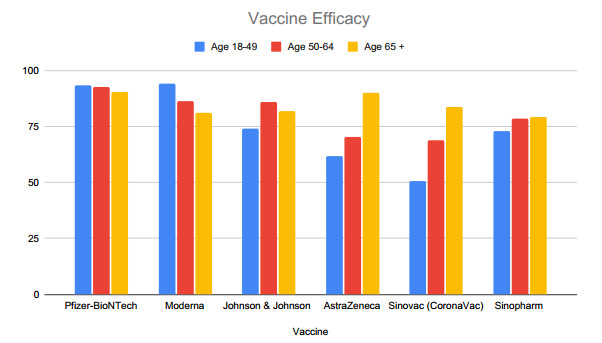
Vaccination programs are crucial for reducing the prevalence of infectious diseases and ultimately eradicating them. A new age-structured SEIRV (S-Susceptible, E-Exposed, I-Infected, R-Recovered, V-Vaccinated) model with imperfect vaccination is proposed. After formulating our model, we show the existence and uniqueness of the solution using semigroup of operators. For stability analysis, we obtain a threshold parameter R0. Through rigorous analysis, we show that if R0<1, then the disease-free equilibrium point is stable. The optimal control strategy is also discussed, with the vaccination rate as the control variable. We derive the optimality conditions, and the form of the optimal control is obtained using the adjoint system and sensitivity equations. We also prove the uniqueness of the optimal controller. To visually illustrate our theoretical results, we also solve the model numerically.
Citation: Manoj Kumar, Syed Abbas, Abdessamad Tridane. Optimal control and stability analysis of an age-structured SEIRV model with imperfect vaccination[J]. Mathematical Biosciences and Engineering, 2023, 20(8): 14438-14463. doi: 10.3934/mbe.2023646
[1] | Xi-Chao Duan, Xue-Zhi Li, Maia Martcheva . Dynamics of an age-structured heroin transmission model with vaccination and treatment. Mathematical Biosciences and Engineering, 2019, 16(1): 397-420. doi: 10.3934/mbe.2019019 |
[2] | Amira Bouhali, Walid Ben Aribi, Slimane Ben Miled, Amira Kebir . Impact of immunity loss on the optimal vaccination strategy for an age-structured epidemiological model. Mathematical Biosciences and Engineering, 2024, 21(6): 6372-6392. doi: 10.3934/mbe.2024278 |
[3] | Eunha Shim . Optimal strategies of social distancing and vaccination against seasonal influenza. Mathematical Biosciences and Engineering, 2013, 10(5&6): 1615-1634. doi: 10.3934/mbe.2013.10.1615 |
[4] | Francesco Salvarani, Gabriel Turinici . Optimal individual strategies for influenza vaccines with imperfect efficacy and durability of protection. Mathematical Biosciences and Engineering, 2018, 15(3): 629-652. doi: 10.3934/mbe.2018028 |
[5] | Han Ma, Qimin Zhang . Threshold dynamics and optimal control on an age-structured SIRS epidemic model with vaccination. Mathematical Biosciences and Engineering, 2021, 18(6): 9474-9495. doi: 10.3934/mbe.2021465 |
[6] | Kai Zhang, Xinwei Wang, Hua Liu, Yunpeng Ji, Qiuwei Pan, Yumei Wei, Ming Ma . Mathematical analysis of a human papillomavirus transmission model with vaccination and screening. Mathematical Biosciences and Engineering, 2020, 17(5): 5449-5476. doi: 10.3934/mbe.2020294 |
[7] | Rinaldo M. Colombo, Mauro Garavello . Optimizing vaccination strategies in an age structured SIR model. Mathematical Biosciences and Engineering, 2020, 17(2): 1074-1089. doi: 10.3934/mbe.2020057 |
[8] | Zhiping Liu, Zhen Jin, Junyuan Yang, Juan Zhang . The backward bifurcation of an age-structured cholera transmission model with saturation incidence. Mathematical Biosciences and Engineering, 2022, 19(12): 12427-12447. doi: 10.3934/mbe.2022580 |
[9] | Toshikazu Kuniya, Taisuke Nakata, Daisuke Fujii . Optimal vaccine allocation strategy: Theory and application to the early stage of COVID-19 in Japan. Mathematical Biosciences and Engineering, 2024, 21(6): 6359-6371. doi: 10.3934/mbe.2024277 |
[10] | Abba B. Gumel, C. Connell McCluskey, James Watmough . An sveir model for assessing potential impact of an imperfect anti-SARS vaccine. Mathematical Biosciences and Engineering, 2006, 3(3): 485-512. doi: 10.3934/mbe.2006.3.485 |
Vaccination programs are crucial for reducing the prevalence of infectious diseases and ultimately eradicating them. A new age-structured SEIRV (S-Susceptible, E-Exposed, I-Infected, R-Recovered, V-Vaccinated) model with imperfect vaccination is proposed. After formulating our model, we show the existence and uniqueness of the solution using semigroup of operators. For stability analysis, we obtain a threshold parameter R0. Through rigorous analysis, we show that if R0<1, then the disease-free equilibrium point is stable. The optimal control strategy is also discussed, with the vaccination rate as the control variable. We derive the optimality conditions, and the form of the optimal control is obtained using the adjoint system and sensitivity equations. We also prove the uniqueness of the optimal controller. To visually illustrate our theoretical results, we also solve the model numerically.
Imperfect or leaky vaccines are those that do not provide complete immunity to the disease in their hosts and are incapable of preventing transmission to others. It is known that leaky vaccines can reduce the symptoms of the disease but do not offer full protection against infection or the spread of the pathogen. Therefore, studying the impact of imperfect vaccines on the human population becomes crucial. Additionally, for certain infectious diseases, it has been observed that the effectiveness of the vaccine may diminish over time. In the recent COVID-19 pandemic, it has been noted that most vaccines remain effective for a specific duration before individuals become susceptible to the virus once again.
During the course of the follow-up period of six months, Stephen et al. [1] studied the vaccine efficacy of different vaccines (shown in Figure 1). Menni et al. [2] showed that in the case of COVID-19, the vaccine's efficacy remained high after five months among those under the age of 55. They also showed that the booster dose can restore the effectiveness of the vaccine. Jake et al. [3] also studied the vaccine efficacy and demonstrated that the evidence of waning immunity is overstated. They demonstrated that antibody levels can be boosted in the general population and robust clinical data are required to assess the need for additional doses. This naturally motivates us to consider a compartmental model for infectious diseases with a vaccine-waning rate.
Kermack and Mckendrick [5,6,7] developed ordinary differential equations (ODEs) based models to study the transmission of infectious diseases. These models are formulated based on the assumption that all individuals have identical vital rates regardless of age or size. Enough literature is available on ODE-based infectious disease models; more details can be found in [8,9,10,11,12]. In epidemic models, the "disease clock" is important for studying the long-term dynamics of an infectious disease. Moreover, in transmitting infectious diseases, the age of infection or the time since infection starts plays a vital role. Age-structured population models characterize individuals based on their age, which can be class-age or demographic age. Class age means the time since infection, and demographic age means the chronological age of individuals.
Iannelli [13] discussed age-structured population models and their role in describing the growth and interaction of populations. Thieme and Castilo [14] examined the role of infection age in the HIV viral dynamics. More details about age and size-structured population models and their applications in biology and epidemiology are given in [16]. For the first time, Arino et al. [17] discussed the role of leaky vaccines with a general waning function. They consider three classes S, I, and V (without age structure), corresponding to susceptible, infected, and vaccinated individuals. They showed that subthreshold equilibria are possible, which may play an important role while designing vaccine strategies. Optimal control strategies for age-structured compartmental models are discussed by [18,19]. Semi-random epidemic network models can also describe the dynamics of many infectious diseases [20]. Awel et al. [19] incorporated imperfect vaccination in their model for Malaria disease, and Wei et al. [21] studied imperfect vaccination on scale-free networks. Many authors have also considered delay terms in fractional-order population models with or without structure variables. For more details, we refer to [22,23,24,25]. There are many interesting works on age and size-structured models for infectious diseases (see, for example, [26,27,28,29,30,31,32]).
