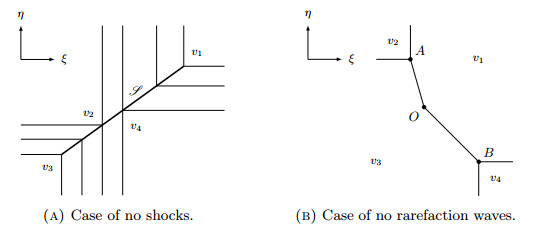
The aim of this work is to introduce a two-dimensional macroscopic traffic model for multiple populations of vehicles. Starting from the paper [
Citation: Caterina Balzotti, Simone Göttlich. A two-dimensional multi-class traffic flow model[J]. Networks and Heterogeneous Media, 2021, 16(1): 69-90. doi: 10.3934/nhm.2020034
[1] | Caterina Balzotti, Simone Göttlich . A two-dimensional multi-class traffic flow model. Networks and Heterogeneous Media, 2021, 16(1): 69-90. doi: 10.3934/nhm.2020034 |
[2] | Mohamed Benyahia, Massimiliano D. Rosini . A macroscopic traffic model with phase transitions and local point constraints on the flow. Networks and Heterogeneous Media, 2017, 12(2): 297-317. doi: 10.3934/nhm.2017013 |
[3] | Michael Herty, Adrian Fazekas, Giuseppe Visconti . A two-dimensional data-driven model for traffic flow on highways. Networks and Heterogeneous Media, 2018, 13(2): 217-240. doi: 10.3934/nhm.2018010 |
[4] | Benjamin Seibold, Morris R. Flynn, Aslan R. Kasimov, Rodolfo R. Rosales . Constructing set-valued fundamental diagrams from Jamiton solutions in second order traffic models. Networks and Heterogeneous Media, 2013, 8(3): 745-772. doi: 10.3934/nhm.2013.8.745 |
[5] | Felisia Angela Chiarello, Paola Goatin . Non-local multi-class traffic flow models. Networks and Heterogeneous Media, 2019, 14(2): 371-387. doi: 10.3934/nhm.2019015 |
[6] | Fabio Della Rossa, Carlo D’Angelo, Alfio Quarteroni . A distributed model of traffic flows on extended regions. Networks and Heterogeneous Media, 2010, 5(3): 525-544. doi: 10.3934/nhm.2010.5.525 |
[7] | Cécile Appert-Rolland, Pierre Degond, Sébastien Motsch . Two-way multi-lane traffic model for pedestrians in corridors. Networks and Heterogeneous Media, 2011, 6(3): 351-381. doi: 10.3934/nhm.2011.6.351 |
[8] | Paola Goatin, Chiara Daini, Maria Laura Delle Monache, Antonella Ferrara . Interacting moving bottlenecks in traffic flow. Networks and Heterogeneous Media, 2023, 18(2): 930-945. doi: 10.3934/nhm.2023040 |
[9] | Tibye Saumtally, Jean-Patrick Lebacque, Habib Haj-Salem . A dynamical two-dimensional traffic model in an anisotropic network. Networks and Heterogeneous Media, 2013, 8(3): 663-684. doi: 10.3934/nhm.2013.8.663 |
[10] | Shimao Fan, Michael Herty, Benjamin Seibold . Comparative model accuracy of a data-fitted generalized Aw-Rascle-Zhang model. Networks and Heterogeneous Media, 2014, 9(2): 239-268. doi: 10.3934/nhm.2014.9.239 |
The aim of this work is to introduce a two-dimensional macroscopic traffic model for multiple populations of vehicles. Starting from the paper [
In this paper, we are concerned with the study of a two-dimensional multi-class traffic model. This work is placed in the constantly evolving framework of mathematical models for traffic flow. The goal of traffic models is to provide tools capable of helping traffic management, in order to optimize transport and obtain economic and environmental benefits, such as the reduction of vehicles queues and pollution.
