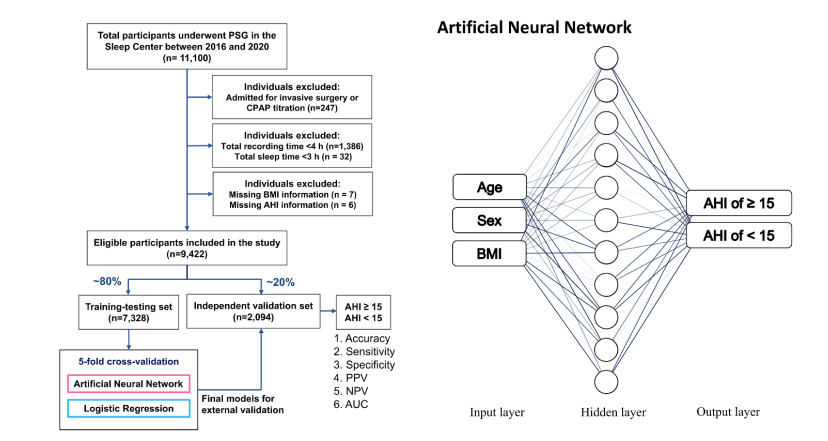
Citation: Henri Berestycki, Guillemette Chapuisat. Traveling fronts guided by the environment for reaction-diffusion equations[J]. Networks and Heterogeneous Media, 2013, 8(1): 79-114. doi: 10.3934/nhm.2013.8.79
[1] | Abdulwahab Ali Almazroi . Survival prediction among heart patients using machine learning techniques. Mathematical Biosciences and Engineering, 2022, 19(1): 134-145. doi: 10.3934/mbe.2022007 |
[2] | Weidong Gao, Yibin Xu, Shengshu Li, Yujun Fu, Dongyang Zheng, Yingjia She . Obstructive sleep apnea syndrome detection based on ballistocardiogram via machine learning approach. Mathematical Biosciences and Engineering, 2019, 16(5): 5672-5686. doi: 10.3934/mbe.2019282 |
[3] | Lili Jiang, Sirong Chen, Yuanhui Wu, Da Zhou, Lihua Duan . Prediction of coronary heart disease in gout patients using machine learning models. Mathematical Biosciences and Engineering, 2023, 20(3): 4574-4591. doi: 10.3934/mbe.2023212 |
[4] | Jingchi Jiang, Xuehui Yu, Yi Lin, Yi Guan . PercolationDF: A percolation-based medical diagnosis framework. Mathematical Biosciences and Engineering, 2022, 19(6): 5832-5849. doi: 10.3934/mbe.2022273 |
[5] | Abigail Ferreira, Rui Lapa, Nuno Vale . A retrospective study comparing creatinine clearance estimation using different equations on a population-based cohort. Mathematical Biosciences and Engineering, 2021, 18(5): 5680-5691. doi: 10.3934/mbe.2021287 |
[6] | Atsushi Kawaguchi . Network-based diagnostic probability estimation from resting-state functional magnetic resonance imaging. Mathematical Biosciences and Engineering, 2023, 20(10): 17702-17725. doi: 10.3934/mbe.2023787 |
[7] | Michele L. Joyner, Cammey Cole Manning, Whitney Forbes, Valerie Bobola, William Frazier . Modeling Ertapenem: the impact of body mass index on distribution of the antibiotic in the body. Mathematical Biosciences and Engineering, 2019, 16(2): 713-726. doi: 10.3934/mbe.2019034 |
[8] | Wenzhu Song, Lixia Qiu, Jianbo Qing, Wenqiang Zhi, Zhijian Zha, Xueli Hu, Zhiqi Qin, Hao Gong, Yafeng Li . Using Bayesian network model with MMHC algorithm to detect risk factors for stroke. Mathematical Biosciences and Engineering, 2022, 19(12): 13660-13674. doi: 10.3934/mbe.2022637 |
[9] | Anastasia-Maria Leventi-Peetz, Kai Weber . Probabilistic machine learning for breast cancer classification. Mathematical Biosciences and Engineering, 2023, 20(1): 624-655. doi: 10.3934/mbe.2023029 |
[10] | Tingxi Wen, Hanxiao Wu, Yu Du, Chuanbo Huang . Faster R-CNN with improved anchor box for cell recognition. Mathematical Biosciences and Engineering, 2020, 17(6): 7772-7786. doi: 10.3934/mbe.2020395 |
Obstructive sleep apnea (OSA) is featured by repeated upper airway narrowing or obstruction, causing a substantial reduction or cessation of airflow during sleep [1,2]. These disruptions in breathing lead to intermittent hypercapnia and hypoxemia, causing metabolic dysregulation, endothelial dysfunction, and systemic inflammatory responses [2,3]. These pathophysiologic changes have contributed to various major comorbidities [2,3,4,5]. OSA is a global health concern and economic burden, with estimates of the incidence of moderate or severe OSA reaching 6%-17% in the general adult population and up to 49% in the elderly population [4,6], with most of the cases being undiagnosed [7].
No single physical examination finding can be used to diagnose or exclusively account for OSA. The gold standard diagnostic test for OSA relies on in-lab all-night polysomnography (PSG) [7,8]. However, this method is costly, and patients require continuous sleep monitoring by medical staff [2]; thus, limited availability of PSG may cause delays in diagnosing OSA and an increased disease burden [7,9].