We propose an age-structured SEIRV model with imperfect vaccination and vaccine-waning rates. After formulation of our model, we show the existence and uniqueness of the solution. We use semigroup of operators to show the existence and uniqueness of the solution. We find steady-state solutions to our model and check the stability of the disease-free steady state. We also study the optimal control problem with vaccination rate as a control variable. Our objective is to minimize the cost of vaccination and to reduce the number of infected individuals. Using adjoint equations, we derive the form of the optimal control variable. We show that the optimal control variable depends on the vaccine waning rate, infection rate, and the cost of vaccination. We also solve our model numerically to validate our theoretical findings. Our study is mathematical, and we study the qualitative properties of the solution. We obtain an explicit form for the optimal control variable, which involves important parameters of the proposed model. Due to the complex partial differential equations-based model, it is difficult to get the data, so we do not validate our model with real data. But the model results are interesting and involve realistic assumptions.
Our work is divided in the following manner. In Section 2, we formulated our model, and using semigroup of operators, we show the existence and uniqueness of the solution. Section 3 is devoted to the stability analysis of disease-free equilibrium points. In Section 4, we study the optimal control problem. In Section 5, we solve our model numerically, and the last section is devoted to the concluding remarks.
In this section, we formulate our model. We also show the existence and uniqueness of the solution to the model under consideration. We convert our problem into the abstract Cauchy problem to show the existence of the solution.
Let S(a,t),E(a,t),I(a,t) and R(a,t) denote the age and time-dependent population densities of individuals in the susceptible, exposed, infected, and recovered class. Whenever there is no confusion in the notation, we use the notation S,E,I and R for S(a,t),E(a,t),I(a,t) and R(a,t) respectively. We denote the age-dependent transmission coefficient by c(a,b), which describes the interaction between susceptible and infected class, that is, c(a1,a2)S(a1,t)I(a2,t)da1da2 is the number of susceptible individuals aged in (a1,a1+da1) that contract the disease after interacting with infected individuals aged in (a2,a2+da2). We assume that the functional form of the force of infection is given by
φ(a,t)=∫am0c(a,σ)I(σ,t)dσ, |
where am is the maximum age which an individual can attain. Then the following system of partial differential equations (PDEs) describes the spread of disease
∂S∂t+∂S∂a=Λ−φ(a,t)S−m(a)S−v(a)S+w0(a)V∂E∂t+∂E∂a=φ(a,t)S−m1E−m(a)E∂I∂t+∂I∂a=m1E−γI−m(a)I+βσ(a)IV∂R∂t+∂R∂a=γI−m(a)R∂V∂t+∂V∂a=−(m(a)+w0(a)+βσ(a)I)VS(0,t)=S1,E(0,t)=I(0,t)=R(0,t)=0,V(0,t)=∫am0v(a)S(a,t)daS(a,0)=S0(a),E(a,0)=E0(a),I(a,0)=I0(a),R(a,0)=R0(a)andV(a,0)=V0(a), | (2.1) |
where φ(a,t)=∫am0c(a,σ)I(σ,t)dσ.
m(a) | Mortality rate of age a individuals |
m1 | Rate at which individuals move from exposure to onset of symptoms |
v(a) | Age-dependent Vaccination policy |
w0(a) | Vaccine wanes rate |
γ | Recovery rate of infected population |
σ(a) | Infection ratio of vaccinated individuals |
βσ(a) | Rate at which vaccinated individuals can be infected |
Let X=R4×L1((0,am),R)×L1((0,am),R)×L1((0,am),R)×L1((0,am),R) and let us define the linear operator
A(0000xyzw)=(x(0)y(0)z(0)w(0)−x′−(m(a)+v(a))x−y′−(m1+m(a))y−z′−(γ+m(a))z−w′−(m(a)+w0(a))w) |
with
D(A)={0}×{0}×{0}×{0}×W1,1((0,am),R)×W1,1((0,am),R)×W1,1((0,am),R)×W1,1((0,am),R). |
Here it is clear that ¯D(A) is not dense in X. Let us also define the nonlinear operator in the following manner
F(0000xyzw)=(S100∫am0v(a)x(a)daΛ−x(a)∫am0c(a)z(a)da+w0(a)wx(a)∫am0c(a)z(a)dam1y+βσ(a)z(a)w(a)−βσ(a)z(a)w(a)). |
Our model in abstract form can be written as
{ddtv(t)=Av(t)+F(v(t)),t≥0v(0)=v0, | (2.2) |
where v(t)=(0,0,0,0,S(⋅,t),E(⋅,t),I(⋅,t),V(⋅,t)) and v0=(0,0,0,0,S0,E0,I0,V0). Instead of solving (2.2), we consider the following integral equation
v(t)=v0+A∫t0v(s)ds+∫t0F(v(s))ds. | (2.3) |
We have the following assumptions:
(i) m,w0,v,σ are positive functions and lies in L∞+((0,am),R).
(ii) For 0≤a≤am, we assume that 0<σ(a)<1.
Now, our task is to prove that A is a Hille-Yosida operator and therefore it generates a C0 semigroup on the closure of D(A). Linearized system of (2.2) can be written as
{ddtv(t)=Av(t)+DF(v∗)v(t),t≥0v(0)=v0−v∗, | (2.4) |
where v∗ is steady state solution of (2.2) and
DF(v∗)(0000xyzw)=(S100∫am0v(a)x(a)da−x∗(a)∫am0c(a)z(a,t)da−x(a,t)∫am0c(a)z∗(a)da+w0(a)w(a,t)x∗(a)∫am0c(a)z(a,t)da+x(a,t)∫am0c(a)z∗(a)dam1y+βσ(a)z∗(a)w(a,t)+βσ(a)z(a,t)w∗(a)−βσ(a)z∗(a)w(a,t)−βσ(a)z(a,t)w∗(a)). |
Here, DF(v∗) is a compact bounded linear operator on X. Let
Ω={λ∈C:Reλ>−w0}, |
where
w0=min{infa(m(a)+v(a)),infa(m1+m(a)),infa(γ+m(a)),infa(m(a)+w0(a))}. |
Now, our task is to prove that (A,D(A)) is a Hille-Yosida operator. For
(ϕ1(⋅),ϕ2(⋅),ϕ3(⋅),ϕ4(⋅),ϕ5(⋅),ϕ6(⋅),ϕ7(⋅),ϕ8(⋅))∈Xand(0,0,0,0,ξ1(⋅),ξ2(⋅),ξ3(⋅),ξ4(⋅)) |
we have
(λI−A)−1(ϕ1(⋅)ϕ2(⋅)ϕ3(⋅)ϕ4(⋅)ϕ5(⋅)ϕ6(⋅)ϕ7(⋅)ϕ8(⋅))=(0000ξ1(⋅)ξ2(⋅)ξ3(⋅)ξ4(⋅)). |
This implies
ξ′1+(λ+m(a)+v(a))ξ1(a)=ϕ5(a)ξ′2+(λ+m1+m(a))ξ2(a)=ϕ6(a)ξ′3+(λ+γ+m(a))ξ3(a)=ϕ7(a)ξ′4+(λ+m(a)+w0(a))ξ4(a)=ϕ8(a)ξ1(0)=ϕ1ξ2(0)=ϕ2ξ3(0)=ϕ3ξ4(0)=ϕ4. |
Solving we get,
ξ1(a)=ϕ1e−∫a0(λ+m(s)+v(s))ds+∫a0ϕ5(s)e−∫as(λ+m(τ)+v(τ))dτdsξ2(a)=ϕ2e−∫a0(λ+m1+m(s))ds+∫a0ϕ6(s)e−∫as(λ+m1+m(τ))dτdsξ3(a)=ϕ3e−∫a0(λ+γ+m(s))ds+∫a0ϕ7(s)e−∫as(λ+γ+m(τ))dτdsξ4(a)=ϕ4e−∫a0(λ+m(s)+w0(s))ds+∫a0ϕ8(s)e−∫as(λ+m(τ)+w0(τ))dτds. |
Now, taking L1 norm we get
‖ξ1‖L1+‖ξ2‖L1+‖ξ3‖L1+‖ξ4‖L1≤1λ+w0(‖ξ1‖L1+‖ξ2‖L1+‖ξ3‖L1+‖ξ4‖L1). |
Therefore, we have
‖(λI−A)−1‖≤1λ+w0for allλ∈Ω. |
So, we conclude that (A,D(A)) is a Hille-Yosida operator.