Traffic models are divided into three main categories, which depend on the scale of observation: microscopic, macroscopic and kinetic models. Microscopic models follow the dynamics of each vehicle and are described by ordinary differential equations (ODEs), see e. g. [2,16,19,33,34,38]. Macroscopic models, based on fluid dynamics, consider aggregated quantities such as the density of vehicles and are governed by partial differential equations (PDEs), see e. g. [3,7,12,17,32,41,46]. Kinetic models [24,28,33,36,39,40] are between the previous two classes since they can be derived by microscopic models while macroscopic models can be derived by kinetic descriptions. We refer to [1,15] and references therein for a more complete review on traffic models.
In recent years, the ever-increasing amount of real data, due to new technologies, has widely influenced the research on mathematical models for traffic flow. The common goal of researchers is to exploit the available real data to build ad hoc traffic models, capable of simulating increasingly realistic scenarios. We refer to [8,9,13,37] for some inspiring examples of data-fitted traffic models. The common feature of these models is the application of vehicles trajectory data collected in datasets such as [25,44]. Datasets of this type generally contain data on multi-lane highways and are able to distinguish the type of vehicle.
The focus in this work is on macroscopic traffic models. In particular, we propose a multi-class generalization in two space dimensions of the well-known first order Lighthill-Whitham-Richards (LWR) model [32,41]. First order models such as the LWR are described by a single conservation law
As we have already mentioned, traffic datasets contain information related to multi-lane highways with different types of vehicles. Most traffic models refer to dynamics of single-lane traffic and therefore do not consider the movements related to lane changes. Our aim is to exploit now all the available data, including the line-changing behavior and the different vehicles classes. To this end, we propose an extension of the work by Herty, Fazekas and Visconti for a single-class traffic model [21] to a multi-class traffic model in two space dimensions. The most common approaches which include lane-changing are the two-dimensional models and the multi-lane models. The first approach is an emerging topic, and we refer to [6,21,22] for some examples. The second approach has been used for instance in [26,27], where the authors propose a microscopic, a kinetic and a fluid dynamic model with lane changing. Here, we stick to the two-dimensional approach and incorporate two types of vehicles interacting through the flux functions. The proposed model is then defined by the coupling of LWR-type models for two classes of vehicles in the
The paper is organized as follows. In Section 2 we introduce the traffic model and study the Riemann problems. In Section 3, we describe the numerical scheme and validate the model via numerical tests. In Section 4, we calibrate the model with a German dataset and compare the results with the real trajectories of vehicles. In Section 5, we propose a modified version of the model calibrated with real data, and finally we investigate on the ability of the model to simulate vehicles overtaking compared to a multi-lane model.
In this section, we introduce the traffic model used throughout the paper. Let us consider two classes of vehicles, whose densities are denoted by
{ρt+(qxρ(ρ,μ))x+(qyρ(ρ,μ))y=0μt+(qxμ(ρ,μ))x+(qyμ(ρ,μ))y=0, | (1) |
where
qxρ(ρ,μ)=ρcx(1−(ρ+μrmax))qyρ(ρ,μ)=ρcy(1−(ρ+μrmax))qxμ(ρ,μ)=μcx(1−(ρ+μrmax))qyρ(ρ,μ)=μcy(1−(ρ+μrmax)), | (2) |
where
ux=cx(1−(ρ+μrmax)),uy=cy(1−(ρ+μrmax)). |
Hence,
First of all, we present the properties of model (1). To simplify the notation, we normalize
U=(ρμ),f(U)=(ρcx(1−(ρ+μ))μcx(1−(ρ+μ))),g(U)=(ρcy(1−(ρ+μ))μcy(1−(ρ+μ))) |
and matrices
A(U)=Df(U),B(U)=Dg(U). |
Therefore, we can rewrite system (1) as
Ut+AUx+BUy=0. | (3) |
System (3) is hyperbolic if any linear combination of
λ1=(κ1cx+κ2cy)(1−(ρ+μ)),λ2=(κ1cx+κ2cy)(1−2(ρ+μ)) |
which are real for any couple
γ1=(−11),γ2=(ρ/μ1). |
The first eigenvalue is linearly degenerate, i.e.,
z1=ρ+μ,z2=log(ρ/μ). |
Next, we describe the two-dimensional Riemann problem [10,47] associated with (1). To simplify the computations, we introduce the variable
{rt+(rcx(1−r))x+(rcy(1−r))y=0r(0,x,y)=r0(x,y). | (4) |
The Riemann problem in two dimensions is given by (4) with constant initial data in the four quadrants, i.e.
