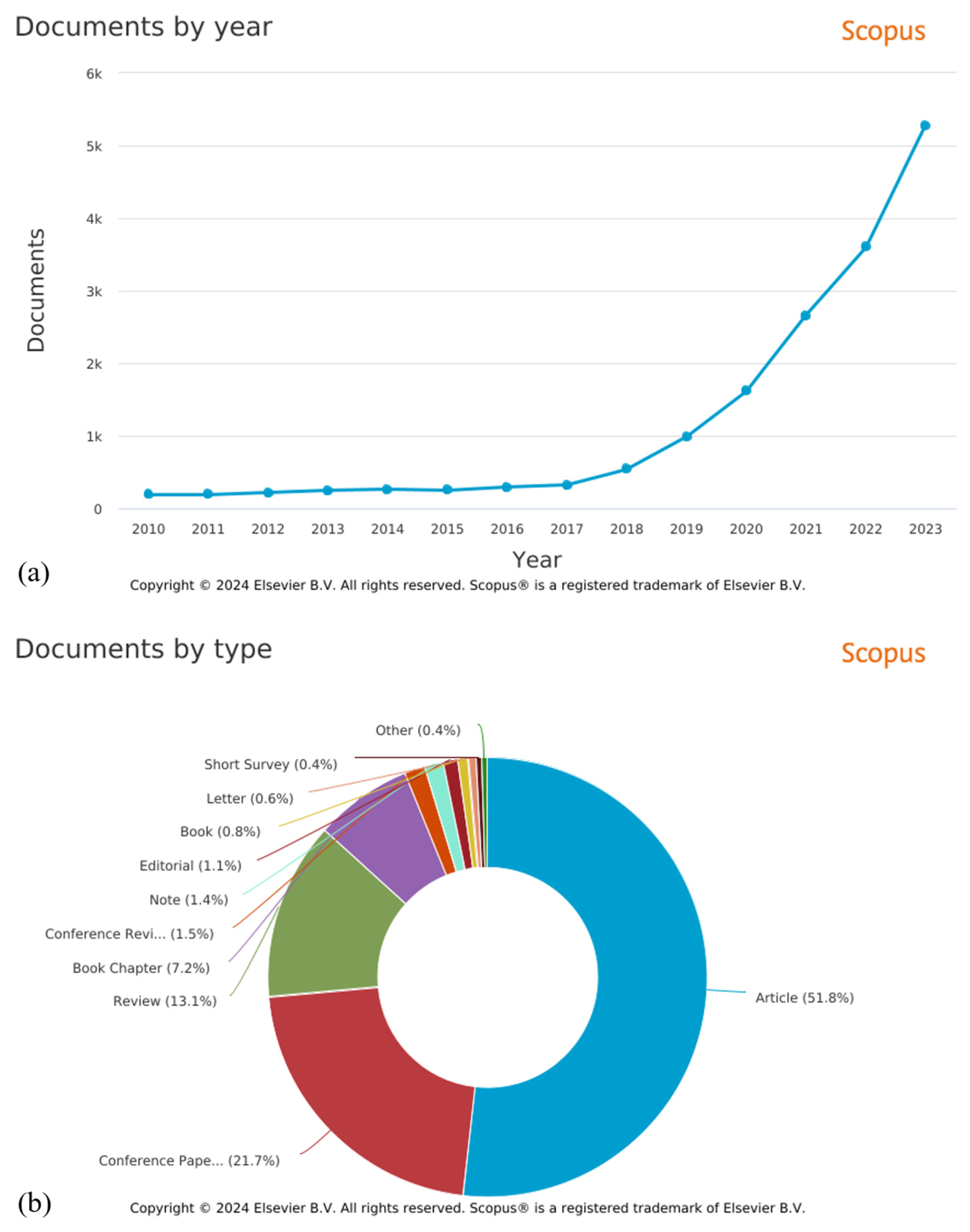
The integration of artificial intelligence (AI) into healthcare has ushered in transformative advancements. In diagnostics, AI has revolutionized precision by excelling in radiology, pathology, and novel approaches like liquid biopsy analysis. Personalized treatment plans benefit from AI's ability to tailor interventions, minimizing risks and maximizing therapeutic efficacy. Administrative efficiency sees notable improvements as AI automates tasks, optimizes resource allocation, and addresses scalability challenges. Ethical considerations, encompassing data privacy, algorithmic bias, and transparency, are crucial for responsible AI adoption. Guided by the fair principles, stakeholders can promote fairness, accountability, and transparency. Findings in this paper reveal AI's rapid advancements in healthcare enhance diagnostics, surgery, and patient care, yet reliance on its accuracy requires caution. The future of AI in healthcare relies on ongoing collaboration, research, and adherence to ethical frameworks, promising a patient-centered and equitable healthcare landscape.