Now, let us recall the following results.
Lemma 2.1. [Pazy [33], 1983] Let X be a Banach space. Let operator A with domain D(A) be a Hille-Yosida operator on X and B be a bounded linear operator on X. Then A+B is also a Hille-Yosida operator.
Lemma 2.2. [Pazy [33], 1983] Let (A,D(A)) be a Hille-Yosida operator, then its part (A0,D(A0)) generates a C0 semigroup {T0(t)|t≥0} on X0, where X0=(¯D(A),‖⋅‖).
Using Lemmas 2.1 and 2.2, and proof given above, we conclude that the operator A+DF(v∗) is a Hille-Yosida operator and the part of (A,D(A) and the part of (A+DF(u∗)) generate C0 semigroups on X0. So, the proof given above and Lemma 2.1, Lemma 2.2 prove the existence and uniqueness of mild solution to our model (for more details we refer to Pazy [33]). So, we have the following theorem:
Theorem 2.3. For any v0∈D+={0}×{0}×{0}×{0}×W1,1((0,am),R+)×W1,1((0,am),R+)×W1,1((0,am),R+)×W1,1((0,am),R+) system 2.4 has a unique continuous solution with initial data in D+. Moreover, the function defined by Ψ(t,v0)=v(t,v0) is strongly continuous semigroup.
Remark 2.4. Since Ψ(t,v0) is strongly continuous semigroup, there exists M≥1,ω≥0 such that ‖Ψ(t)‖≤Meωt. So, if we assume t∈[0,T] for some finite T>0, then we have the boundedness of solution to the abstract Cauchy problem 2.2.
In this section, we discuss the stability analysis of disease-free steady state. Steady-state solutions play an important role to study the qualitative properties of the solution when the explicit form of the solution is not known. Steady-state equations for our model are given by
dSda=Λ−φ(a)IS−m(a)S−v(a)S+w0(a)VdEda=φ(a)IS−m1E−m(a)EdIda=m1E−γI−m(a)I+βσ(a)IVdRda=γI−m(a)RdVda=−(m(a)+w0(a)+βσ(a)I)VS(0)=S1,E(0)=I(0)=R(0)=0V(0)=∫am0v(a)S(a)da. | (3.1) |
Disease free steady state (S0(a),0,0,0,V0(a)) is given by
S0(a)=S1e−∫a0(m(τ)+v(τ))dτ+∫a0(Λ−w0(s)V0(s))e−∫sa(m(τ)+v(τ))dτds | (3.2) |
V0(a)=(∫am0v(τ)S0(τ)dτ)e−∫a0(m(s)+w0(s))ds. | (3.3) |
Now, our task is to derive expressions for S0 which is independent of V0 and similarly for V0. Let
Θ=∫am0v(τ)S0(τ)dτ. | (3.4) |
Now, Eqs (3.2) and (3.3) can be written as
S0(a)=S1e−∫a0(m(τ)+v(τ))dτ+∫a0(Λ−w0(s)Θe−∫s0(m(τ)+w0(τ))dτ)e−∫sa(m(τ)+v(τ))dτds | (3.5) |
V0(a)=Θe−∫a0(m(s)+w0(s))ds. | (3.6) |
Now, substituting value of S0 from (3.5) into (3.4), we get
Θ=∫a0S1v(a)e−∫a0(m(τ)+v(τ))dτda+∫a0Λe−∫sa(m(τ)+v(τ))dτda1+∫am0v(a)(∫a0w0(s)e−∫s0(m(τ)+w0(τ))dτe−∫sa(m(τ)+v(τ))dτds)da | (3.7) |
So, we have steady-state solutions in explicit form. From these solutions, it is clear that both S0 and V0 are non-negative. Let us define the threshold number in the following manner (for more details we refer to [27]):
R0=m1∫am0S0(z)φ(z)e−∫az(m1+m(τ))dτ(∫z0e−∫zs(γ+m(τ)−βσ(τ)V0(τ))dτds)dz. | (3.8) |
The above defined threshold parameter may not be biologically relevant but plays an important role to study the stability of disease-free steady state. We can state the following result which relates the stability of disease-free steady state with the threshold parameter R0.
Theorem 3.1. The disease free steady state is stable if R0<1 and unstable if R0>1.
Proof. For the local stability of the disease-free equilibrium point, firstly we will linearize our system around the disease-free steady state. Linearizing system (2.1) around the disease-free equilibrium, we get
∂S∂t+∂S∂a=−φ(a)S0(a)I−m(a)S−v(a)S+w0(a)V∂E∂t+∂E∂a=φ(a)S0(a)I−m1E−m(a)E∂I∂t+∂I∂a=m1E−γI−m(a)I+βσ(a)V0(a)I∂R∂t+∂R∂a=γI−m(a)R∂V∂t+∂V∂a=−(m(a)+w0(a))V−βσ(a)V0(a)IS(0,t)=S1,E(0,t)=I(0,t)=R(0,t)=0V(0,t)=∫am0v(a)S(a,t)daS(a,0)=S0(a),E(a,0)=E0(a),I(a,0)=I0(a)R(a,0)=R0(a)andV(a,0)=V0(a). | (3.9) |
We are looking for solutions of the form
S(a,t)=ˉS(a)eλt,E(a,t)=ˉE(a)eλt,I(a,t)=ˉI(a)eλt,R(a,t)=ˉR(a)eλt,V(a,t)=ˉV(a)eλt. |
Therefore, system (3.9) becomes
dˉSda+λˉS=−φ(a)S0(a)ˉI−m(a)ˉS−v(a)ˉS+w0(a)ˉVdˉEda+λˉE=φ(a)S0(a)ˉI−m1ˉE−m(a)ˉEdˉIda+λˉI=m1ˉE−γˉI−m(a)ˉI+βσ(a)V0(a)ˉIdˉRda+λˉR=γˉI−m(a)ˉRdˉVda+λˉV=−(m(a)+w0(a))ˉV−βσ(a)V0(a)ˉI | (3.10) |
Solving these equations, we get
ˉS(a)=S1e−∫a0(λ+m(τ)+v(τ))dτ+∫a0(w0(z)ˉV(z)−S0(z)φ(z)ˉI(z))e−∫az(λ+m(τ)+v(τ))dτdzˉE(a)=∫a0S0(z)φ(z)ˉI(z)e−∫az(λ+m1+m(τ))dτdzˉI(a)=∫a0m1ˉE(z)e−∫az(λ+γ+m(τ)−βσ(τ)V0(τ))dτdzˉR(a)=∫a0γˉI(z)e−∫az(λ+m(τ))dτdzˉV(a)=e−∫a0(λ+m(τ)+w0(τ))dτ∫am0v(a)ˉS(a)da−∫a0βσ(z)V0(z)ˉI(z)e−∫az(λ+m(τ)+w0(τ))dτdz. |
Substituting value of ˉE(a) in ˉI(a), we get
ˉI(a)=∫a0m1(∫z0(S0(η)φ(η)ˉI(η))e−∫zη(λ+m1+m(τ))dτdη)e−∫az(λ+γ+m(τ)−βσ(τ)V0(τ))dτdz |
Observe that
dˉIda+λˉI(a)=m1(∫a0S0(η)φ(η)ˉI(η)e−∫aη(λ+m1+m(τ))dτdη)−γˉI(a)−m(a)ˉI(a)+βσ(a)V0(a)ˉI(a). |
In simplified form, it can be written as
dˉIda+(λ+γ+m(a)−βσ(a)V0(a))ˉI(a)=m1(∫a0S0(z)φ(z)ˉI(z)e−∫az(λ+m1+m(τ))dτdz). | (3.11) |
Using (3.11), the characteristic equation can be written as
G(λ)=1, |
where
G(λ)=m1∫a0S0(z)φ(z)e−∫az(λ+m1+m(τ))dτ(∫z0e−∫zs(λ+γ+m(τ)−βσ(τ)V0(τ))dτds)dz | (3.12) |
It is easy to see that G(0)=R0. G can also be written as
G(λ)=m1∫a0e−λ(a−z)S0(z)φ(z)e−∫az(m1+m(τ))dτ(∫z0e−λ(z−s)e−∫zs(γ+m(τ)−βσ(τ)V0(τ))dτds)dz | (3.13) |
Taking derivative of G with respect to λ, we get
G′(λ)=−m1∫a0(a−z)e−λ(a−z)S0(z)φ(z)e−∫az(m1+m(τ))dτ(∫z0e−λ(z−s)e−∫zs(γ+m(τ)−βσ(τ)V0(τ))dτds)dz−m1∫a0e−λ(a−z)S0(z)φ(z)e−∫az(m1+m(τ))dτ(∫z0(z−s)e−λ(z−s)e−∫zs(γ+m(τ)−βσ(τ)V0(τ))dτds)dz. |
Observe that G is a decreasing function of λ as G′(λ)<0 and limλ→∞G(λ)=0. Let us assume that λ=x1+ix2 is a root of equation G(λ)=1. Then for x1≥0, we have
1=|G(λ)|≤|G(0)|≤R0. |
Thus the real part of eigenvalue λ is negative if threshold parameter R0<1. Therefore, the disease free equilibrium point is locally asymptotically stable if R0<1 and unstable if R0>1.