Several body measurements predispose patients to OSA. Body weight, body mass index (BMI), central body fat distribution, and large neck and waist circumferences are possible risk factors [2,6,10]. In addition, increased age and males are also at risk for OSA [2,6]. Despite the epidemiological reports demonstrating that age, sex, and BMI are associated with OSA severity, an integrated predicting model that uses only these three factors to assess OSA risk has not yet been developed.
Machine learning approaches have expanded the ability to design efficient predictive and diagnostic tools and have gained popularity in many areas of medical research and clinical applications [11,12]. Logistic regression (LR) is one of the computational methods for probing multivariate data to detect the independent effects of a variable after adjusting for the contributions of the others [13]. An artificial neural network (ANN) is a supervised learning algorithm with advantages in analyzing nonlinear data, enhancing data interpretation, and designing effective predictive and diagnostic models to assist different clinical tasks [11,14].
The present study investigated the clinical predictive validity of age, sex, and BMI for sleep apnea and applied LR and ANN algorithms to develop a risk-predicting model using these three parameters as predictors.
This retrospective observational study used clinical data from the medical records of participants who had undergone PSG at the Sleep Center of Shuang Ho Hospital, Taipei Medical University, Taiwan, between January 2015 and January 2020.
The inclusion criteria for this study were: (1) participants aged≥18 years and (2) who had undergone a full-night PSG. Participants were excluded if (1) they had received any OSA treatments or been admitted to the hospital for any invasive surgery for OSA, (2) they had undergone PSG for continuous positive airway pressure (CPAP) titration, (3) the total recording time was < 4 h [8], and (4) the total sleep time was < 3 h [15].
Before the PSG examination, a baseline screening questionnaire was used to assess each participant's basic information, medication, and surgical history. The participants were measured for height, weight, and BMI (kg/m2) at the time of registration.
All the participants underwent a full-night PSG recorded by qualified technicians. All the sleep signals were obtained using an Embla N7000 Recording System (Medcare Flaga, Reykjavik, Iceland) and Sandman Elite sleep diagnostic software (Natus Neurology Inc., Middleton, WI, USA).
Experienced sleep technologists scored all sleep apnea and hypopnea events following the American Academy of Sleep Medicine 2012 updated guideline [8,16]. Each participant's apnea-hypopnea index (AHI) was calculated according to the total number of apnea and hypopnea events divided by the total sleeping time. Hypopnea was defined as a 30-89% reduction in nasal airflow for at least 10s, with arousal or at least a decrease of 3% oxygen saturation. Apnea was defined as a more than 90% reduction in oral airflow for at least 10s with or without arousal or decreased saturation.
The severity of OSA was classified as none (AHI < 5), mild (AHI = 5-15), moderate (AHI = 15-30), and severe (AHI ≥ 30) [2,8]. To develop the predictive models for the risk of moderate-to-severe OSA, we divided the participants into none-and-mild (AHI < 15) and moderate-to-severe (AHI ≥ 15) OSA groups for further analysis.
Taipei Medical University-Joint Institutional Review Board (TMU-JIRB: N201911007) approved the protocol of this study. Waivers of informed consent were approved by the TMU-JIRB (TMU-JIRB: N201911007) for this retrospective study involving the secondary analysis of existing data.
All analyses were performed using STATISTICA software (TIBCO Software, Tulsa, OK, USA). Continuous data are presented as mean and standard deviation, whereas categorical variables are expressed as frequencies and percentages. We used Fisher's exact test to determine associations between two categorical variables and Pearson's chi-squared (X2) test to determine the statistical significance between the means of two continuous variables in the participants with none-to-mild and moderate-to-severe OSA. The one-way analysis of variance (ANOVA) was used to determine the statistical significance between the means of ≥ 3 independent groups. A two-tailed p-value less than 0.05 was considered statistically significant.
All the LR and ANN models were established and modeled using STATISTICA software. In the models, age and BMI were set as continuous variables, and sex was designated as a categorical variable. For our ANNs, the employed architecture was feed-forward, combined with a back-propagation algorithm with multilayer perceptron (MLP). A bias term was used for each neural layer in the MLP. We set the number of neurons in the hidden layer from 1 to 50. We initially partitioned the eligible PSG data into a training-testing set and an independent validation set, containing ~80% and ~20% of the data, respectively. The data from the training-testing set were used to determine the generalizability of the LR and ANN analyses using five-fold cross-validation (Figure 1A). All participants were stratified by age, sex, BMI, and OSA severity to maintain consistent proportions of demographic data across the training-testing and validation sets. We reported the mean AUCs for the training and testing sets of the LR and ANN models through five-fold cross-validation. The model with the best AUC in the five testing sets was identified to have the best predictive performance and used as the final model. The final models were then applied to the independent validation set, and we calculated the accuracy, sensitivity, specificity, positive predictive value (PPV), negative predictive value (NPV), and AUCs of the validation set derived from the confusion matrix [17] (Figure 1A). The classification threshold was set to 0.5 for all models.