r0(x,y)={v10<x<∞,0<y<∞v2−∞<x<0,0<y<∞v3−∞<x<0,−∞<y<0v40<x<∞,−∞<y<0. | (5) |
For convenience, we define
Remark 1. The treatment of the two-dimensional Riemann problem proposed in [10] assumes convex flux functions
We look for self-similar solutions
(cx(1−2v)−ξ)vξ+(cy(1−2v)−η)vη=0 | (6) |
which leads us to
(cx(1−2v)−ξ)dη+(cy(1−2v)−η)dξ=0, |
where
The set of singular points parametrized by
S={(ξ,η)|ξ=cx(1−2v),η=cy(1−2v)}. |
Defining
γ(v−,v+)=ˆf(v+)−ˆf(v−)v+−v−=cx(1−v+−v−)ν(v−,v+)=ˆg(v+)−ˆg(v−)v+−v−=cy(1−v+−v−), | (7) |
the Rankine-Hugoniot jump condition is
dηdξ=−ν(v−,v+)−ηγ(v−,v+)−ξ=−cy(1−v+−v−)−ηcx(1−v+−v−)−ξ. | (8) |
Assuming that the normal vector
(γ(v−,v0)−γ(v−,v+))dη+(ν(v−,v0)−ν(v−,v+))dξ=cx(1−v+−v0)dη+cy(1−v+−v0)dξ≥0 | (9) |
for
The initial data in (5) for problem (4) in the variables
limξ/η=const,ξ2+η2→∞v(ξ,η)={v1ξ>0,η>0v2ξ<0,η>0v3ξ<0,η<0v4ξ>0,η<0. | (10) |
The solution of problem (6) with initial data (10) is composed of elementary waves. There are five possible cases: (1) no shocks, (2) no rarefaction waves, (3) exactly one shock, (4) exactly one rarefaction wave, (5) two rarefaction waves and two shocks. In this work we skip the full details of the possible cases and refer to [47] for a detailed discussion. Let us highlight the five cases now:
(1) No shocks: This case is verified when
(2) No rarefaction waves: This case is verified when
(3) Exactly one shock: This case is verified when
v4>v1≥v2≥v3orv2<v3≤v4≤v1. |
The first sub-case is represented in Figure 2(A). Using the Rankine-Hugoniot condition (8) it can be shown that the shock curve is concave, monotonically increasing in
(4) Exactly one rarefaction wave: This case is verified when
v1≤v2≤v3<v4orv2<v1≤v4≤v3. |
The first possibility of initial data gives results similar to the previous case of exactly one shock wave. For the second initial datum there exist several sub-cases but we omit the details. In Figure 3 we show two examples for the two initial data configurations.
(5) Two shocks and two rarefaction waves: This case is verified when
v4>v1≥v3>v2orv4>v3>v1>v2. |
The main difference between the two options of initial data is that in the first case the shock curves are not neighbors while in the second case they are neighbors. There are again several sub-cases, we only show an example of the two possible initial data sets in Figure 4.
In the next section, we introduce a suitable discretization for the multi-class model. For validation purposes of the proposed scheme, we aim to recover the theoretical results from above.
The numerical analysis of system (3) can be done using the dimensional splitting approach [31] which means that the two-dimensional problem is split into two one-dimensional problems. Thus, equation (3) is split into
Ut+AUx=0,Ut+BUy=0. |
We uniformly divide the two-dimensional space
ΔtΔx≤12(maxi,j=1,2{|λi|,|γj|})−1. | (11) |
Then, the time interval
Starting from a given initial datum
Uij∗=Uijn−Δt2Δx(Fni+1/2,j−Fni−1/2,j)Uij∗∗=Uij∗−ΔtΔy(G∗i,j+1/2−G∗i,j−1/2)Uijn+1=Uijn−Δt2Δx(F∗∗i+1/2,j−F∗∗i−1/2,j). |
We use the Local Lax-Friedrichs flux (also known as Rusanov flux) [5,Chapter 3] for
Fi+1/2,j=12(f(Ui+1,j)+f(Ui,j)−αi+1/2,j(Ui+1,j−Ui,j)), |
where
We now test the discretization method for the two-dimensional multi-class model (3) while comparing the numerical results to the theoretical solutions of the Riemann problems introduced in Section 2.1.