In this section, we discuss the optimal control strategy for our problem. Optimal control theory plays an important role in epidemiological models because it is very important to control the spread of disease in an optimal manner. Here, our control variable is vaccination effort and we want to minimize the cost of vaccination and also our aim is to reduce the number of infected individuals. We assume that φ(a,t)=ψ(a,t)I(a,t). For this form of φ(a,t), it is easy to construct operators A and F to show the existence and uniqueness of the solution. Also, we assume that v depends on both age variable a and time t. We consider the following optimal control problem:
∂S∂t+∂S∂a=Λ−ψ(a,t)IS−m(a)S−v(a,t)S+w0(a)V∂E∂t+∂E∂a=ψ(a,t)IS−m1E−m(a)E∂I∂t+∂I∂a=m1E−γI−m(a)I+βσ(a)IV∂R∂t+∂R∂a=γI−m(a)R∂V∂t+∂V∂a=−(m(a)+w0(a)+βσ(a)I)VS(0,t)=S1,E(0,t)=I(0,t)=R(0,t)=0V(0,t)=∫am0v(a,t)S(a,t)daS(a,0)=S0(a),E(a,0)=E0(a),I(a,0)=I0(a)R(a,0)=R0(a)andV(a,0)=V0(a). | (4.1) |
v(a,t) is the control variable and our aim is to minimize the number of infected individuals and the cost of implementing control.
Theorem 4.1. Let (Sv1,Ev1,Iv1,Rv1,Vv1),(Sv2,Ev2,Iv2,Rv2,Vv2) be solutions of system 4.1 corresponding to the control variables v1 and v2, respectively. Then for sufficiently small T>0, the following estimates hold
∫Q(|Sv1−Sv2|+|Ev1−Ev2|+|Iv1−Iv2|+|Rv1−Rv2|+|Vv1−Vv2|)dadt≤C01∫Q(|v1−v2|)dadt, |
where C01 is a positive constant which depends on the bound of m,m1,γ,β,σ,ψ,ω0, and initial data. Moreover,
‖Sv1−Sv2‖L∞(Q)+‖Ev1−Ev2‖L∞(Q)+‖Iv1−Iv2‖L∞(Q)+‖Rv1−Rv2‖L∞(Q)+‖Vv1−Vv2‖L∞(Q)≤C02‖v1−v2‖L∞(Q), |
where C02 is a positive contant.
Proof. Using the method of characteristics, the system 4.1 can be solved as:
S(a,t)={−∫t0[m(τ+a−t)+v(τ+a−t)]S(τ+a−t,τ)dτ−∫t0ψ(τ+a−t)I(τ+a−t,τ)S(τ+a−t,τ)dτ+∫t0(Λ+ω0(τ+a−t)V(τ+a−t,τ)dτ+S0(a−t)ifa>t,−∫tt−a[m(τ+a−t)+v(τ+a−t)]S(τ+a−t,τ)dτ−∫t0ψ(τ+a−t)I(τ+a−t,τ)S(τ+a−t,τ)dτ+∫tt−a(Λ+ω0(τ+a−t)V(τ+a−t,τ)dτ+S1ifa<t | (4.2) |
E(a,t)={−∫t0[m1+m(τ+a−t)]E(τ+a−t,τ)dτ−∫t0ψ(τ+a−t)I(τ+a−t,τ)S(τ+a−t,τ)dτ+E0(a−t)ifa>t,−∫tt−a[m1+m(τ+a−t)]E(τ+a−t,τ)dτ−∫tt−aψ(τ+a−t)I(τ+a−t,τ)S(τ+a−t,τ)dτifa<t | (4.3) |
I(a,t)={−∫t0[γ+m(τ+a−t)]I(τ+a−t,τ)dτ+∫t0βσ(τ+a−t)I(τ+a−t,τ)V(τ+a−t,τ)dτ+∫t0m1E(τ+a−t,τ)dτ+I0(a−t)ifa>t,−∫tt−a[γ+m(τ+a−t)]I(τ+a−t,τ)dτ+∫tt−aβσ(τ+a−t)I(τ+a−t,τ)V(τ+a−t,τ)dτ+∫tt−am1E(τ+a−t,τ)dτifa<t | (4.4) |
R(a,t)={−∫t0m(τ+a−t)R(τ+a−t,τ)dτ+∫t0γI(τ+a−t,τ)dτ+R0(a−t)ifa>t,−∫tt−am(τ+a−t)R(τ+a−t,τ)dτ+∫tt−aγI(τ+a−t,τ)dτifa<t | (4.5) |
V(a,t)={−∫t0[m(τ+a−t)+ω0(τ+a−t)+βσ(τ+a−t)I(τ+a−t,τ)]V(τ+a−t,τ)dτ+V0(a−t)ifa>t,−∫tt−a[m(τ+a−t)+ω0(τ+a−t)+βσ(τ+a−t)I(τ+a−t,τ)]V(τ+a−t,τ)dτ+∫am0v(a)S(a,t−a)daifa<t. | (4.6) |
Let Q=(0,T)×(0,am), and assume that (Sv1,Ev1,Iv1,Rv1,Vv1),(Sv2,Ev2,Iv2,Rv2,Vv2) be solution of system (4.1) corresponding to the control variables v1 and v2 respectively. Then using the solution given by (4.2), we get
∫Q∩{a<t}|Sv1−Sv2|dadt≤C11∫Q(|v1−v2|+|Sv1−Sv2|)dadt+C22∫Q(|Sv1−Sv2|+|Iv1−Iv2|)dadt+C33∫Q|Vv1−Vv2|dadt, |
where C11,C22,C33 are constants which depends on the bound of m,ψ, and ω0. Similarly, we can consider the case when a>t, and we will obtain similar types of estimates. So, we can write
∫Q|Sv1−Sv2|dadt≤C11∫Q(|v1−v2|+|Sv1−Sv2|)dadt+C22∫Q(|Sv1−Sv2|+|Iv1−Iv2|)dadt+C33∫Q|Vv1−Vv2|dadt, |
Using the same procedure for other state variables, we get
∫Q(|Sv1−Sv2|+|Ev1−Ev2|+|Iv1−Iv2|+|Rv1−Rv2|+|Vv1−Vv2|)dadt≤C01∫Q(|v1−v2|)dadt, |
where C01 is a positive constant. Now, estimating the integral in the age variable only, we will get
‖Sv1−Sv2‖L∞(Q)+‖Ev1−Ev2‖L∞(Q)+‖Iv1−Iv2‖L∞(Q)+‖Rv1−Rv2‖L∞(Q)+‖Vv1−Vv2‖L∞(Q)≤C02‖v1−v2‖L∞(Q), |
where C02 is a positive constant that depends on model parameters and initial population distributions. More details about these types of estimates can be found in [31,32].
