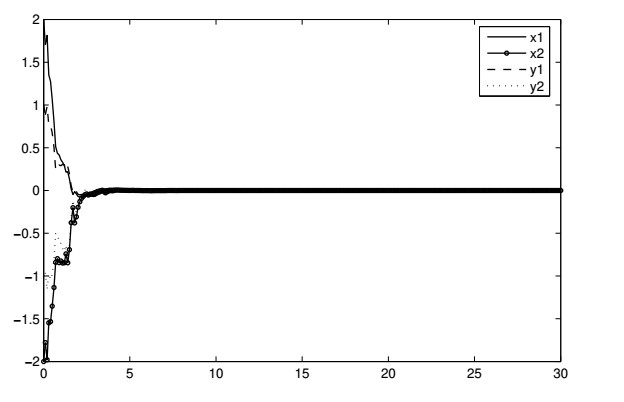
Citation: Basant Pant, Rajesh Kumar Rai, Sushma Bhattarai, Nilhari Neupane, Rajan Kotru, Dipesh Pyakurel. Actors in customary and modern trade of Caterpillar Fungus in Nepalese high mountains: who holds the power?[J]. Green Finance, 2020, 2(4): 373-391. doi: 10.3934/GF.2020020
[1] | Wenjing Wang, Jingjing Dong, Dong Xu, Zhilian Yan, Jianping Zhou . Synchronization control of time-delay neural networks via event-triggered non-fragile cost-guaranteed control. Mathematical Biosciences and Engineering, 2023, 20(1): 52-75. doi: 10.3934/mbe.2023004 |
[2] | Pan Wang, Xuechen Li, Qianqian Zheng . Synchronization of inertial complex-valued memristor-based neural networks with time-varying delays. Mathematical Biosciences and Engineering, 2024, 21(2): 3319-3334. doi: 10.3934/mbe.2024147 |
[3] | Biwen Li, Xuan Cheng . Synchronization analysis of coupled fractional-order neural networks with time-varying delays. Mathematical Biosciences and Engineering, 2023, 20(8): 14846-14865. doi: 10.3934/mbe.2023665 |
[4] | Ruoyu Wei, Jinde Cao . Prespecified-time bipartite synchronization of coupled reaction-diffusion memristive neural networks with competitive interactions. Mathematical Biosciences and Engineering, 2022, 19(12): 12814-12832. doi: 10.3934/mbe.2022598 |
[5] | Bingrui Zhang, Jin-E Zhang . Fixed-deviation stabilization and synchronization for delayed fractional-order complex-valued neural networks. Mathematical Biosciences and Engineering, 2023, 20(6): 10244-10263. doi: 10.3934/mbe.2023449 |
[6] | Trayan Stamov, Gani Stamov, Ivanka Stamova, Ekaterina Gospodinova . Lyapunov approach to manifolds stability for impulsive Cohen–Grossberg-type conformable neural network models. Mathematical Biosciences and Engineering, 2023, 20(8): 15431-15455. doi: 10.3934/mbe.2023689 |
[7] | Hai Lin, Jingcheng Wang . Pinning control of complex networks with time-varying inner and outer coupling. Mathematical Biosciences and Engineering, 2021, 18(4): 3435-3447. doi: 10.3934/mbe.2021172 |
[8] | Zhen Yang, Zhengqiu Zhang, Xiaoli Wang . New finite-time synchronization conditions of delayed multinonidentical coupled complex dynamical networks. Mathematical Biosciences and Engineering, 2023, 20(2): 3047-3069. doi: 10.3934/mbe.2023144 |
[9] | Karim El Laithy, Martin Bogdan . Synaptic energy drives the information processing mechanisms in spiking neural networks. Mathematical Biosciences and Engineering, 2014, 11(2): 233-256. doi: 10.3934/mbe.2014.11.233 |
[10] | Guowei Wang, Yan Fu . Spatiotemporal patterns and collective dynamics of bi-layer coupled Izhikevich neural networks with multi-area channels. Mathematical Biosciences and Engineering, 2023, 20(2): 3944-3969. doi: 10.3934/mbe.2023184 |
Due to the wide variety of applications in fields including associative memories, signal processing, parallel computation, pattern recognition, neural networks have drawn a lot of interest in recent years. In many real-world systems, artificial neural networks, neural networks, biological systems have inevitable applications. [1,2,3,4,5,6,7]. Due to the obvious transmission frequency of interconnected neurons and the finite switching speeds of the amplifiers, time delays are ineluctably given. Their presence might affect a system's stability by causing oscillation and instability features [8,9,10,11,12,13,14,15,16,17,18]. Delayed dynamical networks and many time-delay system kinds have been studied, and numerous important outcomes have been presented [19,20,21,22,23,24,25].