Our test setting is given by
ρ0(x,y)={ρ1(x,y)∈(0,5)×(0,5)ρ2(x,y)∈(−5,0)×(0,5)ρ3(x,y)∈(−5,0)×(−5,0)ρ4(x,y)∈(0,5)×(−5,0). |
and
Since we aim to recover the results of the analysis done in Section 2.1, where the plots are defined for the plane
As we have explained in Section 2.1, there are only five possible configurations of the solution, which are determined by the initial values
(1) No shocks: We fix
(2) No rarefaction waves: We fix
(3) Exactly one shock: We fix
(4) Exactly one rarefaction wave: We fix
(5) Two shocks and two rarefaction waves: We fix
In this section, we calibrate the two-dimensional multi-class model with a dataset of real trajectories data. We employ the public German dataset [25] which contains vehicle trajectories data recorded on the German motorway A3, nearby Frankfurt am Main. The analyzed area is a three lanes highway of about 900 meters in length and 12 meters in width, depicted in Figure 6. A system of five video cameras recorded the vehicles passing through the study area, collecting trajectory data for 20 minutes with a sampling period of about 0.2 seconds. We refer to [25] for a detailed description of the dataset and of the data collection method. We observe that the dataset distinguishes several types of vehicles, and particularly in this work we focus on the dynamics of cars and trucks.
In order to calibrate the two-dimensional model with the German dataset, we need first to derive macroscopic quantities from the microscopic information provided by the dataset. Following [21], we describe how we derive the density of cars and trucks,
We consider the data from the second camera from the right of Figure 6, thus we work with 20 minutes of real data. We introduce the time interval (
˜ρ(tk)=Nρ(tk)Lx,˜μ(tk)=Nμ(tk)Lx. | (12) |
The German dataset only provides the position of vehicles with respect to the two directions, thus we need to derive the speed of vehicles from their positions. We assume that each vehicle travels at constant speed which corresponds to the slope of a linear approximation in the least square sense of the vehicle positions. We denote by
˜ux(tk)=1Nρ(tk)Nρ(tk)∑i=1vxi+1Nμ(tk)Nμ(tk)∑i=1wxi˜uy(tk)=1Nρ(tk)Nρ(tk)∑i=1vyi+1Nμ(tk)Nμ(tk)∑i=1wyi. | (13) |
Finally, we combine equations (12) and (13) to define the flux functions as
˜qxρ(tk)=˜ρ(tk)˜ux(tk),˜qyρ(tk)=˜ρ(tk)˜uy(tk)˜qxμ(tk)=˜μ(tk)˜ux(tk),˜qyμ(tk)=˜μ(tk)˜uy(tk). | (14) |
Once we have the density, speed and flux data as functions of time, we aggregate them with respect to a certain time period
The graphs show that the main direction of the flow is along the
rmax=# laneslength of vehicles + safety distance=37.5 m=400vehkm. | (15) |
However, it should be noted that the dataset only contains data in free-flow regimes without capturing congested traffic phase.
Now we need to compute the parameters
mincx(‖˜qxρ−qxρ(ρ,μ)‖22+‖˜qxμ−qxμ(ρ,μ)‖22)mincy(‖˜qyρ−qyρ(ρ,μ)‖22+‖˜qyμ−qyμ(ρ,μ)‖22). |
The computation is performed using the MATLAB
We observe that the advantage of the multi-class model is that we can cover quite well the clouds of real data by means of the family of flux and velocity functions. However, we note that, since the German dataset contains data which refer only to the not congested phase of traffic, we do not have enough data to better calibrate congested traffic situations. In particular, the choice of
In this section we describe how to treat the microscopic data to define the initial density for the numerical scheme and the reference solution for the comparison of the results. The German dataset gives information about the position of vehicles every 0.2 seconds, thus we work with pointwise data. In order to define a density function
Let
˜ρ(x,y)=N(t)∑i=1δ(x−xi(t))δ(y−yi(t)). |
In order to recover the smooth function
K(x,y)=12πhxhyexp(−x22h2x−y22h2y), |
and then define
ρ(t,x,y)=∫DK(x−ξ,y−η)˜ρ(ξ,η)dξdη=N(t)∑i=1K(x−xi(t),y−yi(t)). | (16) |
We follow a similar procedure to estimate the density of trucks. The parameters
For each video camera of the German dataset we work with records data for about
Now, we compare the numerical simulations of model (1) with the real data computed from equation (16). The simulation refers to the data recorded by the second video camera of the German dataset.