Citation: Segun Akinola. Advancing healthcare with AI: designing frameworks for diagnostics, personalized treatment, and enhanced efficiency[J]. AIMS Medical Science, 2024, 11(3): 248-264. doi: 10.3934/medsci.2024019
[1] | Segun Akinola, Reddy Leelakrishna, Vijayakumar Varadarajan . Enhancing cardiovascular disease prediction: A hybrid machine learning approach integrating oversampling and adaptive boosting techniques. AIMS Medical Science, 2024, 11(2): 58-71. doi: 10.3934/medsci.2024005 |
[2] | Cassandra A DeMarshall, Abhirup Sarkar, Robert G Nagele . Serum Autoantibodies as Biomarkers for Parkinsons Disease: Background and Utility. AIMS Medical Science, 2015, 2(4): 316-327. doi: 10.3934/medsci.2015.4.316 |
[3] | Herbert F. Jelinek, Jemal H. Abawajy, David J. Cornforth, Adam Kowalczyk, Michael Negnevitsky, Morshed U. Chowdhury, Robert Krones, Andrei V. Kelarev . Multi-layer Attribute Selection and Classification Algorithm for the Diagnosis of Cardiac Autonomic Neuropathy Based on HRV Attributes. AIMS Medical Science, 2015, 2(4): 396-409. doi: 10.3934/medsci.2015.4.396 |
[4] | Giulio Filograna Pignatelli, Laith Khasawneh, Annasara Sorrentino, Alessandro Pacella, Andrea De Vito, Giampiero Neri . Stop-bang questionnaire: Practical approach to diagnosis of obstructive sleep apnea in roncopathic subjects. AIMS Medical Science, 2020, 7(3): 93-105. doi: 10.3934/medsci.2020009 |
[5] | Jonathan Kissi, Daniel Kwame Kwansah Quansah, Jonathan Aseye Nutakor, Alex Boadi Dankyi, Yvette Adu-Gyamfi . Telehealth during COVID-19 pandemic era: a systematic review. AIMS Medical Science, 2022, 9(1): 81-97. doi: 10.3934/medsci.2022008 |
[6] | Carlos Menéndez Villalva, Xose Luis Muiño López-Alvarez, Martín Menéndez Rodríguez, María José Modroño Freire, Olalla Quintairos Veloso, Lea Conde Guede, Sandra Vilchez Dosantos, Manuel Blanco Ramos . Blood Pressure Monitoring in Cardiovascular Disease. AIMS Medical Science, 2017, 4(2): 164-191. doi: 10.3934/medsci.2017.2.164 |
[7] | Tracey Cockerton Tattersall, Nadja Rolli, Martin Butwell . Integrative child psychotherapy: discussion of a common core and unified theory approach. AIMS Medical Science, 2024, 11(2): 181-209. doi: 10.3934/medsci.2024015 |
[8] | Valeria Paradies, Filippo Masi, Francesco Bartolomucci, Alfredo Marchese, Armando Liso, Fabrizio Resta, Stefano Favale, Martino Pepe . No Reflow-phenomenon: from Current State of the Art to Future Perspectives. AIMS Medical Science, 2015, 2(4): 374-395. doi: 10.3934/medsci.2015.4.374 |
[9] | David Petillo, Stephen Orey, Aik Choon Tan, Lars Forsgren, Sok Kean Khoo . Parkinsons Disease-related Circulating microRNA Biomarkers——a Validation Study. AIMS Medical Science, 2015, 2(1): 7-14. doi: 10.3934/medsci.2015.1.7 |
[10] | Herbert F. Jelinek, Jemal H. Abawajy, Andrei V. Kelarev, Morshed U. Chowdhury, Andrew Stranieri . Decision trees and multi-level ensemble classifiers for neurological diagnostics. AIMS Medical Science, 2014, 1(1): 1-12. doi: 10.3934/medsci.2014.1.1 |
The integration of artificial intelligence (AI) into healthcare has ushered in transformative advancements. In diagnostics, AI has revolutionized precision by excelling in radiology, pathology, and novel approaches like liquid biopsy analysis. Personalized treatment plans benefit from AI's ability to tailor interventions, minimizing risks and maximizing therapeutic efficacy. Administrative efficiency sees notable improvements as AI automates tasks, optimizes resource allocation, and addresses scalability challenges. Ethical considerations, encompassing data privacy, algorithmic bias, and transparency, are crucial for responsible AI adoption. Guided by the fair principles, stakeholders can promote fairness, accountability, and transparency. Findings in this paper reveal AI's rapid advancements in healthcare enhance diagnostics, surgery, and patient care, yet reliance on its accuracy requires caution. The future of AI in healthcare relies on ongoing collaboration, research, and adherence to ethical frameworks, promising a patient-centered and equitable healthcare landscape.
The integration of artificial intelligence (AI) into healthcare has ushered in a transformative era, particularly in diagnostic processes [1]. This paradigm shift is not merely technological but represents a redefinition of precision and efficiency in medical diagnostics. AI's influence on diagnostic precision has been profound, revolutionizing the methods healthcare professionals use to interpret medical data and make critical decisions [2]. In the dynamic landscape of healthcare, AI has emerged as a powerful ally, enhancing diagnostic capabilities across various medical domains [3]. The advent of advanced algorithms and machine learning techniques has significantly contributed to improving the accuracy and speed of diagnoses, ultimately leading to more effective patient outcomes [4]. The AI developments in the health field contribute to more research content over the years with an increase in publications. The statistics from Scopus in Figures 1a and b show the daily increase in the thread and the number of documents published. This shows how significantly AI in health is revolutionizing the world [4].
The planning methodology was employed as described by [5]. This methodology is designed to systematically map and categorize existing literature across various disciplines, providing a comprehensive overview of the topic (see Figure 2). It is particularly useful for identifying specific issues and contextualizing them within broader literature. Developed by Evidence for Policy & Practice Information Centre, this approach offers a transparent and structured way to support policymakers, practitioners, and researchers in identifying relevant questions. The goal of this review was to inform policy discussions and guide the development of better design practices and deployment strategies for AI in healthcare. We conducted a literature review using five key databases (Scopus, Google Scholar, PhilPapers, Web of Science, and PubMed) to employed broad terms like “health,” “AI,” “artificial intelligence,” and “machine learning” to capture relevant discussions on AI in healthcare, excluding general digital health topics. This framework categorizes ethical concerns into epistemic, normative, and overarching issues related to algorithmic decision-making. We examined these concerns at various levels of abstraction, including individual, interpersonal, group, institutional, sectoral, and societal levels. This multi-level analysis helped identify unique ethical issues beyond individual impacts, offering a comprehensive understanding of the ethical landscape in AI-driven healthcare.