Kosko was the first person to propose that BAM-NNs could be important to the NNs theory [26]. Furthermore, BAM neural frameworks involving delays attracted significant thought and underwent in-depth research. While there is no connection between neurons in the same layer, neurons in another layer are entirely interconnected to those in the first layer. It performs a two-way affiliated hunt to store bipolar vector combines by cycles of forward and backward propagation information streams between the two layers, and it sums up the single-layer auto-cooperative Hebbian connection to a two-layer design coordinated hetero acquainted circuits. As a result, it has numerous applications in the fields of artificial intelligence and pattern identification [27,28,29,30,31,32,33,34,35]. Appropriately, the BAM neural system has been generally concentrated on both in principle and applications. This makes focusing on the stability of the BAM neural framework, which has largely been studied, fascinating and fundamental [36,37,38,39,40,41,42,43,44].
The synchronization control of chaotic networks plays vital role in applications such as image encryption, secure communication, DC-DC motor and etc. [45,46,47,48]. Satellites play a significant part in the advancement of space technology, civic, military, and scientific endeavors. In particular, feedback control, adaptive control, sampled-data control, and other strategies have been employed to synchronize and regulate the satellite systems [49,50]. Recently, the issue of memristive BAM networks synchronization with stochastic feedback gain variations in [50].
It is inferred that in real-world scenarios, a nearby float framework as noted in practical model controller does not avoid the proceed out of coefficient uncertainty, leading to the murkiness in controller execution caused by the required word length in any updated structures or additional parameter turning in the final controller use. Along the same ideas, it is crucial to design a non-fragile controller such that it is insensitive to uncertainty. Non-fragile control has developed into a fascinating topic in both theory and practical application. There has been extensive research into the use of non-fragile controllers in recent years [51,52]. However, the problem of non-fragile control of a BAM delayed neural network and controller gain fluctuation has not been thoroughly examined.
This study examines the non-fragile synchronization for a BAM delayed neural network and a randomly occurring controller gain fluctuation, which is motivated by the studies. New stability requirements for BAM neural networks with arbitrarily occurring controller gain fluctuation are derived in terms of LMI by building an appropriate Lyapunov-Krasovskii functional (LKF) and using conventional integral inequality techniques. Solving the suggested LMI condition yields the gain matrices for the proposed controller design. Finally, a numerical example is used to illustrate the proposed strategy.
Notations: In this work, Rp represents Euclidean space of p dimension. Rp×q denotes the set of all p×q real matrices. The identity matrix is denoted by I. Here R>0(<0) represents R is a symmetric positive (negative) definite matrices. The elements below the main diagonal of a symmetric matrixl is given by '*'.
In this study, we will investigate the synchronization of BAM delayed neural network with randomly occurring. The BAM NNs with time-varying delay components can be modeled as follows
˙θi(ℵ)=−aiθi(ℵ)+∑nj=1w(1)ij˜fj(λj(ℵ))+∑nj=1w(2)ij˜fj(λj(ℵ−σ(ℵ)))+Ii,˙λj(ℵ)=−bjλj(ℵ)+∑ni=1v(1)ji˜gi(θi(ℵ))+∑ni=1v(2)ji˜gi(θi(ℵ−κ(ℵ)))+Jj,} | (2.1) |
where γi(ℵ) and δj(ℵ) are the state variables of the neuron at time ℵ respectively. The non linear functions ˜fj(⋅), ˜gi(⋅) are neuron activation functions. The positive constants ai,bj denote the time scales of the respective layers of the neurons. w(1)ij, w(2)ij, v(1)ji, v(2)ji are connection weights of the network. Ii and Jj denotes the external inputs. σ(ℵ),κ(ℵ) are time varying delays satisfying
0≤σ(ℵ)≤σ, ˙σ(ℵ)=μ1<1, 0≤κ(ℵ)≤κ, ˙κ(ℵ)=μ2<2, | (2.2) |
and, σ, κ, μ1, and μ2 are constants.
Consequently, the corresponding compact matrix form can be used to describe the master system (2.1) as,
M:{˙θ(ℵ)=−Aθ(ℵ)+W1˜f(λ(ℵ))+W2˜f(λ(ℵ−σ(ℵ))),˙λ(ℵ)=−Bλ(ℵ)+V1˜g(θ(ℵ))+V2˜g(θ(ℵ−κ(ℵ))), | (2.3) |
where, B=diag[b1,b2,...,bn]>0, A=diag[a1,a2,...,an]>0, Wk=(w(k)ij)(n×n), Vk=(v(k)ij)(n×n),k=1,2.
Assumption (1). ˜gj(⋅),˜fi(⋅) are neuron activation functions, which is bounded, then there exist constants H−i, H+i, L−j, L+j such that
L−j≤˜gj(ϕ)−˜gj(ϱ)ϕ−ϱ≤L+j,H−i≤˜fi(ϕ)−˜fi(ϱ)ϕ−ϱ≤H+i, | (2.4) |
where, j=1,..,n,i=1,...m, and ϱ,ϕ∈R with ϕ≠ϱ. We define the subsequent matrices for ease of notation:
H1=diag{H+1H−1,H+2H−2,...,H+mH−m},H2=diag{H−1+H+12,H+2+H−22,...,H+m+H−m2},L1=diag{L+1L−1,L+2L−2,...,L+nL−n},L2=diag{L+1+L−12,L+2+L−22,...,L+n+L−n2}. |
Master system and slave system state variables are represented by θ(ℵ),λ(ℵ) and ˆθ(ℵ) and ˆλ(ℵ), respectively. We take into account the master system's slave system in the follows.