During the study period, 11, 100 participants underwent PSG in the Sleep Center. Of these, 1, 665 participants were excluded from the baseline analyses for the following reasons: they had been admitted to the hospital for invasive surgery or CPAP titration (n = 247), the total recording time < 4h (n = 1, 386), the total sleep time < 3h (n = 32), missing BMI information (n = 7) or missing AHI information (n = 6) (Figure 1A). Overall, 9, 422 participants (2918 women and 6504 men, mean age: 49.2 ± 14.0 years) who had undergone PSG were enrolled in this study. Of these, 5, 920 (62.8%) had an AHI of ≥15, indicating moderate-to-severe OSA, and 3, 502 (37.2%) had an AHI < 15, indicating none-to-mild OSA.
A higher proportion of men have moderate-to-severe OSA than women (Table 1). Compared with participants with none-to-mild OSA, participants with moderate-to-severe OSA were older and had a higher BMI. In addition, participants with moderate-to-severe OSA had a shorter total sleep time, lower sleep efficiency, shorter rapid eye movement duration, and lower oxygen saturation during the PSG recording than those with none-to-mild OSA (Table 1).
Variables | Moderate-to-severe OSA (AHI ≥ 15) |
None-to-mild OSA (AHI < 15) |
p-value |
n | 5, 920 | 3, 502 | |
Age | 50.8 ± 13.5 | 46.6 ± 14.4 | < 0.0001 |
Female, n (%) | 1266 (21.4) | 1652 (47.2) | < 0.0001 |
Height (cm) | 167.2 ± 8.5 | 164.4 ± 8.9 | < 0.0001 |
Weight (kg) | 79.7±16.1 | 66.1 ± 13.1 | < 0.0001 |
BMI (kg/m2) | 28.4 ± 4.8 | 24.3 ± 3.8 | < 0.0001 |
Polysomnography | |||
Total recording time (min) | 366.6±11.9 | 367.8±13.4 | < 0.0001 |
Total sleep time (min) | 275.8±60.2 | 281.4±61.1 | < 0.0001 |
Sleep efficiency (%) | 75.2±16.4 | 76.5±16.5 | 0.0002 |
Non-REM sleep (min) | 240.6±51.7 | 242.2±51.3 | 0.133 |
REM sleep (min) | 35.2±22.5 | 39.1±23.7 | < 0.0001 |
AHI | 44.7±23.8 | 6.4±4.5 | < 0.0001 |
SO2 mean (%) | 94.5±2.6 | 96.6±1.3 | < 0.0001 |
Data are expressed as mean ± standard deviation. The p-value represents the comparison between the AHI ≥ 15 and AHI < 15 groups. |
A multivariate analysis was applied to adjust for age, sex, and BMI to determine the independent predictors of moderate-to-severe OSA. Table 2 presents the variables' crude and adjusted odds ratio (OR) values in the LR model. After adjustment for the associated variables, the regression analysis revealed that the female sex was associated with a 0.28-fold reduced risk of moderate-to-severe OSA (95% CI: 0.25-0.32; p < 0.0001), and every unit increase in age or BMI was associated with a 1.04-fold (95%CI: 1.04-1.05; p < 0.0001) or 1.27-fold (95%CI: 1.26-1.29; p < 0.0001) increase in the likelihood of moderate-to-severe OSA, respectively.
Unadjusted Model | Adjusted Model | |||
Variables | OR | 95% CI | OR | 95% CI |
Age | 1.02*† | 1.02-1.03 | 1.04*† | 1.04-1.05 |
Female | 0.30* | 0.28-0.33 | 0.28* | 0.25-0.32 |
BMI | 1.27*† | 1.25-1.28 | 1.27*† | 1.26-1.29 |
The odds ratio (OR) and 95% confidence intervals (CI) represent the association between the variables and the presence of moderate-to-severe OSA (AHI ≥ 15). *p < 0.0001; †Odds ratio of per unit changes. |
As mentioned, age, sex, and BMI were used as input parameters for developing the LR and ANN models for predicting moderate-to-severe OSA. As shown in Figure 1A, the 7328 participants in the training-testing group were randomly partitioned into training and testing sets for five-fold cross-validation. Table 3 showed that age, sex, BMI, and OSA severity distribution remained identical across the five testing sets and the independent validation sets.
Variable | Testing set 1 | Testing set 2 | Testing set 3 | Testing set 4 | Testing set 5 | Independent validation set | p-value |
n | 1466 | 1465 | 1466 | 1465 | 1466 | 2094 | |
AHI≥15, n (%) | 920 (62.8) | 919 (62.7) | 920 (62.8) | 919 (62.7) | 920 (62.8) | 1322 (63.1) | 1.000 |
Age | 49.0 ± 0.4 | 49.0 ± 0.4 | 49.9 ± 0.4 | 49.1 ± 0.4 | 49.0 ± 0.4 | 49.3 ± 0.3 | 0.503 |
Female, n (%) | 483 (33.0) | 438 (29.9) | 472 (32.2) | 445 (30.4) | 431 (29.4) | 649 (31.0) | 0.266 |
BMI (kg/m2) | 26.9 ± 4.9 | 26.9 ± 4.9 | 26.9 ± 5.0 | 26.9 ± 5.0 | 26.8 ± 4.8 | 26.9 ± 4.9 | 0.973 |
The p-value represents the multiple comparisons across the five testing and the independent validation sets. |
Following adequate training, the five testing sets that achieved the best prediction performance after five-fold cross-validation were ANN models with 3, 14, 25, 27, and 29 hidden neurons, respectively. The mean AUCs of the training sets were 0.808 ± 0.004 (Figure 2A) and 0.808 ± 0.015 for the testing sets (Figure 2B). Among the generated ANN models, the model containing 25 hidden neurons obtained the highest testing AUC and was selected as the final model. After being applied to the independent validation set, the ANN-based model achieved the accuracy, sensitivity, specificity, PPV, and NPV of 76.4%, 87.7%, 56.9%, 77.7%, 73.0%, with the AUC of 0.807, respectively. Results of the LR model revealed a mean AUC of 0.802 ± 0.004 for the training sets (Figure 2C) and 0.801 ± 0.015 for the testing sets (Figure 2D). After being validated to the independent validation set, the accuracy, sensitivity, specificity, PPV, and NPV were 76.3%, 87.5%, 57.0%, 77.7%, and 72. 7% with an AUC of 0.806 for the LR-based model. Figure 3 shows the ROC curve and AUC of the final ANN and LR models applied to the independent validation set.
