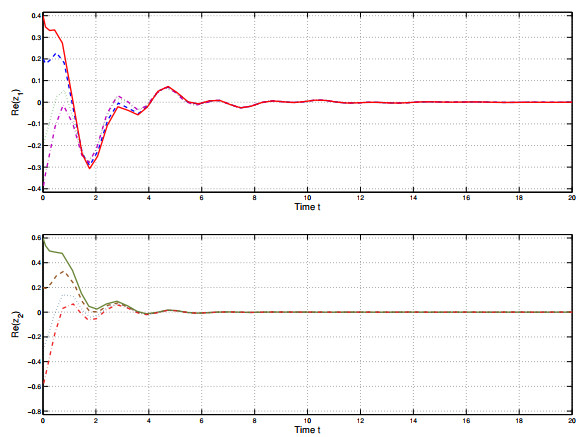
Italian olive oil companies play a significant role in this nation's economy, which is among the top in the world for its geomorphological and meteorological characteristics. This research analyzed the performance of three profitability ratios (return on equity (R.O.E.), return on investment (R.O.I.), and return on sales (R.O.S.)) of 3184 companies from 2013 to 2022. Average ratios for each year and critical descriptive statistics were calculated. Broken lines and interpolating curves, obtained from sixth-degree polynomial equations maximizing R2, represent the trends. One-way ANOVA and Tukey-Kramer methods facilitated statistical comparisons between macro-regions. Despite the regular consumption of olive oil, the profitability of businesses has been erratic and fluctuating, probably due to the varying productivity of raw material crops. The pandemic seems to have had no impact. There are no statistically significant differences between macro-areas. The results are helpful to Italian and foreign entrepreneurs who can relate their situation to the average situation in context, highlighting possible gaps that, if negative, must be bridged with a timely management review. National and supranational political authorities can also use this study to orient the frequent support policies in the agricultural and agro-industrial sectors. So too can the bodies in charge of food education, especially for young people, can encourage the use of olive oil where it is lacking. The main limitation of this study was its focus on a small set of profitability ratios. In the future, the study should consider other profitability and asset ratios and investigate investments in sustainability, keeping in mind that all enterprises should contribute to developing eco-friendly production systems.
Citation: Guido Migliaccio, Antonella De Blasio. The economic performance of Italian olive oil companies: a comparative quantitative approach using the Anova and Tukey-Kramer methods[J]. Quantitative Finance and Economics, 2024, 8(3): 437-465. doi: 10.3934/QFE.2024017
[1] | Huaying Liao, Zhengqiu Zhang . Finite-time stability of equilibrium solutions for the inertial neural networks via the figure analysis method. Mathematical Biosciences and Engineering, 2023, 20(2): 3379-3395. doi: 10.3934/mbe.2023159 |
[2] | Pan Wang, Xuechen Li, Qianqian Zheng . Synchronization of inertial complex-valued memristor-based neural networks with time-varying delays. Mathematical Biosciences and Engineering, 2024, 21(2): 3319-3334. doi: 10.3934/mbe.2024147 |
[3] | Biwen Li, Xuan Cheng . Synchronization analysis of coupled fractional-order neural networks with time-varying delays. Mathematical Biosciences and Engineering, 2023, 20(8): 14846-14865. doi: 10.3934/mbe.2023665 |
[4] | Zhen Yang, Zhengqiu Zhang, Xiaoli Wang . New finite-time synchronization conditions of delayed multinonidentical coupled complex dynamical networks. Mathematical Biosciences and Engineering, 2023, 20(2): 3047-3069. doi: 10.3934/mbe.2023144 |
[5] | Na Zhang, Jianwei Xia, Tianjiao Liu, Chengyuan Yan, Xiao Wang . Dynamic event-triggered adaptive finite-time consensus control for multi-agent systems with time-varying actuator faults. Mathematical Biosciences and Engineering, 2023, 20(5): 7761-7783. doi: 10.3934/mbe.2023335 |
[6] | Ruoyu Wei, Jinde Cao . Prespecified-time bipartite synchronization of coupled reaction-diffusion memristive neural networks with competitive interactions. Mathematical Biosciences and Engineering, 2022, 19(12): 12814-12832. doi: 10.3934/mbe.2022598 |
[7] | Yanjie Hong, Wanbiao Ma . Sufficient and necessary conditions for global attractivity and stability of a class of discrete Hopfield-type neural networks with time delays. Mathematical Biosciences and Engineering, 2019, 16(5): 4936-4946. doi: 10.3934/mbe.2019249 |
[8] | Rui Ma, Jinjin Han, Li Ding . Finite-time trajectory tracking control of quadrotor UAV via adaptive RBF neural network with lumped uncertainties. Mathematical Biosciences and Engineering, 2023, 20(2): 1841-1855. doi: 10.3934/mbe.2023084 |
[9] | Hai Lin, Jingcheng Wang . Pinning control of complex networks with time-varying inner and outer coupling. Mathematical Biosciences and Engineering, 2021, 18(4): 3435-3447. doi: 10.3934/mbe.2021172 |
[10] | Zixiao Xiong, Xining Li, Ming Ye, Qimin Zhang . Finite-time stability and optimal control of an impulsive stochastic reaction-diffusion vegetation-water system driven by Lˊevy process with time-varying delay. Mathematical Biosciences and Engineering, 2021, 18(6): 8462-8498. doi: 10.3934/mbe.2021419 |
Italian olive oil companies play a significant role in this nation's economy, which is among the top in the world for its geomorphological and meteorological characteristics. This research analyzed the performance of three profitability ratios (return on equity (R.O.E.), return on investment (R.O.I.), and return on sales (R.O.S.)) of 3184 companies from 2013 to 2022. Average ratios for each year and critical descriptive statistics were calculated. Broken lines and interpolating curves, obtained from sixth-degree polynomial equations maximizing R2, represent the trends. One-way ANOVA and Tukey-Kramer methods facilitated statistical comparisons between macro-regions. Despite the regular consumption of olive oil, the profitability of businesses has been erratic and fluctuating, probably due to the varying productivity of raw material crops. The pandemic seems to have had no impact. There are no statistically significant differences between macro-areas. The results are helpful to Italian and foreign entrepreneurs who can relate their situation to the average situation in context, highlighting possible gaps that, if negative, must be bridged with a timely management review. National and supranational political authorities can also use this study to orient the frequent support policies in the agricultural and agro-industrial sectors. So too can the bodies in charge of food education, especially for young people, can encourage the use of olive oil where it is lacking. The main limitation of this study was its focus on a small set of profitability ratios. In the future, the study should consider other profitability and asset ratios and investigate investments in sustainability, keeping in mind that all enterprises should contribute to developing eco-friendly production systems.
Hopfield neural network (HNN) is in the powerful class of artificial neural networks (ANNs), which has been introduced in the literature by John Hopfield in 1982. Since then, its investigation has become a worldwide focus [1,2,3,4,5]. All of those models are real-valued neural networks (NNs). However, complex-valued neural networks (CVNNs) can handle problems that cannot be handled with real-valued parallel networks [6,7]. Recently, some authors started the dynamical analysis of CVNNs. For example, in [8], Ali et al. studied the finite time stability analysis of delayed fractional-order memristive CVNNs by using the Gronwall inequality, H¨older inequality and inequality scaling skills. In [9], Zhang and Cao investigated the existence and global exponential stability of periodic solutions of neutral type CVNNs by collecting the Lyapunov functional method with the coincidence degree theory as well as graph theory. In article [21], the authors dealt with the problem stability of impulsive CVNNs with time delay.
In the functioning of ANNs, time-delays always exist in the signal transmission between neurons on account to the limited pace of signal exchange and transmission. Thus, it is one of the main reasons poor performance and instability in a system is the presence of delays. Therefore, in recent years, the analysis of the stability of delayed ANNs has been the great attention of researchers, and various results have been discussed in the literature [16,17,18,19,20,21,22].
ANNs with neutral-type delays were also very seldom discussed in the existing literature; such models are called neutral-type ANNs. They have been the subject of in-depth studies by many researchers, for instance, Guo and Du [17], concerned with the global exponential stability of periodic solution for neutral-type CVNNs.
The theory of passivity is a significant notion of automatic for the analysis and control of models whose certain input/output characteristics are established in terms of energy criteria. The notions of passivity are adapted to several scientific fields and are effective for the regulation of electrical, mechanical, and electromechanical systems present in several fields of engineering, such as robotics, power electronics, aeronautics, etc. Many results are concentrated in the research of the passivity of NN systems. In [11], Li and Zheng proved the global exponential passivity for delayed quaternion-valued memristor-based NNs. Ge et al. [12] studied the robust passivity analysis for a class of uncertain NNs subject to mixed delays. The problem of passivity analysis for uncertain bidirectional associative memory NNs in the presence of mixed delays are discussed in [13]. In [35], Khonchaiyaphum et al. studied FTP analysis of neutral-type NNs.
Many researchers have been interested in finite-time control problems of dynamical systems based on the Lyapunov theory of stability to give big attention to the asymptotic model of systems over an infinite time interval. Recently, many interested results have been published. In [14], Thuan et al. discussed the robust FTP for a fractional order NNs with uncertainties. In paper [19], Wei et al. are focused on a class of coupled quaternion-valued NNs with several delayed couplings to study fixed-time passivity.
The main objective of this manuscript is to deal with the FTP for the following neutral-type CVNNs:
{˙z(t)=−Az(t)+Bf(z(t))+Cf(z(t−τ(t)))+D˙z(t−h(t))+ω(t),u(t)=K1z(t)+K2f(z(t)). | (1.1) |
Here, we have
∘z(⋅)=[z1(⋅),z2(⋅),⋯,zn(⋅)]T∈Cn is the complex valued state vector,
∘ω(⋅)∈Cn is the disturbance input which belongs to L2[0,+∞),p
∘u(⋅)∈Cn is the control output,
∘f(z(⋅))=[f(z1(⋅)),f(z2(⋅)),⋯,f(zn(⋅))]T∈Cn is the complex-valued activation function,
∘A=diag{a1,a2,⋯,an}>0∈Rn×n is a diagonal matrix,
∘B=(bjk)∈Cn×n,C=(cjk)∈Cn×n,D=(djk)∈Cn×n are respectively the connection weight matrix and the connection weight matrix with delays,
∘K1 is a known real constant matrice with appropriate dimension,
∘K2 is a known complex matrice with appropriate dimension.
The initial condition of (1.1) is given as
z(s)=ψ(s),s∈[−ρ,0], | (1.2) |
where ρ=max{supt∈R(τ(t),supt∈R(h(t))}.
The main contributions of our article are :
∘ We use a more adequate hypothesis for the complex-valued activation functions (CVAF) considered in our model. Based on this assumption, a controllable model is formed by divised the real and imaginary parts, and we give a sufficient condition to realize FTP. This result is more general than the existing passivity results on real-valued NNs [35,36,37].
∘ A Lyapunov–Krasovskii function that support triple, four, and five integral terms is introduced, and the Wirtinger-type inequality technique and convex combination approach are espoused.
∘ A new set of sufficient conditions in terms of LMIs is derived to guarantee FTB and FTP results. These conditions can be simply found by the Matlab LMI toolbox.
The main contents of the manuscript are outlined as follows: in Section 2, we established new assumptions, definitions, and lemmas for the dynamic systems (1.1), which will be used later. New sufficient conditions on the FTB and FTP are discussed in Section 3. Two examples are presented in Section 4 to verify our results. At last, in Section 5, we end with the conclusion and perspective.
