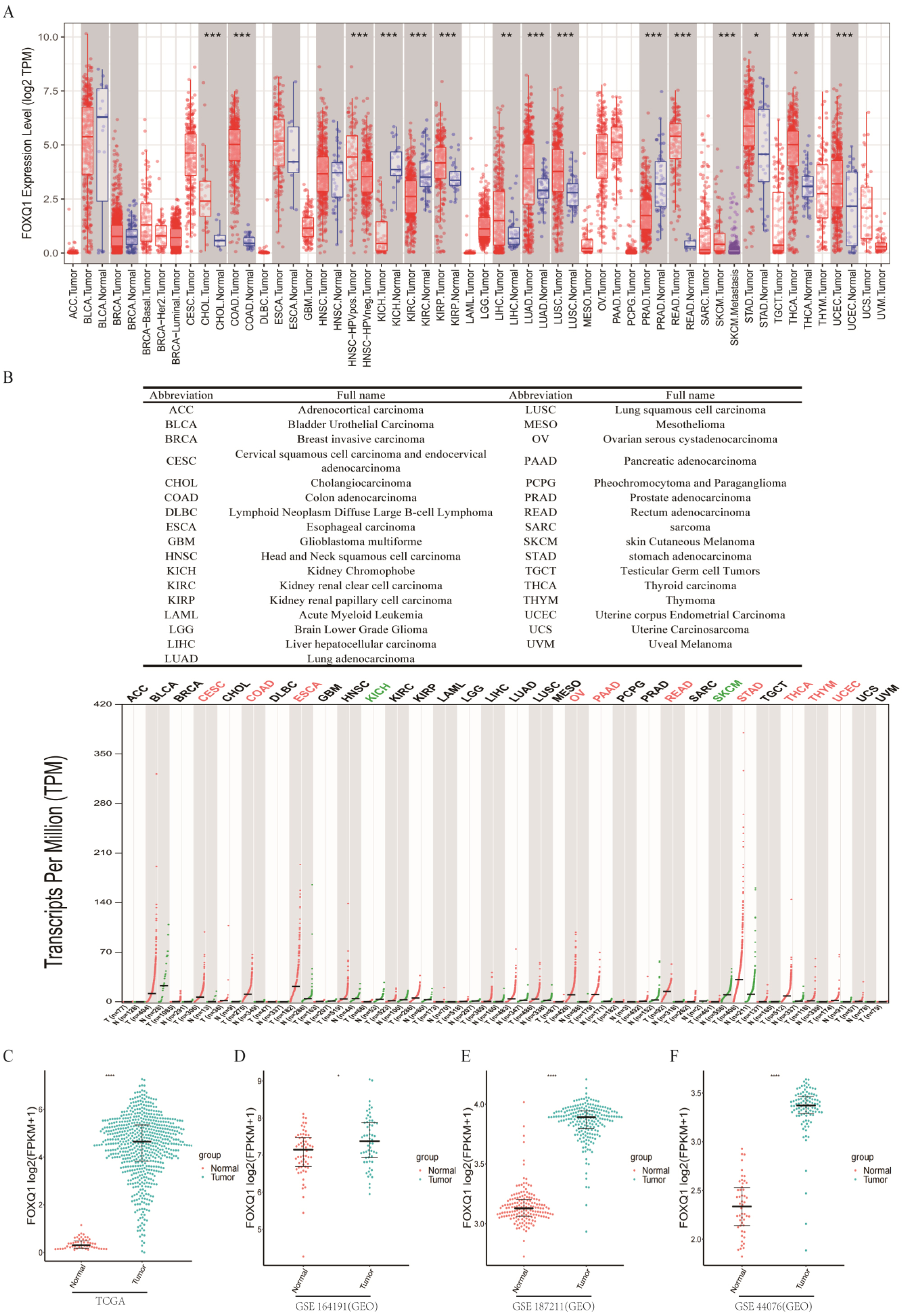
Forkhead box Q1 (FOXQ1) is a nuclear transcription factor that controls the transcriptional activity of downstream genes to exert biological effects. Since its regulatory role in colorectal cancer (CRC) is unknown, we correlated RNA-seq and ATAC-seq sequencing data from the Cancer Genome Atlas Program (TCGA), the Gene Expression Omnibus (GEO), and other databases with FOXQ1 knockdown data from our group and normal DLD1-CRC cell lines. First, we analyzed FOXQ1 gene expression across multiple databases and datasets and found significant differences in FOXQ1 gene expression in most tumors. In addition, we performed an extension analysis of the FOXQ1 gene at different levels and found a close relationship between the FOXQ1 gene and immunity. Then, based on the FOXQ1 gene, we collected its target genes and obtained a total of 107 genes that met the regulatory trend. After a series of target gene analyses, we obtained three key genes (SERPINA1, MYL9, and CFTR). Moreover, an enrichment analysis, an independent prognostic analysis, and an immune-related analysis were performed around FOXQ1 and its target genes. After knocking down FOXQ1 protein in SW480 cells, the SERPINA1 and MYL9 proteins were significantly altered, though the CFTR protein was not significantly changed. These results provide bioinformatics data that support studying and exploring the FOXQ1 gene and its target genes in CRC.