The present study validated age, sex, and BMI as parameters for use in LR- and ANN models to estimate the risk of OSA in participants who had undergone PSG. The established LR- and ANN-based model integrating the three parameters achieved a notable prediction performance with an AUC of 0.806 and 0.807 for predicting moderate-to-severe OSA in the validation set. The relatively high AUC values indicated that the models could distinguish participants between groups of interest, namely, participants with none-to-mild and those with moderate-to-severe OSA. Patients with OSA are prone to various complications and require interventions [2,7]; our models may contribute to further understanding these three variables' fundamental role in assessing OSA risk.
OSA has been recognized as a global health and economic burden, and early identification and diagnosis of OSA is a crucial issue in preventive medicine [4,6,7]. Since standard diagnostic tests for OSA rely on expensive in-laboratory PSG, which can be challenging to schedule in high-demand situations, identifying crucial factors and developing a simple and reliable tool to estimate the risk of OSA is clinically relevant [7,9].
Anatomical and physiological differences in the oropharynx, larynx and upper respiratory tract, changes in respiratory physiology associated with different sleep structures, and differences in the distribution of adipose tissue have been suggested as reasons for sex differences in developing OSA [18]. Deterioration of the collapsibility of the pharyngeal airway and the sensitivity of the respiratory control system may contribute to the pathogenesis of OSA with aging [2,18]. Hormonal changes in menopausal women have been linked to an increased risk of OSA and a decreased sex difference in OSA with age [19,20]. The prevalence of OSA has been reported to increase 1.4 to 3.2-fold with each 10-year increment in age, and men had a 1.7 to 3.0-fold higher odds of developing OSA than women [2,6]. These findings support the rationale for using age and sex as parameters in a generic prediction model to predict OSA risk.
Obesity is a well-recognized risk factor for developing OSA, and a higher BMI is associated with greater severity of OSA for both sexes [19,21]. As already mentioned, anthropometric profiles, such as body weight, BMI, central body fat distribution, and neck and waist circumference, are predisposed to the risk of OSA [2,6,10]. In patients with OSA, occlusion of the nasal and oro-pharynx may occur during sleep when the tongue and epiglottis move backward and lean together. Individuals with obesity have excess soft tissue around the collapsible pharynx, making the narrow airway more prone to collapse [2,3,21]. In line with the findings of other studies, we observed that the participants with moderate-to-severe OSA had a higher BMI. Although other anthropometric profiles have been associated with OSA, neck and waist circumference were considered surrogates for BMI [4,21,22]. The study by Huxley et al. has also suggested that "measures of central obesity were superior to BMI as discriminators of cardiovascular risk factors, although the differences were small and unlikely to be of clinical relevance" [23]. Furthermore, the same study demonstrated that "combining BMI with any measure of central obesity did not improve the discriminatory capability of the individual measures" [23]. In this study, we proposed BMI as a simple, well-known, and readily available predictor of OSA for the prediction models.
Despite the epidemiological reports indicating that age, sex, and BMI are associated with OSA, an integrated predicting model using only these three attributes to assess OSA risk has not yet been developed. Advances in machine learning techniques have improved the ability of researchers and clinical applications to analyze massive amounts of data and develop appropriate diagnostic and predictive methods [24,25,26,27]. Several studies have applied machine learning algorithms to predict OSA risk. However, most of these studies have incorporated more complex parameters, such as sleep questionnaires [28,29,30], at-home PSG [15], cephalometric images [31], neck and waist circumference measurements [28,29,32,33], and facial-cervical measurements [28,32], into the predictive models.
In this context, our study used a large hospital-based sample of adults, allowing accurate comparison between none-to-mild and moderate-to-severe cases. The generated LR- and ANN-boosted models exhibited relatively high AUCs with adequate accuracy, sensitivity, PPV, and NPV, revealing the ability of these models to distinguish between the groups of interest, namely individuals with moderate-to-severe and none-to-mild OSA. Furthermore, our models used simple and easy-to-obtain parameters (age, sex, and BMI), which may provide a direction for developing predictive models under conditions of limited and constrained information.