Let us define the following objective functional:
J(v)=∫T0∫am0(C1I(a,t)−C2S(a,t)+C3v(a,t)2)dadt. | (4.7) |
Here, C1,C2 and C3 are weight factors and our aim is to minimize J(v). We assume that the admissible control variable lies in the set V defined by
V={u:[0,am]×[0,T]↦[0,vm]}, | (4.8) |
where we assume that v∈L∞((0,am)×(0,T)) and 0≤v≤vm. Now, our next result establish the existence of optimal control.
Theorem 4.2. Let J:L1(0,T)→(−∞,∞] be defined by
J(v)={∫T0∫am0(C1I(a,t)−C2S(a,t)+C3v(a,t)2)dadtifv∈V∞otherwise. | (4.9) |
Then to the control problem (4.1), there exists an optimal control u∗ such that
minv∈VJ(v)=J(v∗). |
Proof. We have already shown the existence of unique solution to the system (4.1) which is uniformly bounded. Also control variable v is uniformly bounded in (0,am). Therefore, there exist a sequence vn∈V such that
limn→∞J(un)=lim infv∈VJ(v). |
Because the function C3v2 is a lower semi-continuous function which is also convex, we have
∫am0C3(v∗)2da≤lim infn→∞∫am0C3(vn)2da. | (4.10) |
Also, using the fact that the bound of S,E,I,R and V depends on the control variable v, we have Sn=S(vn),En=E(vn),In=I(vn),Rn=R(vn),Vn=V(vn). Because Sn,En,In,Rn and Vn are uniformly bounded for each n. We have S∗=S(v∗),E∗=E(v∗),I∗=I(v∗),R∗=R(v∗) and V∗=V(v∗). Now,
J(v∗)=∫T0∫am0[C1I∗−C2S∗+C3(v∗)2]dadt≤lim infn→∞∫T0∫am0[C1In−C2Sn+C3(vn)2]dadt=limn→∞∫T0∫am0[C1In−C2Sn+C3(vn)2]dadt=infv∈VJ(v). |
From the relation J(v∗)≤infv∈VJ(v), we conclude that v∗ is optimal control which minimizes the objective functional.
Now, our next task is to obtain the necessary conditions for our optimal control problem. Let us define vϵ(a,t)=v(a,t)+ϵg(a), where 0<ϵ<1,g∈L∞(0,am) and also called the variation function. Let us denote Sϵ=S(vϵ),Eϵ=E(vϵ),Iϵ=I(vϵ),Rϵ=R(vϵ) and Vϵ=V(vϵ). Then we have the following system
∂Sϵ∂t+∂Sϵ∂a=Λ−ψ(a,t)SϵIϵ−m(a)Sϵ−(v(a,t)+ϵg(a))Sϵ+w0(a)Vϵ∂Eϵ∂t+∂Eϵ∂a=ψ(a,t)SϵIϵ−m1Eϵ−m(a)Eϵ∂Iϵ∂t+∂Iϵ∂a=m1Eϵ−γIϵ−m(a)Iϵ+βσ(a)IϵVϵ∂Rϵ∂t+∂Rϵ∂a=γIϵ−m(a)Rϵ∂Vϵ∂t+∂Vϵ∂a=−(m(a)+w0(a)+βσ(a)Iϵ)VϵSϵ(0,t)=S1,Eϵ(0,t)=Iϵ(0,t)=Rϵ(0,t)=0Vϵ(0,t)=∫am0(v(a,t)+ϵg(a))S(a,t)daSϵ(a,0)=S0(a),Eϵ(a,0)=E0(a),Iϵ(a,0)=I0(a)Rϵ(a,0)=R0(a)andVϵ(a,0)=V0(a). | (4.11) |
Let us set the following notations for weak derivatives of S,E,I,R and V:
ˉS(a,t)=limϵ→0S(v+ϵg)−S(v)ϵ,ˉE(a,t)=limϵ→0E(v+ϵg)−E(v)ϵ,ˉI(a,t)=limϵ→0I(v+ϵg)−I(v)ϵ,ˉR(a,t)=limϵ→0R(v+ϵg)−R(v)ϵ,ˉV(a,t)=limϵ→0V(v+ϵg)−V(v)ϵ. Then we have the following system of differential equations
∂ˉS∂t+∂ˉS∂a=−ψ(a,t)(ˉSI+ˉIS)−m(a)ˉS−v(a,t)ˉS+w0(a)ˉV−g(a)S∂ˉE∂t+∂ˉE∂a=ψ(a,t)(ˉSI+ˉIS)−m1ˉE−m(a)ˉE∂ˉI∂t+∂ˉI∂a=m1ˉE−γˉI−m(a)ˉI+βσ(a)(ˉIV+IˉV)∂ˉR∂t+∂ˉR∂a=γˉI−m(a)ˉR∂ˉV∂t+∂ˉV∂a=−(m(a)+w0(a))ˉV−βσ(a)(ˉIV+I(a,t)ˉV)ˉS(0,t)=0,ˉE(0,t)=ˉI(0,t)=ˉR(0,t)=0ˉV(0,t)=∫am0(v(a,t)ˉS(a,t)+g(a)S(a,t))daˉS(a,0)=ˉE(a,0)=ˉI(a,0)=ˉR(a,0)=0andˉV(a,0)=0. | (4.12) |
The directional derivative of J with respect to v in the direction of g is given by
J′(v)=J(vϵ)−J(v)ϵ=∫T0∫am0[C1ˉI(a,t)−C2ˉS(a,t)+2C3v(a,t)g(a)]dadt. | (4.13) |
Now, our next task is to find the adjoint system. Let us define
⟨f,g⟩=∫T0∫∞0f(a,t)g(a,t)dadt. |
Observe that
0=⟨∂ˉS∂t+∂ˉS∂a+ψ(a,t)(ˉSI+ˉIS)+m(a)ˉS+v(a,t)ˉS−w0(a)ˉV+g(a)S,λ1(a,t)⟩0=⟨ˉS(a,t),−∂∂tλ1−∂∂aλ1+(m(a)+v(a,t))λ1+ψ(a,t)λ1I⟩+∫T0∫am0(ψ(a,t)ˉI(a,t)+g(a))S(a,t)λ1(a,t)dadt−∫T0∫am0w0(a)ˉV(a,t)λ1(a,t)dadt | (4.14) |
with the following conditions
λ1(t,am)=λ1(T,a)=0. |
Similarly we have
0=⟨∂ˉE∂t+∂ˉE∂a−ψ(a,t)(ˉSI−ˉIS)+m1ˉE+m(a)ˉE,λ2(a,t)⟩0=⟨ˉE(a,t),−∂∂tλ2−∂∂aλ2+(m1+m(a))λ2⟩−∫T0∫am0ψ(a,t)λ2(a,t)(S(a,t)ˉI(a,t)+I(a,t)ˉS(a,t))dadt | (4.15) |
0=⟨∂ˉI∂t+∂ˉI∂a−m1ˉE+γˉI+m(a)ˉI−βσ(a)(ˉIV−IˉV),λ3(a,t)⟩0=⟨ˉI(a,t),−∂∂tλ3−∂∂aλ3−m1λ3⟩−∫T0∫am0(γ+m(a)−βσ(a)V(a,t))ˉI(a,t)λ3(a,t)dadt−∫T0∫am0λ3(a,t)I(a,t)ˉI(a,t)dadt | (4.16) |
0=⟨∂ˉR∂t+∂ˉR∂a−γˉI+m(a)ˉR,λ4(a,t)⟩0=⟨ˉR(a,t),−∂∂tλ4−∂∂aλ4−m(a)λ4⟩−∫T0∫am0γλ4(a,t)ˉI(a,t)dadt | (4.17) |