Citation: Yuxiang Zou, Jialong Qi, Hui Tang. Regulatory role of FOXQ1 gene and its target genes in colorectal cancer[J]. AIMS Medical Science, 2024, 11(3): 232-247. doi: 10.3934/medsci.2024018
[1] | Paul J Ross, Krishnie Srikandarajah, Julien de Naurois . Right versus left-sided colon cancer: Is it time to consider these as different diseases?. AIMS Medical Science, 2018, 5(3): 303-315. doi: 10.3934/medsci.2018.3.303 |
[2] | Derek A. Corica, Dylan M. Schaap, Trenton G. Mayberry, Braydon C. Cowan, Mark R. Wakefield, Yujiang Fang . New depths: exploring the current landscape of colorectal cancer. AIMS Medical Science, 2025, 12(1): 105-123. doi: 10.3934/medsci.2025008 |
[3] | Salma M. AlDallal . Quick glance at Fanconi anemia and BRCA2/FANCD1. AIMS Medical Science, 2019, 6(4): 326-336. doi: 10.3934/medsci.2019.4.326 |
[4] | Margarida Pujol-López, Luis Ortega-Paz, Manel Garabito, Salvatore Brugaletta, Manel Sabaté, Ana Paula Dantas . miRNA Update: A Review Focus on Clinical Implications of miRNA in Vascular Remodeling. AIMS Medical Science, 2017, 4(1): 99-112. doi: 10.3934/medsci.2017.1.99 |
[5] | Vivek Radhakrishnan, Mark S. Swanson, Uttam K. Sinha . Monoclonal Antibodies as Treatment Modalities in Head and Neck Cancers. AIMS Medical Science, 2015, 2(4): 347-359. doi: 10.3934/medsci.2015.4.347 |
[6] | Renjith Parameswaran Nair, Gulshan Sunavala-Dossabhoy . Promising Gene Therapeutics for Salivary Gland Radiotoxicity. AIMS Medical Science, 2016, 3(4): 329-344. doi: 10.3934/medsci.2016.4.329 |
[7] | Roberto Gutierrez, Yesenia Thompson, Timothy R. O’Connor . DNA direct repair pathways in cancer. AIMS Medical Science, 2018, 5(3): 284-302. doi: 10.3934/medsci.2018.3.284 |
[8] | Anuj A. Shukla, Shreya Podder, Sana R. Chaudry, Bryan S. Benn, Jonathan S. Kurman . Non-small cell lung cancer: epidemiology, screening, diagnosis, and treatment. AIMS Medical Science, 2022, 9(2): 348-361. doi: 10.3934/medsci.2022016 |
[9] | Federica Martorana, Paolo Vigneri, Stefano Sergio Cordio, Concetta Martines, Giuseppe Novello, Marco Maria Aiello, Roberto Bordonaro, Hèctor J. Soto Parra . Efficacy of A Fluoropyrimidine plus Mitomycin C in Pretreated Patients with Metastatic Colorectal Cancer Eligible for Regorafenib: A Retrospective Study. AIMS Medical Science, 2017, 4(4): 456-464. doi: 10.3934/medsci.2017.4.456 |
[10] | Nicole Lavender, David W. Hein, Guy Brock, La Creis R. Kidd . Evaluation of Oxidative Stress Response Related Genetic Variants, Pro-oxidants, Antioxidants and Prostate Cancer. AIMS Medical Science, 2015, 2(4): 271-294. doi: 10.3934/medsci.2015.4.271 |
Forkhead box Q1 (FOXQ1) is a nuclear transcription factor that controls the transcriptional activity of downstream genes to exert biological effects. Since its regulatory role in colorectal cancer (CRC) is unknown, we correlated RNA-seq and ATAC-seq sequencing data from the Cancer Genome Atlas Program (TCGA), the Gene Expression Omnibus (GEO), and other databases with FOXQ1 knockdown data from our group and normal DLD1-CRC cell lines. First, we analyzed FOXQ1 gene expression across multiple databases and datasets and found significant differences in FOXQ1 gene expression in most tumors. In addition, we performed an extension analysis