S:{˙ˆθ(ℵ)=−Aˆθ(ℵ)+W1˜f(ˆλ(ℵ))+W2˜f(ˆλ(ℵ−σ(ℵ)))+u(ℵ),˙ˆλ(ℵ)=−Bˆλ(ℵ)+V1˜g(ˆθ(ℵ))+V2˜g(ˆθ(ℵ−κ(ℵ)))+v(ℵ) | (2.5) |
We choose the following non-fragile controller:
u(ℵ)=(K1+ϕ(ℵ)ΔK1(ℵ))ζ(ℵ), v(ℵ)=(K2+ϱ(ℵ)ΔK2(ℵ))ϖ(ℵ), | (2.6) |
Here the controller gain matrices are given by K1,K2. The matrix ΔKi(ℵ)(i=1,2) satisfy
ΔKi(ℵ)=HiΔ(ℵ)Ei (i=1,2) | (2.7) |
and
ΔT(ℵ)Δ(ℵ)≤I, | (2.8) |
where Hi,Ei are known constant matrices. To desribe randomly accuring control gain fluctuation we introduced the stachastic variables ϕ(ℵ),ϱ(ℵ)∈R. It is a sequence of white noise generated by Bernoulli distribution with values of zero or one
Pr{ϱ(ℵ)=0}=1−ϱ, Pr{ϱ(ℵ)=1}=ϱ,Pr{ϕ(ℵ)=0}=1−ϕ, Pr{ϕ(ℵ)=1}=ϕ, | (2.9) |
where 0≤ϱ,ϕ≤1 is constant.
Set the synchronization error signals ϖ(ℵ)=ˆλ(ℵ)−λ(ℵ) and ζ(ℵ)=ˆθ(ℵ)−θ(ℵ). Error dynamics between systems (2.3) and (2.5) can therefore be written as follows:
˙ζ(ℵ)=(−A+(K1+ϕ(ℵ)ΔK1(ℵ)))ζ(ℵ)+W1f(ϖ(ℵ))+W2f(ϖ(ℵ−σ(ℵ))),˙ϖ(ℵ)=(−B+(K2+ϱ(ℵ)ΔK2(ℵ)))ϖ(ℵ)+V1g(ζ(ℵ))+V2g(ζ(ℵ−κ(ℵ)))}. | (2.10) |
where g(ζ(ℵ))=˜g(ˆθ(ℵ))−˜g(θ(ℵ)), and f(ϖ(ℵ))=˜f(ˆλ(ℵ))−˜f(λ(ℵ)).
The following crucial conditions are used to obtain our main findings:
Lemma 2.1. [53] (Schur complement) Let Z, V,O be given matrices such that Z>0, then
[OVTv−Z]<0iffO+VTZ−1V<0. |
Lemma 2.2. [54] Given matrices Z=ZT,R,O and Q=QT>0 with appropriate dimensions
Z+RL(ℵ)O+OTLT(ℵ)RT<0, |
for all L(ℵ) satisfying LT(ℵ)L(ℵ)≤I if and only if there exists a scalar ε>0 such that
Z+ε−1RRT+εOTQO<0. |
Lemma 2.3. [55] For a given matrix Z∈S+n and a function ς:[c,d]→Rn whose derivative ˙ς∈PC([c,d],Rn), the following inequalities hold: ∫dc˙ςT(r)Z˙ς(r)dr≥1d−c ˆφ¯Zˆφ, where ¯Z=diag{Z,3Z,5Z}, ˆφ=[φT1 φT2 φT3]T, φ1=ς(d)−ς(c), φ2=ς(d)+ς(c)−2d−c∫dcς(r)dr, φ3=ς(d)−ς(c)+6d−c∫dcς(r)dr−12(d−c)2∫dc∫dcς(u)duds.
The necessary requirements for guaranteeing the stability of system (2.10) are established in this part.
