTURE_GLCM_ET_T2_SumAverage | 0 | 0 | 9.95E+83 | 1.57 | 0.11569 |
TEXTURE_GLRLM_NET_T1_LGRE | 0 | 0 | 2.73E+38 | 1.49 | 0.13526 |
TEXTURE_GLRLM_ED_T1_RLV | inf | 0 | inf | 1.4 | 0.16185 |
HISTO_ED_T2_Bin4 | 0.96 | 0.88 | 1.05 | 0.91 | 0.36241 |
TEXTURE_GLCM_ED_FLAIR_Energy | inf | 0 | inf | 0.78 | 0.43387 |
TEXTURE_GLSZM_NET_T1_LZLGE | 0.99 | 0.96 | 1.03 | 0.39 | 0.69976 |
Image features | Associated gene modules | Number of associated genes |
HISTO_ED_T2_Bin8 | module2, module4, module5, module7 | 2794 |
TEXTURE_GLSZM_NET_T1Gd_ZSV | module6 | 506 |
TEXTURE_GLRLM_NET_FLAIR_LRHGE | module6 | 506 |
TEXTURE_GLRLM_NET_T1Gd_GLV | module2, module4, module6, module8, module10 | 1421 |
Image feature | Number of genes in associated modules | Optimal | RMSE | Number of genes selected by Lasso |
HISTO_ED_T2_Bin8 | 2794 | 1.6627 | 6.0847 | 12 |
TEXTURE_GLSZM_NET_T1Gd_ZSV | 506 | 7.81E-06 | 2.0195E-5 | 3 |
TEXTURE_GLRLM_NET_FLAIR_LRHGE | 506 | 163.9677 | 528.16 | 6 |
TEXTURE_GLRLM_NET_T1Gd_GLV | 1421 | 3.01E-03 | 0.0120 | 18 |
Populations sizes | 20 | 25 | 30 | 35 | 40 |
0.2926 | 0.3187 | 0.2792 | 0.3303 | 0.276 | |
0.0663 | 0.0394 | 0.0739 | 0.0505 | 0.068 | |
0.2171 | 0.2329 | 0.2076 | 0.2214 | 0.2102 | |
0.0091 | 0.019 | 0.0107 | 0.0298 | 0.0159 | |
0.4149 | 0.39 | 0.4288 | 0.368 | 0.4298 | |
C-index | 0.8065 | 0.807 | 0.8061 | 0.807 | 0.8057 |
Image features | ||||||
C-index | 0.6945 | 0.7321 | 0.7926 | 0.7985 | 0.7627 | 0.8071 |