In this section, we present new necessary assumptions and some definitions and lemmas, which are utilized in the next section.
Assumption 1: The delays τ(⋅) and h(⋅) are differentiable functions satisfies the inequalities below:
0≤τ(t)≤ˉτ, ˙τ(t)≤μ,0≤h(t)≤ˉh, ˙h(t)≤h1. |
Assumption 2: The neuron activation function fk(⋅) satisfy the below Lipschitz condition:
|fk(z1)−fk(z2)|≤lk|z1−z2|,lk>0(k=1,2,⋯,n). | (2.1) |
Furthermore, by means of Assumption 2, it is clear to get
(f(z1)−f(z2))∗(f(z1)−f(z2))≤(z1−z2)∗LTL(z1−z2), | (2.2) |
where L=diag{l1,l2,⋯,ln}.
Assumption 3: The neuron activation functions f(⋅) can be divided into two parts as follows:
f(z)=fR(x,y)+ifI(x,y), |
where z=x+iy,i shows the imaginary unit, and the real imaginary parts check the below conditions:
1) The partial derivatives ∂f∂x,∂f∂y exist and are continuous.
2) There exist positive constant numbers γRRk,γRIk,γIRk,γIIk such that
∂fRk∂x≤γRRk,∂fRk∂y≤γRIk,∂fIk∂x≤γIRk,∂fIk∂y≤γIIk. |
Thus, one can obtain that for any x1,x2,y1,y2∈R,
|fRk(x1,y1)−fRk(x2,y2)|≤γRRk|x1−x2|+γRIk|y1−y2|,|fIk(x1,y1)−fIk(x2,y2)|≤γIRk|x1−x2|+γIIk|y1−y2|, |
for all k=1,2,⋯,n.
Remark 1: As we know, in many literatures [29,30,31], after the separation of the activation functions into real-imaginary type, ∂f∂x and ∂f∂y are still supposed to exist and be continuous and limited. Via these conditions, we can point that they checked the same inequalities given in hypothesis 3 by considering the mean value theorem for multivariable functions. Here, we delete these constraints of the partial derivatives and make the hypothesis into an appropriate one that imaginary and real parts of the activation functions just check the inequalities in hypothesis 3. Consequently, our study can help a broader class of CVAF.
Remark 2: By applying the modulus of a complex number to some simple inequalities, it is worth mentioning that hypothesis 2 is equivalent to hypothesis 3. Therefore, for writing convenience, we will assume that activation functions satisfy hypothesis 2 to study our main results.
Assumption 4: The neuron activation function fk(⋅) may be divided into two parts according to the complex number z as follows
fk(z)=fRk(Re(z))+ifIk(Im(z)) |
where fRk(⋅),fIk(⋅):R→R, then for any k=1,2,⋯,n, there exist constants ˇlRk,ˆlRk,ˇlIkˆlIk such that
ˇlRk≤fRk(κ1)−fRk(κ2)κ1−κ2≤ˆlRk,ˇlIk≤fIk(κ1)−fIk(κ2)κ1−κ2≤ˆlIk, | (2.3) |
where fRk(0)=0,fIk(0)=0,κ1,κ2∈R,κ1≠κ2.
For presentation convenience, we denote
ˆLR=diag{ˆlR1,⋯,ˆlRn},ˇLR=diag{ˇlR1,⋯,ˇlRn},ˆLI=diag{ˆlI1,⋯,ˆlIn},ˇLI=diag{ˇlI1,⋯,ˇlIn},L1=ˆLRˇLR,L2=ˆLIˇLI,L3=ˆLR+ˇLR2,L4=ˆLI+ˇLI2,ˉL1=diag{L1,L2},ˉL2=diag{L3,L4}. |
Remark 3: In [23,24,25], hypotheses 3 and 4 are given on the activation functions which separate between the real and imaginary parts. Moreover, we can even simply say that hypothesis 3 is quite strict and that it is a special case of hypothesis 2. This fact has already been mentioned in [26] and [27]. In addition, hypothesis 4 is also a strong constraint. For instance, if the activation functions fk(z)(k=1,2,⋯,n) checked hypothesis 4, we have:
|fRk(x1)−fRk(x2)|≤lRk|x1−x2|,|fIk(y1)−fIk(y2)|≤lIk|y1−y2| |
where z1=x1+iy1,z2=x2+iy2, lRk=max{|ˇlRk|,|ˆlRk|}, and lIk=max{|ˇlIk|,|ˆlIk|}, then one can have that
|fk(z1)−fk(z2)|=|(fRk(x1)+ifIk(y1))−(fRk(x2)+ifIk(y2))|≤|fRk(x1)−(fRk(x2)|+|fIk(y1)−fIk(y2)|≤lRk|x1−x2|+lIk|y1−y2|≤√(lRk)2+(lIk)2|z1−z2|. |
Thus, the activation function fk(z)(k=1,2,⋯,n) satisfies the Lipschitz condition of hypothesis 2. Therefore, hypotheses 3 and 4 imply hypothesis 2, that is, the condition of hypothesis 2 is a special case of hypotheses 3 and 4.
Remark 4: Indeed, when the activation function is given as follows: fk(z)=fRk(x,y)+ifIk(x,y) includes fk(z)=fRk(Re(z))+ifIk(Im(z)) as a special case. Therefore, in the following section, we will indicate that fk(z) is of the type of distinct real-imaginary activation functions. Let z(t)=x(t)+iy(t),z(t−τ(t))=zτ(t),x(t−τ(t))=xτ(t), y(t−τ(t))=yτ(t),x(t−h(t))=xh(t), y(t−h(t))=yh(t), B=BR+iBI,C=CR+iCI,f(z(t))=fR(x(t),y(t))+ifI(x(t),y(t)), f(zτ(t))=fR(xτ(t),yτ(t))+ifI(xτ(t),yτ(t)), ω(t)=ωR(t)+iωI(t), and u(t)=uR(t)+iuI(t), then model (1.1) can be divided into imaginary and real parts as the following form
{˙x(t)=−Ax(t)+BRfR(x(t),y(t))−BIfI(x(t),y(t))+CRfR(xτ(t),yτ(t))−CIfI(xτ(t),yτ(t))+DR˙xh(t)−DI˙yh(t)+ωR(t),˙y(t)=−Ay(t)+BIfR(x,y)(t))+BRfI(x(t),y(t))+CIfR(xτ(t),yτ(t))+CRfI(xτ(t),yτ(t))+DR˙yh(t)+DI˙xh(t)+ωI(t),uR(t)=K1x(t)+KR2fR(x(t),y(t))−KI2fI(x(t),y(t)),uI(t)=K1y(t)+KI2fR(x(t),y(t))+KR2fI(x(t),y(t)), | (2.4) |
The initial condition of (2.4) is given by:
{x(s)=φR(s),s∈[−ρ,0],y(s)=φI(s),s∈[−ρ,0], |
where φR(s),φI(s)∈C([−ρ,0],Rn).
Let θ=(xy), ˉf(θ)=(fR(x,y)fI(x,y)), ˉf(θτ)=(fR(xτ,yτ)fI(xτ,yτ)), ˉω=(ωRωI), ˉA=(A00A), ˉB=(BR−BIBIBR), ˉC=(CR−CICICR), ˉD=(DR−DIDIDR), ˉu=(uRuI),ˉK1=(K100K1),ˉK2=(KR1−KI1KI1KR1).
Model (2.4) can be rewritten as:
{˙θ(t)=−ˉAθ(t)+ˉBˉf(θ(t))+ˉCˉf(θτ(t))+ˉD˙θh(t)+ˉω(t),ˉu(t)=ˉK1θ(t)+ˉK2ˉf(θ(t)), | (2.5) |
with
θ(s)=ϕ(s),s∈[−ρ,0], |
where ϕ(s)=(φR(s)φI(s))∈C([−ρ,0],R2n).
The norm is defined as follows: For every ϕ(⋅)∈C([−ρ,0],R2n), ‖ϕ‖ˆh=max{‖ϕ‖,‖ϕ′‖}=max{supς∈[−ρ,0]|ϕ(θ)|,supς∈[−ρ,0]|ϕ′(ς)|}.
It is clear from (2.3) that:
ˉL−k≤ˉfk(κ1)−ˉfk(κ2)κ1−κ2≤ˉL+k, | (2.6) |
where, ˉL−=(ˇLR00ˇLI),ˉL+=(ˆLR00ˆLI).
Assumption 5: Given a positive value bR,bI, then ωR(t),ωI(t) checks
∫T10(ωR)T(t)ωR(t)dt≤bR,∫T10(ωI)T(t)ωI(t)dt≤bI,T1≥0,bR≥0,bI≥0,⇒∫T10ˉωT(t)ˉω(t)dt≤ˉb, | (2.7) |
where ˉb=(bRbI).
Definition 1 (FTB): For a known constant T1>0, system (1.1) is FTB with regard to (ˉc1,ˉc2,T1,ˉL,ˉb), and ˉω(⋅) checked (2.7) if: supt0∈[−ˉτ,0]{θT(t0)ˉLθ(t0),˙θT(t0)ˉL˙θ(t0)}≤ˉc1⇒θT(t)ˉLθ(t)≤ˉc2, for t∈[0,T1], where ˉc1=(c11c12),ˉc2=(c21c22), 0<c11<c21,0<c12<c22, ˉL>0 is a matrix.
Definition 2(FTP): For a known constant T1>0, system (1.1) is said to be FTP, with regard to (ˉc1,ˉc2,T1,ˉL,ˉb), if the beelow conditions are checked:
(ⅰ) Model (1.1) is FTB with regard to (ˉc1,ˉc2,T1,ˉL,ˉb).
(ⅱ) For a known constant γ>0, and since the zero initial condition, the below relation is true:
2∫T10ˉuT(t)ˉω(t)dt≥−γ∫T10ˉωT(t)ˉω(t)dt, |
when ˉω(⋅) checked (2.7).
Lemma 1 [28]: For a symmetric matrix ϑ=ϑT≥0, scalars e1>e2>0, such that the integrations below are well defined. We have
−(e1−e2)∫t−e2t−e1θT(s)ϑθ(s)ds≤−(∫t−e2t−e1θ(s)ds)Tϑ(∫t−e2t−e1θ(s)ds),−(e21−e22)2∫−e2−e1∫tt+sθT(u)ϑθ(u)duds≤−(∫−e2−e1∫tt+sθ(u)duds)Tϑ(∫−e2−e1∫tt+sθ(u)duds),−(e31−e32)6∫−e2−e1∫0s∫tt+uθT(v)ϑθ(v)dvduds≤−(∫−e2−e1∫0s∫tt+uθ(v)dvdudλds)Tθ×(∫−e2−e1∫0s∫tt+uθ(v)dvdudλds). | (2.8) |
Lemma 2: For a symmetric matrix ϑ=ϑT≥0, scalars e1>e2>0, such that the integrations below are well defined. We have
−(e41−e42)24∫−e2−e1∫0s∫0λ∫tt+uθT(v)ϑθ(v)dvdudλds≤−(∫−e2−e1∫0s∫0λ∫tt+uθ(v)dvdudλds)Tϑ×(∫−e2−e1∫0s∫0λ∫tt+uθ(v)dvdudλds). | (2.9) |
Proof: The proof of Lemma 2 is inspired by the proof of Lemma 2 in [28].