AI's influence on diagnostic precision is palpable in various real-world applications, where it has demonstrated exceptional prowess. In radiology, for instance, AI algorithms excel in analyzing medical images, detecting subtle anomalies, and providing rapid and accurate insights. Diagnostic imaging, encompassing modalities such as X-rays, MRIs, and CT scans, has witnessed a transformative surge in efficiency due to AI's ability to decipher complex patterns and subtle abnormalities that might elude the human eye [6]–[8]. Pathology is another domain where AI has carved its niche. Machine learning models, when trained on vast datasets of histopathological images, can detect nuances indicative of diseases such as cancer with unprecedented accuracy [9],[10]. Figure 3 reveals the main domain in AI, as these applications not only quicken the diagnostic procedure but also contribute to more reliable outcomes. Moreover, AI's impact is not confined to traditional diagnostic methods; it extends to novel approaches such as liquid biopsy analysis [11]. By analyzing circulating biomarkers in bodily fluids, AI can detect early signs of diseases, offering a minimally invasive yet highly precise diagnostic avenue [12]. Large language models and AI enhance surgical precision through real-time data analysis, robotic assistance, and improved preoperative planning, optimizing outcomes. AI in healthcare includes personalized treatment plans and advanced imaging analysis, optimizing diagnostics and improving patient outcomes significantly.
Despite the remarkable progress, implementing AI in diagnostic processes is not without its challenges. The foremost concern revolves around the accuracy of AI algorithms [13]. While AI has demonstrated impressive diagnostic capabilities, ensuring the reliability of results across diverse patient populations remains a complex task. Variability in data quality, demographics, and underlying health conditions poses challenges in achieving universal accuracy [14]. Data quality stands out as a pivotal challenge in AI-driven diagnostics. The effectiveness of machine learning models deeply relies on the excellence and diversity of the datasets used for training [15]. Biases present in training data results, affecting the model's generalizability. Achieving a comprehensive and representative dataset that encompasses diverse demographic groups is crucial for overcoming this challenge [16]. Another critical aspect is the interpretability of AI-generated diagnoses. The “black box” nature of some advanced AI models can hinder healthcare professionals' understanding of the underlying decision-making process [17]. Overcoming this challenge is vital for building trust in AI-driven diagnostics and fostering collaboration between AI systems and medical practitioners. Additionally, the incorporation of AI into current healthcare infrastructure poses logistical challenges. Adapting legacy systems to accommodate AI technologies requires substantial investments and careful planning to ensure seamless integration without disruptions to existing workflows. In AI, biases commonly arise from two primary origins: the data employed in algorithm training (data bias) and the inherent design or learning devices of the algorithm (algorithmic bias). Nevertheless, in the healthcare domain, supplementary biases may surface due to the intricate nature of human interactions and decision-making processes [18]. These extra biases fall into dual categories: those stemming from interactions between AI and clinicians and those stemming from interactions between AI and patients [19]. A depiction of these biases is presented in Figure 4 [20], which shows different types of biases in AI comprising data bias, where the training data is not illustrative, algorithmic bias, where the algorithm itself may unintentionally favor certain outcomes, and interpretation bias, where the results may be misinterpreted due to preexisting beliefs or misunderstandings. Furthermore, AI healthcare systems can arise from skewed training data, leading to unequal treatment, misdiagnoses, and disparities in patient care outcomes. These biases can particularly affect marginalized groups, causing issues in fairness, accuracy, and trust in AI-driven healthcare solutions. The synergy between AI and diagnostic precision holds immense potential for the future of healthcare. As we navigate the challenges associated with implementation, it is crucial to acknowledge the transformative impact AI has had on improving diagnostic accuracy and efficiency. The ongoing collaboration between scientists, healthcare specialists, and policymakers is imperative for addressing challenges and unlocking the full possibility of AI in healthcare diagnostics. As AI continues to change, so too will its role in redefining this landscape of diagnostic precision, ultimately enhancing patient care and contributing to a healthier future.