of the FOXQ1 gene at different levels and found a close relationship between the FOXQ1 gene and immunity. Then, based on the FOXQ1 gene, we collected its target genes and obtained a total of 107 genes that met the regulatory trend. After a series of target gene analyses, we obtained three key genes (SERPINA1, MYL9, and CFTR). Moreover, an enrichment analysis, an independent prognostic analysis, and an immune-related analysis were performed around FOXQ1 and its target genes. After knocking down FOXQ1 protein in SW480 cells, the SERPINA1 and MYL9 proteins were significantly altered, though the CFTR protein was not significantly changed. These results provide bioinformatics data that support studying and exploring the FOXQ1 gene and its target genes in CRC.
Colorectal Cancer (CRC), a malignant gastrointestinal tumor caused by multiple pathogenic factors with poor prognosis, is the third most common tumor in the world, and has the fourth highest mortality rate among all cancers (after lung, liver, and stomach cancers) [1].
Forkhead box Q1 (FOXQ1) is a member of the Forkhead box (FOX) gene family. It is located at 6p23–25 and encodes a 403 amino acid protein, named FOXQl, which is a class of nuclear transcription factors stably bind to core elements such as GC boxes in the promoter regions of target genes and controls the transcriptional activity of downstream genes to exert biological effects [2]. Several studies have demonstrated that FOX transcription factors are associated with the initiation, progression, and metastasis of human cancers. In recent years, FOXQ1 has been connected with the promotion of tumor cell proliferation through the upregulation of downstream genes that encode proliferation-related proteins [3]–[6]. Christensen et al. observed that downregulation of FOXQ1 expression decreased the angiogenic ability of SW480 colorectal cancer cells by modulating Vascular endothelial growth factor (VEGF), which is an angiogenic activator secreted by tumor cells [7]–[8]. The FOXQ1 protein positively regulates Laminin Subunit Alpha 4 (LAMA4) expression, which promotes the development of micrometastasis and tumor reinitiation in vivo [9]. Down-regulation of FOXM1 expression by short interfering RNA (siRNA) decreases cell proliferation and metastasis. The tumor microenvironment can be modified by versican V1, a direct transcriptional target of FOXQ1, which has a role in promoting hepatocellular carcinoma cell metastasis and facilitating macrophage recruitment. During FOXQ1-regulated Epithelial-Mesenchymal Transition (EMT), the levels mesenchymal cell markers such as E-cadherin (E-cad) increase, whereas the levels epithelial cell markers such as Vimentin (VIM) decrease [10]. After a short hairpin RNA (shRNA) transfection with a eukaryotic expression vector (FOXQl-shRNA) that targeted the human FOXQl gene, the EMT of tumors is reversed. Several studies have shown that FOXQ1 promotes a resistance to chemotherapeutic agents [11]–[12]. Anuradha et al. reported that the inhibition of FOXQ1 facilitated isothiocyanate benzyl ester-mediated inhibition of cell migration [13]. Guidance for disease treatments may be obtained from studies on the mechanism of action of FOXQ1 in CRC.