0=⟨∂ˉV∂t+∂ˉV∂a+(m(a)+w0(a))ˉV+βσ(a)(ˉIV+IˉV),λ5(a,t)⟩0=⟨ˉV(a,t),−∂∂tλ5−∂∂aλ5+(m(a)+w0(a))λ5+βσ(a)Iλ5⟩+∫T0∫am0βσ(a)ˉI(a,t)λ5(a,t)dadt−∫T0∫am0(v(a,t)ˉS(a,t)+g(a)S(a,t))λ5(0,t)dadt | (4.18) |
Now, we get the following adjoint system
∂λ1∂t+∂λ1∂a=(m(a)+v(a,t))λ1+ψ(a,t)λ1I−ψ(a,t)λ2I−v(a,t)λ5(0,t)−C2∂λ2∂t+∂λ2∂a=(m1+m(a))λ2∂λ3∂t+∂λ3∂a=−m1λ3−(γ+m(a)−βσ(a)V−I)λ3−γλ4+βσ(a)λ5−ψ(a,t)λ2(a,t)S+C1∂λ4∂t+∂λ4∂a=−m(a)λ4∂λ5∂t+∂λ5∂a=(m(a)+w0(a)+βσ(a)I)λ5. | (4.19) |
Also, we have the following conditions
λ1(a,T)=λ2(a,T)=λ3(a,T)=λ4(a,T)=λ5(a,T)=0. |
λ1(am,t)=λ2(am,t)=λ3(am,t)=λ4(am,t)=λ5(am,t)=0. |
Using the same steps as we followed in 4.1, we can state the following theorem
Theorem 4.3. Let (λ11,λ21,λ31,λ41,λ51) and (λ12,λ22,λ32,λ42,λ52) be solution of adjoint system (4.19) with control variable v1 and v2 respectivey. Then
‖λ11−λ12‖L∞(Q)+‖λ21−λ22‖L∞(Q)+‖λ31−λ32‖L∞(Q)+‖λ41−λ42‖L∞(Q)+‖λ51−λ52‖L∞(Q)≤C11‖v1−v2‖L∞(Q), |
where C11 is a positive constant.
Now, we state and prove the following result, which gives the explicit form of optimal control.
Theorem 4.4. Let (S∗,E∗,I∗,R∗,V∗) and (λ1,λ2,λ3,λ4,λ5) be state solutions and solutions to corresponding adjoint system respectively. Also, let v∗∈V be optimal control which minimizes (4.7). Then optimal control will be of the form
v∗=max{0,min{vm,λ1(a,t)(w0(a)V∗(a,t)−ψ(a,t)I∗(a,t))2C3g(a))−(λ1(a,t)−λ5(0,t))S∗(a,t)2C3}}. |
Proof. We know that
J′(v)=∫T0∫am0[C1ˉI(a,t)−C2ˉS(a,t)+2C3v(a,t)g(a)]dadt. | (4.20) |
Now using the adjoint system, we have
J′(v)=∫T0∫am0ˉI(a,t)[∂λ3∂t+∂λ3∂a+m1λ3(a,t)+(γ+m(a)−βσ(a)V(a,t)−I(a,t))λ3(a,t)]datdt+∫T0∫am0ˉI(a,t)[γλ4(a,t)−βσ(a)λ5(a,t)+ψ(a,t)λ2(a,t)S(a,t)]dadt−∫T0∫am0ˉS(a,t)[−∂λ1∂t−∂λ1∂a]dadt+∫T0∫am0ˉS(a,t)[(m(a)+v(a,t))λ1(a,t)]dadt+∫T0∫am0ˉS(a,t)[ψ(a,t)λ1(a,t)I(a,t)−ψ(a,t)λ2(a,t)I(a,t)−v(a,t)λ5(0,t)]dadt−∫T0∫am0ˉE(a,t)[∂λ2∂t+∂λ2∂a−(m1+m(a))λ2(a,t)]dadt−∫T0∫am0ˉR(a,t)[∂λ4∂t+∂λ4∂a+m(a)λ4(a,t)]dadt−∫T0∫am0ˉV(a,t)[−∂λ5∂t−∂λ5∂a+(m(a)+w0(a)+βσ(a)I(a,t))λ5(a,t)]dadt |
Using (4.14, 4.15, 4.16, 4.17) and (4.18), we have
J′(v)=∫T0∫am02C3v(a,t)g(a)dadt+∫T0∫am0(λ1(a,t)−λ5(0,t))S∗(a,t)dadt−∫T0∫am0λ1(a,t)(w0(a)V∗(a,t)−ψ(a,t)I∗(a,t))dadt. | (4.21) |
Because J′(v)≥0, so this implies in this case
v(a,t)=λ1(a,t)(w0(a)V∗(a,t)−ψ(a,t)I∗(a,t))2C3g(a))−(λ1(a,t)−λ5(0,t))S∗(a,t)2C3 |
By using the upper and lower bounds of control variable, we have
v∗=max{0,min{vm,λ1(a,t)(w0(a)V∗(a,t)−ψ(a,t)I∗(a,t))2C3g(a))−(λ1(a,t)−λ5(0,t))S∗(a,t)2C3}}. |
Remark 4.5. It is clear from the characterization of optimal control that it depends on the susceptible, infected, and vaccinated class. Although it depends on function g, we can always fix a particular choice of g.
Theorem 4.6. Let M be a generic positive constant that depends on C02,C11 and the bound of vaccine vaning rate ω0, and transmission coefficient ψ. Then for sufficiently small M2C3, there exists a unique optimal controller v∗.
Proof. Let (Sv1,Ev1,Iv1,Rv1,Vv1),(Sv2,Ev2,Iv2,Rv2,Vv2) be solution of system (4.1) corresponding to the control variables v1 and v2 respectively, and (λ11,λ21,λ31,λ41,λ51),(λ12,λ22,λ32,λ42,λ52) be solution of adjoint system 4.19 with control variable v1 and v2 respectivey. Define the map L:U→U by
L(v)=max{0,min{vm,λ1(a,t)(w0(a)V(a,t)−ψ(a,t)I(a,t))2C3g(a))−(λ1(a,t)−λ5(0,t))S(a,t)2C3}}. |
Then
‖L(v1)−L(v2)‖≤‖λ11(a,t)(w0(a)Vv1(a,t)−ψ(a,t)Iv1(a,t))2C3g(a))−(λ11(a,t)−λ51(0,t))Sv1(a,t)2C3−λ12(a,t)(w0(a)Vv2(a,t)−ψ(a,t)Iv2(a,t))2C3g(a))+(λ12(a,t)−λ52(0,t))Sv2(a,t)2C3‖L∞(Q)≤12C3[ω0(a)g(a)‖λ11Vv1−λ12Vv2‖L∞(Q)+ψ(a,t)g(a)‖λ11Iv1−λ12Iv2‖L∞(Q)+‖λ11Sv1−λ12Sv2‖L∞(Q)+‖λ51(0,t)Sv1−λ52(0,t)Sv2‖L∞(Q)]≤M2C3[‖λ11Vv1−λ12Vv2‖L∞(Q)+‖λ11Iv1−λ12Iv2‖L∞(Q)+‖λ11Sv1−λ12Sv2‖L∞(Q)+‖λ51(0,t)Sv1−λ52(0,t)Sv2‖L∞(Q)], |
where M is a positive generic constant which depends on the bound of ω0,g and ψ. Therefore, we have
‖L(v1)−L(v2)‖≤M2C3‖v1−v2‖L∞(Q), |
where M is a positive generic constant which also depends on C01,C02 and T. So, if M2C3<1, then we have the existence of unique fixed point v∗.