Personalized medicine has emerged as a transformative method in healthcare, pointing to tailored medical care to individual characteristics, needs, and preferences [21]. The significance of personalized treatment plans lies in their potential to revolutionize patient outcomes by moving away from the old one-size-fits-all model. In this paradigm shift, artificial intelligence (AI) plays a pivotal role in optimizing healthcare delivery [22]. AI applications like IBM Watson Health, Tempus, and Path AI analyze patient data, including genomics, to recommend personalized cancer treatment plans and therapies. AI, with its ability to analyze vast quantities of data and identify involved patterns, holds the key to unlocking the full possibility of personalized treatment plans. By leveraging patient-specific information, including genetic, molecular, and clinical data, AI algorithms can produce insights that guide healthcare professionals in crafting tailored interventions [23]. This not only enhances the precision of diagnosis and treatment but also minimizes the risk of adverse reactions and maximizes the efficacy of therapeutic interventions. Furthermore, AI-driven predictive modeling contributes to proactive disease management, enabling early identification of potential health risks and preemptive interventions. This proactive approach not only improves patient results but also reduces the overall burden on healthcare systems. As this research moves into the era of personalized medicine, it becomes evident that AI is not just a tool but a crucial enabler in optimizing healthcare. By harnessing the power of AI, personalized treatment plans become more than a theoretical concept; they become a tangible reality, fostering a new era of patient-centered care that holds the promise of better health outcomes for individuals across diverse populations. The incorporation of AI has markedly reshaped the landscape of drug detection. Through the utilization of AI procedures, experts can now simulate with forecast communications between possible drugs with biological proteins [24]. Molecular docking, a method assessing how a drug particle difficulty to its board protein, forms a crucial part of this process. Complementing molecular cutting, AI-enhanced biological crescendos simulating the valued visions into the dynamic conduct of drug-protein developments per time. These imitations furnish academics with detailed information on the constancy with effectiveness of potential candidates, aiding in the selection of promising molecules for further testing. Additionally, AI models have played a pivotal role inside vaccine growth [25]. Employing progressive prediction methods, these copies precisely delineate pathological protein constructions then analyze possible epitopes that will trigger immune replies. With relying on these predictions, academics will meticulously project vaccines, eliciting precise and robust immune replies against besieged pathogens. As a result, this approaching has considerably condensed the timeline for emergent viable vaccine candidates associated to conventional approaches. Moderna and BioNTech highlighted rapid COVID-19 vaccine development advancements. The impact of AI on drug discovery extends to revolutionizing the prediction of drug-protein interactions, allowing researchers to design lead compounds more efficiently. Moreover, AI has streamlined the drug growth process by mechanizing tasks, ultimately dipping the cost with time related to preclinical and scientific trials. Frameworks for diagnostics, personalized treatment, and enhanced administrative efficiency in healthcare leverage advanced technologies to improve diagnostic accuracy, tailor therapies to individual patient needs, and streamline administrative processes. These frameworks integrate data analytics, machine learning, and AI to optimize healthcare delivery, ensuring better outcomes and resource management. The integration of AI into treatment decision-making brings forth a myriad of challenges that necessitate careful consideration. One projecting hurdle is the issue of data interoperability. Healthcare systems often store data in disparate formats, making seamless integration and exchange challenging. Achieving this is crucial for AI algorithms to draw meaningful insights from diverse datasets, ensuring comprehensive and accurate treatment recommendations. Moreover, the incorporation of AI in treatment decisions demands robust regulatory frameworks. The ethical use of patient data, algorithmic transparency, and accountability are essential aspects that require clear guidelines. The absence of standardized regulations poses a risk to patient privacy and may impede the widespread acceptance of AI in healthcare. As the healthcare landscape evolves with technological advancements, addressing these challenges becomes imperative to unlock the full potential of AI in treatment decision-making, fostering a balance between innovation and regulatory safeguards. There are several case studies that show successful implementation. The work in [26],[27] reveals that AI and ML in healthcare offer transformative potential but face challenges like privacy threats and algorithmic bias. Security measures must address data collection, model development, and implementation phases to ensure safe integration and maximize patient care benefits. Success stories like IBM Watson and Google DeepMind underscore the importance of adaptive regulations and interdisciplinary collaboration for responsible AI implementation in healthcare.
Administrative efficiency is a critical component of a well-functioning healthcare system, directly impacting the quality of patient care and overall operational effectiveness [28]. Modern countries like the USA have the best commitment for legislating AI (see Figure 5). Recognizing the significance of streamlined administrative processes, artificial intelligence (AI) emerges as a powerful tool with the potential to revolutionize healthcare management.
One of the main ways AI contributes to administrative efficiency in healthcare is through the automation of routine tasks. Mundane and time-consuming activities, such as appointment scheduling, billing, and data entry, can be efficiently handled by AI-powered systems [29]. This automation not only reduces the burden on administrative staff but also minimizes the likelihood of errors, ensuring accuracy in crucial operational processes. As a result, healthcare organizations can optimize resources, allowing human staff to focus on extra complex and patient-centric tasks that need empathy with critical thinking.
AI's influence on resource allocation is profound, leading to improved operational efficiency within healthcare systems. Examples of digital health companies are Teladoc, Omada Health, Livongo, HealthTap, and Doctor on Demand. By automating administrative tasks, organizations can redirect personnel to areas where their expertise is most valuable, thereby enhancing overall productivity. However, AI-driven projecting analytics facilitates proactive resource planning, ensuring that healthcare facilities are adequately staffed and equipped to meet fluctuating demands. This not only optimizes resource utilization but also donates to cost-effectiveness, a dangerous issue in the ever-evolving landscape of healthcare economics [30]–[32]. In addressing scalability challenges, AI brings adaptability to healthcare systems. The ability to handle increasing volumes of administrative tasks, such as patient data management and appointment scheduling, ensures that healthcare facilities can scale their operations to accommodate growing populations without compromising on efficiency. This scalability is particularly crucial in times of public health crises, where rapid and flexible responses are imperative [33]. As healthcare continues to evolve, the integration of AI in administrative processes stands as a promising avenue for achieving greater operational efficiency. By leveraging automation and predictive capabilities, AI not only optimizes resource allocation but also positions healthcare systems to meet the challenges of a dynamic and demanding environment, ultimately improving the quality with the accessibility of patient care.
Addressing ethical and legal considerations in the integration of AI into healthcare is imperative, as these have an important impact on the field. First, the question of answerability and obligation arises as AI systems undertake complex tasks, making it challenging to pinpoint responsibility for errors. Robust regulations are essential to define accountability and liability in healthcare AI requests [35],[36]. In response to the ethical, regulatory, safety, and quality challenges associated with AI in healthcare, [37] proposed a comprehensive governance framework called the AI Governance Model for Healthcare (AIGH) (see Figure 6). This model emphasizes four key principles: equity, openness, reliability, and responsibility.