Let us consider the domain
¯ρnij=¯ρ(xi,yj,tn),¯μnij=¯μ(xi,yj,tn) |
for cars and trucks respectively, with
ρtrue,nij=ρtrue(xi,yj,tn),μtrue,nij=μtrue(xi,yj,tn) |
for cars and trucks, respectively. The parameters used in the following test are
The dotted lines divide the road into three lanes. After
In order to better compare the numerical results with the ground-truth data, we introduce the following errors
Eρ(tn)=‖ρtrue(⋅,⋅,tn)−¯ρ(⋅,⋅,tn)‖L1, | (17) |
Eμ(tn)=‖μtrue(⋅,⋅,tn)−¯μ(⋅,⋅,tn)‖L1. | (18) |
The errors at time
In the previous section we have seen that the two dimensional multi-class LWR (1) with the flux functions defined in (2) and calibrated with real data is able to simulate the dynamics of vehicles. However, our main assumption on the flux functions is that the coefficients
We consider again the German dataset [25]. Our aim is to consider different maximum density of cars and trucks, due to the different length of vehicles, and different parameters
qxρ(ρ,μ)=ρcxρ(1−(ρ+2μrmax)),qyρ(ρ,μ)=ρcyρ(1−(ρ+2μrmax))qxμ(ρ,μ)=μcxμ(1−(ρ+2μrmax)),qyμ(ρ,μ)=μcyμ(1−(ρ+2μrmax)). | (19) |
Note that in (19) the different maximum densities between cars and trucks is expressed by the term
With the introduction of different coefficients
Next, we repeat a procedure analogous to the one proposed in Section 4.1 to estimate the velocity functions and the fundamental diagrams. In particular, we define different velocity functions for
˜uxρ(tk)=1Nρ(tk)Nρ(tk)∑i=1vxi,˜uyρ(tk)=1Nρ(tk)Nρ(tk)∑i=1vyi˜uxμ(tk)=1Nμ(tk)Nμ(tk)∑i=1wxi,˜uyμ(tk)=1Nμ(tk)Nμ(tk)∑i=1wyi, |
from which we recover the flux functions as
˜qxρ(tk)=˜ρ(tk)˜uxρ(tk),˜qyρ(tk)=˜ρ(tk)˜uyρ(tk)˜qxμ(tk)=˜μ(tk)˜uxμ(tk),˜qyμ(tk)=˜μ(tk)˜uyμ(tk). | (20) |
We estimate the parameters
mincxρ(‖˜qxρ−qxρ(ρ,μ)‖22)mincyρ(‖˜qyρ−qyρ(ρ,μ)‖22)mincxμ(‖˜qxμ−qxμ(ρ,μ)‖22)mincyρ(‖˜qyμ−qyμ(ρ,μ)‖22), |
using again the
In Figure 11, we show the family of speed and flux functions obtained with the above described procedure. Note that the speed and flux functions related to trucks, shown Figures 11(B), 11(D), 11(F) and 11(H), are defined for
We repeat the same numerical test proposed in Section 4.3 with the new flux functions (19), estimating again the resulting errors with (17) and (18). The density plots we obtain are similar to the plots shown in Figure 9, thus we omit the picture. However, as shown in Figure 12, we obtain a better estimate of the errors compared to the test done in Section 4.3.