Both LR and ANN are widely used models in biomedicine. This study's LR and ANN models achieved similar predictive validity in predicting OSA in patients undergoing PSG, which may be related to our data structure. ANN has the advantage of analyzing complex, multidimensional and nonlinear variables [34,35,36,37]. When the predictor-outcome relationships in the dataset are relatively simple and linear, LR using linear combinations of variables may achieve performance that approximates that of ANN [36,38]. This consideration was contextualized in the conclusion by Dreiseitl and Ohno-Machado [38] that "there is no single algorithm that performs better than all other algorithms on any given data set and application area." and also summarized by Sargent [34] that "both methods should continue to be used and explored in a complementary manner."
The present study has several limitations. First, in our cohort, the incidence of moderate-to-severe OSA was 62.8%, which is higher than the reported prevalence of OSA in the general population [4,19,20,39]. In clinical practice, patients referred for a PSG exam are usually expected to have OSA or another sleep disorder; therefore, the participant population in this study cannot be representative of the general population with a very low prevalence of OSA. Second, the data set used in this study was limited to a single center and included only Asian ethnic groups. Therefore, the trained model may not be generalizable beyond the institution or other ethnic groups [28,29]. Third, this retrospective observational study did not exclude undiagnosed medical conditions associated with OSA, such as neurological, cardiovascular, and pulmonary disorders, which may affect the model's predictive performance. Our model did not contain detailed anthropometric imaging or measurements, which may limit the model's ability to distinguish the disease-specific causes of OSA. Finally, the relatively low specificity for the models (57.0% and 56.9%) suggests a higher rate of false positives, meaning that a healthy person is more likely to receive an incorrect diagnosis of OSA using the proposed tool. As Jonas et al. [30] concluded, "There is uncertainty about the accuracy or clinical utility of all potential screening tools." a PSG to verify OSA severity and confirm the diagnosis is warranted for patients with clinically suspected sleep apnea.
Our study validated the diagnostic relevance of age, sex, and BMI for OSA. The generated LR- and ANN-based model effectively predicted OSA risk using these three simple parameters. Our results, therefore, may improve insights into OSA-related essential risk factors and provide knowledge to facilitate individualized risk stratification and formulate diagnostic decisions for sleep apnea.
This work was funded by the National Science and Technology Council (NSTC 111-2314-B-038-132-MY3) to CC Chung.
The authors declare there is no conflict of interest.
[1] | Matthieu Alfaro, Jérôme Coville and Gaël Raoul, Travelling waves in a nonlocal reaction-diffusion equation as a model for a population structured by a space variable and a phenotypical trait, arXiv:1211.3228, (2013). |
[2] |
Tobias Back, Jochen G. Hirsch, Kristina Szabo and Achim Gass, Failure to demonstrate peri-infarct depolarizations by repetitive mr diffusion imaging in acute human stroke, Stroke, 31 (2000), 2901-2906. doi: 10.1161/01.STR.31.12.2901
![]() |
[3] | H. Berestycki and P.-L. Lions, Une méthode locale pour l'existence de solutions positives de problèmes semi-linéaires elliptiques dans $R^N$, J. Analyse Math., 38 (1980), 144-187. |
[4] |
H. Berestycki and L. Nirenberg, On the method of moving planes and the sliding method, Bol. Soc. Brasil. Mat. (N.S.), 22 (1991), 1-37. doi: 10.1007/BF01244896
![]() |
[5] |
Henri Berestycki, Le nombre de solutions de certains problèmes semi-linéaires elliptiques, J. Funct. Anal., 40 (1981), 1-29. doi: 10.1016/0022-1236(81)90069-0
![]() |
[6] | Henri Berestycki and Guillemette Chapuisat, A numerical study of a non local reaction-diffusion equation in population dynamics, In preparation., (2013). |
[7] | Henri Berestycki and Guillemette Chapuisat, Propagation in a non homogeneous kpp equation arising in cancer modeling, In preparation, (2013). |
[8] |