Theorem 3.1. From Assumption (A), ϕ,ϱ, and ϵi are positive scalars, if there exist symmetric matrices Pi>0, Qi>0, Ri>0, Zi>0, any matrices Ji and Gi, diagonal matrices Si>0 (i=1,2) satisfying
Ξ=[ΠΩ∗Λ]<0, | (3.1) |
where
Π=[Π113Z1κS2L20−24Z1κ260Z1κ3Π17∗Π220036Z1κ2−60Z1κ30∗∗Π330000∗∗∗Π44000∗∗∗∗−192Z1κ3360Z1κ40∗∗∗∗∗−720Z1κ50∗∗∗∗∗∗Π77],Ω=[00J1W1J1W20000000000VT1JT200000VT1JT2VT2JT200000VT2JT20000000000000000J1W1J1W2000],Λ=[Λ113Z2σS1H20−24Z2σ260Z2σ3Λ17∗Λ220036Z2σ2−60Z2σ30∗∗Λ330000∗∗∗Λ44000∗∗∗∗−192Z2σ3360Z2σ40∗∗∗∗∗−720Z2σ50∗∗∗∗∗∗Λ77], |
Π11=R1−S2L1−9Z1κ−J1A+G1+ϕJ1ΔK1(ℵ)+GT1−ATJT1+ϕΔKT1(ℵ)JT1,Π17=−J1−ϵ1ATJT1+ϵ1GT1+ϵ1ϕΔKT1(ℵ)JT1+P1,Π22=−R1−9Z1κ,Π33=Q2−S2,Π44=−(1−μ2)Q2,Π77=−ϵ1J1+κZ1−ϵ1JT1,Λ11=R2−S1H1−J2B+G2+ϱJ2ΔK2(ℵ)−9Z2σ−BTJT+GT2+ϱΔKT2(ℵ)JT2,Λ17=−J2−ϵ2BTJT2+ϵ2GT2+ϵ2ϱΔKT2(ℵ)JT2+P2,Λ22=−R2−9Z2σ,Λ33=Q1−S1,Λ44=−(1−μ1)Q1,Λ77=σZ2−ϵ2J2−ϵ2JT2, |
When this happens, the system (2.10) is asymptotically stable and Ki=J−1iGi. are control gain matrices.
Proof. Take into account the LKF candidate below:
V(ζℵ,ϖℵ,ℵ)=4∑i=1Vi(ζℵ,ϖℵ,ℵ), | (3.2) |
where
V1(ζℵ,ϖℵ,ℵ)=ζT(ℵ)P1ζ(ℵ)+ϖT(ℵ)P2ϖ(ℵ),V2(ζℵ,ϖℵ,ℵ)=∫ℵℵ−σ(ℵ)fT(ϖ(s))Q1f(ϖ(s))ds+∫ℵℵ−κ(ℵ)gT(ζ(s))Q2g(ζ(s))ds,V3(ζℵ,ϖℵ,ℵ)=∫ℵℵ−κζT(s)R1ζ(s)ds+∫ℵℵ−σϖT(s)R2ϖ(s)ds,V4(ζℵ,ϖℵ,ℵ)=∫0−κ∫ℵℵ+θ˙ζT(s)Z1˙ζ(s)dsdθ+∫0−σ∫ℵℵ+θ˙ϖT(s)Z2˙ϖ(s)dsdθ. |
The infinitesimal operator L ofV(ζℵ,ϖℵ,ℵ) is:
LV(ζℵ,ϖℵ,ℵ)=limΔ→01Δ{E{V(ζℵ+Δ,yℵ+Δ,ℵ)|(ζℵ,ϖℵ,ℵ)}−V(ζℵ,ϖℵ,ℵ)},E{LV(ζℵ,ϖℵ,ℵ)}=4∑i=1E{LVi(ζℵ,ϖℵ,ℵ)}. | (3.3) |
Using the stochastic derivative of V(ζℵ,ϖℵ,ℵ), we can determine:
E{LV1(ζℵ,ϖℵ,ℵ)}=2ζT(ℵ)P1˙ζ(ℵ)+2ϖT(ℵ)P2˙ϖ(ℵ), | (3.4) |
E{LV2(ζℵ,ϖℵ,ℵ)}≤fT(ϖ(ℵ))Q1f(ϖ(ℵ))−(1−μ1)fT(ϖ(ℵ−σ(ℵ)))Q1f(ϖ(ℵ−σ(ℵ)))+gT(ζ(ℵ))Q2g(ζ(ℵ))−(1−μ2)gT(ζ(ℵ−κ(ℵ)))Q2g(ζ(ℵ−κ(ℵ))), | (3.5) |
E{LV3(ζℵ,ϖℵ,ℵ)}=ζT(ℵ)R1ζ(ℵ)−ζT(ℵ−κ)R1ζ(ℵ−κ)+ϖT(ℵ)R2ϖ(ℵ)−ϖT(ℵ−σ)R2ϖ(ℵ−σ), | (3.6) |
E{LV4(ζℵ,ϖℵ,ℵ)}=κ˙ζT(ℵ)Z1˙ζ(ℵ)−∫ℵℵ−κ˙ζT(s)Z1˙ζ(s)ds+σ˙ϖT(ℵ)Z2˙ϖ(ℵ)−∫ℵℵ−σ˙ϖT(s)Z2˙ϖ(s)ds, | (3.7) |
By applying Lemma 2.3 in (2.17), we can obtain
−∫ℵℵ−κ˙ζT(s)Z1˙ζ(s)ds≤−1κηT1(ℵ)[Z10003Z10005Z1]η1(ℵ), | (3.8) |
−∫ℵℵ−σ˙ϖT(s)Z2˙ϖ(s)ds≤−1σηT2(ℵ)[Z20003Z20005Z2]η2(ℵ), | (3.9) |
where