Data privacy, a paramount concern, requires strong measures, adhering to values like data minimization and purpose curb. AI's potential to perpetuate biases in healthcare data necessitates guidelines ensuring fairness and accuracy. The “black-box” environment of many AI structures poses transparency and explain ability challenges, impacting healthcare professionals' trust. Consent for patient data usage in AI training is crucial, requiring legal specifications for different data types. Balancing innovation and regulatory oversight is essential, ensuring ethical standards without stifling progress [38]. As the healthcare industry embraces digital health technology to meet heightened patient demand, concerns about patient privacy and legal and ethical issues surrounding AI systems become prominent. The absence of comprehensive regulations raises issues like obligation attribution with confidentiality protection, creating ambiguity in accountability for AI actions. Safeguarding privacy is intertwined with autonomy and identity rights, demanding strict privacy measures and healthy security procedures. Concerns also arise regarding the group of patient data deprived of informed agreement, emphasizing the need for guidelines to govern AI's ethical and responsible use in healthcare. Responsible AI integration is crucial for maintaining trust, minimizing confidentiality invasion, and meeting investor expectations and rules. The American Medical Association's (AMA's) values for AI in healthcare emphasize comprehensive governance, transparency, privacy-centric design, and early identification of bias [39]. These principles aim to optimize AI benefits while reducing potential harms, providing an outline for responsible AI use in healthcare [40].
The rapid advancements in AI have transformed its utilization across various industries, particularly in healthcare. AI demonstrates ability in surgical measures, organizational tasks, diagnostics, imaging methods, medical education, and patient supervision. Collaboration between AI algorithms and clinicians is essential to improve health outcomes. Other factors promote the advancement of AI in healthcare [41]. A key driver is the perception that algorithms will offer additional objective, robust, and evidence-based clinical choices such as in diagnosis, prognosis, or treatment recommendations—than human healthcare practitioners [42]. This view is supported by evidence. Machine learning techniques, particularly ensemble and unsupervised methods [43], can analyze a much broader range of data than a healthcare supplier when making clinical decisions. This includes five of the seven scopes of healthcare information identified by the US Department of Health and Human Services: population with socioeconomic data, indication with current diagnosis data, treatment details, and results data with biological information. When designed with various epistemic considerations in mind, clinical algorithms can serve as digital companions. They help bridge the information gap between healthcare providers and patients by making information accessible to both, ensuring that the most informed decisions are made by the person entitled to make them [44]. This capability for evidence-based decision-making allows AI techniques to significantly enhance or even surpass human abilities in tasks such as: (a) risk factor analysis [45], (b) disease prediction [46], (c) infection prediction [47], (d) population health monitoring [48], (e) predicting adverse effects [49], (f) predicting outcomes and survival likelihood [50], and (g) analyzing electronic health records [51]. These capabilities, especially given their scalability in diagnosing or predicting outcomes for multiple individuals simultaneously, are significant. However, an almost unquestioning belief in the accuracy of AI health solutions is not without its flaws.
The integration of artificial intelligence (AI) in healthcare offers substantial prospective for enhancing diagnostic exactitude, optimizing treatment plans, and increasing operational efficiency. These advancements have the promise to improve patient results and alleviate the burden on healthcare systems globally. However, the adoption of AI in healthcare is not without challenges, and ethical and social reflections must be carefully addressed to ensure successful and equitable integration. This discussion will explore the benefits and complexities associated with AI in healthcare, highlighting both the transformative possibilities and the significant challenges.
AI's application in healthcare spans a wide range of functions, including surgical procedures, administrative tasks, diagnostics, imaging methods, medical education, and patient monitoring. AI algorithms can process and analyze vast amounts of data quickly with high accuracy, making them invaluable tools in clinical settings. For example, in diagnostics, AI can assist in analyzing medical images to detect diseases at early stages, potentially identifying subtle signs that might be overlooked by human eyes. This capability is crucial for early intervention and improved treatment outcomes. In addition, AI can enhance administrative efficiency by streamlining scheduling, resource allocation, and other routine tasks, allows healthcare professionals to focus more on direct patient care. In the surgical domain, AI-powered robotic systems can provide surgeons with enhanced precision and real-time data, reducing the risk of errors and improving the quality of procedures. Moreover, in medical education, AI can create realistic simulations for training, enabling students to hone their skills in a safe environment.
Despite these benefits, the collaboration between AI algorithms and clinicians remains essential to maximizing the potential of AI in healthcare. While AI can offer significant insights and support, it is not intended to replace human healthcare practitioners. Instead, it should be viewed as a complementary tool that enhances the capabilities of clinicians. AI can analyze patient data to identify risk factors and suggest treatment plans, but the ultimate decision-making responsibility must lie with the clinicians, who can incorporate their expertise and consider the patient's unique context and preferences. This partnership ensures that AI's contributions are used responsibly and ethically, prioritizing patient welfare.
However, the integration of AI into healthcare systems brings about several ethical and social challenges. One of the main concerns is data privacy and security. AI systems rely heavily on extensive datasets, often including sensitive personal health information. Ensuring the confidentiality and security of this information is paramount to maintaining patient trust and complying with legal regulations. Robust data governance frameworks must be established to protect against data breaches and misuse. Additionally, there is the issue of algorithmic bias. AI systems are trained on data, and if this data is biased or unrepresentative, the AI's decisions may also be biased, potentially exacerbating existing inequalities in healthcare access and treatment. For example, an AI system trained predominantly on data from a particular demographic may not perform as accurately for individuals from other backgrounds. To mitigate this risk, it is crucial to ensure that training datasets are diverse and representative of the entire population.
Accountability is another significant concern. As AI becomes more integrated into healthcare decision-making processes, it is vital to establish clear lines of responsibility. This includes determining who is accountable when an AI system provides incorrect diagnoses or recommendations. Developers, healthcare providers, and policymakers must collaborate to create ethical guidelines and legal frameworks that address these issues, ensuring that there is a clear understanding of where responsibility lies.