An innovative epigenetic research method called the Assay for Transposase-Accessible Chromatin with high throughput sequencing (ATAC-seq) uses transposases to cleave nuclear chromatin regions open at a specific spatial and temporal locations. This obtains the regulatory sequences of all active transcripts in the genome at the specific spatial and temporal locations [14]–[15].
This study proposes to jointly apply RNA-seq and ATAC-seq to identify genes with altered expressions due to altered chromatin accessibility caused by a FOXQ1 knockdown, to identify FOXQ1-regulated target genes, to develop a prognostic model, and to provide additional theoretical guidance for the treatment of CRC with FOXQ1 as a target.
The TCGA-COADREAD dataset from the Cancer Genome Atlas Program (TCGA)database (https://portal.gdc.cancer.gov/) was used as the master dataset for the survival analysis and other primary analyses for the CRC samples in this study: 51 cases were normal samples; and 616 cases were cancer samples, where 590 samples had available Overall Survival (OS) survival data and 514 samples had available Disease-Free Survival (DFS) survival data. Hence, only the corresponding number of samples were used for analyses that involved OS and DFS survival-related analyses. Additionally, three additional datasets from the Gene Expression Omnibus (GEO)database (https://www.ncbi.nlm.nih.gov/gds) were used. The GSE164191 dataset was used for the expression analysis. The normal samples had 62 cases, while the cancer samples had 59 cases. The GSE87211 dataset was used for the expression analysis, where the normal samples had 106 cases and the cancer samples had 203 cases. The GSE44076 dataset was used for the expression analysis, where the normal samples had 50 cases and the cancer samples had 98 cases. The GSE44076 dataset was used for the expression analysis, where the normal samples had 50 cases and the cancer samples had 98 cases. Moreover, RNA-seq and ATAC-seq sequencing data for the FOXQ1 knockdown and normal DLD1-CRC cell lines from our group were used.
A pan-cancer analysis of the FOXQ1 gene expression was performed using the oncoming database. The differences in FOXQ1 gene expression of TCGA-CRC tumor tissues and normal tissues were analyzed using the Wilcoxon rank sum test. The Kaplan-Meier method was utilized to analyze the survival differences between high and low FOXQ1 expression groups. An analysis of the relationship between FOXQ1 and changes in the transcriptional mechanism was performed by measuring methylation differences, non-coding regulation, copy number alteration differences, mutation differences, and performing a transcription factor analysis. A target gene prediction for FOXQ1 was performed by differential gene expression and differential chromatin open region and association analyses. The Clusterprofiler package was used to analyze Gene Ontology (GO)/Kyoto Encyclopedia of Genes and Genomes (KEGG) enrichment of the FOXQ1 target genes. A Protein protein interaction (PPI)analysis, a survival analysis, an independent prognosis, and a drug prediction study were performed based on target genes.
Three pairs of shRNA interference sequences, named shRNA-A, shRNA-B and shRNA-C, and a control sequence, named shRNA-control, were designed and synthesized. the lenti-shFOXQ1 lentivirus was constructed by applying the pSPAX2 lentiviral packaging system of Addgene, and the PLKO.1-puro-shFOXQ1 plasmid was packaged to obtain the lenti-shFOXQ1 lentivirus. DLD1 and SW480 cells were infected and screened with a medium containing 1 µg/mL puromycin to obtain positive cells. Finally, a qRT-PCR and Western Blots were performed to verify the knockdown efficiency of the FOXQ1 gene.
SW480 cells were purchased from the Cell Resource Centre of Shanghai Academy of Biological Sciences, Chinese Academy of Sciences. Anti-FOXQ1(abcam, ab194564), Alpha 1 Antitrypsin Monoclonal antibody (SERPINA1; Proteintech, 66135-1-Ig), MYL9 Polyclonal antibody (Proteintech, 15354-1-AP), CFTR Monoclonal antibody (Proteintech, 66928-1-Ig), Anti-rabbit IgG, HRP-linked Antibody (CST, 7044s), Anti-mouse IgG, and HRP-linked Antibody (CST, 7076s) were utilized.