Henri Berestycki, Françcois Hamel and Hiroshi Matano, Bistable traveling waves passing an obstacle, Comm. Pures and Appl. Math., 62 (2009), 729-788. doi: 10.1002/cpa.20275
![]() |
[9] |
Henri Berestycki and François Hamel, Fronts and invasions in general domains, C. R. Math. Acad. Sci. Paris, 343 (2006), 711-716. doi: 10.1016/j.crma.2006.09.036
![]() |
[10] |
Henri Berestycki and François Hamel, Generalized travelling waves for reaction-diffusion equations, in "Perspectives in Nonlinear Partial Differential Equations", 446 of Contemp. Math., 101-123. Amer. Math. Soc., Providence, RI, (2007). doi: 10.1090/conm/446
![]() |
[11] |
Henri Berestycki and François Hamel, Generalized transition waves and their properties, Comm. Pure Appl. Math., to appear, (2012). doi: 10.1002/cpa.21389
![]() |
[12] |
Henri Berestycki and Pierre-Louis Lions, Some applications of the method of super and subsolutions, in "Bifurcation and Nonlinear Eigenvalue Problems (Proc., Session, Univ. Paris XIII, Villetaneuse, 1978)", 782 of Lecture Notes in Math., 16-41. Springer, Berlin, (1980). doi: 10.1007/BFb0090426
![]() |
[13] |
Henri Berestycki, Grégoire Nadin, Benoit Perthame and Lenya Ryzhik, The non-local Fisher-KPP equation: Travelling waves and steady states, Nonlinearity, 22 (2009), 2813-2844. doi: 10.1088/0951-7715/22/12/002
![]() |
[14] | Henri Berestycki and Louis Nirenberg, Travelling fronts in cylinders, Ann. Inst. H. Poincaré Anal. Non Linéaire, 9 (1992), 497-572. |
[15] |
Henri Berestycki and Luca Rossi, On the principal eigenvalue of elliptic operators in $\mathbbR^N$ and applications, J. Eur. Math. Soc. (JEMS), 8 (2006), 195-215. doi: 10.4171/JEMS/47
![]() |
[16] |
Henri Berestycki and Luca Rossi, Reaction-diffusion equations for population dynamics with forced speed. I. The case of the whole space, Discrete Contin. Dyn. Syst., 21 (2008), 41-67. doi: 10.3934/dcds.2008.21.41
![]() |
[17] |
Guillemette Chapuisat, Existence and nonexistence of curved front solution of a biological equation, J. Differential Equations, 236 (2007), 237-279. doi: 10.1016/j.jde.2007.01.021
![]() |
[18] |
Guillemette Chapuisat and Emmanuel Grenier, Existence and nonexistence of traveling wave solutions for a bistable reaction-diffusion equation in an infinite cylinder whose diameter is suddenly increased, Comm. Partial Differential Equations, 30 (2005), 1805-1816. doi: 10.1080/03605300500300006
![]() |
[19] |
Guillemette Chapuisat, Emmanuel Grenier, Marie-Aim\'ee Dronne, Marc Hommel and Jean-Pierre Boissel, A global model of ischemic stroke with stress on spreading depression, Progress in Biophysics and Molecular Biology, 97 (2008), 4-27. doi: 10.1016/j.pbiomolbio.2007.10.004
![]() |
[20] |
Guillemette Chapuisat and Romain Joly, Asymptotic profiles for a traveling front solution of a biological equation, Math. Models Methods Appl. Sci., 21 (2011), 2155-2177. doi: 10.1142/S0218202511005696
![]() |
[21] | Jacques De Keyser, Geert Sulter and Paul G. Luiten, Clinical trials with neuroprotective drugs in acute ischaemic stroke: are we doing the right thing?, Trends Neurosci., 22 (1999), 535-540. |
[22] | Laurent Desvillettes, Régis Ferrières and Céline Prevost, Infinite dimensional reaction-diffusion for population dynamics, Prépublication du CMLA No. 2003-04, (2003). |
[23] | Lawrence C. Evans, "Partial Differential Equations," 19 of Graduate Studies in Mathematics, American Mathematical Society, Providence, RI, 1998. |
[24] |
Laurent Excoffier, Matthieu Foll and Rémy J Petit, Genetic consequences of range expansions, Annual Review of Ecology Evolution and Systematics, 40 (2009), 481-501. doi: 10.1146/annurev.ecolsys.39.110707.173414
![]() |
[25] |
Ido Filin, Robert D. Holt and Michael Barfield, The relation of density regulation to habitat specialization, evolution of a species' range, and the dynamics of biological invasions, Am. Nat., 172 (2008), 233-247. doi: 10.1086/589459
![]() |
[26] |
Ali Gorji, Dieter Scheller, Heidrun Straub, Frank Tegtmeier, Rüdiger Köhling, Jörg Michael Höhling, Ingrid Tuxhorn, Alois Ebner, Peter Wolf, Hans Werner Panneck, Falk Oppel and Erwin Josef Speckmann, Spreading depression in human neocortical slices, Brain Res., 906 (2001), 74-83. doi: 10.1016/S0006-8993(01)02557-4
![]() |
[27] |
Oskar Hallatschek, Pascal Hersen, Sharad Ramanathan and David R. Nelson, Genetic drift at expanding frontiers promotes gene segregation, Proceedings of the National Academy of Sciences, 104 (2007), 19926-19930. doi: 10.1073/pnas.0710150104