In this section, we discuss numerical simulation results. We applied an explicit finite difference scheme to solve our model numerically. Since the explicit finite difference scheme is highly sensitive to the discretization size of independent variables, the discretization size is chosen in such a way that the scheme is convergent. A finite difference scheme is applied to solve the system of differential equations and for the adjoint system, we applied a backward finite difference scheme. {For the optimal control problem, we applied the forward-backward sweep method. The forward-backward sweep method for optimal control problems in PDEs combines the forward integration of the state equations with the backward integration of the adjoint equations. By iteratively adjusting the control variables based on the computed gradient, the method aims to find the optimal control that minimizes the cost functional while satisfying the given system of PDEs with initial and boundary data.} We use the following initial age distribution in our model:
S0(a)=exp(−0.09a)+0.7sin(0.05a2)E0(a)=0.0001exp(−a2)+0.05sin(0.05a2)I0(a)=0.0001exp(−a2)+0.02sin(0.05a2)R0(a)=0.0001exp(−0.5a)+0.01sin(0.05a2)V0(a)=0.0001exp(−0.5a)+0.001sin(0.05a2). |
Mortality rate is taken μ(a)=0.01a+0.0001,ψ(a,t)=0.02e−a. Other parameters taken are m1=0.2,q1=0.4,γ1=0.4,γ2=0.6,γ=0.02,β=1,Λ=0.9 and g=1.
For the first two plots Figures 2(a), (b), we assume that a fixed proportion of individuals are vaccinated and observe the dynamics of infected individuals. So, for these figures we do not use the optimal vaccination strategy.
From Figure 2(a), (b), it is clear that increasing the vaccination rate will reduce the proportion of infected individuals. It is clear that increasing the vaccination waning rate will increase the population density of individuals who again become susceptible to disease. In Figure 3(a), (b), we have taken vaccine waning rates as 0 and 0.3 respectively. So, even if we are vaccinating a population, still there will be an increase in the population density of susceptible individuals due to imperfect vaccines.
Figure 4(a), (b) and Figure 5 show the dynamics of control variable for vaccine waning rate 0.1 and 0.01. As time progresses, the profile of the control variable attains a stable behavior. Initially, the control will be high and as time progresses, the control will take its minimum value.
In Section 3, we already prove that if threshold parameter R0 is less than 1, then the steady state in which there are no individuals in the exposed or infected class is stable. In Figures 6 and 7, we have taken age between 10 and 50 and studied the stability of disease-free equilibrium point. We have chosen the parameters in such a way that the threshold number R0<1. These figures show that if threshold parameter R0<1, then the disease-free equilibrium point is stable. So, numerical simulations validate our theoretical results.
Age-structured modeling is important when studying imperfect vaccination because it allows a more realistic representation of how infectious diseases spread in a population. Imperfect vaccination refers to situations where not everyone in a population is fully protected by a vaccine due to vaccine effectiveness or a decline in immunity over time.
In this work, we proposed a new age-structured SEIRV model, where we incorporate vaccine waning rate and imperfect vaccination in our model. The aim was to study the effect of leaky vaccines and to investigate the optimal approach to contain the disease spread. The Well-posedness of the proposed model is shown using the semigroup of operators. We convert our model into an abstract Cauchy problem and then show that the operator in a homogeneous problem is the Hille-Yosida operator. Finally, the basic reproduction number is defined as a threshold parameter to study the stability analysis of the disease-free equilibrium point.
Vaccination strategy and optimal control problems with vaccination effort as a control variable are also discussed. The optimality conditions are derived for the optimal control problem. With the help of the adjoint system, optimal control is characterized. Numerical simulations are also added to establish theoretical results. An explicit finite difference scheme is used to solve the model numerically. The discretization size is chosen so that the scheme is convergent. The stability of disease-free steady state is shown using numerical simulation. Using the forward-backward sweep method, we plot the optimal control variable with respect to age and time variables.
Age-structured compartmental models are a valuable tool for understanding and analyzing the effects of imperfect vaccination. Although getting real data for verification is difficult, the model assumptions and outcomes are quite realistic. This study can help the public health policymakers optimally vaccinate a population and reduce the impact of the disease with a low cost of vaccination. The threshold number R0 involves some parameters, and by increasing or decreasing these parameters, accordingly, we can eradicate the infectious disease. By choosing different vaccine waning rates, the dynamics of individuals in different compartments can be studied using the numerical simulation results. We also encourage other researchers to explore this area. {Moreover, the age-structured SEIR model with stochastic perturbation can be investigated. More general size-structured variables can also be considered for epidemiological models. Finally, It is also natural to consider the delay in age and size-structured population models as it represents the maturation period. Therefore, age-structured compartmental models with delay can also be explored.
The authors declare they have not used Artificial Intelligence (AI) tools in the creation of this article.
The authors would like to thank the anonymous reviewers for their valuable comments and suggestions that helped improve this manuscript's quality.
A. Tridane is supported by UAEU UPAR grant number 12S125.
The authors declare there is no conflict of interest.
[1] |
S. J. Thomas, E. D. Moreira, N. Kitchin, J. Absalon, A. Gurtman, S. Lockhart, et al., Safety and efficacy of the BNT162b2 mRNA Covid-19 vaccine through 6 months, N. Engl. J. Med., 385 (2021), 1761–1773. https://doi.org/10.1056/NEJMoa2110345 doi: 10.1056/NEJMoa2110345
![]() |
[2] |
C. Menni, A. May, L. Polidori, P. Louca, J. Wolf, J. Capdevila, et al., COVID-19 vaccine waning and effectiveness and side-effects of boosters: A prospective community study from the ZOE COVID study, Lancet Infect. Dis., 22 (2022), 1002–1010. https://doi.org/10.1016/S1473-3099(22)00146-3 doi: 10.1016/S1473-3099(22)00146-3
![]() |
[3] | J. Scott, A. Richterman, M. Cevik, Covid-19 vaccination: evidence of waning immunity is overstated, BMJ, 374 (2021). https://doi.org/10.1136/bmj.n2320 |
[4] |
N. Andrews, J. Stowe, F. Kirsebom, F. Kirsebom, T. Rickeard, E. Gallagher, et al., Covid-19 vaccine effectiveness against the Omicron (B. 1.1. 529) variant, N. Engl. J. Med., 386 (2022), 1532–1546. https://doi.org/10.1056/NEJMoa2119451 doi: 10.1056/NEJMoa2119451
![]() |
[5] |
W. O. Kermack, A. G. Mckendrick, Contributions to the mathematical theory of epidemics, Proc. R. Soc. A, 115 (1927), 700–721. https://doi.org/10.1098/rspa.1927.0118 doi: 10.1098/rspa.1927.0118