Figures 1A and 1B show the expression of the FOXQ1 gene in the pan-cancer analysis of different databases, including the Tumor Immune Estimation Resource (TIMER) (http://timer.cistrome.org/) and Gene Expression Profiling Interactive Analysis (GEPIA) databases (http://gepia.cancer-pku.cn/). The TIMER database contains a correlation analysis of immune infiltration, whereas the GEPIA2 database supports the analysis of immune infiltration. Additionally, the GEPIA2 database supports the analysis of specific cancer subtypes and comparisons between subtypes.
The FOXQ1 gene had significant expression changes in most tumors and could promote the immune infiltration of tumors, as shown by the expression of the FOXQ1 gene in the pan-cancer analysis of both databases. This suggests an association between the FOXQ1 gene and tumor formation.
We analyzed the expression of FOXQ1 in colorectal cancer in the TCGA database and the three GEO databases using a rank sum test and bee colony plot to visualize the results. The FOXQ1 gene showed significant upregulation in the cancer samples (Figure 1C–F).
We performed six survival analyses based on the OS and DFS data of the TCGA dataset, which was further divided into colon, rectal, and CRC. The alteration of FOXQ1 gene expression can significantly affect the OS and DFS states of colon, rectal, and CRC (Figure 2A).
We analyzed the genetic mutations of the FOXQ1 gene in CRC on the cbioportal website (https://www.cbioportal.org/) to study FOXQ1 gene mutations. Figure 2B shows that the FOXQ1 gene was mutated in TCGA CRC in only two samples, one with an amplification and one with a deletion. This indicates that the FOXQ1 gene has fewer mutations at the nucleic acid level.
There was a significant difference in the levels of 19 of the 28 cells between the FOXQ1 high and low expression groups (Figure 2C). Moreover, a Tracking of Indels by Decomposition (TIDE) analysis (Figure 2D) predicted the likelihood of a highly significant difference between the high and low FOXQ1 expression groups in terms of an immunotherapy response.
The FOXQ1 gene expression was higher in the DLD1 cell line compared to any other cell line from the group's previous study; therefore, a FOXQ1 knockdown was performed on the DLD1 cell line. We conducted a differential analysis between the DLD1-shControl and DLD1-shFOXQ1 groups, with the differential screening conditions set at a P value < 0.05 and a |log2FC| > 1. By counting, there were 346 significantly differentially expressed genes in the DLD1-shControl and DLD1-shFOXQ1 samples, where 215 were up-regulated genes and 131 were down-regulated genes (Figure 3A left). The heat map in Figure 3A right demonstrates the expression of the top 100 differentially expressed genes in the DLD1-shControl and DLD1-shFOXQ1 samples.
Combining RNA-seq data with the results of the ATAC-seq analysis allowed researchers to determine whether open chromatin areas either promoted or repressed downstream gene expression. The results are shown in Figure 3B. The analysis of the region of a greater chromatin opening in CDLD1-shFOXQ1 compared with DLD1-shCtrl using differential genes of the DLD1 RNA-seq data revealed that the differential open chromatin region has a facilitative function on gene regulation (Figure 3C left). Based on the analysis of the differential genes in the region of a diminished chromatin opening of DLD1-shFOXQ1 compared to the DLD1-shCtrl and DLD1 RNA-seq data, the differential open chromatin region has a repressive function on gene regulation (Figure 3C, right).
The genes regulated by the FOXQ1 gene, known as the target genes of the FOXQ1 gene, can be derived by combining the results from 3.1 and 3.2. Based on the ATAC-seq and RNA-seq regulatory trends, it is necessary to use the up-regulated genes in 3.1 to intersect with the genes in the region with an enhanced chromatin opening in 3.2, and the down-regulated genes in 3.1 to intersect with the genes in the region with a reduced chromatin opening in 3.2. Finally, 107 target genes of the FOXQ1 gene were obtained; in what follows, these genes will be referred to as target genes.
We enriched 107 genes, with a total of 129 terms, including the GO, KEGG, and Reactome databases; then, these genes were visualized using the network form (Figure 4A), where the top 20 are shown in Figure 4B. The DisGeNET database was also used to predict the disease target, and 199 results were enriched, with the top 20 shown in Figure 4C.