η1(ℵ)=[ζT(ℵ)−ζT(ℵ−κ)ζT(ℵ)+ζT(ℵ−κ)−2κ∫ℵℵ−κζT(s)dsζT(ℵ)−ζT(ℵ−κ)+6κ∫ℵℵ−κζT(s)ds−12κ2∫ℵℵ−κ∫tsζT(u)duds]T,η2(ℵ)=[ϖT(ℵ)−ϖT(ℵ−σ)ϖT(ℵ)+ϖT(ℵ−σ)−2σ∫ℵℵ−σϖT(s)dsϖT(ℵ)−ϖT(ℵ−σ)+6σ∫ℵℵ−σϖT(s)ds−12σ2∫ℵℵ−κ∫tsϖT(u)duds]T. |
From the assumption (A), there exists a diagonal matrices S1>0, S2>0 and Hi, Li(i=1,2), the following inequalities hold:
0≤[ϖ(ℵ)f(ϖ(ℵ))]T[−S1H1S1H2∗−S1][ϖ(ℵ)f(ϖ(ℵ))], | (3.10) |
0≤[ζ(ℵ)g(ζ(ℵ))]T[−S2L1S2L2∗−S2][ζ(ℵ)g(ζ(ℵ))]. | (3.11) |
The following equations satisfy for any matrices J1,J2 and scalar ϵ1,ϵ2:
0=2[ζT(ℵ)+ϵ1˙ζ(ℵ)]J1[−˙ζ(ℵ)+(−A+(K1+ϕ(ℵ)ΔK1(ℵ)))ζ(ℵ)+W1f(ϖ(ℵ))+W2f(ϖ(ℵ−σ(ℵ)))], | (3.12) |
0=2[ϖT(ℵ)+ϵ2˙ϖ(ℵ)]J2[−˙ϖ(ℵ)+(−B+(K2+ϱ(ℵ)ΔK2(ℵ)))ϖ(ℵ)+V1g(ζ(ℵ))+V2g(ζ(ℵ−κ(ℵ)))], | (3.13) |
substituting (3.4)–(3.6), and (3.7)–(3.23) in (3.3), we get
E{LV(ζℵ,ϖℵ,ℵ)}≤ξT(ℵ) Ξ ξ(ℵ), | (3.14) |
where ξ(ℵ)=[ζT(ℵ) ζT(ℵ−κ) gT(ζ(ℵ)) gT(ζ(ℵ−κ(ℵ))) ∫ℵℵ−κζT(s)ds ∫ℵℵ−κ∫ℵsζT(s)duds ˙ζT(ℵ) ϖT(ℵ)ϖT(ℵ−σ) fT(ϖ(ℵ)) fT(ϖ(ℵ−κ(ℵ))) ∫ℵℵ−σϖT(s)ds ∫ℵℵ−σ∫ℵsϖT(s)duds ˙ϖT(ℵ)]T.
It gives that Ξ <0. This demonstrates that the system (2.10) is asymptotically stable using the Lyapunov stability theory. The proof is now complete.
Theorem 3.2. Under Assumption (A), ϕ,ϱ and ϵi are positive scalars, then there exist symmetric matrices Pi>0, Qi>0, Ri>0, Zi>0, diagonal matrices Si>0, for any matrices Ji, Gi, and scalars ρi>0 (i=1,2) such that
˜Ξ=[ˆΞΥ1ρ1Υ2Ψ1Ψ2∗−ρ1I000∗∗−ρ1I00∗∗∗−ρ2I0∗∗∗∗−ρ2I]<0, | (3.15) |
where
ˆΞ=[ˆΠΩ∗ˆΛ],ˆΠ=[ˆΠ113Z1κS2L20−24Z1κ260Z1κ3ˆΠ17∗Π220036Z1κ2−60Z1κ30∗∗Π330000∗∗∗Π44000∗∗∗∗−192Z1κ3360Z1κ40∗∗∗∗∗−720Z1κ50∗∗∗∗∗∗Π77],ˆΛ=[ˆΛ113Z2σS1H20−24Z2σ260Z2σ3ˆΛ17∗Λ220036Z2σ2−60Z2σ30∗∗Λ330000∗∗∗Λ44000∗∗∗∗−192Z2σ3360Z2σ40∗∗∗∗∗−720Z2σ50∗∗∗∗∗∗Λ77], |
ˆΠ11=R1−S2L1−9Z1κ−J1A+G1+GT1−ATJT1,ˆΠ17=−J1−ϵ1ATJT1+ϵ1GT1+P1,ˆΛ11=R2−S1H1−J2B+G2−9Z2σ−BTJT+GT2,ˆΛ17=−J2−ϵ2BTJT2+ϵ2GT2+P2,Υ1=[ϕHT1JT1 0,...,0⏟5elements ϕϵ1HT1JT1 0,...,0⏟7elements]T,Υ2=[E1 0,...,0⏟13elements]T,Ψ1=[0,...,0⏟7elements ϱHT2JT2 0,...,0⏟5elements ϱϵ2HT2JT2]T,Ψ2=[0,...,0⏟7elements ρ2E2 0,...,0⏟6elements]T |
The controller gain matrices are also provided by in equation (2.6) are given by Ki=J−1iGi.