AI's role in supporting evidence-based decision-making is one of its most significant contributions to healthcare. AI can analyze a broader range of data than human practitioners, including demographic, socioeconomic, symptom, treatment, and outcome data, among others. This comprehensive analysis allows AI to provide more objective and evidence-based clinical decisions, such as in diagnosis, prognosis, and treatment recommendations. Machine learning techniques, particularly ensemble and unsupervised methods, can uncover patterns and correlations that may not be immediately apparent to human practitioners. This capability is invaluable in predicting disease risk, monitoring population health, and forecasting treatment outcomes with a high degree of accuracy. However, it is important to recognize that AI, despite its capabilities, is not infallible. Its use should always be as a support tool, with human oversight ensuring that final decisions are well-rounded and consider all relevant factors.
Looking forward, the role of AI in healthcare is likely to expand further. Future advancements may include more sophisticated algorithms capable of interpreting complex medical data and the expansion of personalized medicine designed to individual genetic profiles. Additionally, AI has the potential to improve global health by providing high-quality healthcare solutions in regions with limited access to medical professionals. To realize these benefits, continuous investment in research and development is crucial, as well as collaboration among all stakeholders, including healthcare providers, researchers, policymakers, and patients. It is essential to ensure that AI technologies are developing and deployed ethically, transparently, and fairly, addressing challenges such as data privacy, algorithmic bias, and accountability.
While the integration of AI in healthcare presents significant chances for improving patient results and efficiency, it also raises important ethical and social issues that must be addressed. By fostering a collaborative environment where AI and human expertise work together, and by tackling the ethical challenges head-on, we can harness the whole potential of AI to transform healthcare for the better.
To promote fairness in AI-driven healthcare, the FAIR (Fairness of Artificial Intelligence Recommendations) principles are recommended. These include safeguarding diverse and representative data, independent audits of AI algorithms, learning about AI biases for clinicians and patients, strengthening data privacy with security measures, creating liability with accountability frameworks, enhancing AI transparency with explainability, fostering collaboration, involving policymakers, engaging patients in AI development, and garnering support from professional associations.
Addressing AI bias and fairness gaps requires ongoing research efforts. Randomized controlled trials should explore AI's potential impact on diverse populations and demographic groups, monitoring models regularly to address emerging biases. Developing technologies for explain ability and transparency is crucial for nurturing trust among healthcare professionals and patients, ensuring the ethical deployment of AI in healthcare.
The successful integration of AI in healthcare requires a balanced and thoughtful approach, addressing ethical and social implications alongside technical proficiency. By adopting the FAIR principles and continually researching AI's impact, stakeholders can ensure equitable, responsible, and transparent deployment of AI technologies, ultimately contributing to a more efficient and patient-centered healthcare system.
The integration of artificial intelligence (AI) into healthcare has ushered in transformative advancements. In diagnostics, AI has revolutionized precision by excelling in radiology, pathology, and novel approaches like liquid biopsy analysis. Personalized treatment plans benefit from AI's ability to tailor interventions, minimizing risks and maximizing therapeutic efficacy. Administrative efficiency sees notable improvements as AI automates tasks, optimizes resource allocation, and addresses scalability challenges. Ethical considerations, encompassing data privacy, algorithmic bias, and transparency, are crucial for responsible AI adoption. Guided by the FAIR principles, stakeholders can promote fairness, accountability, and transparency. The future of AI in healthcare relies on ongoing collaboration, research, and adherence to ethical frameworks, promising a patient-centered and equitable healthcare landscape.
The author declares he has not used Artificial Intelligence (AI) tools in the creation of this article.
[1] | Qayyum MU, Sherani AMK, Khan M, et al. (2023) Revolutionizing healthcare: the transformative impact of artificial intelligence in medicine. B Inform 1: 71-83. |
[2] |
Gill AY, Saeed A, Rasool S, et al. (2023) Revolutionizing healthcare: how machine learning is transforming patient diagnoses-a comprehensive review of AI's impact on medical diagnosis. J World Sci 2: 1638-1652. https://doi.org/10.58344/jws.v2i10.449 ![]() |
[3] |
Panayides AS, Amini A, Filipovic ND, et al. (2020) AI in medical imaging informatics: current challenges and future directions. IEEE J Biomed Health Inform 24: 1837-1857. https://doi.org/10.1109/JBHI.2020.2991043 ![]() |
[4] |
Ngiam KY, Khor IW (2019) Big data and machine learning algorithms for health-care delivery. Lancet Oncol 20: e262-e273. https://doi.org/10.1016/S1470-2045(19)30149-4 ![]() |