A further investigation of our model is the testing of the ability of capturing vehicles overtaking. We consider the following flux functions
qxρ(ρ,μ)=ρcxρ(1−(ρ+μrmax)),qyρ(ρ,μ)=ρcyρ(1−(ρ+μrmax))qxμ(ρ,μ)=μcxμ(1−(ρ+μrmax)),qyμ(ρ,μ)=μcyμ(1−(ρ+μrmax)), |
where we have different parameters
We consider a numerical grid
As we can see in Figure 13, we consider a road with two lanes with two cars and a truck. At the beginning of the simulation there is a car in the top lane and a truck in front of the other car in the bottom lane. Since the truck does not move and the cars are free to move along the
Finally, we intend to compare the proposed 2D multi-class approach to a first order multi-class multi-lane model. Indeed, the inclusion of lane change dynamics well fits with the simulation of vehicle overtaking and allows us to compare the results obtained with the 2D multi-class model. Specifically, we extend the multi-lane LWR model proposed in [23] to a multi-class model. Hence, let us consider a road with two lanes and two classes of vehicles
Lane 1: {ρ1t+(q1ρ(ρ1,μ1))x=−Sρ(ρ1,μ1,ρ2,μ2)μ1t+(q1μ(ρ1,μ1))x=−Sμ(ρ1,μ1,ρ2,μ2)Lane 2: {ρ2t+(q2ρ(ρ2,μ2))x=Sρ(ρ1,μ1,ρ2,μ2)μ2t+(q2μ(ρ2,μ2))x=Sμ(ρ1,μ1,ρ2,μ2), | (21) |
where
Sρ=C(max{u2ρ(ρ2,μ2)−u1ρ(ρ1,μ1),0}ρ1+min{u2ρ(ρ2,μ2)−u1ρ(ρ1,μ1),0}ρ2)Sμ=C(max{u2μ(ρ2,μ2)−u1μ(ρ1,μ1),0}μ1+min{u2μ(ρ2,μ2)−u1μ(ρ1,μ1),0}μ2), | (22) |
where
The flux functions are chosen similar to the ones used for the two-dimensional multi-class model. Therefore, we define them as
q1,2ρ=ρ1,2cρ(1−(ρ1,2+μ1,2rmax)),q1,2μ=μ1,2cμ(1−(ρ1,2+μ1,2rmax)), |
where
In Figure 14, we show the density of cars and trucks on the two lanes at different times. The plots in the first row show Lane 1, with a car and no trucks, the plots in the second row show Lane 2, with a car and a truck which does not move at all during the simulation since its velocity is 0. The source terms in (21) allow lane changing even if the traffic is not congested and vehicles are free to move along their lane. Hence, in Figure 14(E) we see that the density of cars increases at the end of Lane 2, due to the source term, while in Figures 14(B) and 14(C) the density of cars related to Lane 1 increases since the truck does not move and the cars change lane. At the end of the simulation the density of cars is higher on Lane 1 while it is close to 0 on Lane 2.
From the two tests proposed in Sections 5.2 and 5.2.1 we observe that the 2D multi-class model seems to be more suitable for capturing the overtaking of vehicles. Indeed, the two-dimensional description seem to fit better to such a dynamics, as shown in Figures 13 and 14.
In this work we have introduced a two-dimensional multi-class traffic model. We have analyzed the two-dimensional Riemann problems related to our model and provided numerical validations with a numerical scheme based on dimensional splitting. Then, we have analyzed the model with a dataset of real trajectories data, focusing on the dynamics of cars and trucks. The dataset has been used to calibrate the flux and velocity functions and to compare the numerical results with ground-truth data. The numerical tests have shown the good approximation of the trajectories with our model, obtaining a numerical error of
Future investigations will aim at improving the proposed approach with second order models and deriving the corresponding microscopic model as a system of ordinary differential equations.