Using the STRING (https://string-db.org) website, we constructed PPI networks for 107 target genes to investigate whether there is a reciprocal relationship between the 107 target genes. The confidence level was set to 0.15 (Confidence = 0.15), and 17 discrete proteins were found, which resulted in a reciprocal network of 90 proteins. Meanwhile, using the above 90 nodes, the Minimal Common Oncology Data Elements (MCODE) was analyzed by setting Degree > 4, and a total of 5 MCODEs were obtained. Then, a KEGG enrichment analysis was performed for the genes of each MCODE; however, only the MCODE4 had enrichment in the results. Finally, by sorting the Maximum Clique Centrality (MCC), Neighborhood Component Centralit (MNC), Degree, and Closeness, we took the intersection of the genes obtained by each of these algorithms (Figure 4D). A total of 8 genes were obtained after counting: IGF1, EGR1, CFTR, BDNF, SERPINA1, IL1RN, SAA1, and MYL9.
We conducted a survival analysis based on the OS of the TCGA dataset for 8 key genes. After analysis, we obtained 3 key genes with significant differences in survival: SERPINA1, MYL9, and CFTR. Figure 5A shows the survival curves of these three genes, which serve as the key genes in this study.
Immune checkpoint inhibitors (ICI genes) play different essential roles in immune functions and have clinical implications in immunotherapy. Therefore, we evaluated the potential correlation between disease and the expression of immune checkpoint molecules.
We examined the expression of several key immune checkpoints (Figure 5B), particularly IDO1, PD-L1 (CD274)), PD-L2 (PDCD1LG2), TIM-3 (HAVCR2), TIGIT, PD-1 (PDCD1), LAG3, ICOS, and CD27. The whole spectrum of immune checkpoint molecules was observed in the high and low FOXQ1 expression groups. The expression of immune checkpoint molecules was lower in the high FOXQ1 expression group and all immune checkpoint molecules demonstrated significant differences between the high and low FOXQ1 expression groups.
To explore the independent prognosis of the expression model and clinicopathological factors, we performed an independent prognostic analysis of OS for the three key genes in the TCGA dataset and included the clinicopathological factors and expression data of the three key genes in the TCGA samples in the Cox analysis (Figure 5C).
Among the univariate Cox analysis results (Figure 5C Upper), the factors with P < 0.05 were included in the multifactor Cox analysis. A total of STAGE, M, T, N, age, and CF transmembrane conductance regulator (CFTR) were significant.
The factors that were significant under the univariate analysis were included in the multifactor Cox analysis (Figure 5C lower), and the results were obtained from the multifactor Cox analysis. A total of M, T, N, age, and CFTR appeared in the multifactor results, indicating that M, T, N, age, and CFTR are independent prognostic factors. This implies that, among the key genes, CFTR is an independent prognostic factor, while the remaining two are not.
The R's estimate package was used in the analysis of the immune score, stromal score, ESTIMATE score, and tumor purity for each sample. Then, the key genes were correlated (spearman) with the immune score, stromal score, ESTIMATE score, and tumor score, which were visualized as a heat map (Figure 5D).
After obtaining the key genes, we focused on which drugs would be affected by diagnostic markers and used the Comparative Toxicogenomics Database (CTD) database (http://ctdbase.org/) to predict the drug interactions for the 3 key genes and the FOXQ1 gene in human clinics. For a total of 126 drugs, we selected the drugs that had the relationship pairs of increases^expression and decreases^expression with the genes (Figure 5E).
To explore the expression of the three key gene proteins, we knocked down the FOXQ1 protein in SW480 cells and observed significant changes for the MYL9 and SERPINA1 proteins, but not for the CFTR protein (Figure 6). This indicates that, at the protein level, the MYL9 and SERPINA1 proteins are highly correlated with FOXQ1.