Proof. By using Schur complement,
[ˆΞ]+Υ1ΔK1(ℵ)ΥT2+Υ2ΔKT1(ℵ)ΥT1+Ψ1ΔK2(ℵ)ΨT2+Ψ2ΔKT2(ℵ)ΨT1<0. |
From Lemma 2, we get
˜Ξ=[ˆΞΥ1ρ1Υ2Ψ1Ψ2∗−ρ1I000∗∗−ρ1I00∗∗∗−ρ2I0∗∗∗∗−ρ2I]<0 |
It is clear that the disparities in (3.15) still exist. The evidence is now complete.
Two examples are provided in this part to show the applicability of our findings.
Example 4.1. The following BAM neural network with time varying delays:
˙ζ(ℵ)=(−A+(K1+ϕ(ℵ)ΔK1(ℵ)))ζ(ℵ)+W1f(ϖ(ℵ))+W2f(ϖ(ℵ−σ(ℵ))),˙ϖ(ℵ)=(−B+(K2+ϱ(ℵ)ΔK2(ℵ)))ϖ(ℵ)+V1g(ζ(ℵ))+V2g(ζ(ℵ−κ(ℵ))),} | (4.1) |
with the following parameters:
A=[1001], W1=[2−0.11−53.2],W2=[−1.6−0.8−0.18−2.5], H1=[0.45000.45],E1=[0.35000.35], B=[3002], V1=[1−1.11−21.2],V2=[−0.6−1.8−0.68−0.5],H2=[0.25000.25], E2=[0.15000.15], H1=L1=0, H2=L2=I. |
Additionally, we take κ=3.2, σ=3.2, μ1=0.9, μ2=0.9, ϕ=0.9, ϱ=0.9, ϵ1=0.5, and ϵ2=0.5. By solving the LMIs in Theorem 3.2, we get
P1=[48.3823−0.5707−0.570749.5117], P2=[46.62800.25890.258950.0702],Q1=[19.28354.76024.760228.1068], Q2=[19.03795.74755.747525.7761],R1=[35.0318−1.4108−1.410831.6352],R2=[35.2798−1.5288−1.528833.4075],Z1=[0.01670.00090.00090.0116], Z2=[0.0332−0.0196−0.01960.0971],G1=[−96.51481.09481.0948−99.3356],G2=[−91.4745−1.3821−1.3821−96.5435],J1=[0.15710.03320.03320.1827], J2=[0.3903−0.1807−0.18070.9874]ρ1=42.3781, ρ2=44.9762. |
As well as the following non-fragile controller gains matrices:
K1=[−639.9952126.6829122.2775−566.6860], K2=[−256.7506−53.3312−48.3949−107.5330]. |
In this scenario is proved the given system is asymptotically stable. In Figure 1, state response are depicted.
The issue of BAM delayed neural networks with non-fragile control and controller gain fluctuation has been examined in this article. To ensure the stability of the aforementioned systems, delay-dependent conditions are established. In terms of linear matrix inequalities (LMIs), necessary conditions are obtained by building an L-K functional and utilizing the traditional integral inequality technique. This technique ensures asymptotically stability of addressed to the concerned neural networks. Lastly, a numerical example is provided to demonstrate the viability of the findings in this study. Stochastic differential equations and complex networks both respond well to this approach in future research.
[1] | Adhikary K (2017) Ethnobotany, commercialisation and climate change: consequences of the exploitation of yarsagumba in Nepal. Eur Bull Himal Res 49: 35-58. |
[2] | Banjade MR, Paudel NS (2008) Economic potential of non-timber forest products in Nepal: myth or reality? J For Livelihood 7: 36-48. |
[3] | Bhattacharya P, Hayat SF (2004) Sustainable NTFP management for rural development: a case from Madhya Pradesh, India. Int For Rev 6: 161-168. |
[4] |
Caplins L (2017) Collecting Ophiocordyceps sinensis: an emerging livelihood strategy in the Garhwal, Indian Himalaya. J Mt Sci 14: 390-402. doi: 10.1007/s11629-016-3892-8
![]() |
[5] | Choudhary PR (2007) Forest-route to poverty alleviation-myths and realities: analysis of NTFP-livelihood linkages in some Indian states. A poster presented in the RRI Conference in Bangkok, 4-7. |
[6] | Chowdhury S, Gulati A, Gumbira-Said E (2005) High value products, supermarkets, and vertical arragements in Indonesia. Markets, Trade and Institutions Division (MTID) Discussion Paper no 83, International Food Policy Research Institute. |
[7] |
Dallas MP, Ponte S, Sturgeon TJ (2019) Power in global value chains. Rev Int Polit Econ 26: 666-694. doi: 10.1080/09692290.2019.1608284
![]() |
[8] | Devkota S (2007) Yarsagumba [Cordyceps sinensis (Berk.) Sacc.]; Traditional utilization in Dolpa District, Western Nepal. Our Nat 4: 48-52. |
[9] | Dispatch N (2014) Darchula locals killed while hunting for Yarsagumba. Available from: http://www.nepaldispatch.com/2013/06/8-darchula-locals-killed-while-hunting-for-yarsagumba/. |
[10] | GON (2010) Nepal Trade Integration Strategy 2010, Kathmandu, Nepal. |
[11] | GON (2013) Jadibuti bikri bitaran samchhipta lagat: arthik barsa 2070 (A brief NTFPs sale and distribution in fiscal year 2012/2013), Government of Nepal. |