For any symmetric matrix ϑ=ϑT≥0, we have
[θT(v)ϑθ(v)θT(v)θ(v)ϑ−1]≥0, |
then after integration of it from t+u to t, from λ to 0, from s to 0, and from −e1 to −e2 in turn, we can obtain
(Π11Π12ΠT12Π22)≥0, |
where
Π11=∫−e2−e1∫0s∫0λ∫tt+uθT(v)ϑθ(v)dvdudλds,Π12=∫−e2−e1∫0s∫0λ∫tt+uθT(v)dvdudλds,Π22=∫−e2−e1∫0s∫0λ∫tt+uϑ−1dvdudλds=∫−e2−e1∫0s∫0λ−uϑ−1dudλds=∫−e2−e1∫0s[−u22ϑ−1]0λdλds=∫−e2−e1[λ36ϑ−1]0sds=[−s424M−1]−e2−e1=e41−e4224ϑ−1. |
According to Schur complement, [16] is equivalent to the below condition: Π22>0,Π11−Π12Π−122ΠT12≥0. ⇒Π11≥Π12Π−122ΠT12, which is equivalent to inequality (2.9).
This completes the proof.
Lemma 3 [38]: For a given symmetric definite matrix ξ>0 and for any differentiable function ζ(⋅):[e1,e2]→Rn, the below inequality holds:
∫e2e1˙ζT(s)ξ˙ζ(s)ds≥1e2−e1(ζ(e2)ζ(e1)χ)T×Ξ2(ξ)(ζ(e2)ζ(e1)χ), |
where χ=1e2−e1∫e2e1ζ(s)ds, Ξ2(ξ)=(ξ−ξ0⋆ξ0000)+π24(ξξ−2ξ⋆ξ−2ξ⋆⋆4ξ).
Remark 5: In this manuscript, our goal is to know how to determine the sufficient condition to study the FTB and FTP of the proposed model. So, to get the desired objectives, a processable whole model (2.5) is first formed by dividing the initial model into imaginary and real parts and founding an equivalent real-valued model. Second, using the Lyapunov function approach, the design procedure can be easily performed by checked the LMIs, so in the next section the expected conditions will be obtained.
In this section, we will concentrate on the problem of FTB and FTP.
In this first part, our goal is to study the FTB in the following system:
˙θ(t)=−ˉAθ(t)+ˉBˉf(θ(t))+ˉCˉf(θτ(t))+ˉD˙θh(t)+ˉω(t). | (3.1) |
Theorem 1: Suppose that Assumptions 1–5 hold. Let ˉτ,μ,ˉh,h1, and δ be positive scalars, then system (3.1) is FTB, with respect to (ˉc1, ˉc2, T1, ˉL, ˉb), if there exists symmetric positive definite matrices P, Q1, Q2, R01, R1, R02, R2, R3, R4 R5 R6, T01, T2, T3 and diagonal matrices M1>0, M2>0, M3>0, such that the following LMIs hold:
Ω=[η1,1ˉL1M3η1,3η1,4η1,5G1Dη1,7η1,80η1,11Π22ˉhR6ˉτ22T2ˉτ36T3G1⋆η2,20000−MT3FT2η2,8000000⋆⋆η3,3000000Π22ˉτR40000⋆⋆⋆η4,4000000Π22ˉhR6000⋆⋆⋆⋆η5,5G2ˉDη5,7G2ˉC00000G2⋆⋆⋆⋆⋆η6,600000000⋆⋆⋆⋆⋆⋆η7,7M3000000⋆⋆⋆⋆⋆⋆⋆η8,8000000⋆⋆⋆⋆⋆⋆⋆⋆η9,900000⋆⋆⋆⋆⋆⋆⋆⋆⋆η10,100000⋆⋆⋆⋆⋆⋆⋆⋆⋆⋆η11,11000⋆⋆⋆⋆⋆⋆⋆⋆⋆⋆⋆−T200⋆⋆⋆⋆⋆⋆⋆⋆⋆⋆⋆⋆−T30⋆⋆⋆⋆⋆⋆⋆⋆⋆⋆⋆⋆⋆−δI]<0, | (3.2) |
ˉc1Γ+ˉb(1−e−δT1)<ˉc2λ1e−δT1, | (3.3) |
where
η1,1=R01+R1−R4−Π24R4+ˉτ2R5−R6−Π24R6−ˉτ2T01−ˉτ44T2−ˉτ66T3−ˉL1M1−ˉL1M3−G1ˉA−ˉATGT1−δP,η1,3=R4−π24R4,η1,4=R6−Π24R6,η1,5=P−ˉL−Q1+ˉL+Q2−G1−ˉATGT2,η1,7=ˉL2M1+ˉL2M3+G1ˉB,η1,11=Π22ˉτR4+ˉτT01,η2,2=−(1−μ)R01−ˉL1M2−ˉL1M3,η2,8=ˉL2M2+MT3LT2,η3,3=−R4−π24R4−R1,η4,4=−R6−Π24R6,η5,5=R3+ˉτ2R4+ˉh2R6+ˉτ44T01+ˉτ636T2+ˉτ8576T3−G2−GT2,η5,7=QT1−QT2+G2ˉB,η6,6=−(1−h1)R3,η7,7=R02+R2−M1−M3,η8,8=−(1−μ)R02−M2−M3,η9,9=−R2,η10,10=−Π2ˉτ2R4−R5−T01,η11,11=−Π2ˉh2R6. |
Proof: We consider the Lyapunov functional below:
V(θ(t))=5∑i=1Vi(θ(t)), | (3.4) |
where
V1(θ(t))=θT(t)Pθ(t),V2(θ(t))=2∫θ(t)0Q1(ˉf(s)−ˉL−s)+Q2(ˉL+s−ˉf(s))ds,V3(θ(t))=∫tt−τ(t)θT(s)R01θ(s)ds+∫tt−τθT(s)R1θ(s)ds+∫tt−τ(t)ˉfT(θ(s))R02ˉf(θ(s))ds+∫tt−ˉτˉfT(θ(s))R2ˉf(θ(s))ds,V4(θ(t))=∫tt−h(t)˙θT(s)R3˙θ(s)ds+ˉτ∫0−ˉˉτ∫tt+β˙θT(s)R4˙θ(s)dsdβ+ˉτ∫0−ˉτ∫tt+βθT(s)R5θ(s)dsdβ+ˉh∫0−ˉh∫tt+β˙θT(s)R6˙θ(s)dsdβ,V5(θ(t))=ˉτ22∫0−ˉτ∫0γ∫tt+β˙θT(s)T01˙θ(s)dsdβdγ+ˉτ36∫0−ˉτ∫0λ∫0γ∫tt+β˙θT(s)T2˙θ(s)dsdβdγdλ+ˉτ424∫0−ˉτ∫0λ∫0α∫0γ∫tt+β˙θT(s)T3˙θ(s)dsdβdγdαdλ. |
Calculating the time-derivative of V(θ(⋅)) along any trajectory of model (3.1), we obtain
˙V(θ(t))=5∑i=1˙Vi(θ(t)), | (3.5) |
where
˙V1(θ(t))=2θT(t)P˙θ(t),˙V2(θ(t))=2(ˉf(θ(t))−ˉL−θ(t))TQ1˙θ(t)+2(ˉL+θ(t)−ˉf(θ(t)))TQ2˙θ(t),=2ˉf(θ(t))TQ1˙θ(t)−2θ(t)TˉL−Q1˙θ(t)+2θT(t)ˉL+Q2˙θ(t)−2ˉf(θ(t))TQ2˙θ(t),˙V3(θ(t))≤θT(t)[R01+R1]θ(t)+ˉfT(θ(t))[R02+R2]ˉf(θ(t))−(1−μ)ˉfT(θ(t−τ(t)))R02ˉf(θ(t−τ(t)))−θT(t−ˉτ)R1θ(t−ˉτ)−(1−μ)θT(t−τ(t))R01θ(t−τ(t))−ˉfT(θ(t−ˉτ))R2ˉf(θ(t−ˉτ)), |
˙V4(θ(t))=˙θT(t)R3˙θ(t)−(1−˙h(t))˙θT(t−h(t))R3˙θ(t−h(t))+ˉτ∫0−ˉτ˙θT(t)R4˙θ(t)−˙θT(t+β)R4˙θ(t+β)dβ+ˉτ∫0−ˉτθT(t)R5θ(t)−θT(t+β)R5θ(t+β)dβ+ˉh∫0−ˉh˙θT(t)R6˙θ(t)−˙θT(t+β)R6˙θ(t+β)dβ≤˙θT(t)R3˙θ(t)−(1−h1)˙θT(t−h(t))R3˙θ(t−h(t))+ˉτ2˙θT(t)R4˙θ(t)−ˉτ∫tt−ˉτ˙θT(s)R4˙θ(s)ds+ˉτ2θT(t)R5θ(t)−ˉτ∫tt−ˉτθT(s)R5θ(s)ds+ˉh2˙θT(t)R6˙θ(t)−ˉh∫tt−ˉh˙θT(s)R6˙θ(s)ds. |
Applying Lemma 3 to the above inequality, we get
−ˉτ∫tt−ˉτ˙θT(s)R4˙θ(s)ds≤−(θ(t)θ(t−ˉτ)1ˉτ∫tt−ˉτθ(s)ds)T×Ξ2(R4)(θ(t)θ(t−ˉτ)1ˉτ∫tt−ˉτθ(s)ds), |
where
Ξ2(R4)=(R4−R40⋆R40000)+Π24(R4R4−2R4⋆R4−2R4⋆⋆4R4), |
−ˉτ∫tt−ˉτ˙θT(s)R4˙θ(s)ds≤−[θT(t)(R4+Π24R4)θ(t)+2θT(t)(−R4+Π24R4)θ(t−ˉτ)+2θT(t)(−Π22ˉτR4)∫tt−ˉτθ(s)ds+θT(t−ˉτ)(R4+Π24R4)θ(t−ˉτ)+2θT(t−ˉτ)(−Π22ˉτR4)∫tt−ˉτθ(s)ds+(∫tt−ˉτθT(s)ds)Π2ˉτ2R4∫tt−ˉτθ(s)ds]. |
Similary,
−ˉh∫tt−ˉh˙θT(s)R6˙θ(s)ds≤−[θT(t)(R6+Π24R6)θ(t)+2θT(t)(−R6+Π24R6)θ(t−ˉh)+2θT(t)(−Π22ˉhR6)∫tt−ˉhθ(s)ds+θT(t−ˉh)(R6+Π24R6)θ(t−ˉh)+2θT(t−ˉh)(−Π22ˉhR6)∫tt−ˉhθ(s)ds+∫tt−ˉhθT(s)ds(Π2ˉh2R6)∫tt−ˉhθ(s)ds], |
and by applying Lemma 1,
−ˉτ∫tt−ˉτθT(s)R5θ(s)ds≤−(∫tt−ˉτθT(s)ds)TR5(∫tt−ˉτθ(s)ds). |
˙V5(θ(t))=ˉτ44˙θT(t)T01˙θ(t)−ˉτ22∫0−ˉτ∫tt+γ˙θT(s)T01˙θ(s)dsdγ+ˉτ636˙θT(t)T2˙θ(t)−ˉτ36∫0−ˉτ∫0λ∫tt+γ˙θT(s)T2˙θ(s)dsdγdλ+ˉτ8576˙θT(t)T3˙θ(t)−ˉτ424∫0−ˉτ∫0λ∫0α∫tt+γ˙θT(s)T3˙θ(s)dsdγdαdλ. |
Applying Lemma 1 and 3 to the above inequality, we get
A1=−ˉτ22∫0−ˉτ∫tt+γ˙θT(s)T01˙θ(s)dsdγA1≤−(∫0−ˉτ∫tt+γ˙θT(s)ds)T01(∫0−ˉτ∫tt+γ˙θ(s)dsdγ)=−[ˉτθT(t)−∫tt−ˉτθT(s)ds]T1[ˉτθ(t)−∫tt−ˉτθ(s)ds]=−ˉτ2θT(t)T01θ(t)+2ˉτθT(t)T01∫tt−ˉτθ(s)ds−(∫tt−ˉτθT(s)ds)T1(∫tt−ˉτθ(s)ds).A2=−ˉτ36∫0−ˉτ∫0λ∫tt+γ˙θT(s)T2˙θ(s)dsdγdλ≤(∫0−ˉτ∫0λ∫tt+γ˙θT(s)dsdγdλ)×T2(∫0−ˉτ∫0λ∫tt+γ˙θ(s)dsdγdλ)=−[ˉτ22θT(t)−∫0−ˉτ∫tt+λθT(s)dsdλ]T2[ˉτ22θ(t)−∫0−ˉτ∫tt+λθ(s)dsdλ]=−ˉτ44θT(t)T2θ(t)+2θT(t)(ˉτ22T2)∫0−ˉτ∫tt+λθ(s)dsdλ−(∫0−ˉτ∫tt+λθT(s)dsdλ)T2(∫0−ˉτ∫tt+λθ(s)dsdλ). |