![]() |
[6] |
W. O. Kermack, A. G. Mckendrick, Contributions to the mathematical theory of epidemics. II.—The problem of endemicity, Proc. R. Soc. A, 138 (1932), 55–83. https://doi.org/10.1098/rspa.1932.0171 doi: 10.1098/rspa.1932.0171
![]() |
[7] |
W. O. Kermack, A. G. Mckendrick, Contributions to the mathematical theory of epidemics III—The problem of endemicity, Proc. R. Soc. A, 141 (1933), 94–122. https://doi.org/10.1098/rspa.1933.0106 doi: 10.1098/rspa.1933.0106
![]() |
[8] |
Z. Zhang, S. Kundu, J. P. Tripathi, S. Bugalia, Stability and Hopf bifurcation analysis of an SVEIR epidemic model with vaccination and multiple time delays, Chaos Solitons Fractals, 131 (2020), 109483. https://doi.org/10.1016/j.chaos.2019.109483 doi: 10.1016/j.chaos.2019.109483
![]() |
[9] |
K. Hattaf, N. Yousfi, A. Tridane Mathematical analysis of a virus dynamics model with general incidence rate and cure rate, Nonlinear Anal. Real World Appl., 13 (2012), 1866–1872. https://doi.org/10.1016/j.nonrwa.2011.12.015 doi: 10.1016/j.nonrwa.2011.12.015
![]() |
[10] |
U. Kumar, P. S. Mandal, J. P. Tripathi, V. P. Bajiya, S. Bugalia, SIRS epidemiological model with ratio‐dependent incidence: Influence of preventive vaccination and treatment control strategies on disease dynamics, Math. Methods Appl. Sci., 44 (2021), 14703–14732. https://doi.org/10.1002/mma.7737 doi: 10.1002/mma.7737
![]() |
[11] |
S. Tyagi, S. C. Martha, S. Abbas, A. Debbouche, Mathematical modeling and analysis for controlling the spread of infectious diseases, Chaos Solitons Fractals, 144 (2021), 110707. https://doi.org/10.1016/j.chaos.2021.110707 doi: 10.1016/j.chaos.2021.110707
![]() |
[12] |
S. Tyagi, S. Gupta, S. Abbas, K. P. Das, B. Riadh, Analysis of infectious disease transmission and prediction through SEIQR epidemic model, Nonauton. Dyn. Syst., 8 (2021), 75–86. https://doi.org/10.1515/msds-2020-0126 doi: 10.1515/msds-2020-0126
![]() |
[13] | M. Iannelli, Mathematical Theory of Age-Structured Population Dynamics, Giardini editori e stampatori in Pisa, 1995. |
[14] |
H. R. Thieme, C. Castillo-Chavez, How may infection-age-dependent infectivity affect the dynamics of HIV/AIDS?, SIAM J. Appl. Math., 53 (1993), 1447–1479. https://doi.org/10.1137/0153068 doi: 10.1137/0153068
![]() |
[15] | F. Brauer, Age of infection in epidemiology models, Electron. J. Differ. Equations, 12 (2004), 29–37. |
[16] | G. F. Webb, Population models structured by age, size, and spatial position, in Structured Population Models in Biology and Epidemiology, Springer, (2008), 1–49. https://doi.org/10.1007/978-3-540-78273-5_1 |
[17] |
J. Arino, K. L. Cooke, P. Driessche, J. Velasco-Hernandez, An epidemiology model that includes a leaky vaccine with a general waning function, Discrete Contin. Dyn. Syst. Ser. B, 4 (2004), 479. https://doi.org/10.3934/dcdsb.2004.4.479 doi: 10.3934/dcdsb.2004.4.479
![]() |
[18] |
J. Mohammed-Awel, E. Numfor, R. Zhao, S. Lenhart, A new mathematical model studying imperfect vaccination: Optimal control analysis, J. Math. Anal. Appl., 500 (2021), 125132. https://doi.org/10.1016/j.jmaa.2021.125132 doi: 10.1016/j.jmaa.2021.125132
![]() |
[19] |
H. Tahir, A. Khan, A. Din, A. Khan, G. Zaman, Optimal control strategy for an age-structured SIR endemic model, Discrete Contin. Dyn. Syst. Ser. S, 14 (2021), 2535. https://doi.org/10.3934/dcdss.2021054 doi: 10.3934/dcdss.2021054
![]() |
[20] |
K. Li, H. Zhang, G. Zhu, M. Small, X. Fu, Suboptimal control and targeted constant control for semi-random epidemic networks, IEEE Trans. Syst. Man Cybernet., 51 (2019), 2602–2610. https://doi.org/10.1109/TSMC.2019.2916859 doi: 10.1109/TSMC.2019.2916859
![]() |
[21] |
W. Lv, Q. Ke, K. Li, Dynamical analysis and control strategies of an SIVS epidemic model with imperfect vaccination on scale-free networks, Nonlinear Dyn., 99 (2020), 1507–1523. https://doi.org/10.1007/s11071-019-05371-1 doi: 10.1007/s11071-019-05371-1
![]() |
[22] |
C. Xu, W. Zhang, C. Aouiti, Z. Liu, L. Yao, Bifurcation insight for a fractional‐order stage‐structured predator–prey system incorporating mixed time delays, Math. Methods Appl. Sci., 46 (2023), 9103–9118. https://doi.org/10.1002/mma.9041 doi: 10.1002/mma.9041
![]() |
[23] |
C. Xu, Z. Liu, P. Li, J. Yan, L. Yao, Bifurcation mechanism for fractional-order three-triangle multi-delayed neural networks, Neural Process. Lett., 2022 (2022), 1–27. https://doi.org/10.1007/s11063-022-11130-y doi: 10.1007/s11063-022-11130-y
![]() |
[24] | W. Ou, C. Xu, Q. Cui, Z. Liu, Y. Pang, M. Farman, S. Ahmad, A. Zeb, Mathematical study on bifurcation dynamics and control mechanism of tri‐neuron bidirectional associative memory neural networks including delay, Methods Appl. Sci., (2023). https://doi.org/10.1002/mma.9347 |
[25] |
C. Xu, M. ur Rahman, D. Baleanu, On fractional-order symmetric oscillator with offset-boosting control, Nonlinear Anal. Modell. Control, 27 (2022), 994–1008. https://doi.org/10.15388/namc.2022.27.28279 doi: 10.15388/namc.2022.27.28279
![]() |
[26] | M. Kumar, S. Abbas, Analysis of steady state solutions to an age structured SEQIR model with optimal vaccination, Math. Meth. Appl. Sci., 455 (2022), 1–18. |
[27] |
X. C. Duan, H. Cheng, M. Martcheva, S. Yuan, Dynamics of an Age Structured Heroin Transmission Model with Imperfect Vaccination, Internat. J. Bifur. Chaos, 31 (2021), 2150157. https://doi.org/10.1142/S0218127421501571 doi: 10.1142/S0218127421501571
![]() |
[28] |
M. Kumar, S. Abbas, Age-Structured SIR model for the spread of infectious diseases through indirect contacts, Mediterr. J. Math., 19 (2022), 1–18. https://doi.org/10.1007/s00009-021-01925-z doi: 10.1007/s00009-021-01925-z
![]() |
[29] |
S. Bentout, A. Tridane, S. Djilali, T. M. Touaoula, Age-structured modeling of COVID-19 epidemic in the USA, UAE and Algeria, Alexandria Eng. J., 60 (2021), 401–411. https://doi.org/10.1016/j.aej.2020.08.053 doi: 10.1016/j.aej.2020.08.053
![]() |
[30] |
M. Kumar, S. Abbas, Global dynamics of an age-structured model for HIV viral dynamics with latently infected T cells, Math. Comput. Simul., 198 (2022), 237–252. https://doi.org/10.1016/j.matcom.2022.02.035 doi: 10.1016/j.matcom.2022.02.035
![]() |
[31] |
K. R. Fister, S. Lenhart, Optimal control of a competitive system with age-structure, J. Math. Anal. Appl., 291 (2004), 526–537. https://doi.org/10.1016/j.jmaa.2003.11.031 doi: 10.1016/j.jmaa.2003.11.031
![]() |
[32] | K. R. Fister, H. Gaff, S. Lenhart, E. Numfor, E. Schaefer, J. Wang, Optimal control of vaccination in an age-structured cholera model, in Mathematical and Statistical Modeling for Emerging and Re-emerging Infectious Diseases, (2016), 221–248. https://doi.org/10.1007/978-3-319-40413-4_14 |
[33] | A. Pazy, Semigroups of Linear Operators and Applications to Partial Differential Equations, Springer-Verlag, New York, 2012. |
1. | Yacouba Yoda, Harouna Ouedraogo, Dramane Ouedraogo, Aboudramane Guiro, Mathematical analysis and optimal control of Dengue fever epidemic model, 2024, 2024, 2731-4235, 10.1186/s13662-024-03805-8 | |
2. | Shuping Li, Yuru Yuan, Dynamics of two-strain epidemic model with imperfect vaccination on complex networks, 2024, 70, 1598-5865, 1859, 10.1007/s12190-024-02025-3 | |
3. | Shraddha Salwahan, Syed Abbas, Abdessamad Tridane, Optimal control of a periodically switched epidemic model, 2025, 13, 2195-268X, 10.1007/s40435-025-01600-1 |