[12] | Government of Uttarakhand (2018) Yarsagumba collection procedure, Government of Uttarakhand, India. |
[13] |
Gubbi S, MacMillan DC (2008) Can non-timber forest products solve livelihood problems? A case study from Periyar Tiger Reserve, India. Oryx 42: 222-228. doi: 10.1017/S0030605308071111
![]() |
[14] |
Harsanyi JC (1963) A simplified bargaining model for the n-person cooperative game. Int Econ Rev 4: 194-220. doi: 10.2307/2525487
![]() |
[15] |
Hellmann T (2007) Entrepreneurs and the Process of Obtaining Resources. J Econ Manage Strat 16: 81-109. doi: 10.1111/j.1530-9134.2007.00133.x
![]() |
[16] | Hishe M, Asfaw Z, Giday M (2016) Review on value chain analysis of medicinal plants and the associated challenges. J Med Plants Stud 4: 45-55. |
[17] | Isaksen ET, Richter A (2019) Tragedy, property rights, and the commons: Investigating the causal relationship from institutions to ecosystem collapse. J Assoc Environ Resour Econ 6: 741-781. |
[18] |
Iversen V, Chhetry B, Francis P, et al. (2006) High value forests, hidden economies and elite capture: Evidence from forest user groups in Nepal's Terai. Ecol Econ 58: 93-107. doi: 10.1016/j.ecolecon.2005.05.021
![]() |
[19] | Jolly J (2011) Yarsagumba: Curse of Himalayan Annapurna region. BBC News: South Asia. Available from: http://wwwbbccouk/news/world-south-asia-12110240 Access on: July 25, 2014. |
[20] | Kantipur Daily (2014) Yarcha pickers robbed of millions. Available from: http://www.ekantipur.com/2014/06/21/top-story/yarcha-pickers-robbed-of-millions/391125 Access on: July 25, 2014. |
[21] | Langdon S, Worl R (1981) Distribution and exchange of subsistence resources in Alaska. University of Alaska Arctic Environmental Information and Data Center, Anchorage, Alaska. |
[22] |
Mahapatra AK, Shackleton CM (2011) Has deregulation of non-timber forest product controls and marketing in Orissa state (India) affected local patterns of use and marketing. For Policy Econ 13: 622-629. doi: 10.1016/j.forpol.2011.07.009
![]() |
[23] | MOFE (2017) Yarsagumba Management (Collection and Transportation) Guideline 2017, Ministry of Forests and Environment, Kathmandu, Nepal. |
[24] | Moncrieff CF (2007) Traditional ecological knowledge of customary trade of subsistence harvested fish on the Yukon River. U.S. Fish and Wildlife Service, Office of Subsistence Management, Fisheries Resource Monitoring Program, 2007 Final Report (Study No. 04-265), Anchorage, Alaska. |
[25] |
Negi CS (2007) Declining transhumance and subtle changes in livelihood patterns and biodiversity in the Kumaon Himalaya. Mt Res Dev 27: 114-118. doi: 10.1659/mrd.0818
![]() |
[26] | Neupane N (2015) Solving transborder water issues in changing climate scenarios of South Asia, Governing access to essential resources, Columbia University Press. |
[27] |
Olsen CS, Bhattarai N (2005) A Typology of conomic gents in the Himalayan plant trade. Mt Res Dev 25: 37-43. doi: 10.1659/0276-4741(2005)025[0037:ATOEAI]2.0.CO;2
![]() |
[28] |
Pant B, Rai RK, Wallrapp C, et al. (2017) Horizontal integration of multiple institutions: Solution for yarsagumba related conflicts in the himalayan region of Nepal? Int J Commons 11: 464-486. doi: 10.18352/ijc.717
![]() |
[29] | Pant B, Wallrapp C, Ram AK, et al. (2014) Across the Mahakali, Yarsagumba Collection. International Center for Integrated Mountain Development. Kathmandu, Nepal. Available from: https://www.youtube.com/watch?v=85FLh1HqFds. Accessed on: 11 November, 2020. |
[30] |
Pouliot M, Pyakurel D, Smith C (2018) High altitude organic gold: the production network for Ophiocordyceps sinensis from far-western Nepal. J Ethnopharmacol 218: 59-68. doi: 10.1016/j.jep.2018.02.028
![]() |
[31] |
Purcell SW, Crona BI, Lalavanua W, et al. (2017) Distribution of economic returns in small-scale fisheries for international markets: A value-chain analysis. Mar Policy 86: 9-16. doi: 10.1016/j.marpol.2017.09.001
![]() |
[32] |
Pyakurel D, Bhattarai SI, Smith-Hall C (2018) Patterns of change: The dynamics of medicinal plant trade in far-western Nepal. J Ethnopharmacol 224: 323-334. doi: 10.1016/j.jep.2018.06.004
![]() |