We first performed FOXQ1 gene expression analyses in various databases (TIMER, GEPIA) and datasets (TCGA, GSE164191, GSE87211, and GSE44076), and conducted six survival analyses in different diseases based on TCGAOS and DFS data. We further performed different levels of extension analyses for the FOXQ1 gene (SNP, CNV, ceRNA, TF network, etc.) and an immune correlation analysis, which included immune infiltration and TIDE. Then, we divided FOXQ1 into high and low expression groups for the rank sum test, where the results showed a strong correlation between the FOXQ1 gene and immunity.
We used the DLD1 cell line knockdown sequencing and ATAC-seq data to first calculate a total of 346 differential genes between the knockdown and non-knockdown groups of the DLD1 cell line. Then, we used ATAC-seq data to obtain the regions with an enhanced chromatin opening and with a reduced chromatin opening. We were finally able to obtain a list of up-regulated genes and regions with an enhanced chromatin opening and genes of down-regulated genes. The regions with a reduced chromatin opening were obtained by combining the knockdown sequencing data of the DLD1 cell line and ATAC-seq data. Finally, the DLD1 cell line knockdown sequencing and ATAC-seq data were combined to obtain a list of up-regulated genes and the genes in the regions with an enhanced chromatin opening. The down-regulated genes, the regions with a reduced chromatin opening, and the intersection were taken to obtain 107 genes that meet the regulatory trend, which were called the target genes of the FOXQ1 gene.
We performed several analyses for the target genes, including an enrichment analysis, a PPI analysis, and a survival analysis after obtaining the target genes. After a series of analyses, we obtained 3 genes—SERPINA1, MYL9, and CFTR—which were considered the key genes in this study. Then, an analysis of the key genes was performed at the immune level, where the results indicated some correlation between the key genes with immunity. Then, an independent prognostic analysis was performed, and the results showed that CFTR was an independent prognostic factor, while the remaining two were not. Finally, an extended analysis of the key genes was performed at the estimate and drug levels.
However, in the SW480 cells with a knockdown of FOXQ1, only the SERPINA1 and MYL9 proteins showed significant expression changes, while the CFTR protein showed no significant changes, which may be due to the fact that FOXQ1 affects the downstream regulation by directly controlling the expression of the SERPINA1 and MYL9 proteins (e.g., SERPINA1 is the inhibitor of serine proteases, which controls the activity of various proteases in the intestine) [16]. MYL9 is a myosin that controls intestinal peristalsis [17]. Moreover, FOXQ1 may be possible to control intestinal chloride and transmembrane substance transport through a direct interaction with CFTR rather than affecting protein expression [18].
The above analyses yielded three target genes of the FOXQ1 gene in CRC. An enrichment analysis, an independent prognostic analysis, and an immune-related analysis were performed around the FOXQ1 gene and its target genes, and the expression changes of the three key genes were preliminarily explored after knocking down the FOXQ1 protein, which provided bioinformatics data to support the research and exploration of the roles of the FOXQ1 gene and its target genes in CRC.
Yuxiang Zou, Jialong Qi and Hui Tang conceived and designed the study. Jialong Qi and Hui Tang were responsible for data analysis, and data interpretation. Yuxiang Zou wrote the article. Yuxiang Zou, Jialong Qi and Hui Tang revised the manuscript. All authors have read and agreed to the published version of the manuscript.
The authors declare they have not used Artificial Intelligence (AI) tools in the creation of this article.