[1] |
Vehicular traffic, crowds, and swarms: From kinetic theory and multiscale methods to applications and research perspectives. Math. Models Methods Appl. Sci. (2019) 29: 1901-2005. ![]() |
[2] |
Derivation of continuum traffic flow models from microscopic follow-the-leader models. SIAM J. Appl. Math. (2002) 63: 259-278. ![]() |
[3] |
Resurrection of "second order" models of traffic flow. SIAM J. Appl. Math. (2000) 60: 916-944. ![]() |
[4] |
An n-populations model for traffic flow. European J. Appl. Math. (2003) 14: 587-612. ![]() |
[5] | S. Bertoluzza, S. Falletta, G. Russo and C.-W. Shu, Numerical Solutions of Partial Differential Equations, Advanced Courses in Mathematics, CRM Barcelona, Birkhäuser Verlag, Basel, 2009. |
[6] | B. N. Chetverushkin, N. G. Churbanova, Y. N. Karamzin and M. A. Trapeznikova, A two-dimensional macroscopic model of traffic flows based on KCFD-schemes, in ECCOMAS CFD 2006: Proceedings of the European Conference on Computational Fluid Dynamics, Egmond aan Zee, The Netherlands, September 5-8, 2006, Citeseer, 2006. |
[7] |
A 2×2 hyperbolic traffic flow model. Math. Comput. Modelling (2002) 35: 683-688. ![]() |
[8] |
A traffic model aware of real time data. Math. Models Methods Appl. Sci. (2016) 26: 445-467. ![]() |
[9] |
A fluid dynamic approach for traffic forecast from mobile sensors data. Commun. Appl. Ind. Math. (2010) 1: 54-71. ![]() |
[10] |
C. M. Dafermos, Hyperbolic Conservation Laws in Continuum Physics, 4th edition, Grundlehren der Mathematischen Wissenschaften, 325, Springer-Verlag, Berlin, 2016. doi: 10.1007/978-3-662-49451-6
![]() |
[11] |
A continuum theory of traffic dynamics for freeways with special lanes. Transport. Res. B-Meth. (1997) 31: 83-102. ![]() |
[12] |
In traffic flow, cellular automata = kinematic waves. Transp. Res. B-Meth. (2006) 40: 396-403. ![]() |
[13] |
Comparative model accuracy of a data-fitted generalized Aw-Rascle-Zhang model. Netw. Heterog. Media (2014) 9: 239-268. ![]() |
[14] |
Data-fitted first-order traffic models and their second-order generalizations: Comparison by trajectory and sensor data. Transport. Res. Rec. (2013) 2391: 32-43. ![]() |
[15] | M. Garavello, K. Han and B. Piccoli, Models for Vehicular Traffic on Networks, American Institute of Mathematical Sciences, 2016. |
[16] |
Nonlinear follow-the-leader models of traffic flow. Oper. Res. (1961) 9: 545-567. ![]() |
[17] |
The Aw-Rascle vehicular traffic flow model with phase transitions. Math. Comput. Modelling (2006) 44: 287-303. ![]() |
[18] | A difference method for numerical calculation of discontinuous solutions of the equations of hydrodynamics. Matematicheskii Sbornik (1959) 89: 271-306. |
[19] |
D. Helbing, From microscopic to macroscopic traffic models, in A Perspective Look at Nonlinear Media, Lecture Notes in Physics, 503, Springer, Berlin, 1998, 122–139. doi: 10.1007/BFb0104959
![]() |
[20] |
Multi-class traffic models on road networks. Commun. Math. Sci. (2006) 4: 591-608. ![]() |
[21] |
A two-dimensional data-driven model for traffic flow on highways. Netw. Heterog. Media (2018) 13: 217-240. ![]() |
[22] |
Macroscopic modeling of multilane motorways using a two-dimensional second-order model of traffic flow. SIAM J. Appl. Math. (2018) 78: 2252-2278. ![]() |
[23] |
Models for dense multilane vehicular traffic. SIAM J. Math. Anal. (2019) 51: 3694-3713. ![]() |
[24] |
Vlasov-Fokker-Planck models for multilane traffic flow. Commun. Math. Sci. (2003) 1: 1-12. ![]() |
[25] | E. Kallo, A. Fazekas, S. Lamberty and M. Oeser, Microscopic traffic data obtained from videos recorded on a German motorway, https://data.mendeley.com/datasets/tzckcsrpn6/1, 2019. |
[26] |