A3=−ˉτ424∫0−ˉτ∫0λ∫0α∫tt+γ˙θT(s)T3˙θ(s)dsdγdαdλA3≤−(∫0−ˉτ∫0λ∫0α∫tt+γ˙θT(s)dsdγdαdλ)T3(∫0−ˉτ∫0λ∫0α∫tt+γ˙θ(s)dsdγdαdλ)=−[ˉτ36θT(t)−∫0−ˉτ∫0λ∫tt+αθT(s)dsdαdλ]T3[ˉτ36θ(t)−∫0−ˉτ∫0λ∫tt+αθ(s)dsdαdλ]=−ˉτ636θT(t)T3θ(t)+2θT(t)(ˉτ36T3)(∫0−ˉτ∫0λ∫tt+αθ(s)dsdαdλ)−(∫0−ˉτ∫0λ∫tt+αθT(s)dsdαdλ)T3(∫0−ˉτ∫0λ∫tt+αθ(s)dsdαdλ). | (3.6) |
For any ρ1k>0,ρ2k>0,ρ3k>0,k=1,2,⋯,n, it follows from (2.3) that
[ˉfk(θk(t))−ˉL−kθk(t)]Tρ1k[ˉL+kθk(t)−ˉfk(θk(t))]≥0,[ˉfk(θk(t−τ(t)))−ˉL−kθk(t−τ(t))]Tρ2k[ˉL+kθk(t−τ(t))−ˉfk(θk(t−τ(t)))]≥0,[ˉfk(θk(t))−ˉfk(θk(t−τ(t)))−ˉL−k(θk(t)−θk(t−τ(t)))]Tρ3k[ˉL+k(θk(t)−θk(t−τ(t)))−(ˉfk(θk(t))+ˉfk(θk(t−τ(t))))]≥0, |
which implies
(θT(t)ˉfT(θ(t)))(−ˉL1M1ˉL2M1⋆−M1)×(θ(t)ˉf(θ(t)))≥0, | (3.7) |
(θT(t−τ(t))ˉfT(θ(t−τ(t))))(−ˉL1M2ˉL2M2⋆−M2)×(θ(t−τ(t))ˉf(θ(t−τ(t))))≥0, | (3.8) |
and
(θT(t)ˉfT(θ(t))θT(t−τ(t))ˉfT(θ(t−τ(t))))×(−ˉL1M3ˉL2M3ˉL1M3−ˉL1M3⋆−M3−ˉL2M3ˉL3⋆⋆−ˉL1M3ˉL1M3⋆⋆⋆−M3)(θ(t)ˉf(θ(t))θ(t−τ(t))ˉf(θ(t−τ(t))))≥0, | (3.9) |
where M1=diag{ρ11,ρ12,⋯ρ1n},M2=diag{ρ21,ρ22,⋯ρ2n}, M3=diag{ρ31,ρ32,⋯ρ3n}.
Moreover, for any matrices G1 and G2 with appropriate dimensions, it is true that,
2[θT(t)G1+˙θT(t)G2][−˙θ(t)−ˉAθ(t)+ˉBˉf(θ(t))+ˉCˉf(θ(t−τ(t)))+ˉD˙θ(t−h(t))+ˉω(t)]=0=−2θT(t)G1˙θ(t)−2θT(t)G1ˉAθ(t)+2θT(t)G1ˉBˉf(θ(t))+2θT(t)G1ˉCˉf(θ(t−τ(t)))+2θT(t)G1ˉD˙θ(t−h(t))+2θT(t)G1ˉω(t)−2˙θT(t)G2˙θ(t)−2˙θT(t)G2ˉAθ(t)+2˙θT(t)G2ˉBˉf(θ(t))+2˙θT(t)G2ˉCf(θ(t−τ(t)))+2˙θT(t)G2ˉD˙θ(t−h(t))+2˙θT(t)G2ˉω(t). | (3.10) |
We can say that
˙V(θ(t))−δV1(θ(t))−δˉωT(t)ˉω(t)≤ξT(t)Ωξ(t)<0, | (3.11) |
where
ξ(t)=[θT(t)θT(t−τ(t))θT(t−ˉτ)θT(t−ˉh)˙θT(t)˙θT(t−h(t))ˉfT(θ(t))ˉfT(θ(t−τ(t)))ˉfT(θ(t−ˉτ))∫tt−ˉτθT(s)ds∫tt−ˉhθT(s)ds∫0−ˉτ∫tt+λθT(s)dsdλ∫0−ˉτ∫0λ∫tt+αθT(s)dsdαdλˉω(t)]T, |
and Ω is given in (3.2).
˙V(θ(t))≤δV1(θ(t))+δˉωT(t)ˉω(t)<δV(θ(t))+δˉωT(t)ˉω(t) | (3.12) |
Multiplying by e−δt, we can obtain
e−δt˙V(θ(t))−δe−δtV(θ(t))<δe−δtˉωT(t)ˉω(t),ddt(e−δtV(θ(t)))<δe−δtˉωT(t)ˉω(t). | (3.13) |
By integrating (3.13] between 0 to t, such as t∈[0,T1], we can write
e−δtV(θ(t))−V(x(0))<δ∫t0e−δsˉωT(s)ˉω(s)ds,V(θ(t))<eδt[V(θ(0))+∫t0e−δsˉωT(s)ω(s)ds]. | (3.14) |
So,
V(θ(0))=θT(0)Pθ(0)+2∫θ(0)0Q1(ˉf(s)−ˉL−s)+Q2(ˉL+s−ˉf(s))ds+∫0−τ(t)θT(s)R01θ(s)ds+∫0−ˉτθT(s)R1θ(s)ds+∫0−ˉτˉfT(θ(s))R2ˉf(θ(s))ds+∫0−τ(t)ˉfT(θ(s))R02ˉf(θ(s))ds+∫0−h(t)˙θT(s)R3˙θ(s)ds+ˉτ∫0−ˉτ∫tβ˙θT(s)R4˙θ(s)dsdβ+ˉτ∫0−ˉτ∫0βθT(s)R5θ(s)dsdβ+ˉh∫0−ˉh∫0β˙θT(s)R6˙θ(s)dsdβ+ˉτ22∫0−ˉτ∫0γ∫0β˙θT(s)T01˙θ(s)dsdβdγ+ˉτ36∫0−ˉτ∫0λ∫0γ∫0β˙θT(s)T2˙θ(s)dsdβdγdλ+ˉτ424∫0−ˉτ∫0λ∫0α∫0γ∫0β˙θT(s)T3˙θ(s)dsdβdγdαdλ. |
Letting
\begin{eqnarray*} &&\hat{P} = \bar{L}^{-\frac{1}{2}}P\bar{L}^{-\frac{1}{2}},\; \hat{Q_{1}} = \bar{L}^{-\frac{1}{2}}Q_{1}\bar{L}^{-\frac{1}{2}},\;\hat{Q_{2}} = \bar{L}^{-\frac{1}{2}}Q_{2}\bar{L}^{-\frac{1}{2}},\;\hat{R_{01}} = \bar{L}^{-\frac{1}{2}}R_{01}\bar{L}^{-\frac{1}{2}},\\ &&\hat{R_{1}} = \bar{L}^{-\frac{1}{2}}R_{1}\bar{L}^{-\frac{1}{2}},\;\hat{R_{02}} = \bar{L}^{-\frac{1}{2}}R_{02}\bar{L}^{-\frac{1}{2}},\;\hat{R_{2}} = \bar{L}^{-\frac{1}{2}}R_{2}\bar{L}^{-\frac{1}{2}},\;\hat{R_{3}} = \bar{L}^{-\frac{1}{2}}R_{3}\bar{L}^{-\frac{1}{2}},\\ &&\hat{R_{4}} = \bar{L}^{-\frac{1}{2}}R_{4}\bar{L}^{-\frac{1}{2}},\;\hat{R_{5}} = \bar{L}^{-\frac{1}{2}}R_{5}\bar{L}^{-\frac{1}{2}},\;\hat{R_{6}} = \bar{L}^{-\frac{1}{2}}R_{6}\bar{L}^{-\frac{1}{2}},\;\hat{T}_{01} = \bar{L}^{-\frac{1}{2}}T_{01}\bar{L}^{-\frac{1}{2}},\\ &&\hat{T}_{2} = \bar{L}^{-\frac{1}{2}}T_{2}\bar{L}^{-\frac{1}{2}}, \hat{T}_{3} = \bar{L}^{-\frac{1}{2}}T_{3}\bar{L}^{-\frac{1}{2}},\;\lambda_{1} = \lambda_{\min}(\hat{P}),\; \lambda_{2} = \lambda_{\max}(\hat{P}),\\ &&\lambda_{3} = \lambda_{\max}(\hat{Q}_{1}),\;\lambda_{4} = \lambda_{\max}(\hat{Q}_{2}),\;\lambda_{5} = \lambda_{\max}(\hat{R}_{01}),\;\lambda_{6} = \lambda_{\max}(\hat{R}_{1}),\\ &&\lambda_{7} = \lambda_{\max}(\hat{R}_{02}),\;\lambda_{8} = \lambda_{\max}(\hat{R}_{2}),\;\lambda_{9} = \lambda_{\max}(\hat{R}_{3}),\;\lambda_{10} = \lambda_{\max}(\hat{R}_{4}),\\ &&\lambda_{11} = \lambda_{\max}(\hat{R}_{5}),\;\lambda_{12} = \lambda_{\max}(\hat{R}_{6}),\;\lambda_{13} = \lambda_{\max}(\hat{T}_{01}),\;\lambda_{14} = \lambda_{\max}(\hat{T}_{2}),\\ &&\lambda_{15} = \lambda_{\max}(\hat{T}_{3}), \end{eqnarray*} |
we obtain
\begin{eqnarray*} V(\theta(0))& = &\theta^{T}(0)\bar{L}^{\frac{1}{2}}\hat{P}\bar{L}^{\frac{1}{2}}\theta(0)+2 \int_{0}^{\theta(0)}\bar{L}^{\frac{1}{2}}\hat{Q}_{1}\bar{L}^{\frac{1}{2}}(\bar{f}(s)-\bar{L}^{-}s)\\ &+&\bar{L}^{\frac{1}{2}}\hat{Q}_{2}\bar{L}^{\frac{1}{2}}(\bar{L}^{+}s-\bar{f}(s))ds+\int_{-\tau(t)}^{0}\theta^{T}(s)\bar{L}^{\frac{1}{2}}\hat{R}_{01}\bar{L}^{\frac{1}{2}}\theta(s)ds\\ &+&\int_{-\bar{\tau}}^{0}\theta^{T}(s)\bar{L}^{\frac{1}{2}}\hat{R}_{1}\bar{L}^{\frac{1}{2}}\theta(s)ds+ \int_{-\bar{\tau}}^{0}((\bar{L}^{+})^{2}\theta^{T}(s)\bar{L}^{\frac{1}{2}}\hat{R}_{2}\bar{L}^{\frac{1}{2}}\theta(s)ds\\ &+& \int_{-\tau(t)}^{0}((\bar{L}^{+})^{2}\theta^{T}(s)\bar{L}^{\frac{1}{2}}\hat{R}_{02}\bar{L}^{\frac{1}{2}}\theta(s)ds+ \int_{-h(t)}^{0}\dot{\theta}^{T}(s)\bar{L}^{\frac{1}{2}}\hat{R}_{3}\bar{L}^{\frac{1}{2}}\dot{\theta}(s)ds\\ &+&\bar{\tau} \int_{-\bar{\tau}}^{0}\int_{\beta}^{0}\dot{\theta}^{T}(s)\bar{L}^{\frac{1}{2}}\hat{R}_{4}\bar{L}^{\frac{1}{2}}\dot{\theta}(s)dsd\beta+\bar{\tau} \int_{-\bar{\tau}}^{0}\int_{\beta}^{0}\theta^{T}(s)\bar{L}^{\frac{1}{2}}\hat{R}_{5}\bar{L}^{\frac{1}{2}}\theta(s)dsd\beta\\ &+&\bar{h}\int_{-\bar{h}}^{0}\int_{\beta}^{0}\dot{\theta}^{T}(s)\bar{L}^{\frac{1}{2}}\hat{R}_{6}\bar{L}^{\frac{1}{2}}\dot{\theta}(s)dsd\beta+\frac{\bar{\tau}^{2}}{2} \int_{-\bar{\tau}}^{0}\int_{\gamma}^{0}\int_{\beta}^{0}\dot{\theta}^{T}(s)\bar{L}^{\frac{1}{2}}\hat{T}_{1}\bar{L}^{\frac{1}{2}}\dot{\theta}(s)dsd\beta d\gamma\\ &+&\frac{\bar{\tau}^{3}}{6} \int_{-\bar{\tau}}^{0}\int_{\lambda}^{0}\int_{\gamma}^{0}\int_{\beta}^{0}\dot{\theta}^{T}(s)\bar{L}^{\frac{1}{2}}\hat{T}_{2}\bar{L}^{\frac{1}{2}}\dot{\theta}(s)dsd\beta