[1] |
Mármol I, Sánchez-de-Diego C, Pradilla Dieste A, et al. (2017) Colorectal carcinoma: A general overview and future perspectives in colorectal cancer. Int J Mol Sci 18: 197. https://doi.org/10.3390/ijms18010197 ![]() |
[2] |
Bieller A, Pasche B, Frank S, et al. (2001) Isolation and characterization of the human forkhead gene FOXQ1. DNA Cell Biol 20: 555-561. https://doi.org/10.1089/104454901317094963 ![]() |
[3] |
Kaneda H, Arao T, Tanaka K, et al. (2010) FOXQ1 is overexpressed in colorectal cancer and enhances tumorigenicity and tumor growth. Cancer Res 70: 2053-2063. https://doi.org/10.1158/0008-5472.CAN-09-2161 ![]() |
[4] | Lin L, Miller CT, Contreras JI, et al. (2002) The hepatocyte nuclear factor 3 alpha gene, HNF3alpha (FOXA1), on chromosome band 14q13 is amplified and overexpressed in esophageal and lung adenocarcinomas. Cancer Res 62: 5273-5279. |
[5] |
Li J, Vogt PK (1993) The retroviral oncogene qin belongs to the transcription factor family that includes the homeotic gene fork head. P Natl Acad Sci Usa 90: 4490-4494. https://doi.org/10.1073/pnas.90.10.4490 ![]() |
[6] |
Koo CY, Muir KW, Lam EW (2012) FOXM1: From cancer initiation to progression and treatment. Biochim Biophys Acta-Gene Regul Mech 1819: 28-37. https://doi.org/10.1016/j.bbagrm.2011.09.004 ![]() |
[7] |
Christensen J, Bentz S, Sengstag T, et al. (2013) FOXQ1, a novel target of the Wnt pathway and a new marker for activation of Wnt signaling in solid tumors. PloS One 8: e60051. https://doi.org/10.1371/journal.pone.0060051 ![]() |
[8] |
Ellis LM, Hicklin DJ (2008) VEGF-targeted therapy: mechanisms of anti-tumour activity. Nat Rev Cancer 8: 579-591. https://doi.org/10.1038/nrc2403 ![]() |
[9] |
Ross JB, Huh D, Noble LB, et al. (2015) Identification of molecular determinants of primary and metastatic tumour re-initiation in breast cancer. Nat Cell Biol 17: 651-664. https://doi.org/10.1038/ncb3148 ![]() |
[10] |
Feng J, Zhang X, Zhu H, et al. (2012) FoxQ1 overexpression influences poor prognosis in non-small cell lung cancer, associates with the phenomenon of EMT. PloS One 7: e39937. https://doi.org/10.1371/journal.pone.0039937 ![]() |
[11] |
Meng F, Speyer CL, Zhang B, et al. (2015) PDGFRα and β play critical roles in mediating Foxq1-driven breast cancer stemness and chemoresistance. Cancer Res 75: 584-593. https://doi.org/10.1158/0008-5472.CAN-13-3029 ![]() |
[12] |
Wattenberg LW (1977) Inhibition of carcinogenic effects of polycyclic hydrocarbons by benzyl isothiocyanate and related compounds. J Natl Cancer Inst 58: 395-398. https://doi.org/10.1093/jnci/58.2.395 ![]() |
[13] |
Sehrawat A, Kim SH, Vogt A, et al. (2013) Suppression of FOXQ1 in benzyl isothiocyanate-mediated inhibition of epithelial-mesenchymal transition in human breast cancer cells. Carcinogenesis 34: 864-873. https://doi.org/10.1093/carcin/bgs397 ![]() |
[14] |
Pott S, Lieb JD (2015) Single-cell ATAC-seq: strength in numbers. Genome Biol 16: 172. https://doi.org/10.1186/s13059-015-0737-7 ![]() |
[15] | Buenrostro JD, Wu B, Chang HY, et al. (2015) ATAC-seq: A method for assaying chromatin accessibility genome-wide. Curr Protoc Mol Biol 10. https://doi.org/10.1002/0471142727.mb2129s109 |
[16] |
Collins CB, Aherne CM, Ehrentraut SF, et al. (2013) Alpha-1-antitrypsin therapy ameliorates acute colitis and chronic murine ileitis. Inflamm Bowel Dis 19: 1964-1973. https://doi.org/10.1097/MIB.0b013e31829292aa ![]() |
[17] |
Fournier N, Fabre A (2022) Smooth muscle motility disorder phenotypes: A systematic review of cases associated with seven pathogenic genes (ACTG2, MYH11, FLNA, MYLK, RAD21, MYL9 and LMOD1). Intractable Rare Dis Res 11: 113-119. https://doi.org/10.5582/irdr.2022.01060 ![]() |
[18] |
Thiagarajah JR, Verkman AS (2003) CFTR pharmacology and its role in intestinal fluid secretion. Curr Opin Pharmacol 3: 594-599. https://doi.org/10.1016/j.coph.2003.06.012 ![]() |