[5] |
Grant MJ, Booth A (2009) A typology of reviews: an analysis of 14 review types and associated methodologies. Health Info Libr J 26: 91-108. https://doi.org/10.1111/j.1471-1842.2009.00848.x ![]() |
[6] |
Jayaraman PP, Forkan ARM, Morshed A, et al. (2020) Healthcare 4.0: a review of frontiers in digital health. Wires Data Min Knowl 10: e1350. https://doi.org/10.1002/widm.1350 ![]() |
[7] | Ali M (2023) A comprehensive review of AI's impact on healthcare: revolutionizing diagnostics and patient care. Bullet Jurnal Multidisiplin Ilmu 2: 1163-1173. |
[8] | Najjar R (2024) Digital frontiers in healthcare: integrating mHealth, AI, and radiology for future medical diagnostics, In: Heston, T.F., Doarn, C.E. Editors. A Comprehensive Overview of Telemedicine. London: IntechOpen Limited. https://doi.org/10.5772/intechopen.114142 |
[9] |
Feretzakis G, Juliebø-Jones P, Tsaturyan A, et al. (2024) Emerging trends in AI and radiomics for bladder, kidney, and prostate cancer: a critical review. Cancers 16: 810. https://doi.org/10.3390/cancers16040810 ![]() |
[10] |
Dlamini Z, Francies FZ, Hull R, et al. (2020) Artificial intelligence (AI) and big data in cancer and precision oncology. Comput Struct Biotechnol J 18: 2300-2311. https://doi.org/10.1016/j.csbj.2020.08.019 ![]() |
[11] |
Cucchiara F, Petrini I, Romei C, et al. (2021) Combining liquid biopsy and radiomics for personalized treatment of lung cancer patients. State of the art and new perspectives. Pharmacol Res 169: 105643. https://doi.org/10.1016/j.phrs.2021.105643 ![]() |
[12] |
Ginghina O, Hudita A, Zamfir M, et al. (2022) Liquid biopsy and artificial intelligence as tools to detect signatures of colorectal malignancies: a modern approach in patient's stratification. Front Oncol 12: 856575. https://doi.org/10.3389/fonc.2022.856575 ![]() |
[13] |
Stenzinger A, Alber M, Allgäuer M, et al. (2022) Artificial intelligence and pathology: from principles to practice and future applications in histomorphology and molecular profiling. Semin Cancer Biol 84: 129-143. https://doi.org/10.1016/j.semcancer.2021.02.011 ![]() |
[14] |
Aminizadeh S, Heidari A, Dehghan M, et al. (2024) Opportunities and challenges of artificial intelligence and distributed systems to improve the quality of healthcare service. Artif Intell Med 149: 102779. https://doi.org/10.1016/j.artmed.2024.102779 ![]() |
[15] |
Pasrija P, Jha P, Upadhyaya P, et al. (2022) Machine learning and artificial intelligence: a paradigm shift in big data-driven drug design and discovery. Curr Top Med Chem 22: 1692-1727. https://doi.org/10.2174/1568026622666220701091339 ![]() |
[16] |
Akinola S, Telukdarie A (2023) Sustainable digital transformation in healthcare: advancing a digital vascular health innovation solution. Sustainability 15: 10417. https://doi.org/10.3390/su151310417 ![]() |
[17] |
Hassija V, Chamola V, Mahapatra A, et al. (2024) Interpreting black-box models: a review on explainable artificial intelligence. Cogn Comput 16: 45-74. https://doi.org/10.1007/s12559-023-10179-8 ![]() |
[18] | Schwartz R, Vassilev A, Greene K, et al. (2022) Towards a standard for identifying and managing bias in artificial intelligence. NIST Special Publication. https://doi.org/10.6028/NIST.SP.1270 |
[19] |
Rajkomar A, Hardt M, Howell MD, et al. (2018) Ensuring fairness in machine learning to advance health equity. Ann Intern Med 169: 866-872. https://doi.org/10.7326/M18-1990 ![]() |
[20] |
Ueda D, Kakinuma T, Fujita S, et al. (2024) Fairness of artificial intelligence in healthcare: review and recommendations. Jpn J Radiol 42: 3-15. https://doi.org/10.1007/s11604-023-01474-3 ![]() |
[21] |
Aminizadeh S, Heidari A, Toumaj S, et al. (2023) The applications of machine learning techniques in medical data processing based on distributed computing and the internet of things. Comput Methods Programs Biomed 241: 107745. https://doi.org/10.1016/j.cmpb.2023.107745 ![]() |
[22] |
Sahu M, Gupta R, Ambasta RK, et al. (2022) Artificial intelligence and machine learning in precision medicine: a paradigm shift in big data analysis. Prog Mol Biol Transl Sci 190: 57-100. https://doi.org/10.1016.pmbts.2022.03.002 ![]() |
[23] |
Schork NJ (2019) Artificial intelligence and personalized medicine, In: Von Hoff, D., Han, H. Editors. Precision medicine in Cancer therapy. 1 Ed., Switzerland: Springer, Cham, 265-283. https://doi.org/10.1007/978-3-030-16391-4_11 ![]() |
[24] |
Wang H, Xiong R, Lai L (2023) Rational drug design targeting intrinsically disordered proteins. Wires Comput Mol Sci 13: p.e1685. https://doi.org/10.1002/wcms.1685 ![]() |
[25] |
Pun FW, Ozerov IV, Zhavoronkov A (2023) AI-powered therapeutic target discovery. Trends Pharmacol Sci 44: 561-572. https://doi.org/10.1016/j.tips.2023.06.010 ![]() |
[26] |
Chen Y, Esmaeilzadeh P (2024) Generative AI in medical practice: in-depth exploration of privacy and security challenges. J Med Internet Res 26: e53008. https://doi.org/10.2196/53008 ![]() |
[27] |
Lainjo B (2024) A meta-study on optimizing healthcare performance with artificial intelligence and machine learning. J Autonom Intell 7: 1535. https://doi.org/10.32629/jai.v7i5.1535 ![]() |
[28] |
Tao WJ, Zeng Z, Dang HX, et al. (2020) Towards universal health coverage: lessons from 10 years of healthcare reform in China. BMJ Glob Health 5: e002086. https://doi.org/10.1136/bmjgh-2019-002086 ![]() |
[29] |