[33] | Pyakurel D, Oli BR (2012) NTFPs/MAPs business promotion strategy (2012-2016), from private sector perspective, FNCCI-AEC/NEHHPA, Kathmandu, Nepal. |
[34] | Rausser GC, Zusman P (1991) Organizational failure and the political economy of water resources management, California, United States of America. |
[35] |
Safarzynska K, van den Bergh JCJM (2010) Evolving power and environmental policy: explaining institutional change with group selection. Ecol Econ 69: 743-752. doi: 10.1016/j.ecolecon.2009.04.003
![]() |
[36] |
Shrestha UB, Bawa KS (2014) Economic contribution of Chinese caterpillar fungus to the livelihoods of mountain communities in Nepal. Biol Conserv 177: 194-202. doi: 10.1016/j.biocon.2014.06.019
![]() |
[37] |
Shrestha UB, Bawa KS (2013) Trade, harvest, and conservation of caterpillar fungus (Ophiocordyceps sinensis) in the Himalayas. Biol Conserv 159: 514-520. doi: 10.1016/j.biocon.2012.10.032
![]() |
[38] | Shrestha UB, Dhital KR, Gautam AP (2017) Economic dependence of mountain communities on Chinese caterpillar fungus Ophiocordyceps sinensis (yarsagumba): a case from western Nepal. Oryx 53: 1-9. |
[39] | Sills E, Shanley P, Paumgarten F, et al. (2011) Evolving perspectives on non-timber forest products, In: Non-timber forest products in the global context, Springer, Berlin, Heidelberg, 23-51. |
[40] | Sunderland T, Ndoye O (2004) Forest products, livelihoods and conservation: Case studies of non-timber forest products systems. FAO 2: 1-55. |
[41] | TEPC (2013) Export import data bank, trade and energy promotion centre, Ministry of Commerce and Supplies, Government of Nepal. |
[42] | THT (2013) Yarsa worth millions flown to Kathmandu. The Himalayan Times. Available from: http://www.thehimalayantimescom/fullNewsphp?headline=Yarsa+worth+millions+flown+to+Kathmandu&NewsID=391049. |
[43] | Tokatli N (2006) Asymmetrical power relations and upgrading among suppliers of global clothing brands: Hugo Boss in Turkey. J Econ Geogr 7: 67-92. |
[44] | United Nations (2017) The Sustainable Development Goals Report. United Nations. |
[45] |
Wallrapp C, Faust H, Keck M (2019a) Production networks and borderlands: cross-border yarsagumba trade in the Kailash Landscape. J Rural Stud 66: 67-76. doi: 10.1016/j.jrurstud.2019.01.016
![]() |
[46] |
Wallrapp C, Keck M, Faust H (2019b) Governing the yarshagumba 'gold rush': a comparative study of governance systems in the Kailash landscape in India and Nepal. Int J Commons 13: 1-24. doi: 10.18352/ijc.884
![]() |
[47] | Wangchuk S, Norbu N, Sherub S (2012) Impacts of Cordyceps collection on livelihoods and alpine ecosystems in Bhutan as ascertained from questionnaire survey of Cordyceps collectors, Royal Government of Bhutan, UWICE Press, Bumthang. |
[48] |
Winkler D (2008a) Yartsa Gunbu (Cordyceps sinensis) and the fungul commodification of Tibet's rural economy. Econ Bot 62: 291-305. doi: 10.1007/s12231-008-9038-3
![]() |
[49] | Winkler D (2008b) The mushrooming fungi market in Tibet exemplified by Cordyceps sinensis and Tricholoma matsutake. J Int Assoc Tibetan Stud 4: 1-46. |
[50] |
Winkler D (2009) Caterpillar fungus (Ophiocordyceps sinensis) production and sustainability on the Tibetan plateau and in the Himalayas. Asian Med 5: 291-316. doi: 10.1163/157342109X568829
![]() |
[51] |
Wood RC (1994) Negotiation basics: concepts, skills and exercises. Service Ind J 14: 590-591. doi: 10.1080/02642069400000060
![]() |
[52] |
Wu SY, Roe B (2007) Contract enforcement, social efficiency, and distribution: some experimental evidence. Am J Agr Econ 89: 243-258. doi: 10.1111/j.1467-8276.2007.00996.x
![]() |
[53] | Yang ZL (2020) Ophiocordyceps sinensis, The IUCN red list of threatened species 2020: e.T58514773A58514845. |
1. | Chengqiang Wang, Xiangqing Zhao, Can Wang, Zhiwei Lv, Synchronization of Takagi–Sugeno Fuzzy Time-Delayed Stochastic Bidirectional Associative Memory Neural Networks Driven by Brownian Motion in Pre-Assigned Settling Time, 2023, 11, 2227-7390, 3697, 10.3390/math11173697 | |
2. | A. Karnan, G. Nagamani, Event-triggered extended dissipative synchronization for delayed neural networks with random uncertainties, 2023, 175, 09600779, 113982, 10.1016/j.chaos.2023.113982 |