A hierarchy of models for multilane vehicular traffic. Ⅰ. Modeling. SIAM J. Appl. Math. (1999) 59: 983-1001. ![]() |
[27] |
A hierarchy of models for multilane vehicular traffic. Ⅱ. Numerical investigations. SIAM J. Appl. Math. (1999) 59: 1002-1011. ![]() |
[28] |
Kinetic derivation of macroscopic anticipation models for vehicular traffic. SIAM J. Appl. Math. (2000) 60: 1749-1766. ![]() |
[29] |
A mechanism to describe the formation and propagation of stop-and-go waves in congested freeway traffic. Philos. T. R. Soc. A (2010) 368: 4519-4541. ![]() |
[30] |
Two-phase bounded-acceleration traffic flow model: Analytical solutions and applications. Transp. Res. Rec. (2003) 1852: 220-230. ![]() |
[31] |
(2002) Finite Volume Methods for Hyperbolic Problems. Cambridge: Cambridge Texts in Applied Mathematics, Cambridge University Press. ![]() |
[32] |
On kinematic waves. Ⅱ. A theory of traffic flow on long crowded roads. Proc. Roy. Soc. A (1955) 229: 317-345. ![]() |
[33] |
P. Nelson, A kinetic model of vehicular traffic and its associated bimodal equilibrium solutions, in Special Issue Devoted to the Proceedings of the 13th International Conference on Transport Theory (Riccione, 1993), 24, 1995,383–409. doi: 10.1080/00411459508205136
![]() |
[34] |
Nonlinear effects in the dynamics of car following. Oper. Res. (1961) 9: 209-229. ![]() |
[35] |
On estimation of a probability density function and mode. Ann. Math. Statist. (1962) 33: 1065-1076. ![]() |
[36] |
A kinetic model for traffic flow with continuum implications. Transport. Plan. Techn. (1979) 5: 131-138. ![]() |
[37] |
Second-order models and traffic data from mobile sensors. Transp. Res. C-Emer. (2015) 52: 32-56. ![]() |
[38] |
An operational analysis of traffic dynamics. J. Appl. Phys. (1953) 24: 274-281. ![]() |
[39] |
A Boltzmann-like approach for traffic flow. Oper. Res. (1960) 8: 789-797. ![]() |
[40] |
Kinetic models for traffic flow resulting in a reduced space of microscopic velocities. Kinet. Relat. Models (2017) 10: 823-854. ![]() |
[41] |
Shock waves on the highway. Oper. Res. (1956) 4: 42-51. ![]() |
[42] |
Remarks on some nonparametric estimates of a density function. Ann. Math. Statist. (1956) 27: 832-837. ![]() |
[43] | Empirical features of congested traffic states and their implications for traffic modeling. Transport. Sci. (2007) 41: 135-166. |
[44] | US Department of Transportation and Federal Highway Administration, Next Generation Simulation (NGSIM), http://ops.fhwa.dot.gov/trafficanalysistools/ngsim.htm. |
[45] |
A multi-class traffic flow model–an extension of LWR model with heterogeneous drivers. Transport. Res. A-Pol (2002) 36: 827-841. ![]() |
[46] |
A non-equilibrium traffic model devoid of gas-like behavior. Transp. Res. B (2002) 36: 275-290. ![]() |
[47] |
Two-dimensional Riemann problem for a single conservation law. Trans. Amer. Math. Soc. (1989) 312: 589-619. ![]() |
1. | Maya Briani, Emiliano Cristiani, Paolo Ranut, Macroscopic and Multi-Scale Models for Multi-Class Vehicular Dynamics with Uneven Space Occupancy: A Case Study, 2021, 10, 2075-1680, 102, 10.3390/axioms10020102 | |
2. | Durgesh Vikram, Sanjay Mittal, Partha Chakroborty, Stabilized finite element computations with a two-dimensional continuum model for disorderly traffic flow, 2022, 232, 00457930, 105205, 10.1016/j.compfluid.2021.105205 | |
3. | Shrey Agrawal, Venkatesan Kanagaraj, Martin Treiber, Two-dimensional LWR model for lane-free traffic, 2023, 625, 03784371, 128990, 10.1016/j.physa.2023.128990 | |
4. | Anamika Pandey, Rahul Barthwal, T. Raja Sekhar, Construction of solutions of the Riemann problem for a two-dimensional Keyfitz-Kranzer type model governing a thin film flow, 2025, 498, 00963003, 129378, 10.1016/j.amc.2025.129378 |