![]() |
[28] |
Oskar Hallatschek and David R. Nelson, Gene surfing in expanding populations, Theoretical Population Biology, 73 (2008), 158-170. doi: 10.1016/j.tpb.2007.08.008
![]() |
[29] |
Oskar Hallatschek and David R. Nelson, Life at the front of an expanding population, Evolution, 64 (2010), 193-206. doi: 10.1111/j.1558-5646.2009.00809.x
![]() |
[30] |
Robert D. Holt, Michael Barfield, Ido Filin and Samantha Forde, Predation and the evolutionary dynamics of species ranges, Am. Nat., 178 (2011), 488-500. doi: 10.1086/661909
![]() |
[31] |
Mark Kirkpatrick and Nicholas H. Barton, Evolution of a species' range, Am. Nat., 150 (1997), 1-23. doi: 10.1086/286054
![]() |
[32] |
Jean-François Mallordy and Jean-Michel Roquejoffre, A parabolic equation of the KPP type in higher dimensions, SIAM J. Math. Anal., 26 (1995), 1-20. doi: 10.1137/S0036141093246105
![]() |
[33] |
Hiroshi Matano, Asymptotic behavior and stability of solutions of semilinear diffusion equations, Publ. Res. Inst. Math. Sci., 15 (1979), 401-454. doi: 10.2977/prims/1195188180
![]() |
[34] | Hiroshi Matano, Traveling waves in spatially inhomogeneous diffusive media - the non-periodic case, Preprint, 2012. |
[35] |
Avraham Mayevsky, Avi Doron, Tamar Manor, Sigal Meilin, Nili Zarchin and George E. Ouaknine, Cortical spreading depression recorded from the human brain using a multiparmetric monitoring system, Brain Res., 740 (1996), 268-274. doi: 10.1016/S0006-8993(96)00874-8
![]() |
[36] |
Maiken Nedergaard, Arthur J. Cooper and Steven A. Goldman, Gap junctions are required for the propagation of spreading depression, J. Neurobiol., 28 (1995), 433-444. doi: 10.1002/neu.480280404
![]() |
[37] |
James Nolen, Jean-Michel Roquejoffre, Lenya Ryzhik and Andrej Zlatoš, Existence and non-existence of fisher-kpp transition fronts, Arch. Ration. Mech. Anal., to appear, 2012. doi: 10.1007/s00205-011-0449-4
![]() |
[38] |
L. A. Peletier and James Serrin, Uniqueness of nonnegative solutions of semilinear equations in $R^n$, J. Differential Equations, 61 (1986), 380-397. doi: 10.1016/0022-0396(86)90112-9
![]() |
[39] |
Cristina Pocci, Ayman Moussa, Florence Hubert and Guillemette Chapuisat., Numerical study of the stopping of aura during migraine, in "CEMRACS (2009): Mathematical Modelling in Medicine", 30 of ESAIM Proc., 44-52. EDP Sci., Les Ulis, (2010). doi: 10.1051/proc/2010005
![]() |
[40] |
Indiana Univ. Math. J., 23 (1973/74), 173-186. doi: 10.1512/iumj.1973.23.23014
![]() |
[41] |
Lionel Roques, Jimmy Garnier, François Hamel and Etienne K. Klein, Allee effect promotes diversity in traveling waves of colonization, Proceedings of the National Academy of Sciences, 109 (2012), 8828-8833. doi: 10.1073/pnas.1201695109
![]() |
[42] | Laurent Schwartz, "Analyse Hilbertienne," Collection Méthodes. Hermann, Paris, 1979. |
[43] | Bruno Shapiro, Osmotic forces and gap junctions in spreading depression: A computational model, J. Comput. Neurosci., 10 (2001), 99-120. |
[44] |
Wenxian Shen, Dynamical systems and traveling waves in almost periodic structures, J. Differential Equations, 169 (2001), 493-548. Special issue in celebration of Jack K. Hale's 70th birthday, Part 4 (Atlanta, GA/Lisbon, 1998). doi: 10.1006/jdeq.2000.3906
![]() |
[45] | George G. Somjen, "Ions in the Brain: Normal Function, Seizures, and Stroke," Oxford University Press, New York, 2004. |
[46] |
Anthony J. Strong, Martin Fabricius, Martyn G. Boutelle, Stuart J. Hibbins, Sarah E. Hopwood, Robina Jones, Mark C. Parkin and Martin Lauritzen, Spreading and synchronous depressions of cortical activity in acutely injured human brain, Stroke, 33 (2002), 2738-2743. doi: 10.1161/01.STR.0000043073.69602.09
![]() |
[47] |
Henry C. Tuckwell, Predictions and properties of a model of potassium and calcium ion movements during spreading cortical depression, Int. J. Neurosci., 10 (1980), 145-164. doi: 10.3109/00207458009160493
![]() |
[48] |
José M. Vega, Travelling wavefronts of reaction-diffusion equations in cylindrical domains, Comm. Partial Differential Equations, 18 (1993), 505-531. doi: 10.1080/03605309308820939
![]() |