d\gamma d\lambda\\ &+&\frac{\bar{\tau}^{4}}{24} \int_{-\bar{\tau}}^{0}\int_{\lambda}^{0}\int_{\alpha}^{0}\int_{\gamma}^{0}\int_{\beta}^{0}\dot{\theta}^{T}(s)\bar{L}^{\frac{1}{2}}\hat{T}_{3}\bar{L}^{\frac{1}{2}}\dot{\theta}(s)dsd\beta d\gamma d\alpha d\lambda\\ &\leq&\bigg\{\lambda_{\max}(\hat{P})\theta^{T}(0)\bar{L}\theta(0)+2\lambda_{\max}(\hat{Q_{1}})[\max\{|\bar{L}^{+},\;\bar{L}^{-}|^{2}-\bar{L}^{-}\}]\\ &+&2\lambda_{\max}(\hat{Q_{2}})[\max\{\bar{L}^{+}-|\bar{L}^{+},\;\bar{L}^{-}|^{2}\}]+\bar{\tau}[\lambda_{\max}(\hat{R_{01}})+\lambda_{\max}(\hat{R_{1}})]\\ &+&\bar{\tau} [\max\{|\bar{L}^{+},\;\bar{L}^{-}|^{2}\}][\lambda_{\max}(\hat{R_{02}})+\lambda_{\max}(\hat{R_{2}})]+\bar{h}\lambda_{\max}(\hat{R_{3}})+\frac{\bar{\tau}^3}{2}\lambda_{\max}(\hat{R_{4}})\\ &+&\frac{\bar{\tau}^3}{2}\lambda_{\max}(\hat{R_{5}})+\frac{\bar{h}^3}{2}\lambda_{\max}(\hat{R_{6}})+\frac{\bar{\tau}^5}{12}\lambda_{\max}(\hat{T_{1}})+\frac{\bar{\tau}^7}{144}\lambda_{\max}(\hat{T_{2}})+\frac{\bar{\tau}^9}{2880}\lambda_{\max}(\hat{T_{3}})\bigg\}\\ &&\times \sup\limits_{t_{0}\in[-\bar{\tau},0]}\big\{\theta^{T}(t_{0})\bar{L}\theta(t_{0}),\;\dot{\theta}^{T}(t_{0})\bar{L}\dot{\theta}(t_{0})\big\},\\ &&V(\theta(0))\leq \bar{c}_{1}\Gamma, \end{eqnarray*} |
where
\begin{eqnarray*} \Gamma& = &[\lambda_{2}+\lambda_{3}[\tilde{L}^{2}-\bar{L}^{-}]+\lambda_{4}[\bar{L}^{+}-\tilde{L}^{2}]+\bar{\tau}[\lambda_{5}+\lambda_{6}]+\bar{\tau} \tilde{L}^{2}[\lambda_{7}+\lambda_{8}]+\bar{h}\lambda_{9}+\frac{\bar{\tau}^{3}}{2}[\lambda_{10}\\ &+&\lambda_{11}]+\frac{\bar{h}^{3}}{2}\lambda_{12}+\frac{\bar{\tau}^{5}}{12}\lambda_{13}+\frac{\bar{\tau}^7}{144}\lambda_{14}+\frac{\bar{\tau}^9}{2880}\lambda_{15}, \end{eqnarray*} |
where \tilde{L} = \max\{|\bar{L}^{+}, \; \bar{L}^{-}|^{2}.
Furthermore, it follows from (3.14) that
\begin{eqnarray} V(\theta(t))\geq\theta^{T}(t)P\theta(t)\geq\lambda_{min}(\hat{P})\theta^{T}(t)\bar{L}\theta(t) = \lambda_{1}\theta^{T}(t)\bar{L}\theta(t). \end{eqnarray} | (3.15) |
Because of inequalities (3.14) and (3.15), we obtain
\begin{eqnarray} &&\lambda_{1} \theta^{T}(t)P\theta(t)\leq e^{\delta t}[V(\theta(0))+\int_{0}^{t}e^{-\delta t}\bar{\omega}^{T}(s)\bar{\omega}(s)ds],\\ &&\Rightarrow \theta^{T}(t)\bar{L}\theta(t)\leq \frac{e^{\delta T_{1}}[\bar{c}_{1}\Gamma+b(1-e^{(-\delta T_{1})})]}{\lambda_{1}}. \end{eqnarray} | (3.16) |
From condition (3.3), we arrive at \theta^{T}(t)L\theta(t) < \bar{c}_{2}. From Definition 1, the model (3.1) is FTB with regard to (\bar{c}_{1}, \; \bar{c}_{2}, \; T_{1}, \; \bar{L}, \; \bar{b}).
This allowed the proof to be obtained.
Remark 6: In Theorem 1, sufficient conditions are met to verify that the model (3.1) is FTB, then Theorem 2 will present FTP conditions.
In this second part, we study the FTP analysis for the below model
\begin{eqnarray} \left\{ \begin{array}{ll} \dot{\theta}(t) = -\bar{A}\theta(t)+\bar{B}\bar{f}(\theta(t))+\bar{C}\bar{f}(\theta(t-\tau(t)))+\bar{D}\dot{\theta}(t-h(t))+\bar{\omega}(t), \\ \bar{u}(t) = \bar{K}_{1}\theta(t)+\bar{K}_{2}\bar{f}(\theta(t)). \end{array} \right. \end{eqnarray} | (3.17) |
Theorem 2: Suppose that Assumptions 1–5 hold. Let \bar{\tau}, \; \mu, \; \bar{h}, \; h_{1}, and \delta be scalars, then system (3.17) is FTP with keeping the parameter (\bar{c}_{1}, \bar{c}_{2}, T_{1}, \bar{L}, \bar{b}), if there exists symmetric positive definite matrices P, Q_{1}, Q_{2}, R_{01}, R_{1}, R_{02}, R_{2}, R_{3}, R_{4}, R_{5}, T_{01}, T_{2}, T_{3} and diagonal matrices M_{1} > 0, M_{2} > 0, M_{3} > 0, and scalar \beta > 0 such that the following LMIs (3.18) hold:
\begin{eqnarray} \tilde{\Omega} = \left[ \begin{array}{cccccccccccccc} \eta_{1,1} & \bar{L}_{1}M_{3} & \eta_{1,3} & \eta_{1,4} & \eta_{1,5} & G_{1}D & \eta_{1,7} & \eta_{1,8} & 0 & \eta_{1,10} & \frac{\Pi^{2}}{2h}R_{6} & \frac{\bar{\tau}^{2}}{2}T_{2} & \frac{\bar{\tau}^{3}}{6}T_{3} & G_{1}-\beta I-\bar{K}_{1} \\ \star& \eta_{2,2} & 0 & 0 & 0 & 0 & -M_{3}^{T}\bar{L}_{2}^{T} & \eta_{2,8} & 0 & 0 & 0 & 0 & 0 & 0 \\ \star & \star & \eta_{3,3} & 0 & 0 & 0 & 0 & 0 & 0 & \frac{\Pi^{2}}{2\bar{\tau}}R_{4} & 0 & 0 & 0 & 0 \\ \star & \star & \star & \eta_{4,4} & 0 & 0 & 0 & 0 & 0 & 0 & \frac{\Pi^{2}}{2h}R_{6} & 0 & 0 & 0 \\ \star & \star & \star & \star & \eta_{5,5} & G_{2}\bar{D} & \eta_{5,7} & G_{2}\bar{C} & 0 & 0 & 0 & 0 & 0 & G_{2}\\ \star & \star & \star & \star & \star & \eta_{6,6} & 0 &0 & 0 & 0 & 0 & 0 & 0 & 0\\ \star & \star & \star & \star & \star & \star & \eta_{7,7} & M_{3} & 0 & 0 & 0 & 0 & 0 & -\bar{K}_{2}\\ \star & \star & \star & \star & \star & \star & \star & \eta_{8,8} & 0 & 0 & 0 & 0 & 0 & 0\\ \star & \star & \star & \star & \star & \star & \star & \star & \eta_{9,9} & 0 & 0 & 0 & 0 & 0\\ \star & \star & \star & \star & \star & \star & \star & \star & \star & \eta_{10,10} & 0 & 0 & 0 & 0 \\ \star & \star & \star & \star & \star & \star & \star & \star & \star & \star & \eta_{11,11} & 0 & 0 & 0 \\ \star & \star & \star & \star & \star & \star & \star & \star & \star & \star & \star & -T_{2} & 0 & 0\\ \star & \star & \star & \star & \star & \star & \star & \star & \star & \star & \star & \star & -T_{3} & 0 \\ \star & \star & \star & \star & \star & \star & \star & \star & \star & \star & \star & \star & \star & -\beta I \\ \end{array} \right] < 0,\qquad \end{eqnarray} | (3.18) |
\begin{eqnarray*} \bar{c}_{1}\Gamma+\bar{b}(1-e^{-\delta T_{1}}) < \bar{c}_{2}\lambda_{1}e^{-\delta T_{1}}, \end{eqnarray*} |
where the parameters are kept the same as Theorem 3.1.