Haleem A, Javaid M, Singh RP, et al. (2022) Medical 4.0 technologies for healthcare: features, capabilities, and applications. Int Things Cyber Physic Syst 2: 12-30. https://doi.org/10.1016/j.iotcps.2022.04.001 ![]() |
[30] |
Wamba-Taguimdje SL, Wamba SF, Kamdjoug JRK, et al. (2020) Influence of artificial intelligence (AI) on firm performance: the business value of AI-based transformation projects. Bus Process Manag J 26: 1893-1924. https://doi.org/10.1108/BPMJ-10-2019-0411 ![]() |
[31] | Chowdhury S, Dey P, Joel-Edgar S, et al. (2023) Unlocking the value of artificial intelligence in human resource management through AI capability framework. Hum Resour Manag Rev 33: 100899. https://doi.org/10.1016/j.hrmr.2022.100899 |
[32] |
Akindote OJ, Adegbite AO, Omotosho A, et al. (2024) Evaluating the effectiveness of it project management in healthcare digitalization: a review. Int Med Sci Res J 4: 37-50. https://doi.org/10.51594/imsrj.v4i1.698 ![]() |
[33] | Rodrigues JJPC (2009) Health information systems: concepts, methodologies, tools, and applications: concepts, methodologies, tools, and applications. Igi Global. https://doi.org/10.4018/978-1-60566-988-5 |
[34] | World Health Organization (2021) Ethics and governance of artificial intelligence for health: WHO guidance. Available from: https://www.who.int/publications-detail-redirect/9789240029200 |
[35] |
Carter SM, Rogers W, Win KT, et al. (2020) The ethical, legal and social implications of using artificial intelligence systems in breast cancer care. Breast 49: 25-32. https://doi.org/10.1016/j.breast.2019.10.001 ![]() |
[36] |
Smith H (2021) Clinical AI: opacity, accountability, responsibility and liability. AI Soc 36: 535-545. https://doi.org/10.1007/s00146-020-01019-6 ![]() |
[37] |
Reddy S, Allan S, Coghlan S, et al. (2020) A governance model for the application of AI in health care. J Am Med Inform Assoc 27: 491-497. https://doi.org/10.1093/jamia/ocz192 ![]() |
[38] |
Williamson SM, Prybutok V (2024) Balancing privacy and progress: a review of privacy challenges, systemic oversight, and patient perceptions in AI-driven healthcare. Appl Sci 14: 675. https://doi.org/10.3390/app14020675 ![]() |
[39] |
Ali A, Al-Rimy BAS, Tin TT, et al. (2023) Empowering precision medicine: unlocking revolutionary insights through blockchain-enabled federated learning and electronic medical records. Sensors 23: 7476. https://doi.org/10.3390/s23177476 ![]() |
[40] |
Floridi L, Cowls J, Beltrametti M, et al. (2018) AI4People—an ethical framework for a good AI society: opportunities, risks, principles, and recommendations. Minds Mach 28: 689-707. https://doi.org/10.1007/s11023-018-9482-5 ![]() |
[41] |
Bhidayasiri R, Goetz CG (2024) Embracing the promise of artificial intelligence to improve patient care in movement disorders, In: Bhidayasiri, R., Maetzler, W. Editors. Handbook of Digital Technologies in Movement Disorders. 1 Ed., Massachusetts: Academic Press, 11-23. https://doi.org/10.1016/B978-0-323-99494-1.00015-0 ![]() |
[42] | Kalmady SV, Paul AK, Narayanaswamy JC, et al. (2022) Prediction of obsessive-compulsive disorder: importance of neurobiology-aided feature design and cross-diagnosis transfer learning. Biol Psychiatry Cogn Neurosci Neuroimaging 7: 735-746. https://doi.org/10.1016/j.bpsc.2021.12.003 |
[43] |
Harerimana G, Jang B, Kim JW, et al. (2018) Health big data analytics: a technology survey. IEEE Access 6: 65661-65678. https://doi.org/10.1109/ACCESS.2018.2878254 ![]() |
[44] |
Morley J, Floridi L (2020) An ethically mindful approach to AI for health care. Lancet 395: 254-255. https://doi.org/10.1016/S0140-6736(19)32975-7 ![]() |
[45] |
Morley J, Floridi L (2021) How to design a governable digital health ecosystem, In: Cowls, J., Morley, J. Editors. The 2020 Yearbook of the Digital Ethics Lab. 1 Ed., Netherland: Springer, Cham, 69-88. https://doi.org/10.1007/978-3-030-80083-3_8 ![]() |
[46] |
Cleret de Langavant L, Bayen E, Yaffe K (2018) Unsupervised machine learning to identify high likelihood of dementia in population-based surveys: development and validation study. J Med Internet Res 20: e10493. https://doi.org/10.2196/10493 ![]() |
[47] |
Moscoso A, Silva-Rodríguez J, Aldrey JM, et al. (2019) Prediction of Alzheimer's disease dementia with MRI beyond the short-term: implications for the design of predictive models. Neuroimage Clin 23: 101837. https://doi.org/10.1016/j.nicl.2019.101837 ![]() |
[48] |
López-Martínez F, Núñez-Valdez ER, Gomez JL, et al. (2019) A neural network approach to predict early neonatal sepsis. Comput Electr Eng 76: 379-388. https://doi.org/10.1016/j.compeleceng.2019.04.015 ![]() |
[49] | Morley J, Machado C, Burr C, et al. (2019) The debate on the ethics of AI in health care: a reconstruction and critical review. Available at SSRN 3486518. https://doi.org/10.2139/ssrn.3486518 |
[50] | Morley J, Machado CCV, Burr C, et al. (2021) The ethics of AI in health care: a mapping, In: Floridi, L., Editor. Ethics, Governance, and Policies in Artificial Intelligence. 1 Ed., Netherland: Springer, Cham, 313-346. https://doi.org/10.1007/978-3-030-81907-1_18 |
[51] |
Karimian G, Petelos E, Evers SMAA (2022) The ethical issues of the application of artificial intelligence in healthcare: a systematic scoping review. AI Ethics 2: 539-551. https://doi.org/10.1007/s43681-021-00131-7 ![]() |
1. | Rocco Salvatore Calabrò, Sepehr Mojdehdehbaher, AI-Driven Telerehabilitation: Benefits and Challenges of a Transformative Healthcare Approach, 2025, 6, 2673-2688, 62, 10.3390/ai6030062 |