[49] | Marcel O. Vlad, L. Luca Cavalli-Sforza and John Ross, Enhanced (hydrodynamic) transport induced by population growth in reactiondiffusion systems with application to population genetics, Proceedings of the National Academy of Sciences, 101 (2004), 10249-10253. |
[50] | Andrej Zlatoš, Generalized traveling waves in disordered media: Existence, uniqueness, and stability, Preprint, 2012. |
Variables | Moderate-to-severe OSA (AHI ≥ 15) |
None-to-mild OSA (AHI < 15) |
p-value |
n | 5, 920 | 3, 502 | |
Age | 50.8 ± 13.5 | 46.6 ± 14.4 | < 0.0001 |
Female, n (%) | 1266 (21.4) | 1652 (47.2) | < 0.0001 |
Height (cm) | 167.2 ± 8.5 | 164.4 ± 8.9 | < 0.0001 |
Weight (kg) | 79.7±16.1 | 66.1 ± 13.1 | < 0.0001 |
BMI (kg/m2) | 28.4 ± 4.8 | 24.3 ± 3.8 | < 0.0001 |
Polysomnography | |||
Total recording time (min) | 366.6±11.9 | 367.8±13.4 | < 0.0001 |
Total sleep time (min) | 275.8±60.2 | 281.4±61.1 | < 0.0001 |
Sleep efficiency (%) | 75.2±16.4 | 76.5±16.5 | 0.0002 |
Non-REM sleep (min) | 240.6±51.7 | 242.2±51.3 | 0.133 |
REM sleep (min) | 35.2±22.5 | 39.1±23.7 | < 0.0001 |
AHI | 44.7±23.8 | 6.4±4.5 | < 0.0001 |
SO2 mean (%) | 94.5±2.6 | 96.6±1.3 | < 0.0001 |
Data are expressed as mean ± standard deviation. The p-value represents the comparison between the AHI ≥ 15 and AHI < 15 groups. |
Unadjusted Model | Adjusted Model | |||
Variables | OR | 95% CI | OR | 95% CI |
Age | 1.02*† | 1.02-1.03 | 1.04*† | 1.04-1.05 |
Female | 0.30* | 0.28-0.33 | 0.28* | 0.25-0.32 |
BMI | 1.27*† | 1.25-1.28 | 1.27*† | 1.26-1.29 |
The odds ratio (OR) and 95% confidence intervals (CI) represent the association between the variables and the presence of moderate-to-severe OSA (AHI ≥ 15). *p < 0.0001; †Odds ratio of per unit changes. |
Variable | Testing set 1 | Testing set 2 | Testing set 3 | Testing set 4 | Testing set 5 | Independent validation set | p-value |
n | 1466 | 1465 | 1466 | 1465 | 1466 | 2094 | |
AHI≥15, n (%) | 920 (62.8) | 919 (62.7) | 920 (62.8) | 919 (62.7) | 920 (62.8) | 1322 (63.1) | 1.000 |
Age | 49.0 ± 0.4 | 49.0 ± 0.4 | 49.9 ± 0.4 | 49.1 ± 0.4 | 49.0 ± 0.4 | 49.3 ± 0.3 | 0.503 |
Female, n (%) | 483 (33.0) | 438 (29.9) | 472 (32.2) | 445 (30.4) | 431 (29.4) | 649 (31.0) | 0.266 |
BMI (kg/m2) | 26.9 ± 4.9 | 26.9 ± 4.9 | 26.9 ± 5.0 | 26.9 ± 5.0 | 26.8 ± 4.8 | 26.9 ± 4.9 | 0.973 |
The p-value represents the multiple comparisons across the five testing and the independent validation sets. |
Variables | Moderate-to-severe OSA (AHI ≥ 15) |
None-to-mild OSA (AHI < 15) |
p-value |
n | 5, 920 | 3, 502 | |
Age | 50.8 ± 13.5 | 46.6 ± 14.4 | < 0.0001 |
Female, n (%) | 1266 (21.4) | 1652 (47.2) | < 0.0001 |
Height (cm) | 167.2 ± 8.5 | 164.4 ± 8.9 | < 0.0001 |
Weight (kg) | 79.7±16.1 | 66.1 ± 13.1 | < 0.0001 |
BMI (kg/m2) | 28.4 ± 4.8 | 24.3 ± 3.8 | < 0.0001 |
Polysomnography | |||
Total recording time (min) | 366.6±11.9 | 367.8±13.4 | < 0.0001 |
Total sleep time (min) | 275.8±60.2 | 281.4±61.1 | < 0.0001 |
Sleep efficiency (%) | 75.2±16.4 | 76.5±16.5 | 0.0002 |
Non-REM sleep (min) | 240.6±51.7 | 242.2±51.3 | 0.133 |
REM sleep (min) | 35.2±22.5 | 39.1±23.7 | < 0.0001 |
AHI | 44.7±23.8 | 6.4±4.5 | < 0.0001 |
SO2 mean (%) | 94.5±2.6 | 96.6±1.3 | < 0.0001 |
Data are expressed as mean ± standard deviation. The p-value represents the comparison between the AHI ≥ 15 and AHI < 15 groups. |
Unadjusted Model | Adjusted Model | |||
Variables | OR | 95% CI | OR | 95% CI |
Age | 1.02*† | 1.02-1.03 | 1.04*† | 1.04-1.05 |
Female | 0.30* | 0.28-0.33 | 0.28* | 0.25-0.32 |
BMI | 1.27*† | 1.25-1.28 | 1.27*† | 1.26-1.29 |
The odds ratio (OR) and 95% confidence intervals (CI) represent the association between the variables and the presence of moderate-to-severe OSA (AHI ≥ 15). *p < 0.0001; †Odds ratio of per unit changes. |
Variable | Testing set 1 | Testing set 2 | Testing set 3 | Testing set 4 | Testing set 5 | Independent validation set | p-value |
n | 1466 | 1465 | 1466 | 1465 | 1466 | 2094 | |
AHI≥15, n (%) | 920 (62.8) | 919 (62.7) | 920 (62.8) | 919 (62.7) | 920 (62.8) | 1322 (63.1) | 1.000 |
Age | 49.0 ± 0.4 | 49.0 ± 0.4 | 49.9 ± 0.4 | 49.1 ± 0.4 | 49.0 ± 0.4 | 49.3 ± 0.3 | 0.503 |
Female, n (%) | 483 (33.0) | 438 (29.9) | 472 (32.2) | 445 (30.4) | 431 (29.4) | 649 (31.0) | 0.266 |
BMI (kg/m2) | 26.9 ± 4.9 | 26.9 ± 4.9 | 26.9 ± 5.0 | 26.9 ± 5.0 | 26.8 ± 4.8 | 26.9 ± 4.9 | 0.973 |
The p-value represents the multiple comparisons across the five testing and the independent validation sets. |