Proof: Select the Lyapunov function used in the Theorem 3.1. We get
\begin{eqnarray*} &&\dot{V}(\theta(t))-\delta V_{1}(\theta(t))-2\bar{u}^{T}(t)\bar{\omega}(t)-\beta\bar{\omega}^{T}(t)\bar{\omega}(t)\leq\xi^{T}(t)\tilde{\Omega}\xi(t) < 0,\\ &&\Rightarrow \dot{V}(\theta(t))-\delta V(\theta(t))-2\bar{u}^{T}(t)\bar{\omega}(t)-\beta\bar{\omega}^{T}(t)\bar{\omega}(t) < 0, \end{eqnarray*} |
where \tilde{\Omega} is given in (3.18), then
\begin{eqnarray} \dot{V}(\theta(t))-\delta V(\theta(t))&\leq&2\bar{u}^{T}(t)\bar{\omega}(t)+\beta\bar{\omega}^{T}(t)\bar{\omega}(t). \end{eqnarray} | (3.19) |
Multiplying (3.19) by e^{-\delta t}, we can get
\begin{eqnarray*} e^{-\delta t}\dot{V}(\theta(t))-\delta e^{-\delta t} V(\theta(t))\leq2e^{-\delta t}\bar{u}^{T}(t)\bar{\omega}(t)+\beta e^{-\delta t}\bar{\omega}^{T}(t)\bar{\omega}(t), \end{eqnarray*} |
\begin{eqnarray*} \frac{d}{dt}\big(e^{-\delta t}V(\theta(t))\big)\leq2e^{-\delta t}\bar{u}^{T}(t)\bar{\omega}(t)+\beta e^{-\delta t}\bar{\omega}^{T}(t)\bar{\omega}(t). \end{eqnarray*} |
Integrating the above inequality between 0 to T_{1}, we can write
\begin{eqnarray*} e^{-\delta T_{1}}V(\theta(t))-V(\theta(0))&\leq&2\int_{0}^{T_{1}}e^{-\delta t}\bar{u}^{T}(t)\bar{\omega}(t)dt+\beta \int_{0}^{T_{1}}e^{-\delta t}\bar{\omega}^{T}(t)\bar{\omega}(t)dt. \end{eqnarray*} |
Consider the zero initial condition for \theta_{0} = 0 and we have V(\theta(0)) = 0, then
\begin{eqnarray*} e^{-\delta T_{1}}V(\theta(t))&\leq&2\int_{0}^{T_{1}}e^{-\delta t}\bar{u}^{T}(t)\bar{\omega}(t)dt+\beta\int_{0}^{T_{1}}e^{-\delta t}\bar{\omega}^{T}(t)\bar{\omega}(t)dt, \end{eqnarray*} |
\begin{eqnarray} V(\theta(t))&\leq&e^{\delta T_{1}}\int_{0}^{T_{1}}[2\bar{u}^{T}(t)\bar{\omega}(t)+\beta\bar{\omega}^{T}(t)\bar{\omega}(t)]dt. \end{eqnarray} | (3.20) |
Since V(\theta(t))\geq 0, we can say from (3.20) that
\begin{eqnarray} 2\int_{0}^{T_{1}}\bar{u}^{T}(t)\bar{\omega}(t)dt\geq-\beta \int_{0}^{T_{1}}\bar{\omega}^{T}(t)\bar{\omega}(t)dt. \end{eqnarray} | (3.21) |
Finally, we can say that the system (3.17) is FTP. This allowed the proof to be obtained.
Remark 7: It should be emphasized that the LMI approach proposed in this paper is more useful for reducing the conservatism of the delay system, which may lead to obtain less conservative results. This proves the advantage of our proposed method.
Remark 8: We note that the optimal value of \bar{c}_{2} depends on the parameter \delta, then the optimal minimum value of \bar{c}_{2} is determined from the minimum value of \delta, such that the LMIs matrix solution remains feasible.
Remark 9: In the majority of published work, the Lyapunov function theory is the most effective approach to investigating the problem of stability and passivity for divers dynamical models. Moreover, it can be seen that the existing literature [11,12,13,15,19,32,33,34,35] contains the Lyapunov function with single, double, tripe, and four integral terms. However, in this article, we give the Lyapunov–Krasovskii function with five integral terms such as \frac{\bar{\tau}^{4}}{24} \int_{-\bar{\tau}}^{0}\int_{\lambda}^{0}\int_{\alpha}^{0}\int_{\gamma}^{0}\int_{t+\beta}^{t}\dot{\theta}^{T}(s)T_{3}\dot{\theta}(s)dsd\beta d\gamma d\alpha d\lambda. Different from the published work, this is the first time studying the problem of FTP of neutral-type CVNNs. Via a Lyapunov–Krasovskii function with triple, four and five integral terms, by utilizing Jensons inequality and the Wirtinger-type inequality technique, new sufficient conditions for FTB and FTP are taken in terms of LMIs, which is effective on reducing conservatism.
In this section, two examples are given to show the feasibility of our results.
Example 1: Consider the following model
\begin{eqnarray} \left\{ \begin{array}{ll} \dot{z}(t) = -Az(t)+Bf(z(t))+Cf(z(t-\tau(t)))+D\dot{z}(t-h(t))+\omega(t),\\ z(s) = \psi(s),\;\;s\in[-\rho,\;0], \end{array} \right. \end{eqnarray} | (4.1) |
where
\begin{eqnarray*} A& = &\left( \begin{array}{cc} 1.9 & 0 \\ 0 & 1.2 \\ \end{array} \right),\;B = \left( \begin{array}{cc} 0.5+0.5i & 0.03+0.03i \\ -0.4-0.4i & -0.1-0.1i \\ \end{array} \right),\;C = \left( \begin{array}{cc} 1.1+1.1i & 0.03+0.03i \\ 0.07+0.07i & 0.1+0.1i \\ \end{array} \right),\\ D& = &\left( \begin{array}{cc} 0.1+0.1i & 0 \\ 0 & 0.1+0.1i \\ \end{array} \right),\;\bar{A} = \left( \begin{array}{cccc} 1.9 & 0 & 0 & 0 \\ 0 & 1.2 & 0 & 0 \\ 0 & 0 & 1.9 & 0 \\ 0 & 0 & 0 & 1.2 \\ \end{array} \right),\;\bar{B} = \left( \begin{array}{cccc} 0.5 & 0.03 & -0.5 & -0.03 \\ -0.4 & -0.1 & 0.4 & 0.1 \\ 0.5 & 0.03 & 0.5 & 0.03 \\ -0.4 & -0.1 & -0.4 & -0.1 \\ \end{array} \right),\\ \bar{C}& = &\left( \begin{array}{cccc} 1.1 & 0.03 & -1.1 & -0.03 \\ 0.07 & 0.1 & -0.07 & -0.1 \\ 1.1 & 0.03 & 1.1 & 0.03 \\ 0.07 & 0.1 & 0.07 & 0.1 \\ \end{array} \right),\;\bar{D} = \left( \begin{array}{cccc} 0.1 & 0 & -0.1 & 0 \\ 0 & 0.1 & 0 & -0.1 \\ 0.1 & 0 & 0.1 &0 \\ 0 & 0.1 & 0 & 0.1 \\ \end{array} \right), \end{eqnarray*} |
f_{j}(z) = 0.3(|x_{j}+1|-|x_{j}-1|)+i0.3(|y_{j}+1|-|y_{j}-1|). Thus, L_{1} = L_{2} = 0_{2\times 2} and L_{3} = L_{4} = \left(\begin{array}{cc} 0.3 & 0 \\ 0 & 0.3 \\ \end{array} \right), \Rightarrow \bar{L}_{1} = 0_{4\times 4} and \bar{L}_{2} = 0.3 I_{4} \tau(t) = 0.15(1-\sin(2t)), \; h(t) = 0.15(1-\cos(2t)), \; \omega(t) = 0.9\sin(\pi t)e^{-0.5t}+\sin(\pi t)e^{-0.3t}i , \delta = 1, \; \bar{c}_{1} = \left(\begin{array}{c} 0.5 \\ 0.5 \\ \end{array} \right) 0.5, \; \bar{b} = \left(\begin{array}{c} 0.81 \\ 0.60 \\ \end{array} \right), \; T_{1} = 15, matrix \bar{L} = I. Using the Matlab LMI toolbox to solve the LMIs (3.2) and (3.3) in Theorem 1, we obtained the feasible solutions for an optimal minimum value of \bar{c}_{2} = \left(\begin{array}{c} 9.8208 \\ 9.4072 \\ \end{array} \right). The trajectories of the solution of system (4.1) in Example 1 are shown in Figures 1 and 2, and the time history of (Re(z))^{T}L(Re(z)) and (Im(z))^{T}L(Im(z)) are given in Figure 3. Hence, it can be concluded that the system (4.1) is FTB.
Example 2: Consider the following system :
\begin{eqnarray} \left\{ \begin{array}{lll} \dot{z}(t) = -Az(t)+Bf(z(t))+Cf(z(t-\tau(t)))+D\dot{z}(t-h(t))+\omega(t),\\ u(t) = K_{1}z(t)+K_{2}f(z(t)),\\ z(s) = \psi(s),\;\;s\in[-\rho,\;0], \end{array} \right. \end{eqnarray} | (4.2) |
where
\begin{eqnarray*} A& = &\left( \begin{array}{cc} 3.8 & 0 \\ 0 & 2.4 \\ \end{array} \right),\;B = \left( \begin{array}{cc} 1+i & 0.06+0.06i \\ -0.8-0.8i & -0.2-0.2i \\ \end{array} \right),\;C = \left( \begin{array}{cc} 2.2+2.2i & 0.06+0.06i \\ 0.14+0.14i & 0.2+0.2i \\ \end{array} \right),\\D& = &\left( \begin{array}{cc} 0.2+0.2i & 0 \\ 0 & 0.2+0.2i \\ \end{array} \right),\;K_{1} = \left( \begin{array}{cc} 1.2 & 1.6 \\ 1.25 & 1 \\ \end{array} \right),\;K_{2} = \left( \begin{array}{cc} 1+i & 0.5+0.6i \\ 0.5-0.4i & 1-i \\ \end{array} \right),\\ \bar{A}& = &\left( \begin{array}{cccc} 3.8 & 0 & 0 & 0 \\ 0 & 2.4 & 0 & 0 \\ 0 & 0 & 3.8 & 0 \\ 0 & 0 & 0 & 2.4 \\ \end{array} \right),\;\bar{B} = \left( \begin{array}{cccc} 1 & 0.06 & -1 & -0.06 \\ -0.8 & -0.2 & 0.8 & 0.2 \\ 1 & 0.06 & 1 & 0.06 \\ -0.8 & -0.2 & -0.8 & -0.2 \\ \end{array} \right),\; \bar{C} = \left( \begin{array}{cccc} 2.2 & 0.06 & -2.2 & -0.06 \\ 0.14 & 0.2 & -0.14 & -0.2 \\ 2.2 & 0.06 & 2.2 & 0.06 \\ -0.14 & 0.2 & 0.14 & 0.2 \\ \end{array} \right),\\\bar{D}& = &\left( \begin{array}{cccc} 0.2 & 0 & -0.2 & 0 \\ 0 & 0.2 & 0 & -0.2 \\ 0.2 & 0 & 0.2 &0 \\ 0 & 0.2 & 0 & 0.2 \\ \end{array} \right),\;\bar{K}_{1} = \left( \begin{array}{cccc} 1.2 & -1.6 & 0 & 0 \\ -1.25 & 1 & 0 & 0 \\ 0 & 0 & 1.2 & -1.6 \\ 0 & 0 & -1.25 & 1 \\ \end{array} \right),\; \bar{K}_{2} = \left( \begin{array}{cccc} 1 & 0.5 & 1 & -0.6 \\ 0.5 & 1 & 0.4 & 1 \\ -1 & 0.6 & 1 & 0.5 \\ -0.4 & -1 & 0.5 & 1 \\ \end{array} \right), \end{eqnarray*} |
f_{j}(z) = 0.6\tanh(x_{j})+i0.7\tanh(y_{j}). Thus, L_{1} = L_{2} = 0_{2\times 2} and L_{3} = \left(\begin{array}{cc} 0.3 & 0 \\ 0 & 0.3 \\ \end{array} \right), \; L_{4} = \left(\begin{array}{c} 0.35 \\ 0.35 \\ \end{array} \right) \Rightarrow \bar{L}_{1} = 0_{4\times 4} and \bar{L}_{2} = \left(\begin{array}{cccc} 0.3 & 0 & 0 & 0 \\ 0 & 0.3 & 0 & 0 \\ 0 & 0 & 0.35 & 0 \\ 0 & 0 & 0 & 0.35 \\ \end{array} \right), \tau(t) = 0.15(1-\cos(2t)), \; h(t) = 0.15(1-\sin(2t)), \; \omega(t) = 0.2e^{-0.5t}+0.3e^{-0.3t}i, \delta = 1, \; \bar{c}_{1} = \left(\begin{array}{c} 0.1 \\ 0.1 \\ \end{array} \right) 0.5, \bar{b} = \left(\begin{array}{c} 0.019 \\ 0.053 \\ \end{array} \right), \; T_{1} = 12, matrix \bar{L} = I. Using the Matlab LMI toolbox to solve the LMIs (3.2) and (3.3) in Theorem 2, we obtained the feasible solutions for an optimal minimum value of \bar{c}_{2} = \left(\begin{array}{c} 12.4431 \\ 15.0562 \\ \end{array} \right). From Table 1, The results presented in this manuscript are significantly better than those of [32,33,34,35], which confirms the validity of our work.
\mu | 0.8 | 0.9 |
[32] | 3.9212 | 2.3901 |
[33] | 5.2403 | 3.5211 |
[34] | 5.6384 | 3.7718 |
[35] | 6.5411 | 4.5074 |
\bar{\tau} of our result | 6.9307 | 5.2113 |
The trajectories of the solution of model (4.2) in Example 4 are given in Figure 4 and Figure 5. The time history of (Re(z))^{T}L(Re(z)) and (Im(z))^{T}L(Im(z)) are given in Figure 6. Hence, it can be concluded that the system (4.2) is FTP.
This paper is focused on the FTP problem of neutral-type complex-valued NNs in the presence of time-varying delays. By using Lyapunov functionals, the Wirtinger inequality, and LMIs, new sufficient conditions are gotten to guarantee the finite-time boundedness and finite-time passivity of our model. Finally, two examples are presented to prove the effectiveness of our main results. In the future work, we will take the challenge to study the finite-time dissipativity of stochastic complex NNs with mixed delays via a non-separation approach and the fixed-time passivity of coupled clifford-valued NNs subject to multiple delayed couplings.
The authors declare they have not used Artificial Intelligence (AI) tools in the creation of this article.
The authors declare there is no conflict of interest.
[1] | Accorsi R, Cascini A, Ferrari E, et al. (2013) Life cycle assessment of an extra-virgin olive oil supply chain, In Bevilacqua, M. (ed.) Proceeding of the XVⅡ Summer School Francesco Turco: A challenge for the future - The role of industrial engineering in a global sustainable economy, Senigallia (Ancona) Italy, September 11–13, 2013,172–178. https://hdl.handle.net/11585/197332 |
[2] | Adamo P, Baldoni L, Benalia S, et al. (2020) Intensificazione sostenibile. Proceedings of the 17th Conference A.I.S.S.A., February 2020, 1: 17–18. |
[3] | Alfei B, Pannelli G, Ricci A (2013) Olivicoltura. Coltivazione, olio e territorio, Bologna, Edagricole. |
[4] | Aparicio R, Harwood J (2013) Handbook of Olive Oil: Analysis and Properties, New York: Springer-Verlag NY Inc. https://doi.org/10.1007/978-1-4614-7777-8 |
[5] | Attorre A, Ricci N, Soracco D (2021) Il mondo dell'olio. Storia, produzione, uso in cucina dell'extravergine, Bra: Slow Food. |
[6] | Benedetti M, Corti F, Guagnini C (Yearless) Agroalimentare: Italia, una (pen)isola felice, Available from: https://www.sace.it/docs/default-source/default-document-library/sace-focus-on-agroalimentare.pdf?sfvrsn = 87a146b9_0 (accessed on 4 July 2024) |
[7] |
Bhattacharya S, Momaya KS, Iyer KC (2020) Benchmarking enablers to achieve growth performance: a conceptual framework. Benchmarking 27: 1475–1501. https://doi.org/10.1108/BIJ-08-2019-0376 doi: 10.1108/BIJ-08-2019-0376
![]() |
[8] |
Boesen S, Bey N, Niero M (2019) Environmental sustainability of liquid food packaging: Is there a gap between Danish consumers' perception and learnings from life cycle assessment? J Clean Prod 210: 1193–1206. https://doi.org/10.1016/j.jclepro.2018.11.055 doi: 10.1016/j.jclepro.2018.11.055
![]() |
[9] | Boria P (2023) L'evasione fiscale. Ricerca su natura giuridica e dimensione quantitativa, Roma: Università La Sapienza. |
[10] | Borsellino V, Zinnanti C, Migliore G, et al. (2018) An exploratory analysis of website quality in the agrifood sector: The case of extra virgin olive oil. Quality - Access to Success 19: 132–138. |
[11] | Boskou D (2006) Olive Oil. Chemistry and Technology, New York: A.O.C.S. https://doi.org/10.4324/9781003040217 |
[12] | Boskou D (2015) Olive and Olive Oil Bioactive Constituents, New York: A.O.C.S. https://doi.org/10.1016/B978-1-63067-041-2.50007-0 |
[13] | Caricato L (2017) OOF International Magazine. The shapes of oil. Packaging, the silent revolution, Milano: Olio Officina. |
[14] |
Castilla-Polo F, Gallardo-Vázquez D, Sánchez-Hernández MI, et al. (2018) An empirical approach to analyze the reputation-performance linkage in agrifood cooperatives. J Clean Prod 195: 163–175. https://doi.org/10.1016/j.jclepro.2018.05.210 doi: 10.1016/j.jclepro.2018.05.210
![]() |
[15] | Conte L, Servili M (2022) Oleum. Qualità, tecnologia e sostenibilità degli oli da olive, Bologna: Edagricole. |
[16] | Contò F (2005) Economia e organizzazione delle filiere agroalimentari. La filiera dell'olio di oliva di qualità, Milano: Franco Angeli. |
[17] |
De Blasio V, Pavone P, Migliaccio G (2022) Cosmetics companies: income developments in time of crisis. J Small Bus Enterp D 29: 1017–1048. https://doi.org/10.1108/JSBED-11-2019-0369 doi: 10.1108/JSBED-11-2019-0369
![]() |
[18] |
De Gennaro B, Notarnicola B, Roselli L, et al. (2012) Innovative olive-growing models: An environmental and economic assessment. J Clean Prod 28: 70–80. https://doi.org/10.1016/j.jclepro.2011.11.004 doi: 10.1016/j.jclepro.2011.11.004
![]() |
[19] | Di Gioia F (2022) Olivicoltura e produzione dell'olio di oliva, Roma: Andromeda. |
[20] | Di Schino J, Liguori G, Stefani M (2016) L' oro del Mediterraneo. Olio d'oliva, Firenze: goWare. |
[21] | Felice E (2007) Divari regionali e intervento pubblico. Per una rilettura dello sviluppo in Italia, Bologna: Il Mulino. |
[22] | Fink A (1995) How to AnalyzeSurvey Data, Los Angeles: U.C.L.A. |
[23] |
Fusco F, Migliaccio G (2018) Crisis, Sectoral and Geographical Factors: Financial Dynamics of Italian Cooperatives. Euromed J Bus 13: 130–148. https://doi.org/10.1108/EMJB-02-2016-0002 doi: 10.1108/EMJB-02-2016-0002
![]() |
[24] |
Fusco F, Migliaccio G (2019) Cooperatives and Crisis: Economic Dynamics in Italian Context. Int J Bus Glob 22: 638–654. https://doi.org/10.1504/IJBG.2019.100258 doi: 10.1504/IJBG.2019.100258
![]() |
[25] | Garibaldi R (2024) Turismo dell'olio. Available from: https://www.robertagaribaldi.it. |
[26] |
Gómez-Carmona D, Paramio A, Cruces-Montes S, et al. (2023) The effect of the wine tourism experience. J Destin Mark Manage 29: 100793. https://doi.org/10.1016/j.jdmm.2023.100793 doi: 10.1016/j.jdmm.2023.100793
![]() |
[27] | Gorgitano MT, Sodano V (2019) Differentiation policies in the Italian market of extra virgin olive oil. Quality - Access to Success 20: 274–279. |
[28] | Gu C (2013) Smoothing Spline ANOVA Models. 2nd ed., New York: Springer. https://doi.org/10.1007/978-1-4614-5369-7 |
[29] | Gurrieri AR, Spallini S (2016) Networking Entrepreneurship in Non-Technology Sectors. The Case of Olive Oil, Developments in Marketing Science: Proceedings of the Academy of Marketing Science, 343–346. https://doi.org/10.1007/978-3-319-29877-1_69 |
[30] | Hernández-Mogollón JM, Di-Clemente E, Campón-Cerro AM, et al. (2021) Olive oil tourism in the euro-mediterranean area. Int J Euro-Mediterr Stud 14: 85–101. |
[31] |
Iovino F, Migliaccio G (2019) Financial Dynamics of Energy Companies During Global Economic Crisis. Int J Bus Glob 22: 541–554. https://doi.org/10.1504/IJBG.2019.100256 doi: 10.1504/IJBG.2019.100256
![]() |
[32] | Ismea (2021) Olio di oliva - Ultime dal settore. Olio di oliva: le prime stime produttive in Italia. Available from: https://www.ismeamercati.it/flex/cm/pages/ServeBLOB.php/L/IT/IDPagina/11750 (accessed on 4 July 2024). |
[33] | Kharlamova O, Tkachenko S, Poliakova Y, et al. (2020) Management accounting using benchmarking tools. Acade Account Financ Stud J 24: 1–7. |
[34] |
Kramer CY (1956) Extension of multiple range tests to group means with unequal numbers of replications. Biometrics 12: 307–310. https://doi.org/10.2307/3001469 doi: 10.2307/3001469
![]() |
[35] | Lanzara O (2017) Quando la qualità si fa concorrenza: il made in Italy e la legge 'Salva olio'. Il diritto dell'agricoltura, 127–156. |
[36] | Liao Q, Li J (2018) An Adaptive Reduced Basis ANOVA method for High-dimensional Bayesian Inverse Problems. J Comput Physics, arXiv: 1811.05151[math.NA]. https://doi.org/10.1016/j.jcp.2019.06.059 |
[37] | Lupi R (2020) Manuale di evasione fiscale. Conoscerla per contrastarla. Roma: Castelvecchi. |
[38] | Mella P, Navaroni M (2016) Analisi di bilancio, Sant'Arcangelo di Romagna: Maggioli. |
[39] | Menzani T (2007) Prima e dopo Mezzogiorno. Le regioni italiane fra arretratezza e sviluppo, Meridiana - Rivista di storia e scienze sociali (58-Nuove forme di democrazia), 245–249. |
[40] |
Mesic Ž, Molnár A, Cerjak M (2018) Assessment of traditional food supply chain performance using triadic approach: the role of relationships quality. Supply Chain Manage 23: 396–411. https://doi.org/10.1108/SCM-10-2017-0336 doi: 10.1108/SCM-10-2017-0336
![]() |
[41] | Migliaccio G (2018) The profitability of Italian hotels during and after the 2008 Economic Crisis. Afr J Hosp Tour Leisure 7: 1–21. |
[42] |
Migliaccio G, Arena MF (2021a) Il benchmarking per il controllo della performance: esiti di una ricerca nei distretti conciari italiani. Manage Control, 87–110. https://doi.org/10.3280/MACO2021-003005 doi: 10.3280/MACO2021-003005
![]() |
[43] |
Migliaccio G, Arena MF (2021b) Financial performance of Italian tanning manufacturers before, during and after the global crisis. Int J Glob Small Bus 12: 213–246. https://doi.org/10.1504/IJGSB.2021.10040807 doi: 10.1504/IJGSB.2021.10040807
![]() |
[44] |
Migliaccio G, D'Alelio CC (2022) The profitability of Italian jewellery between the two international economic crisis. Int J Glob Small Bus 13: 79–107. https://doi.org/10.1504/IJGSB.2022.10047040 doi: 10.1504/IJGSB.2022.10047040
![]() |
[45] |
Migliaccio G, De Blasio V (2024) The Italian Plastics Industries toward a Plastic-Free Society: what Possible Strategies? World Rev Entrep Manage Sust Dev 20: 179–218. https://doi.org/10.1504/WREMSD.2024.10061683 doi: 10.1504/WREMSD.2024.10061683
![]() |
[46] |
Migliaccio G, De Palma A (2024) Profitability and financial performance of Italian real estate companies: quantitative profiles. Int J Product Perfor Manage 73: 122–160. https://doi.org/10.1108/IJPPM-02-2023-0075 doi: 10.1108/IJPPM-02-2023-0075
![]() |
[47] |
Migliaccio G, Lucadamo A, Napoli G, et al. (2022) Economic and Sporting Performance and Player Registration Rights in Italian Soccer: Connections? Int J Manage Enterp Dev 21: 392–415. https://doi.org/10.1504/IJMED.2022.126577 doi: 10.1504/IJMED.2022.126577
![]() |
[48] |
Migliaccio G, Pavone P (2021a) Innovative Small Start-Ups in Italy: a Successful Business Model? Int J Manage Enterp Dev 20: 405–455. https://doi.org/10.1504/IJMED.2022.10042082 doi: 10.1504/IJMED.2022.10042082
![]() |
[49] | Migliaccio G, Pavone P (2021b) Italian Innovative Start-up Cohorts: an Empirical Survey on Profitability, in Bevilacqua, C., Calabrò, F. and Della Spina, L. (Eds.), New Metropolitan Perspectives - Knowledge Dynamics and Innovation-driven Policies Towards Urban and Regional Transition (Vol. 2), Springer Nature Switzerland AG, Heidelberg & Berlin, 834–843. https://doi.org/10.1007/978-3-030-48279-4 |
[50] |
Migliaccio G, Pavone P (2022) Primary sector in Italy: profitability dynamics and relationship with the international economic crisis. Int J Product Perfor Manage 71: 2893–2912. https://doi/10.1108/IJPPM-05-2020-0229 doi: 10.1108/IJPPM-05-2020-0229
![]() |
[51] |
Migliaccio G, Pavone P (2024) Innovativeness in the economic system: the Italian experience. Int J Bus Innov Res 34: 109–138. https://doi.org/10.1504/IJBIR.2024.138141 doi: 10.1504/IJBIR.2024.138141
![]() |
[52] |
Migliaccio G, Tucci L (2020) Economic assets and financial performance of Italian wine companies. Int J Wine Bus Res 32: 325–352. https://doi.org/10.1108/IJWBR-04-2019-0026 doi: 10.1108/IJWBR-04-2019-0026
![]() |
[53] | Migliaccio G, Tucci L, Simonetti B (2021) The Effects of the Global Crisis on the Economic-Financial Performance of Italian Bed and Breakfasts: a Ten-year Quantitative Analysis from the Financial reports. e-Rev Tour Res 18: 611–646. |
[54] | Moro E (2014) La dieta mediterranea. Mito e storia di uno stile di vita, Bologna: Il Mulino. |
[55] | Pato ML (2024) A Decade of Olive Oil Tourism: A Bibliometric Survey. Sustainability (Switzerland), 16: 1665. https://doi.org/10.3390/su16041665 |
[56] |
Pavone P, Migliaccio G (2021) Profitability of the Italian farming companies and the impact of financial crisis: quantitative research using accounting data. Int J Bus Perfor Manage 22: 394–425. https://doi.org/10.1504/IJBPM.2021.10041320 doi: 10.1504/IJBPM.2021.10041320
![]() |
[57] |
Pavone P, Migliaccio G, Simonetti B (2023) Investigating financial statements in hospitality: a quantitative approach. Qual Quant 57: S383–S407. https://doi.org/10.1007/s11135-020-01086-3 doi: 10.1007/s11135-020-01086-3
![]() |
[58] |
Pellegrini G, La Sala P, Camposeo S et al. (2017) Economic sustainability of the olive oil high and super-high density cropping systems in Italy. Global Bus Econ Rev 19: 553–569. https://doi.org/10.1504/GBER.2017.086604 doi: 10.1504/GBER.2017.086604
![]() |
[59] | Preedy VR, Watson RR (2020) Olives and Olive Oil in Health and Disease Prevention, Amsterdam: Elsevier. |
[60] |
Pulido-Fernández JI, Casado-Montilla J, Carrillo-Hidalgo I, et al. (2022) Evaluating olive oil tourism experiences based on the segmentation of demand. Int J Gastron Food Sci 27: 100461. https://doi.org/10.1016/j.ijgfs.2021.100461 doi: 10.1016/j.ijgfs.2021.100461
![]() |
[61] | Ross A, Willson VL (2017) One-way ANOVA. In Ross, A., Willson V.L. (Eds.), Basic and Advanced Statistical Tests. Sense: Rotterdam, 21–24. https://doi.org/10.1007/978-94-6351-086-8_5 |
[62] | Rossi R (2017) Il settore delle olive e dell'olio d'oliva nell'UE. Principali caratteristiche, sfide e prospettive. Available from: https://www.europarl.europa.eu/RegData/etudes/BRIE/2017/608690/EPRS_BRI%282017%29608690_IT.pdf (accessed on 4 July 2024). |
[63] |
Sanchez-Famoso V, Cano-Rubio M, Fuentes-Lombardo G (2019) The role of cooperation agreements in the internationalization of Spanish winery and olive oil family firms. Int J Wine Bus Res 31: 555–577. https://doi.org/10.1108/IJWBR-08-2018-0042 doi: 10.1108/IJWBR-08-2018-0042
![]() |
[64] | Sarnari T (2022) Tendenze e dinamiche recenti. Olio d'oliva. Available from: https://olivonews.it/wp-content/uploads/2022/09/20221309_Tendenze_olio.pdf. |
[65] | Scarfì P (2024) Evasione fiscale. Un'idea che risolve la questione. Tricase: Youcanprint. |
[66] | Scarpato D, Borrelli IP, Ardeleanu MP (2013) Competitiveness and environmental performance in the olive oil sector: An analysis of the Campania region. Qual - Access Success 14: 165–169. |
[67] |
Scherhag K, Rüdiger J, Dreyer A (2023) Introduction to wine tourism. Zeitschrift für Tourismuswissenschaft 15: 231–238. https://doi.org/10.2307/3001913 doi: 10.2307/3001913
![]() |
[68] | Solari A, Salmaso L, Pesarin F, et al. (2009) Permutation Tests for Stochastic Ordering and ANOVA. Theory and Applications with R., 194. New York: Springer-Verlag. http://dx.doi.org/10.1007/978-0-387-85956-9 |
[69] | Soós G, Dávid L (2015) Wine Marketing - Tools for Innovation, Creativity and Sustainability, Proceedings of International Conference on New Trends in Sustainable Business and Consumption (B.A.S.I.Q. 2015), Bucharest, JUN 18-19, 2015,473–480. |
[70] | Stillitano T, De Luca AI, Iofrida N, et al. (2017) Economic analysis of olive oil production systems in southern Italy. Qual - Access Success 18: 107–112. |
[71] |
Tukey JW (1949) Comparing individual means in the analysis of variance. Biometrics 5: 99–114. https://doi.org/10.2307/3001913 doi: 10.2307/3001913
![]() |
[72] | Tukey JW (1953) The problem of multiple comparisons. unpublished manuscript, In: Karen, The Collected Works of John W Tukey VIII. Multiple Comparisons: 1948–1983. New York: Chapman and Hall. |
[73] | Tukey JW (1993) Where should multiple comparisons go next? In: Hoppe, F.M., Multiple Comparisons, Selection, and Applications in Biometry. New York: Dekkerpp, 187–207. |
[74] |
Vena-Oya J, Parrilla-González JA (2023) Importance–performance analysis of olive oil tourism activities: Differences between national and international tourists. J Vacat Market. https://doi.org/10.1177/13567667221147316 doi: 10.1177/13567667221147316
![]() |
[75] | Viesti G (2021) Centri e periferie. Europa, Italia, Mezzogiorno dal XX al XXI secolo, Bari-Roma: La Terza. |
[76] | Watson GH (2000) Il Benchmarking. Come Migliorare i Processi e la Competitività Aziendale Adattando e Adottando le Pratiche Delle Imprese Leader, Milano: Franco Angeli. |